The human intestinal bacterium Eggerthella lenta influences gut metabolomes in gnotobiotic mice
Abstract
The intestinal microbiota and its metabolites are known to influence host metabolic health. However, little is known about the role of specific microbes. In this work, we used the minimal consortium Oligo-Mouse-Microbiota (OMM12) to study the function of Coriobacteriia under defined conditions in gnotobiotic mice. OMM12 mice with or without the addition of the dominant gut bacterium Eggerthella lenta (E. lenta) were fed with diets varying in fat content and primary bile acids. E. lenta stably colonised the mouse caecum at high relative abundances (median: 27.5%). This was accompanied by decreased occurrence of Akkermansia muciniphila and Enterococcus faecalis, but results did not reach statistical significance in all groups depending on diet and inter-individual differences. Changes in host parameters (anthropometry, blood glucose, and cholesterol) and liver proteomes were primarily due to diet. In contrast, metabolomes in colon content differed significantly between the colonisation groups. The presence of
Keywords
INTRODUCTION
The intestinal microbiota influences metabolic health[1,2]. For instance, there is good evidence that the communities of microbes in our gut alter diet-host interactions, and that they play a causal role in the development and progression of metabolic diseases[3-6]. However, there is little data about specific microbes that are important for these processes[7-9]. This is partly due to the complexity of gut microbial ecosystems, including multiple types of microorganisms and hundreds of different bacterial species, of which a substantial fraction is still unknown[10]. Hence, it is useful to develop and utilize simplified models of the gut microbiota to study the function of specific ecosystem members[11,12].
Coriobacteriaceae represents a family of Gram-positive bacteria within the phylum Actinomycetota (formerly Actinobacteria). While species within this family have regularly been associated with bacteraemia, they are also important members of the human gut microbiome such as Atopobium, Collinsella, Eggerthella, and Slackia spp[13]. Coriobacteriaceae was recently split into multiple families within the class Coriobacteriia, including Eggerthellaceae [represented by the type genus Eggerthella, including its type species Eggerthella lenta (E. lenta), formerly Eubacterium lentum], Atopobiaceae, and Coriobacteriaceae[14]. E. lenta is a dominant and prevalent member within human gut microbial ecosystems[15]. Besides belonging to the core microbiome, strains within this species are functionally versatile; they can metabolise multiple host-derived molecules (e.g., hormones, bile acids, neurotransmitters)[16-19] and oral compounds such as dietary substrates (e.g., polyphenols)[20,21] and drugs (e.g., digoxin)[22]. Despite these important functional assets, few studies have investigated the role of E. lenta within the ecosystem and its effects on the host under defined conditions.
Using multiple strains of E. lenta in monocolonised mice, Alexander et al. recently demonstrated the causal role of E. lenta in Th17-dependent colitis, which was attributable to the bacterial enzyme cardiac glycoside reductase 2 (Cgr2)[23]. Interestingly, the induction of inflammation could be prevented by the addition of arginine to the diet due to the sensitivity of Cgr2 for this substrate, pointing to the importance of diet-microbe interactions for the regulation of host functions. In the context of metabolic health, several descriptive studies have investigated associations between the occurrence of Eggerthella spp. and E. lenta in human faeces as detected by sequencing and obesity or type 2 diabetes (T2D). Koh et al. reported a higher relative abundance in T2D patients (n = 33) linked to the ability of the species to produce imidazole propionate[24]. Qin et al. also reported a positive association between E. lenta and T2D (n = 170)[25], but the results from these investigations were confounded by metformin treatment[26]. With respect to obesity, Yun et al. (n = 940) reported a negative association between Eggerthella and body mass index (BMI)[27], while Fu et al. (n = 893) found no significant association with BMI but strong positive or negative association with blood triglyceride or high-density lipoprotein (HDL) cholesterol levels[28], respectively. Hence, results on the occurrence of Eggerthella spp. and E. lenta in metabolic diseases are conflicting and experimental studies are lacking.
In this work, mice colonised with the Oligo-Mouse-Microbiota (OMM12), a bacterial consortium consisting of 12 bacterial isolates from the mouse intestine[29], with or without additional colonisation by
METHODS
Cultivation and preparation of gavage solutions
The original aim of this work was to use a consortium of Coriobacteriaceae that represent major functional traits of this family. Hence, Adlercreutzia mucosicola DSM 19490T (formerly Enterorhabdus mucosicola), Collinsella aerofaciens DSM 3979T, E. lenta DSM 2243T, and Lancefieldella parvula DSM 20469T (formerly Atopobium parvulum) were cultured in broth of Wilkins-Chalgren-Anaerobe medium (WCA, Thermo Fisher Scientific) or brain-heart-infusion (BHI, Oxoid). These culture media were supplemented with
For colonising germfree mice, microbial suspensions containing the OMM12 strains[29] with or without the Coriobacteriia were produced and aliquoted in an anaerobic chamber (MBraun, Garching, Germany; 89.3% N2, 6% CO2, 4.7% H2) and stored at -80 °C. Three frozen caeca from OMM12 mice (kindly provided by
Gnotobiotic mouse experiments
Animal use was approved by the local institution in charge (Regierung von Oberbayern, approval No. 55.2-1-54-2532-156-2013). The animals were housed in the mouse facility of the Technical University of Munich, ZIEL - Institute for Food & Health.
The experimental scheme is presented in Supplementary Figure 1A. Germfree male C57BL/6N mice were associated with 0.2 mL of the freshly thawed microbial mixtures aforementioned via oral gavage three times within one week at the age of five weeks immediately after weaning. During this process, the mice were allocated to cages, ensuring that each colonisation/diet group included mice from different litters housed in multiple cages [Supplementary Figure 1B]. Male mice were used due to their responsiveness to diet-induced obesity. From week 8 onwards, the diet was changed from autoclaved standard chow (Ssniff GmbH) to synthetic control diet (CD) for two weeks. Thereafter, the mice were fed one of the following diets for eight weeks (n = 6-8 per group): CD; CD-BA, control diet supplemented with the primary bile acids cholic acid (CA) and chenodeoxycholic acid (CDCA) (each 0.1% w/w); HFD, lard-based high-fat diet (48% energy from fat); HFD-BA, HFD supplemented with CA and CDCA. These diets were used due to the link between Coriobacteriia/E. lenta and both bile acid metabolism and host metabolic health. Their references and main differences in composition are provided in Supplementary Figure 1C. Before euthanizing the mice with CO2, they were fasted for a minimum of 6 h and blood glucose was measured from the tail using the FreeStyle Lite system (Abbott). During necropsy, systemic EDTA plasma was obtained from the central vein (vena cava). Contents of the caecum and colon as well as liver lobes were snap frozen. Epididymal, mesenteric, and subcutaneous white adipose tissues were weighed, and their cumulative weight referred to as white adipose tissue (WAT) mass.
Cholesterol analysis
Cholesterol concentrations in plasma were quantified using the VITROS Chemistry Products CHOL Slides (Ortho Clinical Diagnostics) with the VITROS 350 Chemistry System (Ortho Clinical Diagnostics).
Microbial DNA isolation
Caecal content from the gnotobiotic mice or the bacterial stocks used for gavage were mixed with 500 μL Tris-EDTA (TE) buffer containing 7.5 mg lysozyme and incubated at 37 °C for 30 min. 50 μL 10% sodium dodecyl sulfate (SDS, Carl Roth) and 300 μg proteinase K (Carl Roth) were added before incubation at 50 °C for 1 h. The samples were then transferred to 2 mL screw-cap tubes containing 500 mg zirconia/silica beads (0.1 mm; Carl Roth), 250 μL 4M Guanidinethiocyanate (Sigma Aldrich), and 500 μL 5% N-laurolylsarcosine (Sigma Aldrich), and they were incubated at 70 °C for 1 h with constant shaking. Mechanical lysis was then performed using a FastPrep®-24 (3 times, 40 s, 6.5 m/s) (MP Biomedicals). Following the addition of
High-throughput 16S rRNA gene amplicon sequencing
The V3/V4 regions of 16S rRNA genes were amplified (25 cycles; two-step PCR)[30] using primer 341F and 785R[31], including combinatorial dual indexing. Libraries were purified using magnetic beads (Beckman-Coulter) and then pooled in an equimolar manner prior to sequencing in single-end mode on a MiSeq according to the manufacturer’s instructions (Illumina). The UPARSE-based[32] platform IMNGS[33] was used to process the raw sequence reads with the following parameters: barcode mismatches, 1; expected error, 9; quality trimming score, 20; trimming length, 10 nt; min. sequence length, 200 nt; max. sequence length, 300 nt. Operational taxonomic units (OTUs) were clustered at 97% sequence similarity. Relative abundances of each Coriobacteriia and the OMM12 species were obtained after BLAST search (97% sequence identity; 80% of the query sequence length; e-value < 1-25) against the corresponding reference
Proteomics
Proteins from liver samples were extracted by adding 800 µL ice-cold lysis buffer [150 mM NaCl, 10 mM Tris (pH 7.2), 0.1% SDS, 1% Triton X-100, 1% deoxycholate, 5 mM EDTA, pH 7.4, cOmpleteTM protease inhibitor] to frozen samples, followed by bead milling (10 min, frequency 30) and 1 h incubation on ice. Lysates were centrifuged (12,000 rpm, 15 min, 4 °C), supernatants were recovered, and protein concentration was determined by DC protein assay (BIO-RAD) according to the manufacturer’s instructions.
For each sample, 20 µg protein lysate was used for sample preparation, as described previously[35]. Briefly, proteins were reduced with TCEP [tris(2-carboxyethyl)phosphine hydrochloride] and alkylated with iodoacetamide (IAA). A protein clean-up was conducted using paramagnetic beads in 70% ethanol and acetonitrile (ACN) without acidification of the samples, as described previously[35]. Proteins were then proteolytically cleaved using Trypsin (Promega) in a 1:50 ratio (trypsin:protein) for 16 h at 37 °C. Next, samples were labelled using 5× tandem mass tags (TMT, TMT-10plex, Thermo Scientific, USA) for 1 h at room temperature, followed by adding 1 µL 5% (v/v) hydroxylamine in 100 mM TEAB to quench the labelling. Samples were combined into 7 mixes, each containing 1 replicate of the different treatments. After peptide clean-up with 100% ACN, peptides were eluted in 2 fractions using 87% ACN in 10 mM ammonium formate (pH 10, Sigma Aldrich) for fraction 1 and 2% dimethylsulfoxide (DMSO, Sigma Aldrich) for fraction 2.
LC-MS/MS analysis was then performed using an UltiMate 3000 RSLCnano system (Dionex, USA), coupled online to a Q Exactive HF mass spectrometer (Thermo Fisher Scientific, USA) by a chip-based electrospray ionisation source (TriVersa NanoMate, Advion, USA), as previously described[35,36]. First, peptides were loaded on a trapping column with 5 μL/min by using 98% water/2% ACN/0.05% trifluoroacetic acid (Acclaim PepMap 100 C18, 3 μm, nanoViper, 75 μm × 5 cm, Thermo Fisher Scientific, Germany) and peptides were then separated on an analytical column (Acclaim PepMap 100 C18, 3 μm, nanoViper,
MS raw data were processed against the Mus musculus UniProtKB reference proteome from 16th November 2021 using ProteomeDiscoverer 2.2 with carbamidomethylation. TMT are set as fixed, and methionine oxidation and acetylation of protein N-terminus are set as variable modifications. Reporter ion intensities were corrected according to the manufacturer’s instructions. A total of 1,914 proteins were identified. Samples were normalised against a pool control containing a mixture of all samples included in each TMT mix. Samples were filtered for proteins quantified in at least four replicates per condition, resulting in 1,710 proteins.
Metabolomics
Samples were prepared, measured, and analysed as described by Pedersen et al.[37], using a method adapted from Krause et al.[38]. The luminal content in the entire colon was mixed with 5× ACN:H2O solvent and
For measurement, 10 or 5 µL (positive and negative ionisation, respectively) of each extract was injected into a HPLC system coupled online with a 6546 UHD Accurate-Mass Q-TOF (Agilent Technologies). Metabolites were separated with an Agilent Zorbax Eclipse Plus C18 column (2.1 × 100 mm, 1.8 µm) equipped with a matching pre-column (2.1 × 50 mm, 1.8 µm). The autosampler was kept at 5 °C and column oven set to 45 °C. Separation was achieved using a binary solvent system of A (0.1% FA in water) and B (0.1% FA in ACN). The gradient was as follows: 0-5.5 min: 1% B; 5-20 min: 1%-100% B; 20-22 min: 100% B; 22-22.5: 100%-1% B; 22.5-25 min: 1% B. Metabolites were eluted at a constant flow rate of
The spectral data (.d files) were imported into the Progenesis QI software (Non-Linear Dynamics, Milford, MA, USA). The two ionisation modes were analysed separately. The adduct ions were [M+H], [M+H-H2O] for positive mode and [M-H], [M-H2O-H] and [M+FA-H] for negative mode. Chromatograms were aligned using an automatically chosen reference chromatogram from the dataset. The following software-guided peak-picking tool resulted in a data matrix including retention time, mass-to-charge ratio, and corresponding normalised peak area. For the in-silico database search, the Fecal Metabolome Database was used as a resource. After exporting the results (compound measurement and putative identifications) for all measured compounds, the data was further processed by only keeping peaks that were putatively identified with an MS2 fragment spectrum and a minimum Progenesis score of 40. Only those annotations with the highest Progenesis score were kept if peaks were annotated to multiple compounds.
Statistics
For microbiota data, statistics were calculated using Rhea[34] in R or using GraphPad Prism (Graphpad Software, Inc) to perform the specific tests mentioned throughout the text and figures. For proteomics, after log2-transformation, fold changes were calculated relative to the respective controls with adjusted P-values calculated using the Benjamini-Hochberg method. Proteins were considered significantly altered with an adjusted P-value ≤ 0.05. The analysis was conducted in R-3.5.0 using the following packages: mixOmics[39], ggplot2[40], qpcR[41], corrplot[42], PerformanceAnalytics[43], calibrate[44], dendsort[45], dendextend[46], ComplexHeatmap[47], limma[48], plyr[49], reshape2[50], xlsx[51], DEP[52], ggsci[53], ggpubr[54], pheatmap[55], circlize[56]. Principal component analysis of metabolome peaks was done in R with the significance of group differences calculated by PERMANOVA using the adonis2 function in the R package vegan[57]. Differences in annotated metabolites were calculated in R using a Kruskal-Wallis test with correction for multiple testing by Benjamini-Hochberg method followed by post hoc Dunn’s test for pairwise comparisons.
RESULTS
E. lenta colonised the intestine of OMM12 mice
Germfree mice were colonised with cryopreserved caecal suspensions of OMM12 mice with or without Coriobacteriia. At the end of the experiment (18 weeks of age; ca. 12 weeks of colonisation), the presence of each species in caecum was evaluated by 16S rRNA gene amplicon sequencing, which delivered 13,202 ± 4,266 high-quality, chimera-checked sequences per sample. Of the four Coriobacteriia, only E. lenta colonised the intestine.
Beta-diversity analysis based on generalised UniFrac distances revealed that the microbial communities mainly differed due to the presence or absence of E. lenta (P = 0.001, PERMANOVA; Figure 1A). Effects of the diets (BAs and HFD) on the overall phylogenetic makeup were more pronounced in the mice colonised with E. lenta, reaching statistical significance only in the case of BA supplementation in HFD (E.L HFD vs. E.L HFD-BA, adj. P = 0.0482) [Figure 1B]. BAs also affected microbiota profiles in the caecum of OMM12 mice (MM CD vs. MM CD-BA, adj. P = 0.028). All other pairwise comparisons between diet groups were not statistically significant (adj. P > 0.05).
Figure 1. E. lenta colonised the caecum of OMM12 mice. (A) Beta-diversity analysis of colonisation groups shown as a multi-dimensional scaling plot of generalised UniFrac distances (P = 0.001; PERMANOVA); (B) Same as in panel (A), visualised per colonisation/diet groups (P = 0.001; PERMANOVA); (C) Bacterial composition in caecum of gnotobiotic mice (16S rRNA gene amplicon sequencing). Data plotted as dots representing individual mice; black bars indicate median values. B. animalis and A. muris were not detected. Statistics: strains detected in > 50% of the mice in at least one diet group were compared statistically using Mann-Whitney tests with Benjamini-Hochberg adjustment. Stars indicate significant differences between colonisation groups (*adj. P < 0.05; **adj.
All four Coriobacteriia species were detected in the gavage solution used to colonise the mice: E. lenta (6.3% relative abundance), L. parvula (4.8%), C. aerofaciens (3.5%), A. mucosicola (1.6%), OMM12 strains (83.9%) [Supplementary Figure 2]. In contrast, only E. lenta was detected in mice at a median relative abundance of 27.5%, without significant differences between the diets due to marked inter-individual differences [Figure 1C]. Similar results were obtained in small intestinal content, i.e., E. lenta was the only Coriobacteriia detected (data not shown).
Ten of the twelve OMM12 strains were detected by sequencing in the gavage solutions [Supplementary Figure 2]. Bifidobacterium animalis DSM 26074 (= YL2) and Acutalibacter muris DSM 26090T (= KB18) were not detected, as reported in previous mouse studies[29,59]. The same ten OMM12 strains were detected in the mouse caecal contents [Figure 1C]. Akkermansia muciniphila DSM 26127 (= YL44) was the most dominant species (> 30% relative abundance in most mice), followed by Enterococcus faecalis DSM 32036
E. lenta did not significantly alter host parameters and liver proteomes
Weight of the caecum, body, liver, and total WAT as well as fasting blood glucose and plasma cholesterol levels were not significantly altered by E. lenta colonisation [Figure 2A]. Caecum weight was significantly influenced by diet in E. lenta-colonised mice; BA supplementation was associated with smaller caeca. Additional colonisation-specific effects due to diet included: (i) highest body weight and cholesterol levels in OMM12 mice fed the HFD-BA and HFD, respectively, although results did not reach statistical significance; (ii) low liver weight in E. lenta-colonised mice fed the HFD-BA diet.
Figure 2. Colonisation with E. lenta had no significant effects on the host. (A) Weight of the caecum, body, liver, and total WAT as well as blood levels of fasting glucose and cholesterol. Data plotted as dots representing individual mice. Within each group, mice housed in different cages are indicated with empty or filled circles. Black bars show median values. Statistics: Kruskal-Wallis test followed by Dunn’s test for multiple comparisons. Stars indicate significant differences between diets (*adj. P < 0.05; **adj. P < 0.01); (B) PCA of liver proteomes per colonisation group (P = 0.582; PERMANOVA); (C) Same as in panel (B), shown per diet groups (P = 0.001; PERMANOVA). Panel (A) of this figure was published in the PhD thesis by co-author Theresa Streidl[58]. WAT: White adipose tissue; PCA: principal component analysis; MM: mice colonised with the mouse synthetic community OMM12; E.L: mice colonised with OMM12 and Coriobacteriia; CD: control diet; CD-BA: control diet supplemented with 0.2% primary bile acids; HFD: lard-based high-fat diet; HFD-BA: HFD supplemented with 0.2% primary bile acids.
Principal component analysis (PCA) of liver proteomes showed no significant differences between colonisation groups (P = 0.582, PERMANOVA) [Figure 2B]. In contrast, the different diets led to significant differences in the proteome (P = 0.001, PERMANOVA) [Figure 2C]. When comparing fold-changes of single proteins between OMM12 and E. lenta-colonised mice for each of the four diet groups, no significantly different proteins were identified. In contrast, significant effects were triggered by the diet, especially fat content [Supplementary Figure 3]. Data on individual proteins and differences for all comparisons are available in Supplementary Table 1. When comparing the HFD-fed mice to those fed the corresponding diet without additional fat (i.e., HFD vs. CD and HFD-BA vs. CD-BA), consistent changes were observed in both colonisation groups. Fatty acid-binding protein 5 (UniProtKB ID: Q05816) had the highest negative Log2(FC) (-4.23 to -4.84). Cytoplasmic aspartate aminotransferase (UniProtKB ID: P05201), ATP-citrate synthase (UniProtKB ID: Q3V117), and thyroid hormone-inducible hepatic protein (UniProtKB ID: Q62264) were consistently under the top-ten downregulated proteins in the HFD groups. Cholesteryl ester hydrolase (UniProtKB ID: Q9Z0M5) was consistently under the top-ten upregulated proteins in the HFD groups [Log2(FC) 3.28 to 4.69]. BA supplementation also led to significant changes in liver proteomes. In E. lenta-colonised mice fed the CD-BA diet, peroxisomal acyl-coenzyme A oxidase 1 (UniProtKB ID: Q9R0H0) was the most substantially downregulated protein, while corticosteroid 11-beta-dehydrogenase isozyme 1 (UniProtKB ID: P50172) was strongly upregulated.
E. lenta colonisation affected metabolomes in the colon
Next, we examined the functional effect of E. lenta colonisation in the distal gut using non-targeted metabolomics. A total of 381 and 576 metabolite peaks were identified in the positive and negative ionisation modes, respectively. Of those metabolite peaks, 71 and 87 metabolites could be annotated, respectively. The peak intensity for all annotated metabolites is provided in Supplementary Tables 2 and 3. Heatmaps with individual z-scores for all annotated metabolites are provided in Supplementary Figures 4 and 5.
PCA of all detected metabolite peaks revealed significant differences between the colonisation groups in the positive (P = 0.001, PERMANOVA) but not negative (P = 0.548) ionisation mode [Figure 3A and E]. Clear significant differences (P = 0.001) in the overall metabolite landscape were observed between mice fed the different diets, independent of the ionisation mode [Figure 3B and F]. The occurrence of single annotated metabolites in the colon of E. lenta-colonised vs. OMM12 mice within each diet group was then compared using a Kruskal-Wallis test followed by pairwise comparisons (Dunn’s test) [Figure 3C and G]. In total, 33 (positive mode) and 32 (negative mode) of the annotated metabolites showed significant changes due to colonisation in at least one diet group. Interestingly, two (positive mode) and four (negative mode) metabolites were characterised by consistent changes between the mice across multiple diets (≥ 3) (Figure 3D and H; marked in grey in Figure 3C and G). While latifolicinin C acid was the only metabolite that was elevated in E. lenta-colonised vs. OMM12 mice (CD, HFD, and HFD-BA diet), creatine (detected in both ionisation modes), sarcosine, N,N-dimethylarginine, and N-Acetyl-DL-methionine were significantly reduced in E. lenta-colonised mice.
Figure 3. Colonisation with E. lenta significantly changed metabolomes in the colon. PCA of all identified metabolite peaks detected in positive ionisation according to (A) colonisation, and (B) diet; (C) Annotated metabolites with significant differences between E.L and MM mice within at least one dietary group as measured in positive ionisation mode. Individual values for the metabolites in grey boxes are shown in panel (D). The symbols after metabolite names indicate those detected in the two ionisation modes and statistically significant in both (*) or only in the specific ionisation mode (#). The strength of significance is shown with a colour gradient (pale yellow to brown). The first coloured column indicates significance (adjusted P-values) according to multi-group comparisons (Kruskal-Wallis with Benjamini-Hochberg adjustment). The remaining columns indicate the results of Dunn’s test for post hoc comparisons between E.L and MM mice per diet. The grey arrows indicate the direction of changes as specified in the figure legend; (D) Peak intensity of the individual metabolites showing significant pairwise comparisons between the two colonisation groups in at least 3 of the 4 diets. Dots represent the values for individual mice; black bars indicate median values; within each group, mice housed in different cages are indicated with empty or filled circles. Statistics: **adj. P < 0.01, ****adj. P < 0.0001, Kruskal-Wallis; for each metabolite, different letters indicate significance between the corresponding groups (Dunn’s test); (E-H) Same as in panels (A-D) for the negative ionisation mode. PCA: Principal component analysis; E.L: mice colonised with OMM12 and Coriobacteriia; MM: mice colonised with the mouse synthetic community OMM12; CD: control diet; CD-BA: control diet supplemented with 0.2% primary bile acids; HFD: lard-based high-fat diet; HFD-BA: HFD supplemented with 0.2% primary bile acids; OMM12: Oligo-Mouse Microbiota.
DISCUSSION
In this work, we investigated the effects of the dominant human gut bacterial species E. lenta on microbial communities and on the host under controlled conditions in gnotobiotic mice.
The three Coriobacteriia species C. aerofaciens, L. parvula, and A. mucosicola were not detected by amplicon sequencing. While we cannot exclude that they formed subdominant populations, E. lenta was a clear dominant member of the synthetic community in the two gut regions tested. Previous work highlighted that the host origin of strains influences the ability to colonise the mouse intestine[60,61]. This may apply to
Although E. lenta has important metabolic functions and colonised at high relative abundances, its colonisation did not result in strong effects on the investigated host parameters. Hence, we could not experimentally confirm previous observational data linking Coriobacteriia and E. lenta to host metabolism. We observed a trend towards lower blood cholesterol levels in E. lenta-colonised vs. OMM12 mice across the different diet groups; however, this was not statistically significant. Martinez et al. reported a high positive association between the occurrence of Coriobacteriia (as measured by pyrosequencing of 16S rRNA gene amplicons) and non-HDL plasma cholesterol levels after dietary intervention in a hamster model of hypercholesterolemia[64]. However, the underlying species were unclear. In 2011, Claus et al. reported a strong and positive association between the occurrence of E. lenta and hepatic triglyceride levels following 20 days of reconventionalisation of 8-week-old female germfree mice[65]. In our study, liver proteomic data did not hint at major changes in liver functions due to E. lenta. In contrast, fat and primary bile acids in the diet had a significant influence on liver proteomes. For example, proteins involved in the arginine biosynthesis pathway were downregulated in mice fed diets with high amounts of fat.
Marked inter-individual differences between mice within some of the dietary groups were observed, which may have masked effects due to colonisation; this is an important limitation of this study. We specifically used an experimental design that included multiple litters of mice and two cages per group to account for these potential confounding factors. While the clear split between mice within the MM/HFD groups in terms of WAT mass and body weight was linked to cage effects, this was not the case, for example, regarding cholesterol in the E.L/HFD group, fasting glucose in the E.L/CD-BA group, and WAT mass in the E.L/HFD-BA group. The two diets enriched in fat changed multiple parameters significantly, including proteomes in the liver and metabolomes in the gut of all mice, elevated body weight (up to +10 g) and white adipose tissue (up to +5 g) in several mice, and increased blood cholesterol in OMM12 mice. Hence, there is no concern about the diets themselves (e.g., composition) or the success of the feeding protocol. Reasons behind the observed inter-individual differences are unclear, but may include differences within a single cage, e.g., differing feed access due to rivalry/hierarchy between male mice.
Liver weight was reduced significantly in mice colonised with E. lenta and fed primary bile acids in the HFD. Differences between the other groups were not significant. We observed a similar effect of bile acids being associated with decreased liver weights in other experiments; the mechanism underlying this effect requires further investigation and is out of the scope of this study. With respect to metabolic responses of the mice altogether, two factors are worth noting: (i) mice were fed for 8 weeks only, with a HFD containing 48% energy content from fat (compared with 60% in other studies); the development of fatty liver in
Colonisation with E. lenta altered the levels of specific metabolites in the mouse colon: latifolicinin C acid was elevated, while creatine, sarcosine, N,N-dimethylarginine, and N-Acetyl-DL-methionine were reduced. It was remarkable that these changes were consistent across all groups of mice, as they were fed very different diets (exceptions were latifolicinin C acid and N-Acetyl-DL-methionine, which were not significantly different in mice fed the CD-BA and HFD-BA diet, respectively). Multiple other metabolite changes were observed, but were dependent on the diet fed to the mice. The elevated levels of latifolicinin C acid observed in E. lenta-colonised mice are in agreement with the literature, as latifolicinin C acid is a metabolite of tyrosine degradation, which can be produced by multiple gut bacteria, including E. lenta[66,67]. The decreased colonic levels of creatine suggest that E. lenta can degrade it. Gut bacteria have been reported to express enzymes involved in creatinine and creatine degradation[68]. The gene encoding for creatininase, which catalyses the reversible conversion of creatinine to creatine, was detected in the genome of E. lenta (GCA_000024265.1_01172, WP_009304706.1). Creatine can be further metabolised to urea, as well as sarcosine which can be further degraded to glycine[68]. Sarcosine was also significantly decreased in E. lenta-colonised mice. However, genes encoding the enzymes that catalyse these reactions were not found in the
In conclusion, this study reveals that the addition of the dominant gut bacterial species E. lenta to a synthetic community led to significantly altered metabolomes in the mouse colon. Effects of the specific metabolites on the host remain to be determined. While the omics data provided is descriptive, the gnotobiotic setting of this study provides new insights into the causal role of E. lenta within the gut in vivo. Studying the effects of a broader range of Coriobacteriia species will require refined colonisation strategies. Regarding E. lenta, the advent of genetic tools and phages available for targeted manipulations will accelerate the pace of discoveries on its role in gut microbiome-host interactions[78-80].
DECLARATIONS
Acknowledgments
We thank members of: (i) the Institute of Animal Science, University Hospital of RWTH Aachen, for measurements of cholesterol in the mouse blood; and (ii) the ZIEL Core Facility Microbiome at the Technical University of Munich for support with the animal experiment and for 16S rRNA gene amplicon sequencing. We are grateful to Amy Coates and Susan Jennings (Functional Microbiome Research Group, Institute of Medical Microbiology, University Hospital of RWTH Aachen) for language editing.
Authors’ contributions
Designed the studies: Viehof A, Streidl T, Clavel T
Performed the animal experiments: Streidl T
Performed experiments: Viehof A, Streidl T, Schubert K, Engelmann B, Haange SB
Analysed and curated data: Viehof A, Haange SB, Engelmann B, Streidl T, Schubert K, Rolle-Kampczyk U
Methods and resources: Haange SB, Schubert K, Rolle-Kampczyk U
Interpreted data: Viehof A, Streidl T, Haange SB, Schubert K, Rolle-Kampczyk U, von Bergen M, Clavel T
Provided materials and infrastructure: Haller D, von Bergen M, Clavel T
Secured funding: von Bergen M, Clavel T
Supervised the project: Clavel T
Created figures: Viehof A, Streidl T, Haange SB
Reviewed and corrected the figures: Viehof A, Streidl T, Clavel T
Wrote the manuscript: Viehof A, Clavel T
All authors reviewed the manuscript.
Availability of data and materials
Raw data from the 16S rRNA gene amplicon analysis was submitted to ENA (project PRJEB67952). The mass spectrometry proteomics data have been deposited to the ProteomeXchange Consortium via the PRIDE partner repository[81] and can be accessed using the identifier PXD047016 and 10.6019/PXD047016. Metabolomics data are available at the NIH Common Fund’s National Metabolomics Data Repository (NMDR) website, the Metabolomics Workbench[82] (https://www.metabolomicsworkbench.org), where it has been assigned Study ID ST003011. The data can be accessed directly via its Project DOI (http://dx.doi.org/10.21228/M8GM8G).
Financial support and sponsorship
von Bergen M and Clavel T received funding from the German Research Foundation (DFG), Project No. 403224013 - SFB1382. von Bergen M also received funding from the Novo Nordisk Foundation (grant NNF21OC0066551).
Conflicts of interest
All authors declared that there are no conflicts of interest.
Ethical approval and consent to participate
The mouse studies performed in this work were approved by the German government (Regierung von Oberbayern, approval No. 55.2-1-54-2532-156-2013).
Consent for publication
Not applicable.
Copyright
© The Author(s) 2024.
Supplementary Materials
REFERENCES
1. Clavel T, Ecker J. Microbiome and diseases: metabolic disorders. In: Haller D, editor. The gut microbiome in health and disease. Cham: Springer; 2018. pp. 251-77.
2. Koh A, Bäckhed F. From association to causality: the role of the gut microbiota and its functional products on host metabolism. Mol Cell 2020;78:584-96.
3. Kübeck R, Bonet-Ripoll C, Hoffmann C, et al. Dietary fat and gut microbiota interactions determine diet-induced obesity in mice. Mol Metab 2016;5:1162-74.
4. Hanssen NMJ, de Vos WM, Nieuwdorp M. Fecal microbiota transplantation in human metabolic diseases: from a murky past to a bright future? Cell Metab 2021;33:1098-110.
5. Rinott E, Youngster I, Yaskolka Meir A, et al. Effects of diet-modulated autologous fecal microbiota transplantation on weight regain. Gastroenterology 2021;160:158-73.e10.
6. Sharpton SR, Schnabl B, Knight R, Loomba R. Current concepts, opportunities, and challenges of gut microbiome-based personalized medicine in nonalcoholic fatty liver disease. Cell Metab 2021;33:21-32.
7. Cani PD, Depommier C, Derrien M, Everard A, de Vos WM. Akkermansia muciniphila: paradigm for next-generation beneficial microorganisms. Nat Rev Gastroenterol Hepatol 2022;19:625-37.
9. Fei N, Zhao L. An opportunistic pathogen isolated from the gut of an obese human causes obesity in germfree mice. ISME J 2013;7:880-4.
10. Hitch TCA, Afrizal A, Riedel T, et al. Recent advances in culture-based gut microbiome research. Int J Med Microbiol 2021;311:151485.
11. Afrizal A, Jennings SAV, Hitch TCA, et al. Enhanced cultured diversity of the mouse gut microbiota enables custom-made synthetic communities. Cell Host Microbe 2022;30:1630-45.e25.
13. Clavel T, Lepage P, Charrier C. The family coriobacteriaceae. In: Rosenberg E, Delong EF, Lory S, Stackebrandt E, Thompson F, editors. The prokaryotes. Berlin: Springer; 2014. pp. 201-38.
14. Gupta RS, Chen WJ, Adeolu M, Chai Y. Molecular signatures for the class Coriobacteriia and its different clades; proposal for division of the class Coriobacteriia into the emended order Coriobacteriales, containing the emended family Coriobacteriaceae and Atopobiaceae fam. nov., and Eggerthellales ord. nov., containing the family Eggerthellaceae fam. nov. Int J Syst Evol Microbiol 2013;63:3379-97.
15. Qin J, Li R, Raes J, et al. MetaHIT Consortium. A human gut microbial gene catalogue established by metagenomic sequencing. Nature 2010;464:59-65.
16. Bokkenheuser VD, Winter J, Dehazya P, Kelly WG. Isolation and characterization of human fecal bacteria capable of
17. Harris SC, Devendran S, Méndez-García C, et al. Bile acid oxidation by Eggerthella lenta strains C592 and DSM 2243T. Gut Microbes 2018;9:523-39.
18. Rekdal V, Bess EN, Bisanz JE, Turnbaugh PJ, Balskus EP. Discovery and inhibition of an interspecies gut bacterial pathway for Levodopa metabolism. Science 2019;364:eaau6323.
19. Maini Rekdal V, Nol Bernadino P, Luescher MU, et al. A widely distributed metalloenzyme class enables gut microbial metabolism of host- and diet-derived catechols. Elife 2020;9:e50845.
20. Clavel T, Doré J, Blaut M. Bioavailability of lignans in human subjects. Nutr Res Rev 2006;19:187-96.
21. Bess EN, Bisanz JE, Yarza F, et al. Genetic basis for the cooperative bioactivation of plant lignans by Eggerthella lenta and other human gut bacteria. Nat Microbiol 2020;5:56-66.
22. Haiser HJ, Gootenberg DB, Chatman K, Sirasani G, Balskus EP, Turnbaugh PJ. Predicting and manipulating cardiac drug inactivation by the human gut bacterium Eggerthella lenta. Science 2013;341:295-8.
23. Alexander M, Ang QY, Nayak RR, et al. Human gut bacterial metabolism drives Th17 activation and colitis. Cell Host Microbe 2022;30:17-30.e9.
24. Koh A, Molinaro A, Ståhlman M, et al. Microbially produced imidazole propionate impairs insulin signaling through mTORC1. Cell 2018;175:947-61.e17.
25. Qin J, Li Y, Cai Z, et al. A metagenome-wide association study of gut microbiota in type 2 diabetes. Nature 2012;490:55-60.
26. Forslund K, Hildebrand F, Nielsen T, et al. MetaHIT consortium. Disentangling type 2 diabetes and metformin treatment signatures in the human gut microbiota. Nature 2015;528:262-6.
27. Yun Y, Kim HN, Kim SE, et al. Comparative analysis of gut microbiota associated with body mass index in a large Korean cohort. BMC Microbiol 2017;17:151.
28. Fu J, Bonder MJ, Cenit MC, et al. The gut microbiome contributes to a substantial proportion of the variation in blood lipids. Circ Res 2015;117:817-24.
29. Brugiroux S, Beutler M, Pfann C, et al. Genome-guided design of a defined mouse microbiota that confers colonization resistance against Salmonella enterica serovar Typhimurium. Nat Microbiol 2016;2:16215.
30. Pjevac P, Hausmann B, Schwarz J, et al. An economical and flexible dual barcoding, two-step PCR approach for highly multiplexed amplicon sequencing. Front Microbiol 2021;12:669776.
31. Klindworth A, Pruesse E, Schweer T, et al. Evaluation of general 16S ribosomal RNA gene PCR primers for classical and next-generation sequencing-based diversity studies. Nucleic Acids Res 2013;41:e1.
32. Edgar RC. UPARSE: highly accurate OTU sequences from microbial amplicon reads. Nat Methods 2013;10:996-8.
33. Lagkouvardos I, Joseph D, Kapfhammer M, et al. IMNGS: A comprehensive open resource of processed 16S rRNA microbial profiles for ecology and diversity studies. Sci Rep 2016;6:33721.
34. Lagkouvardos I, Fischer S, Kumar N, Clavel T. Rhea: a transparent and modular R pipeline for microbial profiling based on 16S rRNA gene amplicons. PeerJ 2017;5:e2836.
35. Wang Z, Karkossa I, Großkopf H, et al. Comparison of quantitation methods in proteomics to define relevant toxicological information on AhR activation of HepG2 cells by BaP. Toxicology 2021;448:152652.
36. Streidl T, Karkossa I, Segura Muñoz RR, et al. The gut bacterium Extibacter muris produces secondary bile acids and influences liver physiology in gnotobiotic mice. Gut Microbes 2021;13:1-21.
37. Pedersen KJ, Haange SB, Žížalová K, et al. Eggerthella lenta DSM 2243 alleviates bile acid stress response in clostridium ramosum and anaerostipes caccae by transformation of bile acids. Microorganisms 2022;10:2025.
38. Krause JL, Haange SB, Schäpe SS, et al. The glyphosate formulation Roundup® LB plus influences the global metabolome of pig gut microbiota in vitro. Sci Total Environ 2020;745:140932.
39. Rohart F, Gautier B, Singh A, Lê Cao KA. mixOmics: an R package for ’omics feature selection and multiple data integration. PLoS Comput Biol 2017;13:e1005752.
40. Wickham H. ggplot2: elegant graphics for data analysis. Springer: New York; 2016. Available from: https://link.springer.com/book/10.1007/978-3-319-24277-4. [Last accessed on 16 Jan 2024].
41. Spiess AN. qpcR: modelling and analysis of real-time PCR data. 2018. Available from: https://cran.r-project.org/web/packages/qpcR/index.html. [Last accessed on 16 Jan 2024].
42. Wei T, Simko V, Xie Y, et al. R package “corrplot”: visualization of a correlation matrix 2017. Available from: https://github.com/taiyun/corrplot. [Last accessed on 16 Jan 2024].
43. Peterson BG, Carl P, Pulkit, et al. PerformanceAnalytics: econometric tools for performance and risk analysis. 2014. Available from: https://github.com/braverock/PerformanceAnalytics. [Last accessed on 16 Jan 2024].
44. Graffelman J, van Eeuwijk F. Calibration of multivariate scatter plots for exploratory analysis of relations within and between sets of variables in genomic research. Biom J 2005;47:863-79.
45. Sakai R, Winand R, Verbeiren T, Moere AV, Aerts J. dendsort: modular leaf ordering methods for dendrogram representations in R. F1000Res 2014;3:177.
46. Galili T. dendextend: an R package for visualizing, adjusting and comparing trees of hierarchical clustering. Bioinformatics 2015;31:3718-20.
47. Gu Z, Eils R, Schlesner M. Complex heatmaps reveal patterns and correlations in multidimensional genomic data. Bioinformatics 2016;32:2847-9.
48. Ritchie ME, Phipson B, Wu D, et al. limma powers differential expression analyses for RNA-sequencing and microarray studies. Nucleic Acids Res 2015;43:e47.
51. Dragulescu A, Arendt C. xlsx: read, write, format excel 2007 and excel 97/2000/XP/2003 files. 2020. Available from: https://cran.r-project.org/web/packages/xlsx/index.html. [Last accessed on 16 Jan 2024].
52. Zhang X, Smits AH, van Tilburg GB, Ovaa H, Huber W, Vermeulen M. Proteome-wide identification of ubiquitin interactions using UbIA-MS. Nat Protoc 2018;13:530-50.
53. Xiao N, Cook J, Jégousse C, Li M. ggsci: scientific journal and Sci-Fi themed color palettes for “ggplot2”. 2023. Available from: https://cran.r-project.org/web/packages/ggsci/index.html. [Last accessed on 16 Jan 2024].
54. Kassambara A. ggpubr: “ggplot2” based publication ready plots. 2023. Available from: https://rpkgs.datanovia.com/ggpubr/. [Last accessed on 16 Jan 2024].
55. Kolde R, Taunometsalu, Lizee A. Pheatmap: pretty heatmaps. 2012. Available from: https://github.com/raivokolde/pheatmap. [Last accessed on 16 Jan 2024].
56. Gu Z, Gu L, Eils R, Schlesner M, Brors B. circlize implements and enhances circular visualization in R. Bioinformatics 2014;30:2811-2.
57. Oksanen J, Simpson G, Blanchet FG, et al. vegan: community ecology package. R package version 2.6-4. 2022. Available from: https://cran.r-project.org/web/packages/vegan/index.html. [Last accessed on 16 Jan 2024].
58. Streidl T. Effects of the bacterial conversion of lipids in the gut on mouse metabolism. RWTH Aachen University; 2021.
59. Eberl C, Ring D, Munch PC, et al. Reproducible colonization of germ-free mice with the oligo-mouse-microbiota in different animal facilities. Front Microbiol 2019;10:2999.
60. Frese SA, Mackenzie DA, Peterson DA, et al. Molecular characterization of host-specific biofilm formation in a vertebrate gut symbiont. PLoS Genet 2013;9:e1004057.
61. Seedorf H, Griffin NW, Ridaura VK, et al. Bacteria from diverse habitats colonize and compete in the mouse gut. Cell 2014;159:253-66.
62. Kasahara K, Krautkramer KA, Org E, et al. Interactions between Roseburia intestinalis and diet modulate atherogenesis in a murine model. Nat Microbiol 2018;3:1461-71.
63. Kovatcheva-Datchary P, Shoaie S, Lee S, et al. Simplified intestinal microbiota to study microbe-diet-host interactions in a mouse model. Cell Rep 2019;26:3772-83.e6.
64. Martínez I, Wallace G, Zhang C, et al. Diet-induced metabolic improvements in a hamster model of hypercholesterolemia are strongly linked to alterations of the gut microbiota. Appl Environ Microbiol 2009;75:4175-84.
65. Claus SP, Ellero SL, Berger B, et al. Colonization-induced host-gut microbial metabolic interaction. mBio 2011;2:e00271-10.
66. Beloborodova NV, Khodakova AS, Bairamov IT, Olenin AY. Microbial origin of phenylcarboxylic acids in the human body. Biochemistry 2009;74:1350-5.
67. Beloborodova N, Bairamov I, Olenin A, Shubina V, Teplova V, Fedotcheva N. Effect of phenolic acids of microbial origin on production of reactive oxygen species in mitochondria and neutrophils. J Biomed Sci 2012;19:89.
69. Sperry JF, Wilkins TD. Arginine, a growth-limiting factor for Eubacterium lentum. J Bacteriol 1976;127:780-4.
70. Maria J, Vallance P, Charles IG, Leiper JM. MicroCorrespondence: identification of microbial dimethylarginine dimethylaminohydrolase enzymes. Mol Microbiol 1999;33:1278-9.
71. Böger RH, Bode-Böger SM, Szuba A, et al. Asymmetric dimethylarginine (ADMA): a novel risk factor for endothelial dysfunction: its role in hypercholesterolemia. Circulation 1998;98:1842-7.
72. Abbasi F, Asagmi T, Cooke JP, et al. Plasma concentrations of asymmetric dimethylarginine are increased in patients with type 2 diabetes mellitus. Am J Cardiol 2001;88:1201-3.
73. Miyazaki H, Matsuoka H, Cooke JP, et al. Endogenous nitric oxide synthase inhibitor: a novel marker of atherosclerosis. Circulation 1999;99:1141-6.
74. Surdacki A, Nowicki M, Sandmann J, et al. Reduced urinary excretion of nitric oxide metabolites and increased plasma levels of asymmetric dimethylarginine in men with essential hypertension. J Cardiovasc Pharmacol 1999;33:652-8.
75. Zairis MN, Patsourakos NG, Tsiaousis GZ, et al. Plasma asymmetric dimethylarginine and mortality in patients with acute decompensation of chronic heart failure. Heart 2012;98:860-4.
76. Jacobi J, Tsao PS. Asymmetrical dimethylarginine in renal disease: limits of variation or variation limits? A systematic review. Am J Nephrol 2008;28:224-37.
77. Noecker C, Sanchez J, Bisanz JE, et al. Systems biology elucidates the distinctive metabolic niche filled by the human gut microbe Eggerthella lenta. PLoS Biol 2023;21:e3002125.
78. Dong X, Guthrie BGH, Alexander M, et al. Genetic manipulation of the human gut bacterium Eggerthella lenta reveals a widespread family of transcriptional regulators. Nat Commun 2022;13:7624.
79. Sprotte S, Rasmussen TS, Cho GS, et al. Morphological and genetic characterization of Eggerthella lenta bacteriophage PMBT5. Viruses 2022;14:1598.
80. Bisanz JE, Soto-Perez P, Noecker C, et al. A genomic toolkit for the mechanistic dissection of intractable human gut bacteria. Cell Host Microbe 2020;27:1001-13.e9.
81. Perez-Riverol Y, Bai J, Bandla C, et al. The PRIDE database resources in 2022: a hub for mass spectrometry-based proteomics evidences. Nucleic Acids Res 2022;50:D543-52.
Cite This Article
Export citation file: BibTeX | RIS
OAE Style
Viehof A, Haange SB, Streidl T, Schubert K, Engelmann B, Haller D, Rolle-Kampczyk U, von Bergen M, Clavel T. The human intestinal bacterium Eggerthella lenta influences gut metabolomes in gnotobiotic mice. Microbiome Res Rep 2024;3:14. http://dx.doi.org/10.20517/mrr.2023.65
AMA Style
Viehof A, Haange SB, Streidl T, Schubert K, Engelmann B, Haller D, Rolle-Kampczyk U, von Bergen M, Clavel T. The human intestinal bacterium Eggerthella lenta influences gut metabolomes in gnotobiotic mice. Microbiome Research Reports. 2024; 3(2): 14. http://dx.doi.org/10.20517/mrr.2023.65
Chicago/Turabian Style
Alina Viehof, Sven-Bastiaan Haange, Theresa Streidl, Kristin Schubert, Beatrice Engelmann, Dirk Haller, Ulrike Rolle-Kampczyk, Martin von Bergen, Thomas Clavel. 2024. "The human intestinal bacterium Eggerthella lenta influences gut metabolomes in gnotobiotic mice" Microbiome Research Reports. 3, no.2: 14. http://dx.doi.org/10.20517/mrr.2023.65
ACS Style
Viehof, A.; Haange S.B.; Streidl T.; Schubert K.; Engelmann B.; Haller D.; Rolle-Kampczyk U.; von Bergen M.; Clavel T. The human intestinal bacterium Eggerthella lenta influences gut metabolomes in gnotobiotic mice. Microbiome. Res. Rep. 2024, 3, 14. http://dx.doi.org/10.20517/mrr.2023.65
About This Article
Copyright
Data & Comments
Data
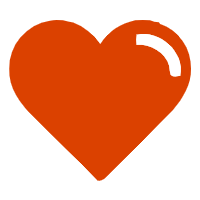

Comments
Comments must be written in English. Spam, offensive content, impersonation, and private information will not be permitted. If any comment is reported and identified as inappropriate content by OAE staff, the comment will be removed without notice. If you have any queries or need any help, please contact us at support@oaepublish.com.