Electroencephalogram (EEG) network-level excitation-inhibition in GRIN2B-related neurodevelopmental disorders: a pilot case-control series
Abstract
Background: Loss of function (LoF) variants in GRIN2B lead to neurodevelopmental delay, which is expected to be mediated by alterations in the excitation-inhibition (E/I) balance resulting from reduced activity of the N-Methyl-D-Aspartate receptor. To test this hypothesis, we use quantitative electroencephalogram to provide insights into network-level estimates of the excitation-inhibition ratio. Methods: a single-center, case-control pilot study was conducted. Eyes-open rest EEG recordings were obtained from children with GRIN2B LoF variants (n = 5, aged
Keywords
INTRODUCTION
Glutamate Receptor Ionotropic N-Methyl-D-Aspartate or GRIN-related disorder encompasses a spectrum of neurodevelopmental disorders. Within this family of disorders, about 400 individuals carry pathogenic GRIN variants (https://www.grin-database.de/); GRIN2B neurodevelopmental disorder (GRIN2B-NDD) is a rare genetic encephalopathy. About 120 of these individuals entail mutations in GRIN2B. Although GRIN prevalence is underestimated and patients underdiagnosed, the diagnostic frequency of GRIN2B-NDD ranges between 0.19-0.22 in a patient group with NDD and epilepsy[1,2].
GRIN2B-NDD is caused by mutations in the GRIN2B gene encoding for the GluN2B subunit of the
Pathophysiology
The vast majority of excitatory synapses in the brain use glutamate, the main excitatory amino acid neurotransmitter, for neuronal communication. Glutamate functionally separates transmembrane receptors into two categories of receptors, namely the metabotropic and ionotropic glutamate receptors.
GRIN-NDD is related to ionotropic glutamate receptors and has seven GRIN genes, four of which show pathogenic variants: GRIN1, GRIN2A, GRIN2B and GRIN2D, all encoding for NMDAR subunits[5]. GRIN2B disturbances due to the impaired ionotropic glutamate NMDAR might markedly affect critical steps of neuronal, synaptic, and brain circuitry development[6]. More specifically, this variant with impaired glutamate expression causes abnormal synaptogenesis with an imbalance between excitatory and inhibitory currents - key factors in brain development, learning, memory, and other higher cognitive functions. GRIN2B-NDD seems to converge on a disturbed balance between excitatory and inhibitory (E/I) activities in neuronal networks in the brain, which occurs in early postnatal stages[7-10].
The newly developed functional E/I ratio (fE/I) is a promising marker for studying mechanistic disruptions in the micro-level regulation of E/I balance. This includes molecular, cellular, and local circuit disturbances and how these disruptions affect or trigger shifts in spontaneous brain activity and subsequent brain function. With respect to the latter, the functional E/I balance is seen as a proxy for the weighted “network” average of neural inputs and outputs, which determines elements of brain states[11].
This measure is grounded in the concept of criticality, which states that networks tend to function most efficiently when organized between order and disorder. Computational modeling has shown that the network-level E/I balance in the brain subserves criticality, thereby preserving information processing[11-13]. This criticality-based measure of fE/I enables inferences about different phases: the sub-critical phase, where the network operates in a low-activity phase, indicative of increased inhibition that impairs information transfer; the super-critical phase, characterized by excessive excitation, which can be associated with conditions such as epilepsy; and the balanced phase, where the E/I state is poised between these two extremes. Through this balance, neuronal oscillations emerge as spontaneous brain activity that effectively propagates, synchronizes, and integrates neural activity to form efficient neuronal networks[14,15]. The fE/I complements other measures sensitive to changes in E/I and critical brain dynamics, such as the spectral EEG power and the detrended fluctuation analysis (DFA) of long-range temporal correlations (LRTC)[16]. The E/I balance is considered the main mechanism regulating the strength of LRTC subserving amplitude fluctuations in neuronal oscillations. The DFA computes long-range correlations in the temporal structure of signals. This approach provides insights into the balance between network stability and flexibility, which is necessary for complex information processing. The clinical relevance of E/I imbalances, as explored through changes in fE/I, DFA, and spectral power, is supported by various NDD studies. In patients with autism spectrum disorder (ASD), E/I imbalances were indexed by large fE/I variability and increased DFA values compared to healthy controls, with the fE/I marker even applicable in distinguishing EEG abnormalities among patients[11]. Additionally, these markers were responsive to pharmacological interventions[11,17]. In the monogenetic disorder of tuberous sclerosis complex (TSC), characterized by brain tubers, intellectual disability, ASD-related behavior and epilepsy, patients showed notably lower fE/I than controls, indicating a low-critical or inhibition-dominated network[18]. In the syntaxin-binding protein 1 (STXBP1) syndrome, a rare genetic NDD characterized by a spectrum of severe developmental delay, intellectual disability, and early onset epilepsy, low fE/I values were accompanied with higher DFA values but also slowing of activity as expressed by increased low-frequency power. These findings were interpreted as inhibition-dominated network dynamics[19].
Since LoF mutations in GRIN2B result in NMDAR dysfunction with E/I imbalances, leading to the clinical phenotype observed in previous NDD, it is essential to understand these E/I changes. Here, we apply quantitative EEG (qEEG) such as fE/I, DFA, and spectral power in a cohort of underaged GRIN2B-NDD patients, comparing them to age- and gender-matched typically developing controls (TDC). The aim is to investigate whether impaired NMDAR signaling related to GRIN2B shifts E/I balance at the network level, as indicated by qEEG markers. We hypothesize that qEEG biomarkers will show alterations in patients with LoF mutations, revealing impairments indicative of neurodevelopmental delay, such as E/I imbalances with lower fE/I values, higher DFA values, and increased low-frequency power, suggesting a slowing of activities.
METHODS
Participants
This study was a single-center, case-control study performed at the Emma Center for Personalized Medicine (ECPM), Amsterdam UMC, the Netherlands. We included pediatric patients with a GRIN2B LoF variant who participated in a placebo-controlled, randomized n-of-1 trial assessing the effect of L-serine (2022.0271-NL80290.018.22)[4,20], with one patient participating in an open-label format which included a baseline EEG measurement. In this study, the effects of L-Serine are beyond the scope of the paper and will be reported elsewhere. Patients were eligible when they were between 36 weeks of gestational age and 18 years and had a pathogenic LoF GRIN2B mutation. Exclusion criteria were ingestion of L-serine within 30 days prior to enrollment and treatment with another investigational product within 30 days prior to enrollment. In addition, 35 children served as an age- and gender-matched typically developing group. Written informed consent was obtained from the legal guardians of all participants, as the participants were not capable of providing consent themselves prior to prospectively performed measurements. The study was approved by the Ethics Review Committee and conducted in accordance with the provisions of the Declaration of Helsinki and good clinical practice.
EEG acquisition
EEG recordings were acquired during the resting state with eyes open, lasting between 230-600 s for the patients and 300 s for TDC. High-density 128-channel HydroCel Geodesic with the NetAmps400 amplifier (Magstim-Electrical Geodesics Inc.) was used. The sampling rate was 1,000 Hz, with the reference electrode placed at Cz, positioned between CPz and Pz. Electrode impedances were kept below 100 KΩ.
EEG preprocessing
EEG preprocessing was done with the Neurophysiological Biomarker Toolbox (NBT) based on MNE-Python[21]. Raw continuous EEG recordings were down-sampled to 250 Hz and subsequently notch- (50 Hz) and bandpass-filtered for 1-45 Hz using a Finite Impulse Response filter with a Hamming window. Bad electrodes with excessive noise or flat signals were automatically detected by using the Random Sample Consensus algorithm as implemented in MNE. EEG signals from the identified bad electrodes were interpolated with a spherical spline using all electrodes. To remove transient and large amplitude artifacts, the artifact subspace reconstruction algorithm implemented in MNE was applied, which detected and rejected artifacts automatically, followed by the user's visual screening[22]. Signals were re-referenced to the average of all electrodes. Eye movements/blinks, heartbeat and muscle-related artifacts were visually inspected and projected from the EEG signals using independent component analysis (Infomax) algorithm. On average, five independent components (min-max: 1-8) were removed, and 10 bad electrodes were identified and interpolated per session (min-max = 5-17). After cleaning, the signal duration was between 30-290 s.
Quantitative EEG measures
Spectral power
To investigate the absolute and relative power, patients with a GRIN2B LoF variant were compared to gender- and age-matched controls (TDC), and the spectral power was computed for each individual cortical patch. Instead of the traditional canonical five frequency bands (delta, theta, alpha, beta, and gamma), high-frequency resolution analyses were performed. Eleven narrow frequency bins from 1-45 Hz (1.0-4.0;
Detrended fluctuation analysis
DFA research has shown that the temporal structure of ongoing oscillations follows a power-law distribution, also defined as scale-free oscillations, which characterizes long-range temporal correlations (LRTC). The DFA exponent reflects the slope, or the temporal structure based on the mean variability in a signal over several different time scales, also known as the mean fluctuation function. This function estimates the power-law decay or LRTC within the signal. DFA is performed on the amplitude envelope of the bandpass filtered signal (see for technical details[12,23]), whereas the exponent is defined as the least-squares linear fit of mean fluctuation over timescale on a log-log scale. A DFA exponent of 0.5 indicates no autocorrelations, while values between 0.5-1 indicate positive autocorrelations, reflecting the presence of LRTC.
fE/I
To investigate the EEG-derived E/I ratio of GRIN2B patients compared to TDC, the fE/I algorithm from the critical oscillations (CROS) model of ongoing neuronal activity was derived. This computational model asserts strong associations between E/I ratio on a structural level, amplitude, and DFA component of the amplitude modulation or LRTC of oscillations. The fE/I can be estimated from the covariance of the amplitude envelope and the normalized fluctuation function which correlates strongly with the DFA exponent. Stable estimates of fE/I can be obtained from a few seconds of preprocessed EEG signals[11]. In this study, the computation of fE/I was performed in the signal of each cortical patch in the above-mentioned 11 frequency ranges. FE/I < 1 indicates inhibition-dominated networks, fE/I > 1 reflects excitation-dominated networks, while fE/I ≈ 1 indicates a balanced E/I network (see for technical details:[23]).
Source space reconstruction
Electrode-level EEG time series were source-modelled using the L2 minimum norm estimation implemented by MNE-Python to obtain cortical surface current estimates. To construct the boundary element head model, the FreeSurfer average brain template was implemented for the forward operator for the source modeling[24-26]. The time series of the source vertices was further collapsed into 68 cortical patches on the Desikan Killiany Atlas[27]. Next, the EEG features (absolute and relative power, DFA, and fE/I) were computed for each cortical patch and averaged across cortical brain regions retrieved from patches subserving frontal, temporal, parietal, cingulate and insular regions (See for all details[28]).
Statistical tests
Statistical analyses of the qEEG biomarkers on source space were performed for each cortical patch and each frequency bin. Because of the non-normal distribution of the data due to the small sample size, and to circumvent results due to chance, age, or sex, we performed bootstrapping analyses for the frequency spectrum at the source space level. Each patient was tested against their age- and gender-matched controls. Paired comparisons at the source level were performed separately within the bootstrapping analyses for these values. Resampling with replacement for each patient-control group at the source level was utilized to estimate the statistical power of the observed paired mean difference between patients and controls. Resampling was performed 1,000 times, and in each iteration, the paired mean difference was computed to create the distribution of sampling mean differences for each level. The P-value was calculated as the proportion of resampled absolute paired mean differences that were greater than the observed absolute paired mean difference, and the significance level was set at P < 0.05. We used the false discovery rate (FDR) correction (q = 0.05) for multiple comparisons across each level, so for the frequency bins and brain regions. The resampling procedure was implemented in a custom-made Python script.
RESULTS
EEG recordings of five GRIN2B patients (aged 3.0 to 12.7 years, 2 females) were compared to a TDC group of 35 children (aged 4.3 to 12.7 years, 15 females). The GRIN2B patient group consisted of different pathogenic de novo mutations, which all resulted in missense mutations and deletions impairing NMDA receptor activity [Table 1]. The patient phenotype is characterized by mild to severe developmental delay, communication deficits, and motor impairments with varying degrees of severity. None of the carriers showed a history of seizures or epilepsy [Table 1]. The neurotypical group consisted of 35 children and were age- and gender-matched to the individual patients for the bootstrap analyses (The distribution of matched neurotypical participants was as follows: patient 1 (n = 3), patient 2 (n = 7), patient 3 (n = 8), patient 4 (n = 9), and patient 5 (n = 8) [Table 1].
Demographics of patients and TDC group and overview of genetic data + phenotype
GRIN2B | Patient 1 | Patient 2 | Patient 3 | Patient 4 | Patient 5 |
Sex | Male | Female | Female | Male | Male |
Age when EEG recorded (years) | 3.00 | 4.77 | 6.11 | 8.25 | 12.72 |
Genotypic spectrum | |||||
GRIN2B variants | 12013.2.13.1 | c.1583 C>T | c.2459 G>C | c.2496 C>G | c.2087 G>T |
Protein level | p.(Pro528Leu) | p.(Gly820Ala) | p.(Ser832Arg) | p.(Arg696Leu) | |
Variant type | Missense | Missense | Missense | Missense | Missense |
Origin | de novo | de novo | de novo | de novo | de novo |
Functional analysis | LoF | LoF | LoF | LoF | LoF |
Phenotype | |||||
Epilepsy | No | No | No | No | No |
Severity of ID | Mild | Moderate | Severe | Moderate | Mild |
Co-occurring neurodevelopmental disorders | None | None | None | ASD | ADHD |
Speech and language | Delayed, 1-2 word sentences | Delayed, 1-2 word sentences | Non-verbal | Delayed, 2-3 word sentences | Delayed, delay of |
Motor development/musculoskeletal | Delayed | Hypotonia, hypermobility | Not able to walk, Hypotonia | No | No |
Sleeping problems | No | No | Yes | Yes | No |
Gastrointestinal problems | No | Yes | Yes | Yes | No |
Demographics TDC group | Control1 | Control2 | Control3 | Control4 | Control5 |
N | 3 | 7 | 8 | 9 | 8 |
Sex | Male | Female | Female | Male | Male |
Age range (years) | 4.8-5.1 | 4.6-5.5 | 5.7-6.7 | 7.5-9.3 | 11.2-14.8 |
IQ range | 98-108 | 98-125 | 78-121 | 103-134 | 95-126 |
P-value of age with patient | 0.07 (t = 3.71) | 0.370 (t = 0.969) | 0.845 (W = 5) | 0.982 (t = 0.02) | 0.985 (t = 0.020) |
Absolute power
We evaluated whether LoF GRIN2B patients show changes in the spectral power of the EEG. To this end, absolute power was compared between GRIN2B and matched TDC between 1-45 Hz. At the source level, increased absolute power was found for the patient group (P < 0.05) with a whole brain distribution in the lower oscillations of 1.0-4.0 Hz, (P < 0.05) [Figure 1A, Figure 2A and B]. In this group, increased absolute power in the left parietal, left and right temporal lobe, and right frontal lobe was found in the remaining frequencies except for 17.05-21.71 Hz [Figure 2A and B]. For reasons of brevity, the individual values averaged across significant regions are plotted per frequency bin; Figure 2C shows increased values for the GRIN2B group (P < 0.05).
Figure 1. Overview of the qEEG biomarkers of eyes open (EO) resting state in the range of 1-45 Hz in source space. GRIN2B group is plotted in orange while the TDC group is plotted in black. The averaged values with the standard error of the mean are plotted of (A) absolute power in dB, (B) relative power in proportion, (C) DFA and (D) fEl. Bootstrapping analyses were corrected with FDR of 0.05. Significant results are indicated by the red dots. Power effects are mostly found for the lowest to middle oscillations (1-8.3 Hz), In
Figure 2. Absolute power in source space in the range of 1-45 Hz. (A) shows absolute power in regions on source level per 11 bins. Power was averaged across 68 patches of the Desikan Atlas and plotted for 6 regions. Red color shows significant regions for the GRIN2B patients while blue indicates significant regions for the TDC group. Grey areas show no significant results. Bootstrapping analyses were FDR corrected with 0.05. (B) shows an overview of region names. (C) shows the individual values of significant regions in source space per bin. Orange boxplots represent the GRIN2B patients, while black boxplots represent the TDC group with Δ indicating the average group difference with the P value of the significant regions. Absolute power in the lowest bin 1-4 Hz whole brain activities most pronounced in the GRIN2B patients. In subsequent bins the left parietal lobe and right temporal lobe show most effects for the GRIN2B group, followed by the left temporal lobe and right frontal lobe.
Relative power
Source estimation analyses revealed increased relative power in the lowest oscillations (1-4 Hz) across all regions, apart from the occipital areas [Figure 1B, Figure 3A and B] (P < 0.05). The opposite pattern was found from 4.0-27.64 Hz: lower relative power was found for the patient group compared to the TDC. Areas spanning the entire brain (4.0-6.49 Hz) and areas spanning the frontal and parietal lobes (6.49-17.05 and 21.71-27.64 Hz) showed higher relative power in the TDC group compared to the patient group (P < 0.05)
Figure 3. Relative power in source space in the range of 1-45 Hz. (A) shows relative power as proportion in regions on source level per 11 bins. Power was averaged across 68 patches of the Desikan Atlas and plotted for 6 regions. Red color shows significant regions for the GRIB patients while blue indicates significant regions for the TDC group. Grey areas show no significant results. Bootstrapping analyses were FDR corrected with 0.05. (B) shows an overview of region names. (C) shows the individual values of significant regions in source space per bin. Orange boxplots represent the GRIN2B patients, while black boxplots represent the TDC group with Δ indicating the average group difference with the P value of the significant regions. Relative power in the lowest bin 1-4 Hz shows whole brain activities most pronounced in the GRIN2B patients while the opposite was found for subsequent bins: Higher power were found for the TDC group for 4.0-27.64 Hz. Whole brain activities were shown from 4.0-6.49 Hz followed by left and right frontal and parietal lobes, left temporal and occipital lobe.
DFA
To investigate whether the LoF GRIN2B mutations affect the temporal structure of EEG signals, the quantification of long-range temporal correlations measured by DFA was computed. The DFA showed increased values across almost all bins [Figure 1C]. The scalp topographies indicated brain-wide increased temporal correlations (P < 0.05) [Figure 1C, Figure 4A-C].
Figure 4. Long range temporal correlations as expressed by DFA in source space in the range of 1-45 Hz. (A) shows DFA values in regions on source level per 11 bins. DFA was averaged across 68 patches of the Desikan Atlas and plotted for 6 regions. Red color shows significant regions for the GRIN2B patients while blue indicates significant regions for the TDC group. Grey areas show no significant results. Bootstrapping analyses were FDR corrected with 0.05. (B) shows an overview of region names. (C) shows the individual values of significant regions in source space per bin. Orange boxplots represent the GRIN2B patients, while black boxplots represent the TDC group with Δ indicting the average group difference with the P value of the significant regions. Whole brain activities for the DFA were most pronounced throughout the brain for the GRIN2B patients, across all bins.
fEI
To test if LoF GRIN2B mutations lead to network-level changes in E/I balance, the fE/I was studied. The fE/I showed lower values across all frequencies (P < 0.05) [Figure 1D]. Higher values were found for the patients in the left insula 4.0-5.09 Hz (P < 0.05), while higher values for the TDC group were found in areas spanning the frontal to occipital areas from 1.0-13.39 Hz (P < 0.05) [Figure 5A-C]. The individual spectrums and results in electrode space are presented in the Supplementary Materials.
Figure 5. Functional E/I in source space in the range of 1-45 Hz. (A) shows fEl values in regions on source level per 11 bins. FEI was averaged across 68 patches of the Desikan Atlas and plotted for 6 regions. Red color shows significant regions for the GRIN2B patients while blue indicates significant regions for the TDC group. Grey areas show no significant results. Bootstrapping analyses were FDR corrected with 0.05. (B) shows an overview of region names. (C) shows the individual values of significant regions in source space per bin. Orange boxplots represent the GRIN2B patients, while black boxplots represent the TDC group with Δ indicating the average group difference with the P value of the significant regions. Higher values were found for the TDC group almost across all regions from
DISCUSSION
In this case-control EEG study, we tested, for the very first time, the hypothesis that ionotropic glutamate NMDAR dysregulation in GRIN2B disorder affects qEEG measures associated with network-level activity retrieved from resting-state, eyes-open EEG recordings. In five patients with de novo LoF, widespread resting-state EEG abnormalities were found in absolute and relative spectral power, DFA, and fE/I after accounting for age and gender. These findings indicate slowing of activities confirmed by increased absolute and relative power in low frequencies and inhibition-dominated brain dynamics implied by decreased fE/I ratio and increased DFA values. Our results suggest potential evidence that brain-wide excessive slow frequency oscillations with an inhibition-dominated brain state reflect a core aspect of GRIN2B pathophysiology and may serve a clinical utility.
QEEG suggests a shift to slower power dynamics in GRIN2-NDD disorder
Increased low-frequency (1-4 Hz) activity is found for the GRIN2B patients. This frequency, also known as delta activity, has been involved with basic processes such as sleep, homeostasis, and autonomic regulation, as well as motivation and cognition[29]. Delta frequencies are generated in the thalamocortical system, involving both cortical and subcortical areas, and play a key role in functionally linking different brain regions[29]. Delta activity also plays an important role in the development of healthy children, with decreased delta power as they age. This is due to maturational changes, including synaptic pruning, grey matter reductions, and the maturation of the GABA (gamma-aminobutyric acid) system[30,31]. Interestingly, the excess of spontaneous oscillation in the delta frequency range has been observed in developmental disorders such as Angelman syndrome[32], autism spectrum disorder[33], and STXBP1 syndrome[19], showing similar atypical widespread results and clinical features. However, prominent delta power has also been observed in states of diminished consciousness, such as slow-wave sleep, anesthesia, generalized epilepsy, and disorders of consciousness, including coma and the vegetative state. These states are characterized by the significant involvement of GABA in inhibitory neuronal activity[34]. Clinical reports on Rett syndrome, Lennox-Gastaut syndrome, hepatic encephalopathy, mitochondrial diseases, and schizophrenia have also suggested similar excessive slowing during wake states, which seems to contradict the established correlation between diminished consciousness and increased delta power[35]. Interestingly, the work on schizophrenia has shown that NMDAR antagonists increase delta oscillations[36,37]. This suggests that increased delta oscillations, essential in schizophrenia symptoms, show a switch in affected regions from a wake to a sleeplike state with its typical cognitive deficits[38]. Our findings with the GRIN2B patients suggest that impaired NMDAR signaling due to the GluN2B variant involved in glutamate expression might similarly affect oscillations, leading to increased delta power. As low-frequency oscillations are involved in long-distance neural communication, the increase in delta suggests less efficient long-range connections between neurons, increased inefficient integrated local networks, and reduced grey matter integrity across brain regions. In addition, when linking neurons to behavior, oscillations form behavior-dependent oscillating networks by mediating spatial and temporal synchrony across frequencies, which play a role in perception, memory, emotions, thoughts, and actions[5,39]. This implies that different frequency oscillations are related to different processes, indicating that EEG power suppression in most frequencies can provide a possible link with clinical phenotypes such as intellectual disability, developmental delay, communication impairment, autism spectrum disorder, and sleep disorders. This elucidates the role of delta power to a small extent and suggests the observed increase in delta power in NMDAR dysregulation in GRIN2B-NDD patients, but future studies are needed to address the mechanistic relationship in delta oscillations.
Power effects were also observed for the high-frequency oscillations. Absolute power in the range of
Taken together, the delta effects in GRIN2B results show the increased global background slowing, closely tracking cognitive decline in neurodevelopmental delay. At the same time, cognitive processes are regulated by the compensatory effects of high-frequency oscillations.
Brain-wide higher DFA values were found in children with GRIN2B across almost the entire frequency spectrum. The DFA is used to quantify the long-range temporal correlations of the brain oscillations when showing a positive correlation (between 0.5-1). It is thought that the DFA exponent provides insights into information transfer in neuronal networks[44,45]. The DFA exponent provides a statistical index of dynamical oscillations and indicates the persistence of autocorrelations on different time scales. Weak autocorrelation is reflected by DFA exponent values around 0.5, assuming uncorrelated and random walk-like processes, while strong autocorrelation is reflected by a DFA exponent closer to 1. Reduced DFA exponents have been observed in Alzheimer's disease[46], schizophrenia[47], and depression[48], while higher values were found in NDD such as ASD[11,18] and STXBP1[19]. GRIN2B patients show alterations in the temporal structure of brain oscillations throughout the brain in line with previous findings in ASD and STXBP1, suggesting a more structured temporal profile of fluctuations in oscillations across all frequencies and brain regions. This suggests that impaired NMDAR signaling may play an important role in neuronal differentiation, presumably leading to a global effect on the temporal structure of EEG oscillations yet leading to different clinical features.
Lastly, fE/I was used to index changes in the E/I balance at the network level. The fE/I quantifies the covariance between the amplitude envelope and DFA of EEG oscillations[11]. The fE/I values are lower in GRIN2B patients compared to age- and gender-matched controls across frequencies and the cortex. A previous fE/I study involving healthy children showed that fE/I values were computed only for the alpha band (8-12 Hz) of approximately 1 for healthy controls and lower values for ASD patients[11]. This, however, consisted of spontaneous activity observed during the resting state of eyes closed, a different brain state that cannot be equated with eyes-open recordings which typically show fE/I values of ≈ 0.80[11,23]. In the present study, patients were tested in a resting state with their eyes open, showing decreased fE/I values in oscillations across a broad range of frequencies. This broadband decrease aligns with the DFA values showing similar inhibitory-related broadband results. Furthermore, these results are consistent with the fE/I values of children with STXBP1 syndrome, which show similar effects across the cortex and indicate a shift toward stronger inhibition in GRIN2B. Interestingly, the GRIN2B patients showed higher fE/I values in the left insula within the 4.0-5.09 Hz bin, which falls within the classical theta band (4-8 Hz) and plays a crucial role in neurocognitive development. Resting-state studies in children have reported negative correlations between theta power and cognitive abilities[49], presumably indicating more impairments observed in GRIN2B-NDD with the increased theta fE/I. The insula is known to be an important switching hub for sensory input between various networks, including the default mode, salience, and executive networks[50]. Moreover, atypical insula activation has increasingly been linked to autism spectrum disorder[50,51]. Therefore, it can be speculated that the insula-related fE/I increase in GRIN2B may have similar dysfunctional consequences to those observed in autism spectrum disorder.
LoF variants of the NMDAR cause abnormal neuronal differentiation, synaptogenesis, synaptic plasticity, and an imbalance between excitatory and inhibitory signaling at the micro and macro levels. Although genetic alterations can result in changes in the E/I across circuitries, less is known about the underlying mechanisms of these cortico-cortical circuits and projections leading to E/I imbalances. While cortical networks require a finely tuned coordination of excitatory and inhibitory inputs for efficient information processing, contributing to better cognitive performance, widespread imbalances might be compensated with higher-frequency oscillations for information processing restoration. Future studies should investigate the exact mechanistic link between the distinct levels of neural organization and should include additional neurodevelopmental disorders to test whether the findings are GRIN2B-NDD-specific, or a more general feature of disorders characterized by similar manifestations. Overall, network-level qEEG features indicate that GRIN2B-NDD patients with de novo LoF variants display inhibition-related dynamics compared to age- and gender-matched healthy controls. Changes along the frequency spectrum as well as widespread cortical regions were observed for almost all qEEG features, which may be associated with clinical manifestations of the patients.
Methodological considerations
Testing hypotheses regarding the neuronal underpinnings of rare NDD is challenging. One difficulty lies in the small sample sizes, which are relatively smaller compared to those in studies of more common disorders. Additionally, testing children with developmental and behavioral disabilities is particularly difficult, as adherence to instructions can be problematic in severely affected patients, resulting in suboptimal data recording and poor data quality with excessive artifacts in EEG signals. Although the data can be preprocessed semi-automatically, visual inspection by the researcher is a crucial and necessary step. Preprocessed clean signals in this study varied between 30-290 s as parts of patient data were too noisy and needed to be removed. Despite the data loss, quantification of the EEG biomarkers data was acceptable for five patients [Supplementary Figure 1]. Spectral power can be reliably estimated from recordings with a data length of > 20 s[52,53], whereas the minimal signal length for reliable estimation of DFA and fE/I is around
Limitations
One limitation of this study is that the control group was matched only for age and gender. Future studies should consider adding control groups with intellectual disabilities to more accurately compare a child's chronological age with their developmental age. This would allow for a better study of EEG markers, as well as the cognitive, social, physical, and emotional domains.
A second limitation is the absence of associations between the clinical data and the EEG markers. The EEG recordings reported in this manuscript are part of a study designed to evaluate the effectiveness of L-Serine for GRIN2B-NDD using an N-of-1 trial design. A series of randomized, double-blind, placebo-controlled single-patient trials were therefore conducted. During this period, the researchers were blinded to the allocation and had no access to clinical data and behavioral scales.
A third limitation concerns the source space reconstruction used to calculate the EEG markers. Despite the efforts to match age and gender, the developmental changes occurring in the brain require realistic age-specific head models for accurate source reconstruction. Incorporating the realistic anatomy of children's heads into EEG source analysis improves both accuracy and reliability[54]. Ideally, individual realistic head models can be derived from the subject's MRI, but obtaining MRI data from infants, children, and especially children with NDD is challenging. Our EEG source analysis pipeline did not include age-specific template models that represent the child-typical anatomy. Ideally, we would like to have age-specific realistic head model templates to analyze EEG data while considering the typical anatomical head features. Unfortunately, our analyses relied on adult averaged template models incorporated in MNE, which did not yield accurate source reconstruction. Future studies should focus on creating more realistic head models.
CONCLUSION AND FUTURE DIRECTIONS
This is the first study to present qEEG markers in a case series of patients with GRIN2Bde novo LoF variants, guided by bootstrapping statistics. This can bridge the gap between preclinical studies on molecular and cellular pathogenic mechanisms as well as clinical manifestations. The quantitative EEG markers provide insights into pathophysiological changes in brain dynamics, which are valuable in developing personalized mechanism treatments.
DECLARATIONS
Acknowledgments
The authors thank the patients and their families for their participation in the EEG measurements. Ramautar JR would like to thank Frank Bennis for the statistical input and Annelieke Muller for helpful suggestions.
Authors’ contributions
Responsible for data collection, preprocessing, data analysis, methodology, and original draft preparation: Ramautar JR
Conducted the clinical study: den Hollander B
Conducted the control study: Kooper CC
Handled data preprocessing: Candellero M
Wrote the bootstrapping scripts and performed the bootstrapping analyses: Diachenko M
Data analysis: Sharma A
Data collection: Asbreuk MABC
Handled the data preprocessing: Sprengers JJ, Linkenkaer-Hansen K
Supervised the project: van Haelst MM, van Karnebeek CD, Bruining H
Availability of data and materials
The datasets used and/or analyzed during the current study are available from the corresponding author upon reasonable request.
Financial support and sponsorship
This study was supported by BECAUSE ZonMW TOP 91216064; NewTDEC Netherlands Organization for Scientific Research (NWO) Dutch National Research Agenda, NWA-ORC Call (NWA.1160.18.200); Aspasia Grant of the Dutch Research Council (015.014.036); Netherlands Organization for Health Research and Development (91718310); BRAINMODEL ZonMW PSIDER 10250022110003; and Amsterdam UMC TKI grant BRAINinBalance. The L-serine and placebo capsules were supported by the platform Medicine for Society. This work was also supported by United for Metabolic Disease [UMD-CG-2021-019, 2021], ‘s Heeren Loo. Stichting Metakids NL [2020-01-UMD, 2020], and Ladies Circle Nederland to CK.
Conflicts of interest
Bruining H and Linkenkaer-Hansen K are shareholders of Aspect Neuroprofiles BV, which develops physiology-informed prognostic measures for neurodevelopmental disorders. Linkenkaer-Hansen K has filed the patent claim (PCT/NL2019/050167) “Method of determining brain activity”, with a priority date of March 16, 2018. All other authors declared that there are no conflicts of interest.
Ethics approval and consent to participate
The patient study obtained approval from the Amsterdam UMC Ethics Committee prior to the study commencement (30 June 2022, 2022.0271 - NL802290.081.22). All procedures were executed according to the Declaration of Helsinki. The control study obtained approval from the Medical Ethical Committee Amsterdam University Medical Centers (4 June 2021, location AMC, reference number NL76915.018.21), and was registered at the International Clinical Trials Registry Platform (NL9574).
Consent for publication
Written informed consent for participation and publication was provided by all children aged > 11 years old and parents of children aged < 16 years old. Written informed consent for publication of their details was obtained from the patient/study participant/guardian/next of kin. The family was fully informed about the nature of the study and the potential implications of the publication, and agreed to the use of this information in this publication.
Copyright
© The Author(s) 2025.
Supplementary Materials
REFERENCES
2. Platzer K, Yuan H, Schütz H, et al. GRIN2B encephalopathy: novel findings on phenotype, variant clustering, functional consequences and treatment aspects. J Med Genet. 2017;54:460-70.
3. Bell S, Maussion G, Jefri M, et al. Disruption of GRIN2B impairs differentiation in human neurons. Stem Cell Rep. 2018;11:183-96.
4. den Hollander B, Rothuizen-Lindenschot M, Geertjens L, et al. Effectiveness of L-serine supplementation in children with a GRIN2B loss-of-function mutation: rationale and protocol for single patient (n-of-1) multiple cross-over trials. Contemp Clin Trials Commun. 2023;36:101233.
5. Santos-Gómez A, Miguez-Cabello F, García-Recio A, et al. Disease-associated GRIN protein truncating variants trigger NMDA receptor loss-of-function. Hum Mol Genet. 2021;29:3859-71.
6. Burnashev N, Szepetowski P. NMDA receptor subunit mutations in neurodevelopmental disorders. Curr Opin Pharmacol. 2015;20:73-82.
7. Bozzi Y, Provenzano G, Casarosa S. Neurobiological bases of autism-epilepsy comorbidity: a focus on excitation/inhibition imbalance. Eur J Neurosci. 2018;47:534-48.
8. Foss-Feig JH, Adkinson BD, Ji JL, et al. Searching for cross-diagnostic convergence: neural mechanisms governing excitation and inhibition balance in schizophrenia and autism spectrum disorders. Biol Psychiatry. 2017;81:848-61.
9. Nelson SB, Valakh V. Excitatory/inhibitory balance and circuit homeostasis in autism spectrum disorders. Neuron. 2015;87:684-98.
10. Selten M, van Bokhoven H, Nadif Kasri N. Inhibitory control of the excitatory/inhibitory balance in psychiatric disorders. F1000Res. 2018;7:23.
11. Bruining H, Hardstone R, Juarez-Martinez EL, et al. Measurement of excitation-inhibition ratio in autism spectrum disorder using critical brain dynamics. Sci Rep. 2020;10:9195.
12. Hardstone R, Poil SS, Schiavone G, et al. Detrended fluctuation analysis: a scale-free view on neuronal oscillations. Front Physiol. 2012;3:450.
13. Poil SS, van Ooyen A, Linkenkaer-Hansen K. Avalanche dynamics of human brain oscillations: relation to critical branching processes and temporal correlations. Hum Brain Mapp. 2008;29:770-7.
14. Avramiea AE, Hardstone R, Lueckmann JM, Bím J, Mansvelder HD, Linkenkaer-Hansen K. Pre-stimulus phase and amplitude regulation of phase-locked responses are maximized in the critical state. Elife. 2020;9:e53016.
15. Avramiea AE, Masood A, Mansvelder HD, Linkenkaer-Hansen K. Long-range amplitude coupling is optimized for brain networks that function at criticality. J Neurosci. 2022;42:2221-33.
16. Poil SS, Hardstone R, Mansvelder HD, Linkenkaer-Hansen K. Critical-state dynamics of avalanches and oscillations jointly emerge from balanced excitation/inhibition in neuronal networks. J Neurosci. 2012;32:9817-23.
17. Juarez-Martinez EL, Sprengers JJ, Cristian G, et al. Prediction of behavioral improvement through resting-state electroencephalography and clinical severity in a randomized controlled trial testing bumetanide in autism spectrum disorder. Biol Psychiatry Cogn Neurosci Neuroimaging. 2023;8:251-61.
18. Juarez-Martinez EL, van Andel DM, Sprengers JJ, et al. Bumetanide effects on resting-state EEG in tuberous sclerosis complex in relation to clinical outcome: an open-label study. Front Neurosci. 2022;16:879451.
19. Houtman SJ, Lammertse HCA, van Berkel AA, et al. STXBP1 syndrome is characterized by inhibition-dominated dynamics of resting-state EEG. Front Physiol. 2021;12:775172.
20. den Hollander B, Veenvliet ARJ, Rothuizen-Lindenschot M, et al. Evidence for effect of l-serine, a novel therapy for GRIN2B-related neurodevelopmental disorder. Mol Genet Metab. 2023;138:107523.
21. Gramfort A, Luessi M, Larson E, et al. MEG and EEG data analysis with MNE-Python. Front Neurosci. 2013;7:267.
22. Weiler R, Diachenko M, Juarez-Martinez EL, Avramiea AE, Bloem P, Linkenkaer-Hansen K. Robin's viewer: using deep-learning predictions to assist EEG annotation. Front Neuroinform. 2022;16:1025847.
23. Diachenko M, Sharma A, Smit DJA, et al. Functional excitation-inhibition ratio indicates near-critical oscillations across frequencies. Imaging Neurosci. 2024;2:1-17.
25. Hämäläinen MS, Ilmoniemi RJ. Interpreting magnetic fields of the brain: minimum norm estimates. Med Biol Eng Comput. 1994;32:35-42.
26. Matsuura K, Okabe Y. Selective minimum-norm solution of the biomagnetic inverse problem. IEEE Trans Biomed Eng. 1995;42:608-15.
27. Desikan RS, Ségonne F, Fischl B, et al. An automated labeling system for subdividing the human cerebral cortex on MRI scans into gyral based regions of interest. Neuroimage. 2006;31:968-80.
28. Li Q, Weiland RF, Konvalinka I, et al. Intellectually able adults with autism spectrum disorder show typical resting-state EEG activity. Sci Rep. 2022;12:19016.
29. Knyazev GG. EEG delta oscillations as a correlate of basic homeostatic and motivational processes. Neurosci Biobehav Rev. 2012;36:677-95.
30. Feinberg I, Campbell IG. Sleep EEG changes during adolescence: an index of a fundamental brain reorganization. Brain Cogn. 2010;72:56-65.
31. Smit DJ, Boersma M, Schnack HG, et al. The brain matures with stronger functional connectivity and decreased randomness of its network. PLoS One. 2012;7:e36896.
32. Hipp JF, Frohlich J, Keute M, Tan WH, Bird LM. Electrophysiological abnormalities in angelman syndrome correlate with symptom severity. Biol Psychiatry Glob Open Sci. 2021;1:201-9.
33. Wang J, Barstein J, Ethridge LE, Mosconi MW, Takarae Y, Sweeney JA. Resting state EEG abnormalities in autism spectrum disorders. J Neurodev Disord. 2013;5:24.
34. Hobson JA, Pace-Schott EF. The cognitive neuroscience of sleep: neuronal systems, consciousness and learning. Nat Rev Neurosci. 2002;3:679-93.
35. Frohlich J, Toker D, Monti MM. Consciousness among delta waves: a paradox? Brain. 2021;144:2257-77.
36. Zhang Y, Llinas RR, Lisman JE. Inhibition of NMDARs in the nucleus reticularis of the thalamus produces delta frequency bursting. Front Neural Circuits. 2009;3:20.
37. Zhang Y, Yoshida T, Katz DB, Lisman JE. NMDAR antagonist action in thalamus imposes delta oscillations on the hippocampus. J Neurophysiol. 2012;107:3181-9.
38. Hunt MJ, Kasicki S. A systematic review of the effects of NMDA receptor antagonists on oscillatory activity recorded in vivo. J Psychopharmacol. 2013;27:972-86.
40. Herrmann CS, Strüber D, Helfrich RF, Engel AK. EEG oscillations: from correlation to causality. Int J Psychophysiol. 2016;103:12-21.
41. Lundqvist M, Miller EK, Nordmark J, Liljefors J, Herman P. Beta: bursts of cognition. Trends Cogn Sci. 2024;28:662-76.
42. Fell J, Klaver P, Lehnertz K, et al. Human memory formation is accompanied by rhinal-hippocampal coupling and decoupling. Nat Neurosci. 2001;4:1259-64.
43. Doesburg SM, Green JJ, McDonald JJ, Ward LM. Rhythms of consciousness: binocular rivalry reveals large-scale oscillatory network dynamics mediating visual perception. PLoS One. 2009;4:e6142.
44. Linkenkaer-Hansen K, Monto S, Rytsälä H, Suominen K, Isometsä E, Kähkönen S. Breakdown of long-range temporal correlations in theta oscillations in patients with major depressive disorder. J Neurosci. 2005;25:10131-7.
45. Linkenkaer-Hansen K, Smit DJ, Barkil A, et al. Genetic contributions to long-range temporal correlations in ongoing oscillations. J Neurosci. 2007;27:13882-9.
46. Montez T, Poil SS, Jones BF, et al. Altered temporal correlations in parietal alpha and prefrontal theta oscillations in early-stage Alzheimer disease. Proc Natl Acad Sci USA. 2009;106:1614-9.
47. Nikulin VV, Jönsson EG, Brismar T. Attenuation of long-range temporal correlations in the amplitude dynamics of alpha and beta neuronal oscillations in patients with schizophrenia. Neuroimage. 2012;61:162-9.
48. Hou D, Wang C, Chen Y, Wang W, Du J. Long-range temporal correlations of broadband EEG oscillations for depressed subjects following different hemispheric cerebral infarction. Cogn Neurodyn. 2017;11:529-38.
49. Tan E, Troller-Renfree SV, Morales S, et al. Theta activity and cognitive functioning: Integrating evidence from resting-state and task-related developmental electroencephalography (EEG) research. Dev Cogn Neurosci. 2024;67:101404.
50. Namkung H, Kim SH, Sawa A. The insula: an underestimated brain area in clinical neuroscience, psychiatry, and neurology. Trends Neurosci. 2017;40:200-7.
51. Das A, Myers J, Mathura R, et al. Spontaneous neuronal oscillations in the human insula are hierarchically organized traveling waves. Elife. 2022;11:e76702.
52. Gasser T, Bächer P, Steinberg H. Test-retest reliability of spectral parameters of the EEG. Electroencephalogr Clin Neurophysiol. 1985;60:312-9.
53. Salinsky MC, Oken BS, Morehead L. Test-retest reliability in EEG frequency analysis. Electroencephalogr Clin Neurophysiol. 1991;79:382-92.
Cite This Article
How to Cite
Download Citation
Export Citation File:
Type of Import
Tips on Downloading Citation
Citation Manager File Format
Type of Import
Direct Import: When the Direct Import option is selected (the default state), a dialogue box will give you the option to Save or Open the downloaded citation data. Choosing Open will either launch your citation manager or give you a choice of applications with which to use the metadata. The Save option saves the file locally for later use.
Indirect Import: When the Indirect Import option is selected, the metadata is displayed and may be copied and pasted as needed.
About This Article
Special Issue
Copyright
Data & Comments
Data
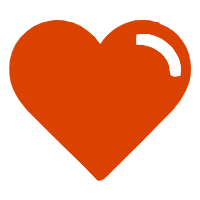
Comments
Comments must be written in English. Spam, offensive content, impersonation, and private information will not be permitted. If any comment is reported and identified as inappropriate content by OAE staff, the comment will be removed without notice. If you have any queries or need any help, please contact us at [email protected].