Special Issue
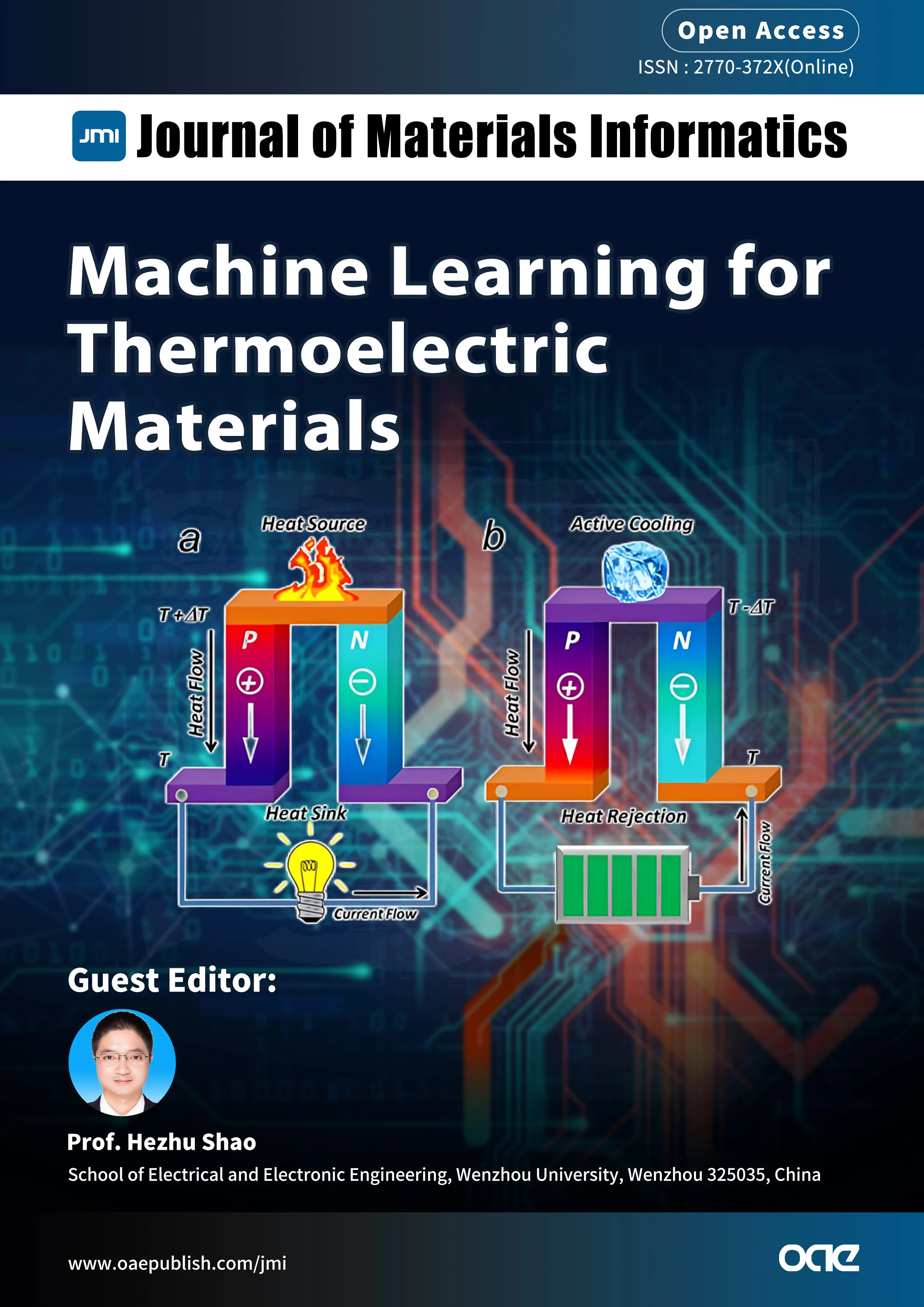
Topic: Machine Learning for Thermoelectric Materials
A Special Issue of Journal of Materials Informatics
ISSN 2770-372X (Online)
Submission deadline: 31 Mar 2025
Guest Editor(s)
Special Issue Introduction
Thermoelectric (TE) materials can directly convert energy between heat and electricity and have been widely applied in power generation and refrigeration. Their performance could be quantified by the figure of merit, ZT = S2σT/κ, where S, κ, σ, and T represent the Seebeck coefficient, thermal conductivity, electrical conductivity, and absolute temperature, respectively. S2σ is the power factor, and thermal conductivity κ comprises the carriers (κe) and lattice (κL) contributions. Generally, the complex coupling among these coefficients, such as S, κ, and σ, hinders significant improvements of TE performance in materials.
Throughout the centuries-long history of human scientific development, three research paradigms have emerged: experimental, theoretical, and computational. However, in complex systems such as biology, astronomy, and materials, the intricate interactions and numerous variables greatly limit the effectiveness of theoretical and computational research models. This limitation necessitates combining big data and artificial intelligence (AI), known as the “fourth paradigm”. With the advancement of big data and AI technologies, the material genome project research, characterized by high-throughput experiments, high-throughput computing, and AI big data analysis, has shown astonishing advantages in various materials fields. The fourth paradigm of materials science relies on machine learning to generate and process massive amounts of data, thus receiving growing attention. Its application in the research and development of new TE materials is expected to greatly accelerate its application and enhance our understanding of the fundamental mechanisms of the electronic and phonon transport properties in materials.
This Special Issue aims to provide a comprehensive understanding of the most recent advancements, challenges, and future directions in TE materials, emphasizing the application of machine learning methods.
It will feature original research articles, reviews, and perspectives from leading experts in TE materials and machine learning. Topics covered in this Special Issue include, but are not limited to:
1. Development of high throughput experimental/computational methods for establishing high-fidelity databases of TE materials;
2. Machine learning-based approaches for the design and optimization of TE materials;
3. Application of machine learning or machine learning-assisted simulations in predicting mechanical, heat transport, and electronic transport in TE materials;
4. Advanced machine learning techniques for characterizing the structure, defects, electrical, and heat transport properties of TE materials and for optimizing the manufacturing and service processes for TE devices.
Throughout the centuries-long history of human scientific development, three research paradigms have emerged: experimental, theoretical, and computational. However, in complex systems such as biology, astronomy, and materials, the intricate interactions and numerous variables greatly limit the effectiveness of theoretical and computational research models. This limitation necessitates combining big data and artificial intelligence (AI), known as the “fourth paradigm”. With the advancement of big data and AI technologies, the material genome project research, characterized by high-throughput experiments, high-throughput computing, and AI big data analysis, has shown astonishing advantages in various materials fields. The fourth paradigm of materials science relies on machine learning to generate and process massive amounts of data, thus receiving growing attention. Its application in the research and development of new TE materials is expected to greatly accelerate its application and enhance our understanding of the fundamental mechanisms of the electronic and phonon transport properties in materials.
This Special Issue aims to provide a comprehensive understanding of the most recent advancements, challenges, and future directions in TE materials, emphasizing the application of machine learning methods.
It will feature original research articles, reviews, and perspectives from leading experts in TE materials and machine learning. Topics covered in this Special Issue include, but are not limited to:
1. Development of high throughput experimental/computational methods for establishing high-fidelity databases of TE materials;
2. Machine learning-based approaches for the design and optimization of TE materials;
3. Application of machine learning or machine learning-assisted simulations in predicting mechanical, heat transport, and electronic transport in TE materials;
4. Advanced machine learning techniques for characterizing the structure, defects, electrical, and heat transport properties of TE materials and for optimizing the manufacturing and service processes for TE devices.
Keywords
Thermoelectric materials, computational methods, machine learning, high throughput experimental
Submission Deadline
31 Mar 2025
Submission Information
For Author Instructions, please refer to https://www.oaepublish.com/jmi/author_instructions
For Online Submission, please login at https://oaemesas.com/login?JournalId=JMI&SpecialIssueId=JMI240529
Submission Deadline: 31 Mar 2025
Contacts: Linda Cui, Assistant Editor, Editor@jmijournal.com
Published Articles
Coming soon