Profiling the gut and oral microbiota of hormone receptor-positive, HER2-negative metastatic breast cancer patients receiving pembrolizumab and eribulin
Abstract
Aim: Changes in host-associated microbial communities (i.e., the microbiota) may modulate responses to checkpoint blockade immunotherapy. In the KELLY phase II study (NCT03222856), we previously demonstrated that pembrolizumab [anti-programmed cell death protein 1 (PD-1)] combined with eribulin (plus microtubule-targeting chemotherapy) showed encouraging antitumor activity in patients with hormone receptor (HR)-positive/human epidermal growth factor receptor 2 (HER2)-negative metastatic breast cancer (mBC) who had received prior treatments.
Methods: A total of 58 fecal and 67 saliva samples were prospectively collected from a subset of 28 patients at baseline (BL), after three treatment cycles, and end of treatment. Shotgun metagenomics, 16S rRNA gene amplicon sequencing, and bioinformatics and statistical approaches were used to characterize fecal and oral microbiota profiles.
Results: Treatment caused no substantial perturbations in gut or oral microbiota, suggesting minimal drug-related microbial toxicity. Bacteroides and Faecalibacterium were the dominant gut microbiota genera, while Prevotella and Streptococcus were present in both oral and gut samples, highlighting potential gut-oral microbial interactions. Additionally, clinical benefit (CB) appeared to be associated with gut-associated Bacteroides fragilis (B. fragilis) and a BL oral abundance of Streptococcus ≥ 30%. Notably, B. fragilis NCTC 9343 supernatant induced dose-dependent lactate dehydrogenase (LDH) release from the MCF-7 (HR-positive/HER2-negative) BC cell line.
Conclusion: These findings suggest that specific gut and oral microbiota may modulate the effectiveness of combinatory anti-BC therapies, potentially through the action of microbial metabolites.
Keywords
INTRODUCTION
Breast cancer (BC) is the most common cancer in women worldwide, with hormone receptor (HR)-positive (HR[+])/human epidermal growth factor receptor 2 (HER2)-negative (HER2[-]) BC being the most prevalent biological subtype[1]. Determining the optimal treatment for metastatic breast cancer (mBC) is complex and typically involves sequential therapies, often based on cytotoxic chemotherapy. These treatments carry an increased risk of cumulative toxicities, which are unique to each patient, and the potential for developing drug resistance.
Checkpoint inhibitor monotherapy has shown only modest activity in HR[+]/HER2[-] mBC[2]. However, the addition of pembrolizumab to standard neoadjuvant therapy was found to significantly improve the pathological complete response rate in HER2[-] early BC patients[3]. The KELLY trial (NCT03222856) evaluated the safety and efficacy of combining pembrolizumab with eribulin for the treatment of HR[+]/HER2[-] mBC. The KELLY trial demonstrated a clinical benefit (CB) rate of 56.8% [95%CI (confidence interval): 41.0%-71.7%] and a median progression-free survival (PFS) of 6 months (95%CI: 3.7-8.4 months)[4], exceeding the activity observed with eribulin monotherapy in a similar patient population[5].
Factors predicting responses to immunotherapy may be related to either the patient or the tumor. Over the past decade, numerous studies have explored the relationship between the gut microbiota and clinical outcomes in anti-cancer therapies[6-11]. Notably, higher gut diversity has been associated with better responses to anti-programmed cell death protein 1 (PD-1) immunotherapy in metastatic melanoma patients[11], and Bifidobacterium species and strains have been shown to enhance antitumor activity in anti-programmed cell death ligand 1 (PD-L1) therapies[6,7,12]. These changes in gut microbiota composition are thought to modulate immune response, potentially enhancing T-cell activity through the production of specific microbial metabolites[7,11,13-15].
While most studies have focussed on strongly immunogenic cancers such as melanoma, few have explored microbiota profiles in poorly immunogenic cancers such as BC[16]. Additionally, the majority of these studies focus on the gut microbiota’s correlation with anti-cancer therapies, with limited attention given to the oral microbiota. Emerging evidence suggests that bacterial dissemination between the oral and gut microbiota may disrupt the gut microbiota ecosystem, potentially leading to adverse health outcomes[17].
The CALADRIO study was an exploratory retrospective analysis aimed at evaluating the associations between both the oral and gut microbiota and clinical outcomes of patients included in the KELLY study.
METHODS
Study design and sample collection
The KELLY study (NCT03222856) was a phase II clinical trial assessing the safety and efficacy of a novel combination therapy of pembrolizumab and eribulin for HR[+]/HER2[-] mBC patients[4]. Eligible patients were ≥ 18 years old and had previously received hormone therapy and one to two lines of chemotherapy for advanced disease. The primary endpoint of the KELLY study was to determine the CB rate, defined as the percentage of patients experiencing a complete response, partial response or stable disease enduring equal to or more than 24 months, according to the Response Evaluation Criteria in Solid Tumors (RECIST) v1.1[4].
The CALADRIO study (NCT03222856, EUDRACT: 2016-004513-27) presented here is an exploratory retrospective analysis aiming to profile the oral and gut microbiota of a subset of patients enrolled in the KELLY study to establish if microbiota-associated profiles could be used as predictive biomarkers of responses and/or CB. Consenting patients from the KELLY study (n = 28) provided fecal and saliva samples at baseline (BL), after three treatment cycles [Cycle 4 Day 1, (C4D1)], and at end-of-treatment (EoT). A total of 65 fecal samples and 70 buccal samples were collected. Specifically, fecal samples collected were: BL 28/28, at C4D1 22/22, and at EoT 15/20, and all buccal samples were collected at all time points. Fecal samples were collected as stipulated by the International Human Microbiome Standard guidelines: SOP 03 V1[18], and stored in a deoxyribonucleic acid (DNA) preservation buffer.
All patients included in the study provided written informed consent (including biomarker analyses on biological samples) before any protocol-related activities started. The study protocol, patient information leaflet, and informed consent form were reviewed by each location’s Ethics Committee. The study was conducted in accordance with the Declaration of Helsinki, the International Council for Harmonization of Technical Requirements for Pharmaceuticals for Human Use Good Clinical Practice guidelines, and all applicable regulations and laws.
Sample processing and sequencing
Saliva samples (5 mL each) were collected from all patients into sterile containers, aliquoted, and stored at
Fecal samples were shipped to the Quadram Institute Bioscience, Norwich, United Kingdom, for processing. Processing and quantification of the fecal samples were done using the Promega Maxwell RSC PureFood GMO and Authentication kit (Promega, AS1600). A 200 µL aliquot of fecal sample was transferred into a Lysing Matrix E tube. Then, 1 mL of CTAB was added to the sample and vortexed for 30 s before being incubated on a heat block at 95 °C for 5 min, and then vortexed for a minute. Samples were homogenized in a FastPrep-24 machine for 45 s at a speed of 6.0 m/s. To each sample, 40 µL of Proteinase K and 20 µL of RNase A were added and vortexed to mix. The samples were heated to 70 °C for 10 min, while cartridges were prepared for the Maxwell robot according to the manufacturer’s instructions. DNA was quantified using Qubit 2.0 dsDNA BR assay kit (ThermoFisher Scientific, Q32850). For fecal samples, library preparation was done following the manufacturer’s instructions using the DNA flex kit with 8 mer UDI and then sent to Source Biosciences (Cambridge, United Kingdom) to be sequenced on the Novoseq platform, 2 × 250 bp up to 750 Gb. A total of 65 fecal samples were submitted for shotgun metagenomic sequencing. Four samples were below the minimum quality for downstream analysis and three samples were removed. One patient withdrew consent. We removed two other samples because the patient’s PD-L1 status could not be assessed due to low neoplastic cellularity.
For shotgun sequencing (fecal samples only), the mean read depth was 4.14 Gbp, the median number of reads of this dataset was 30,197,291 reads, and the mean number of reads per sample was 27,592,595. For 16S rRNA gene amplicon sequencing (saliva samples), the mean read depth was 37.2 Mbp, the median number of reads in this dataset was 122,973 reads, and the mean number of reads per sample was 12,378.
Bioinformatics and data analysis
16S rRNA gene amplicon sequencing assembly was done using Qiime v1.9.1 as previously described[20]. Once operational taxonomical units (OTUs) were assigned, biological observational matrix files were generated and data visualized on MEGAN v6.20.19[21] or on R[22] with the package “Phyloseq-v3.18”, and samples with less than 20,000 reads were removed. Data were normalized using variance stabilization with DeSeq2 to relative abundances (%)[23].
For metagenome shotgun sequencing, fastp v0.20.0[24] was used to run a quality filtering on the FASTQ reads with a -q 20. KneadData v0.10.0[25] was used to remove host-associated reads, i.e., human reads, using the database “GrCh38_noalt_decoy_as” using --bypass-trim, --reorder and --bypass-trf (found at: https://benlangmead.github.io/aws-indexes/bowtie). Metagenome sequences were co-assembled using Megahit v1.2.9[26], run via MetaWrap v1.3.2[27]. To assign taxonomy, Kraken2 v2.1.2[28] was used with a confidence level of 0.1 on the FASTQ reads and the output run through Bracken v2.6.2[29] to accurately estimate relative abundances with a threshold of 10. Metagenome assembled genomes (MAGs) were computationally extracted via MetaWrap v1.3.2 (Concoct v1.1.0[30-32], Maxbin2[33], and MetaBAT2[34]) based on metagenome sequencing reads and co-assemblies. Bin refinement was performed and only high-quality MAGs with the parameters > 80% completeness and < 10% contamination (via CheckM v1.1.3) were used for further analysis. GTDB-tk v 1.5.1[35] was utilized to assign taxonomy to the MAGs extracted at the species level.
For functional assignment, we used Humann3 v3.0.0[25] via Metaphlan v3.0.13[25] pipeline, using Chocophlan (downloaded 24 August 2021) as the database. The output file “humann_all_pathabundance_cpm.tsv” was normalized and then stratified before data analysis.
Data analysis was performed using RStudio 2022.07.1+554 “Spotted Wakerobin” Release and R version 4.2.2[22]. Alpha diversity was assessed by Shannon diversity index, while beta diversity was evaluated using non-metric distance scaling (NMDS) with the package “Vegan- 2.6-4”. Statistical significance of alpha diversity was performed using Kruskal-Wallis, while beta diversity was assessed with PERMANOVA using adonis2 as part of the “Vegan package v2.6-4”[36]. Linear discriminant analysis effect size (LEfSe) analysis followed the statistical analysis described in[37]. Plots and figures were created using “ggplot2-v3.4.3”. A P < 0.05 was taken to be significant. The statistical tests performed are specified in the figure legend.
To calculate the clustering for the heatmap, we used Bray-Curtis dissimilarity matrix for the vegdist function and average for the hclust function, i.e., sample and genus clustering, and hierarchal clustering, respectively. We used the Huttenhower LEfSe[37] Galaxy pipeline. For Bacteroides fragilis (B. fragilis) MAG screening, we used the METABOLIC pipeline as described in[38] and utilized Resfinder[39,40] to assess antibiotic resistance potential. Using the paper by Franco[41], we downloaded 22 genomic sequences to run through Abricate v1.0.1[42] and screen for the presence or absence of the bft gene [Table 1].
NCBI Accession numbers of the 22 genomic sequences for the bft gene
Accession number (Genbank) | Genome location (bp-bp), (c, chromosome) |
AB026626.1 | 345-1538 |
CP098482.1 | 2670205-2671398 |
CP098482.1 | c5312584-5311391 |
JAHYPF010000020.1 | 55345-56538 |
JALFMY010000010.1 | 81936-83129 |
JANUSS010000001.1 | 3806129-3807322 |
JANUTD010000001.1 | 2513275-2514468 |
JANUTH010000001.1 | 2513065-2514258 |
JANUTZ010000001.1 | 347095-348288 |
JANUTZ010000002.1 | 1981850-1983043 |
JANUUF010000001.1 | 2527119-2528312 |
JANUUF010000001.1 | 347004-348197 |
NZ_CP011073.1 | 4564579-4565772 |
NZ_CP098482.1 | c5312584-5311391 |
NZ_JAPUAE010000018.1 | 56787-57980 |
NZ_JGEF01000023.1 | 64376-65569 |
NZ_JH724206.1 | c3476585-3475392 |
NZ_JH724218.1 | c72677-71484 |
NZ_LIDS01000027.1 | 55915-57108 |
NZ_LIDT01000031.1 | c8881-7688 |
NZ_LIDV01000087.1 | c315654-314461 |
NZ_PDCT01000007.1 | c60762-59569 |
In vitro testing of B. fragilis supernatants with BC cell lines
To obtain soluble B. fragilis metabolites for testing in vitro, we cultured the type strain B. fragilis NCTC 9343 anaerobically (85%/5%/10% for N2/CO2/H2 respectively) at 37 °C. The supernatant of the cultures was harvested at exponential [14 h, optical density (OD) 1.01], late exponential (18 h, OD 1.13), stationary (26 h, OD 1.11), and death phase (36 h, OD 1.04) of growth and filter-sterilized (0.22 µm). Sterile aliquots were kept at -20 °C until further use. MCF-7 BC cells were seeded at a density of 9,000 cells per well in a 48-well plate in complete media (DMEM high-glucose, no phenol red, supplemented with 5% fetal bovine serum, 1% L-glutamine, 1% Pen/Strep, 1% sodium pyruvate). The cells were exposed to the cell-free bacterial supernatant (CFS) at 1:5 dilution for various time points (2 h up to 36 h) before being replaced with minimal media (DMEM high-glucose, no phenol red, supplemented with 2% fetal bovine serum, 1% L-glutamine, 1% Pen/Strep, 1% sodium pyruvate) with 10% MTS (Abcam, ab197010) to measure cell viability. The reagent was left to incubate for 1 h before reading at OD490 nm. Controls included: cells in minimal media, cells in complete media, lysed cells as a positive control for lactate dehydrogenase (LDH) assay, and brain heart infusion (BHI) with cell media as the background control. A LDH assay (Promega, G1780) was performed with the CFS after initial incubation, according to the manufacturer’s instructions.
RESULTS
Patient characteristics and summary of sequencing data
A total of 28 patients provided clinical samples for the CALADRIO study, with 16 patients experiencing a CB (57%, 95%CI: 38.8%-75.4%). The median age of patients was 53.5 years (range: 47.5-63.0 years) and 48.1% of patients had PD-L1 positive tumors (combined positive score ≥ 1 using the 22C3 pharmDx assay), as shown in Table 2[4]. We collected 65 fecal and 70 buccal samples: BL 28/28, C4D1 22/22, and EoT 15/20. Four fecal samples were excluded due to insufficient sequencing depth and an additional three samples were removed due to missing clinical data or withdrawn consent. In total, 58 fecal samples and 67 saliva samples were used for final analysis.
Patient BL characteristics and samples collected
Characteristic | Overall (n = 28) |
Female (%): age, median (IQR), years | 100: 53.5 (47.5-63.0) |
ER status (%): positive | negative | 100 | 0 |
PgR status (%): positive | negative | 82.1 | 17.9 |
PD-L1 status (%)a: positive | negative | 48.1 | 51.9 |
Primary endpoint: CB | no-CB [n, (%)] | 16 (57%) | 12 (43%) |
Samples collected (n): fecal | saliva | 65 | 70 |
The combination therapy of P + E did not confer microbiota toxicity
Microbial alpha diversity was assessed at each sample collection time point using Shannon’s diversity index to evaluate whether treatment impacted overall microbial diversity. No significant drug-related changes were observed in either gut or oral microbiota alpha diversity, which was also confirmed at the genus level by Kruskal-Wallis test, P = 0.43 (gut) and 0.72 (oral).
When comparing overall alpha diversity, there were no substantial differences at the genus level between CB and no-CB groups for both the gut [Supplementary Figure 1A] and oral [Supplementary Figure 1B] microbiota profiles across all time points. However, we observed that Shannon diversity remained stable in the CB group, while diversity fluctuated in the no-CB group, decreasing in the gut and increasing in the oral profiles.
No significant differences were found in beta diversity after treatment with pembrolizumab and eribulin. Specifically, there was no clear segregation of either gut or oral microbiota profiles based on clinical metadata [PD-L1 status, neutrophil-to-lymphocyte ratio (NLR, with CB and without CB), CB status or PFS (> 6 months and ≤ 6 months)]. For the gut microbiota, this was confirmed using PERMANOVA analysis:
Shared taxa between the oral and gut microbiota
We assessed specific taxonomic profiles using alluvial and stacked bar plots of the top ten genera to visualize temporal changes in taxa, stratified by clinical parameters. Although moderate changes in Bacteroides were across time points, these were not statically significant and may have been influenced by the treatment or unaccounted factors such as diet. Changes were also noted in gut-associated Prevotella [Figure 1A], though further analysis indicated that this expansion was driven by three patients who had > 20% Prevotella in their profiles. In the oral microbiota profiles [Figure 1B], a reduction in Prevotella was observed in the no-CB group, although these samples were not paired with those from the same patient showing changes in the gut.
Figure 1. Relative abundances of (A) gut and (B) oral microbiota profiles showed little changes throughout the study treatment, thereby indicating that pembrolizumab and eribulin do not confer microbiota toxicity; (C) There were 57 common genera between the oral and gut microbiota profiles.
Overall, no substantive differences were detected in either the oral or gut microbiota based on clinical parameters, but 57 genera were common to both oral and fecal samples [Figure 1C]. This overlap may suggest potential oral-gut translocation, though further sequencing at greater depth is necessary to explore this possibility in more detail.
Potential functions of the gut microbiota in CB and no-CB patients are similar
The overall functional potential[25] of gut microbiota samples was assessed using shotgun metagenomic sequencing. Consistent with the taxonomic profiles, no significant differences were observed between CB and no-CB groups. However, LEfSe analysis identified two functional pathways that were significantly associated (P < 0.05) with the clinical parameter PFS. These pathways were: “DeNovo Purine2 Pathway” and “PRPP Pathway” [Figure 2A]. The DeNovo Purine 2 pathway is involved in purine nucleotides de novo biosynthesis II, while the PRPP Pathway is related to histidine, purine, and pyrimidine biosynthesis.
Figure 2. (A) Heatmap of metabolic pathways identified to have a LDA > 2.0 using LEfSe analysis for the clinical feature: time to PFS. Unstratified abundances of all pathways produced by Humann3 were run through LEfSe. Pathways identified as a discriminative feature as a LDA > 2.0 was then selected to create the heatmap. We categorized PFS into two groups: > 6 months and ≤ 6 months; (B) Antibiotic resistance genes identified in CALADRIO samples using Resfinder associated with CB. LDA: Linear discriminant analysis; LEfSe: linear discriminant analysis effect size; PFS: progression-free survival; CB: clinical benefit.
When analyzing antibiotic resistance genes within the gut microbiota, no distinct pattern was observed between CB and no-CB groups [Figure 2B]. Given previous findings that cytochrome (CYP) P450 isoform 3A4 can metabolize eribulin[43-45], we also examined microbial CYP genes in relation to CB status. Interestingly, patients who experienced CB exhibited a higher abundance of CYP genes in their gut microbiota [Supplementary Figure 2].
Oral Streptococcus and gut B. fragilis as potential biomarkers for CB
While diversity and overall taxonomic profiles did not reveal significant differences, more nuanced changes in specific microbiota members may occur during treatment. Heatmap visualization of the oral microbiota, clustered using a Bray Curtis dissimilarity matrix, suggested that patient samples with a relative abundance of > 30% Streptococcus were associated with CB [Figure 3A]. The trend was most evident at BL [Figure 3B], where patients with a CB appeared to have slightly higher relative abundances of Streptococcus, although this finding was not statistically significant [Figure 3C]. No significant differences were observed at subsequent time points (C4D1 and EoT), possibly due to the effects of antibiotic administration [Figure 3D]. A similar analysis of the gut microbiota data showed no correlation with CB status [Figure 3E].
Figure 3. Samples with a relative abundance of > 30% Streptococcus came from patients who experienced a CB. (A) Heatmap by Bray-Curtis dissimilarity matrix on saliva samples by the top ten genera; (B) further visualization showed this tended to be BL samples. This difference between oral Streptococcus in CB and no-CB group was lost at consecutive time points (C), most likely due to a combination of antibiotic usage and a lower number of EOT samples provided. Mann-Whitney U test was performed on the CB vs. no-CB group where no significance was observed. Antibiotics, categorized by class, taken by patients based on clinical metadata. Not all patients enrolled in the CALADRIO study had complete metadata about antibiotics, which is why the number of patients presented differs from the number of patients enrolled (D). No clustering of gut microbiota data was observed according to CB status, suggesting no overall taxonomic changes (E). Mann-Whitney U was performed to compare the relative abundances of Streptococcus by time point. CB: Clinical benefit; BL: baseline; EOT: end-of-treatment.
LEfSe was applied to identify genera and/or species that characterize the CB and no-CB groups. In the oral microbiota, LEfSe analysis revealed six genera with discriminative features: Capnocytophaga, Filifactor, Clostridium, Pyramidobacter, and Sphaerochaeta were associated with no-CB, while only Atopobium was associated with CB [Figure 4A]. Capnocytophaga, Filifactor, and Clostridium were also detected in gut samples, but only Capnocytophaga showed an association with no-CB.
Figure 4. LEfSe analysis of the salivary microbiota data showed five genera associated with no-CB and one for CB (A). B. fragilis in the gut is associated with CB. LEfSe analysis (B) showed that B. fragilis is associated with CB. Box plots (C) further showed that patients with CB consistently had a greater relative abundance of B. fragilis than no-CB. This observation was most significant at EoT [Mann Whitney U:
LEfSE analysis of gut microbiota identified 30 potentially significant taxa correlating with CB status [Figure 4B]. Notably, B. fragilis was enriched in the CB group, while Escherichia coli was associated with no-CB. Given previous research suggesting B. fragilis may act as a key mediator of antitumor effects in cancer immunotherapy and serve as a promising therapeutic target[14,46,47], further analysis was undertaken. Visualization showed an increasing trend in the relative abundance of B. fragilis in the CB patients compared to the no-CB group [Figure 4C], with a statistically significance difference observed at EoT (P = 0.0009).
Screening functional potential of B. fragilis
Given the therapeutic potential of B. fragilis, we performed a genomic analysis of this species using shotgun metagenomics data[14]. Five B. fragilis MAGs were extracted, most closely matching the type-strain B. fragilis NCTC 9343, with 4 out of 5 MAGs aligning at > 98%. Notably, all MAGs, except Z0983077.bin.48, originated from patients who experienced a CB (data not shown).
To investigate the functional potential of B. fragilis in CB patients, we screened the MAGs for antimicrobial resistance determinants. Genes conferring resistance to trimethoprim, tetracycline, D-cycloserine, cotrimoxazole, and aztreonam were identified [Figure 5]. Among these, trimethoprim was the only antibiotic administered to the patients, suggesting that antibiotic resistance alone does not explain the higher relative abundance of B. fragilis in CB patients.
Figure 5. Antibiotic resistance presence in B. fragilis MAGs based on ResFinderFG. MAGs: Metagenome assembled genome.
Further analysis using the METABOLIC pipeline indicated a specific cluster of sulfur cycling enzymes, i.e., methionine metabolizing enzymes[48], present in these MAGs. Additionally, we screened for immunomodulatory components, focusing on capsular polysaccharide and the B. fragilis toxin (BFT), as these components are known to influence immune responses[47]. Three out of the five B. fragilis MAGs contained a putative protein involved in capsular polysaccharide export (Genbank: BAD47972.1)[49], while none of the MAGs encoded the bft gene (fragilysin), as summarized in Table 1.
B. fragilis conditioned media induces intracellular LDH release in an HR[+]/HER2[-] BC cell line
Exploratory microbiota studies face the challenge of determining whether the bacterial presence influences clinical outcomes or vice versa. To investigate this, we conducted an in vitro assay using a BC cell line similar to those from the KELLY trial: (HR[+]/HER2[-]). Since microbial metabolites are more likely to reach distant sites, such as tumors, via the bloodstream under physiological conditions, we incubated
Results showed that MCF-7 exposed to B. fragilis supernatant (from 14 to 36 h growth phases) released significantly more intracellular LDH compared to cells treated with BHI media alone [Figure 6A]. However, this did not lead to direct cell death [Figure 6B]. Instead, a significant reduction in metabolic activity (P-value = 0.019) was observed in MCF-7 cells exposed to B. fragilis supernatant, indicating compromised cell viability. Additionally, a linear regression analysis confirmed a significant and positive (slope = 0.0186, P-value = 4.52 × 10-6%), dose-dependent relationship between B. fragilis growth and cytotoxicity [Figure 6C].
Figure 6. B. fragilis NCTC 9343 supernatant harvested during growth (0-36 h) could significantly (***P < 0.001) stimulate more LDH release, measured by OD490, from MCF-7 (HR[+]/HER2[-]) breast cancer cells in vitro compared to BHI control (A). However, this was not reflected as cell death since viability measured by OD490 (B) was not significantly different than BHI (*P < 0.05). Linear regression analysis showed that this was a dose dependent relationship (slope = 0.0186, P-value = 4.52 × 10-8%) (C). All conditions had n = 16, OD490 readings are corrected for background noise i.e., BHI media. Welch’s two sample t-test was used to compare the means of BHI and B. fragilis for both (A) and (B). LDH: Lactate dehydrogenase; BHI: brain-heart infusion.
DISCUSSION
The gut and oral microbiota play key roles in human health, and recent research suggests these microbial communities may influence anti-cancer responses[6-8,10-14,50-55]. While most studies on the microbiota-cancer relationship have focused on melanoma or non-small cell lung cancer[15,56,57], the CALADRIO study is one of the first to investigate the role of both gut and oral microbiota in BC patients undergoing novel combination therapy for mBC. Given the novelty of this therapeutic approach, we had a unique opportunity to comprehensively assess how this combination treatment influences both the oral and gut microbiota in mBC patients.
Patients who provided clinical samples for the CALADRIO study were enrolled in Spain, where previous work has identified a “typical” Spanish gut microbiota, including Bacteroides, Faecalibacterium, Prevotella, Alistipes, and Oscillospiraceae[58]. In agreement with these findings, we observed these core genera in the majority of our BC patient fecal samples [Figure 1A], with Prevotella also present in the oral microbiota samples at relatively high abundances. This suggests a potential oral-gut axis, as 57 genera appeared to be “common” between these two niches among patients. However, some taxa, such as Capnocytophaga, are more typically associated with the oral cavity than the gut [Supplementary Table 1]. Due to taxonomic resolution limitations with 16S rRNA gene amplicon sequencing, further exploration of the oral-gut axis was not possible. In terms of clinical parameters, several oral-associated microbes linked to the no-CB group were also detected in the gut samples after LEfSe analysis. These include Streptococcus_sp_KS_6, Neisseria meningitidis, and Capnocytophaga sputigena (though the latter result was likely influenced by two samples with high abundances, Supplementary Figure 3). This raises the possibility of translocation between body sites, which is relevant because oral microbes entering the gut have been implicated in diseases such as Crohn’s and colorectal cancer[17]. Notably, Fusobacterium, an oral-associated genus linked with colorectal cancer and BC in murine models, is not significant in our analysis[59-62].
Unlike studies on melanoma, where higher gut diversity has been associated with a stronger response in immunotherapy[11], we did not observe this in our cohort. This may be due to the nature of BC, which is not as immunogenic as melanoma, potentially resulting in a different microbiota host. Additionally, the patients in our cohort have undergone two rounds of prior chemotherapy, which could have altered their “BL” profiles.
Taxonomic shifts were observed primarily in the oral microbiota, particularly Streptococcus, which was more abundant in BL samples from CB patients, although the difference was not statistically significant. While Streptococcus is a common, and typically benign member of the oral microbiota[63], it has been linked to cancers associated with the oral respiratory tract, such as oesophageal[64], tongue[65], and gastric cancer[66]. Despite these associations, the underlying mechanisms remain unclear. Interestingly, we observed a potential association between oral Streptococcus and CB at BL, though this trend disappeared by C4D1 and EoT, possibly due to antibiotic use, as all patients have received at least two different antibiotic classes by C4D1. Streptococci, such as Streptococcus thermophilus[67] and Streptococcus salivarius, have been reported to have immune-modulatory effects[68]. However, the limitations of 16S rRNA gene amplicon sequencing hinder accurate species-level identification and the investigation of antimicrobial resistance profiles that could provide insights into the loss of this trend over time.
With respect to gut microbiota profiles, the observed association between B. fragilis and CB, although only significant at EoT, suggests that this species may serve as a potential biomarker for CB in the context of this novel therapy. However, a key challenge in exploratory microbiota studies is reliance on post-hoc analysis of sequencing data. Studies such as the one by Nearing et al. have highlighted the limitations of commonly used multi-variate analysis tools[69], which can lead to false positives. Therefore, in vitro validation is needed to further investigate the validity of findings and establish possible causation. In our study, we conducted in vitro experiments and showed that a product produced by B. fragilis (using a type strain almost identical to those strains found in CB patients - as identified through MAGs) induced LDH release, indicating cellular stress, in MCF-7 BC cells. However, this effect was not linked to cell death, as cells retained metabolic activity, suggesting that the underlying mechanism by which gut microbiota changes influence BC outcomes remains unclear. It is plausible that gut microbiota alterations affect serum metabolites in cancer patients. A previous study, which modeled serum metabolites based on gut microbiota changes, identified eight gut-associated serum metabolites. While the study relied on in silico modeling, it lays the groundwork for further in vitro validation[70]. Additionally, other research has demonstrated that microbiota-derived metabolites, such as tryptophan, can influence tumor-associated macrophages and modulate antitumor activity, as shown in pancreatic ductal adenocarcinoma[71]. Although these studies have limitations, they provide evidence that gut microbiota changes can affect distal sites via metabolite production.
Vétizou et al. demonstrated the favorable outgrowth of B. fragilis in CTLA-4 anti-cancer blockade in RET-mutated melanoma and MC38 colon cancer models. Their study suggested that this effect may be achieved through TH1-mediated immune responses[14], potentially linked to polysaccharide components of
Studies investigating the relationship between the microbiota and responses to anti-PD-1 therapy have provided evidence that certain members may influence antitumor responses. For instance, Gopalakrishnan et al. assessed the oral and gut microbiota of melanoma patients receiving anti-PD-1 immunotherapy, finding that Faecalibacterium was linked to prolonged PFS and was significantly more abundant in responders. Interestingly, they also observed an enrichment of Bacteroidales in non-responders[11]. Similarly, Routy et al. reported an association between Akkermansia muciniphila and clinical responses mediated by interleukin-12[13]. From a treatment perspective, Tanoue et al. demonstrated that an eleven-strain microbial consortium, consisting of seven Bacteroidales strains and four non-Bacteroidales strains[8], could prime CD 8 T cells for an antitumor response. However, no study to date has assessed the effect of eribulin on the gut microbiota, or any association with Bacteroides species and strains. While the results of these studies have been inconsistent, they do support the hypothesis that gut microbes can modulate the efficacy of anti-PD-1 therapy. The discrepancies between the studies may be due to variations in patient demographics and cancer types - this study focussed on BC, while others have examined non-small cell lung cancer and melanoma. Nevertheless, these findings underscore the importance of exploratory studies to investigate the potential of the microbiota as adjuvant options for enhancing immunotherapy outcomes.
While our investigation revealed promising insights into microbiota associations with clinical outcomes, it has several limitations. As this was an exploratory study, further well-powered multi-center studies comparing the gut and oral microbiota in a larger cohort of BC patients across treatment time points are necessary. Even when a microbiota association appears significant, the sample size can limit confidence in whether this is a genuine finding or a chance observation[77]. Thus, additional mechanistic work, in vitro and/or in vivo, could provide further evidence and potentially provide a platform for therapeutic development. Our in vitro analysis suggested that B. fragilis produces a metabolite or compound that stimulates LDH release from MCF-7 cells, although this effect did not reach cytotoxic levels. Since we only tested the total supernatant, further studies should aim to isolate and identify the specific compounds responsible for this activity. Moreover, the oral microbiota in our study was profiled using 16S rRNA gene amplicon sequencing, which has its limitations in resolution. Applying shotgun metagenomics profiling would allow for more detailed strain tracking and potential insights into oral-gut translocation. Finally, much of the current literature has focused on microbiota associations with immunotherapy efficacy, but there is limited information on how chemotherapy affects the microbiota, which may have influenced our findings[78]. Addressing these gaps in future research will help clarify the role of microbiota in cancer therapies.
We have demonstrated that the combination therapy of pembrolizumab and eribulin does not induce microbiota toxicity in Spanish pre-treated patients with HR[+]/HER2[-] mBC. The study is among the first to explore the impact of this novel combination on the oral and gut microbiota of mBC patients. Our findings suggest that BL oral Streptococcus may serve as a potential biomarker for CB, while gut-derived
DECLARATIONS
Acknowledgments
We would like to sincerely thank the patients of the CALADRIO study and the clinical staff who helped with the logistics of the study. In addition, we would like to thank Dr. Regis Stentz, who provided the
Authors’ contributions
Conceptualization: Hall LJ, Robinson SD, Malfettone A, Mancino M, Cortés J
Methodology: Hall LJ, Malfettone A, Mancino M
Software: Teng NMY, Kiu R, Dalby MJ, Seki D
Validation: Teng NMY, Kiu R, Dalby MJ, Seki D, Hall LJ
Formal analysis: Teng NMY, Dalby MJ, Kiu R, Seki D
Investigation, data curation: Teng NMY, Dalby MJ, Kiu R
Resources: Teng NMY, Kiu R, Dalby MJ
Writing - original draft preparation: Teng NMY, Kiu R, Hall LJ, Robinson SD
Writing - reviewing and editing: Teng NMY, Kiu R, Hall LJ, Robinson SD, Pérez-García JM, Mancino M, Shimizu E, Rodríguez-Morató J, Cortés J, Dalby MJ, Robinson T, Seki D, Bermejo B, Curigliano G
Visualization: Teng NMY, Dalby MJ
Supervision: Hall LJ, Robinson SD
Project administration: Teng NMY, Hall LJ, Robinson SD, Robinson T
Funding acquisitions: Hall LJ, Robinson SD, Malfettone A, Mancino M, Cortés J
Investigation - data curation and writing - reviewing and editing: Gion M, Prat A, Vázquez RM, Llombart-Cussac A, Schmid P, Barroso-Sousa R, Mina L
All authors gave final approval for the version to be published.
Availability of data and materials
The gut metagenome shotgun sequencing data can be found at PRJNA1050903 and the saliva 16S rRNA gene amplicon sequencing data can be found at PRJNA1052073. Code for the analysis undertaken in R can be found at: https://github.com/nteng22/CALADRIO-paper.
Financial support and sponsorship
The CALADRIO Project received funding from Fundació Privada Institut d’Investigació Oncològica de Vall d’Hebron. This work was funded by BigC grant 17-16R to Hall LJ and Robinson SD (studentship for Teng NMY). Hall LJ and Robinson SD are also supported by the Biotechnology and Biological Sciences Research Council (BBSRC), Institute Strategic Programme Gut Microbes and Health BB/R012490/1, and its constituent projects BBS/E/F/000PR10353 and BBS/E/F/000PR10356. Hall LJ is also supported by Wellcome Trust Investigator Awards 100974/C/13/Z and 220876/Z/20/Z. Robinson T is supported by a National Institute for Health Research Development and Skills Enhancement Award (NIHR 302363).
Conflicts of interest
Robinson T received funding for conference attendance from MSD and funding from Daiichi0-Sanko for educational workshops, while the other authors have declared that they have no conflicts of interest.
Ethical approval and consent to participate
All patients included in the study provided written informed consent (including biomarker analyses on biological samples) before any protocol-related activities started. The study protocol, patient information leaflet, and informed consent form were reviewed by each location’s Ethics Committee (registration number 8270). The study was conducted in accordance with the Declaration of Helsinki, the International Council for Harmonization of Technical Requirements for Pharmaceuticals for Human Use Good Clinical Practice guidelines, and all applicable regulations and laws. The EUDRACT number for the CALADRIO trial was: 2016-004513-27 and NCT03222856.
Consent for publication
Not applicable.
Copyright
© The Author(s) 2024.
Supplementary Materials
REFERENCES
1. NIH. Cancer stat facts: female breast cancer subtypes. Available from: https://seer.cancer.gov/statfacts/html/breast-subtypes.html. [Last accessed on 26 Oct 2024].
2. Rugo HS, Delord JP, Im SA, et al. Safety and antitumor activity of pembrolizumab in patients with estrogen receptor-positive/human epidermal growth factor receptor 2-negative advanced breast cancer. Clin Cancer Res 2018;24:2804-11.
3. Nanda R, Liu MC, Yau C, et al. Effect of pembrolizumab plus neoadjuvant chemotherapy on pathologic complete response in women with early-stage breast cancer: an analysis of the ongoing phase 2 adaptively randomized I-SPY2 trial. JAMA Oncol 2020;6:676-84.
4. Pérez-García JM, Llombart-Cussac A, G Cortés M, et al. Pembrolizumab plus eribulin in hormone-receptor-positive, HER2-negative, locally recurrent or metastatic breast cancer (KELLY): an open-label, multicentre, single-arm, phase II trial. Eur J Cancer 2021;148:382-94.
5. Cortes J, O’Shaughnessy J, Loesch D, et al; EMBRACE (Eisai Metastatic Breast Cancer Study Assessing Physician’s Choice Versus E7389) investigators. Eribulin monotherapy versus treatment of physician’s choice in patients with metastatic breast cancer (EMBRACE): a phase 3 open-label randomised study. Lancet 2011;377:914-23.
6. Lee SH, Cho SY, Yoon Y, et al. Bifidobacterium bifidum strains synergize with immune checkpoint inhibitors to reduce tumour burden in mice. Nat Microbiol 2021;6:277-88.
7. Sivan A, Corrales L, Hubert N, et al. Commensal Bifidobacterium promotes antitumor immunity and facilitates anti-PD-L1 efficacy. Science 2015;350:1084-9.
8. Tanoue T, Morita S, Plichta DR, et al. A defined commensal consortium elicits CD8 T cells and anti-cancer immunity. Nature 2019;565:600-5.
9. Viaud S, Saccheri F, Mignot G, et al. The intestinal microbiota modulates the anticancer immune effects of cyclophosphamide. Science 2013;342:971-6.
10. Iida N, Dzutsev A, Stewart CA, et al. Commensal bacteria control cancer response to therapy by modulating the tumor microenvironment. Science 2013;342:967-70.
11. Gopalakrishnan V, Spencer CN, Nezi L, et al. Gut microbiome modulates response to anti-PD-1 immunotherapy in melanoma patients. Science 2018;359:97-103.
12. Davar D, Dzutsev AK, McCulloch JA, et al. Fecal microbiota transplant overcomes resistance to anti-PD-1 therapy in melanoma patients. Science 2021;371:595-602.
13. Routy B, Le Chatelier E, Derosa L, et al. Gut microbiome influences efficacy of PD-1-based immunotherapy against epithelial tumors. Science 2018;359:91-7.
14. Vétizou M, Pitt JM, Daillère R, et al. Anticancer immunotherapy by CTLA-4 blockade relies on the gut microbiota. Science 2015;350:1079-84.
15. Routy B, Gopalakrishnan V, Daillère R, Zitvogel L, Wargo JA, Kroemer G. The gut microbiota influences anticancer immunosurveillance and general health. Nat Rev Clin Oncol 2018;15:382-96.
16. Burugu S, Asleh-Aburaya K, Nielsen TO. Immune infiltrates in the breast cancer microenvironment: detection, characterization and clinical implication. Breast Cancer 2017;24:3-15.
17. Kitamoto S, Nagao-Kitamoto H, Hein R, Schmidt TM, Kamada N. The bacterial connection between the oral cavity and the gut diseases. J Dent Res 2020;99:1021-9.
18. Dore J, Ehrlich SD, Levenez F, et al.
19. Caporaso JG, Lauber CL, Walters WA, et al. Ultra-high-throughput microbial community analysis on the Illumina HiSeq and MiSeq platforms. ISME J 2012;6:1621-4.
20. Kiu R, Brown J, Bedwell H, et al. Genomic analysis on broiler-associated Clostridium perfringens strains and exploratory caecal microbiome investigation reveals key factors linked to poultry necrotic enteritis. Anim Microbiome 2019;1:12.
21. Huson DH, Beier S, Flade I, et al. MEGAN community edition - interactive exploration and analysis of large-scale microbiome sequencing data. PLoS Comput Biol 2016;12:e1004957.
22. The comprehensive R archive network. https://cran.r-project.org/. [Last accessed on 26 Oct 2024]
23. Love MI, Huber W, Anders S. Moderated estimation of fold change and dispersion for RNA-seq data with DESeq2. Genome Biol 2014;15:550.
24. Chen S, Zhou Y, Chen Y, Gu J. fastp: an ultra-fast all-in-one FASTQ preprocessor. Bioinformatics 2018;34:i884-90.
25. Beghini F, McIver LJ, Blanco-Míguez A, et al. Integrating taxonomic, functional, and strain-level profiling of diverse microbial communities with bioBakery 3. Elife 2021;10:e65088.
26. Li D, Liu CM, Luo R, Sadakane K, Lam TW. MEGAHIT: an ultra-fast single-node solution for large and complex metagenomics assembly via succinct de Bruijn graph. Bioinformatics 2015;31:1674-6.
27. Uritskiy GV, DiRuggiero J, Taylor J. MetaWRAP-a flexible pipeline for genome-resolved metagenomic data analysis. Microbiome 2018;6:158.
28. Wood DE, Lu J, Langmead B. Improved metagenomic analysis with Kraken 2. Genome Biol 2019;20:257.
29. Lu J, Breitwieser FP, Thielen P, Salzberg SL. Bracken: estimating species abundance in metagenomics data. PeerJ Computer Science 2017;3:e104.
30. Parks DH, Imelfort M, Skennerton CT, Hugenholtz P, Tyson GW. CheckM: assessing the quality of microbial genomes recovered from isolates, single cells, and metagenomes. Genome Res 2015;25:1043-55.
31. Finn RD, Clements J, Eddy SR. HMMER web server: interactive sequence similarity searching. Nucleic Acids Res 2011;39:W29-37.
32. Hyatt D, LoCascio PF, Hauser LJ, Uberbacher EC. Gene and translation initiation site prediction in metagenomic sequences. Bioinformatics 2012;28:2223-30.
33. Wu YW, Simmons BA, Singer SW. MaxBin 2.0: an automated binning algorithm to recover genomes from multiple metagenomic datasets. Bioinformatics 2016;32:605-7.
34. Kang DD, Li F, Kirton E, et al. MetaBAT 2: an adaptive binning algorithm for robust and efficient genome reconstruction from metagenome assemblies. PeerJ 2019;7:e7359.
35. Chaumeil PA, Mussig AJ, Hugenholtz P, Parks DH. GTDB-Tk: a toolkit to classify genomes with the genome taxonomy database. Bioinformatics 2019;36:1925-7.
36. vegan: an R package for community ecologists. Available from: https://github.com/vegandevs/vegan/. [Last accessed on 26 Oct 2024].
37. Segata N, Izard J, Waldron L, et al. Metagenomic biomarker discovery and explanation. Genome Biol 2011;12:R60.
38. Zhou Z, Tran PQ, Breister AM, et al. METABOLIC: high-throughput profiling of microbial genomes for functional traits, metabolism, biogeochemistry, and community-scale functional networks. Microbiome 2022;10:33.
39. Florensa AF, Kaas RS, Clausen PTLC, Aytan-Aktug D, Aarestrup FM. ResFinder - an open online resource for identification of antimicrobial resistance genes in next-generation sequencing data and prediction of phenotypes from genotypes. Microb Genom 2022;8:000748.
40. Zankari E, Hasman H, Cosentino S, et al. Identification of acquired antimicrobial resistance genes. J Antimicrob Chemother 2012;67:2640-4.
41. Franco AA, Mundy LM, Trucksis M, Wu S, Kaper JB, Sears CL. Cloning and characterization of the Bacteroides fragilis metalloprotease toxin gene. Infect Immun 1997;65:1007-13.
42. Seemann T. ABRicate. 1.0.1 ed. Available from: https://github.com/tseemann/abricate. [Last accessed on 26 Oct 2024].
43. Dybdal-Hargreaves NF, Risinger AL, Mooberry SL. Eribulin mesylate: mechanism of action of a unique microtubule-targeting agent. Clin Cancer Res 2015;21:2445-52.
44. Swami U, Chaudhary I, Ghalib MH, Goel S. Eribulin - a review of preclinical and clinical studies. Crit Rev Oncol Hematol 2012;81:163-84.
45. Zhang ZY, King BM, Pelletier RD, Wong YN. Delineation of the interactions between the chemotherapeutic agent eribulin mesylate (E7389) and human CYP3A4. Cancer Chemother Pharmacol 2008;62:707-16.
46. Mazmanian SK, Liu CH, Tzianabos AO, Kasper DL. An immunomodulatory molecule of symbiotic bacteria directs maturation of the host immune system. Cell 2005;122:107-18.
47. Dasgupta S, Erturk-Hasdemir D, Ochoa-Reparaz J, Reinecker HC, Kasper DL. Plasmacytoid dendritic cells mediate anti-inflammatory responses to a gut commensal molecule via both innate and adaptive mechanisms. Cell Host Microbe 2014;15:413-23.
48. Barton LL, Ritz NL, Fauque GD, Lin HC. Sulfur cycling and the intestinal microbiome. Dig Dis Sci 2017;62:2241-57.
49. Krinos CM, Coyne MJ, Weinacht KG, Tzianabos AO, Kasper DL, Comstock LE. Extensive surface diversity of a commensal microorganism by multiple DNA inversions. Nature 2001;414:555-8.
50. Baruch EN, Youngster I, Ben-Betzalel G, et al. Fecal microbiota transplant promotes response in immunotherapy-refractory melanoma patients. Science 2021;371:602-9.
51. Dizman N, Meza L, Bergerot P, et al. Nivolumab plus ipilimumab with or without live bacterial supplementation in metastatic renal cell carcinoma: a randomized phase 1 trial. Nat Med 2022;28:704-12.
52. Iida N, Stewart C, Goldszmid R, Dzutsev A, Trinchieri G. Gut commensal bacteria promote anti-tumor innate immune responses in distant tumors after immunotherapy and chemotherapy (162.5). J Immunol 2012;188:162.5.
53. Li Y, Tinoco R, Elmén L, et al. Gut microbiota dependent anti-tumor immunity restricts melanoma growth in Rnf5-/- mice. Nat Commun 2019;10:1492.
54. Mager LF, Burkhard R, Pett N, et al. Microbiome-derived inosine modulates response to checkpoint inhibitor immunotherapy. Science 2020;369:1481-9.
55. McKee AM, Kirkup BM, Madgwick M, et al. Antibiotic-induced disturbances of the gut microbiota result in accelerated breast tumor growth. iScience 2021;24:103012.
56. Roy S, Trinchieri G. Microbiota: a key orchestrator of cancer therapy. Nat Rev Cancer 2017;17:271-85.
57. Teng NMY, Price CA, McKee AM, Hall LJ, Robinson SD. Exploring the impact of gut microbiota and diet on breast cancer risk and progression. Int J Cancer 2021;149:494-504.
58. Latorre-Pérez A, Hernández M, Iglesias JR, et al. The Spanish gut microbiome reveals links between microorganisms and Mediterranean diet. Sci Rep 2021;11:21602.
59. Han YW. Fusobacterium nucleatum: a commensal-turned pathogen. Curr Opin Microbiol 2015;23:141-7.
60. Castellarin M, Warren RL, Freeman JD, et al. Fusobacterium nucleatum infection is prevalent in human colorectal carcinoma. Genome Res 2012;22:299-306.
61. Kostic AD, Gevers D, Pedamallu CS, et al. Genomic analysis identifies association of Fusobacterium with colorectal carcinoma. Genome Res 2012;22:292-8.
62. Parhi L, Alon-Maimon T, Sol A, et al. Breast cancer colonization by Fusobacterium nucleatum accelerates tumor growth and metastatic progression. Nat Commun 2020;11:3259.
63. Sulyanto RM, Thompson ZA, Beall CJ, Leys EJ, Griffen AL. The predominant oral microbiota is acquired early in an organized pattern. Sci Rep 2019;9:10550.
64. Kawasaki M, Ikeda Y, Ikeda E, et al. Oral infectious bacteria in dental plaque and saliva as risk factors in patients with esophageal cancer. Cancer 2021;127:512-9.
65. Mukherjee PK, Wang H, Retuerto M, et al. Bacteriome and mycobiome associations in oral tongue cancer. Oncotarget 2017;8:97273-89.
66. Zhou CB, Pan SY, Jin P, et al. Fecal signatures of Streptococcus anginosus and Streptococcus constellatus for noninvasive screening and early warning of gastric cancer. Gastroenterology 2022;162:1933-47.e18.
67. Perdigon G, Nader de Macias ME, Alvarez S, Oliver G, Pesce de Ruiz Holgado AA. Enhancement of immune response in mice fed with Streptococcus thermophilus and Lactobacillus acidophilus. J Dairy Sci 1987;70:919-26.
68. Kaci G, Goudercourt D, Dennin V, et al. Anti-inflammatory properties of Streptococcus salivarius, a commensal bacterium of the oral cavity and digestive tract. Appl Environ Microbiol 2014;80:928-34.
69. Nearing JT, Douglas GM, Hayes MG, et al. Microbiome differential abundance methods produce different results across 38 datasets. Nat Commun 2022;13:342.
70. Chen F, Dai X, Zhou CC, et al. Integrated analysis of the faecal metagenome and serum metabolome reveals the role of gut microbiome-associated metabolites in the detection of colorectal cancer and adenoma. Gut 2022;71:1315-25.
71. Hezaveh K, Shinde RS, Klötgen A, et al. Tryptophan-derived microbial metabolites activate the aryl hydrocarbon receptor in tumor-associated macrophages to suppress anti-tumor immunity. Immunity 2022;55:324-40.e8.
72. Neff CP, Rhodes ME, Arnolds KL, et al. Diverse intestinal bacteria contain putative zwitterionic capsular polysaccharides with anti-inflammatory properties. Cell Host Microbe 2016;20:535-47.
73. Gul L, Modos D, Fonseca S, et al. Extracellular vesicles produced by the human commensal gut bacterium Bacteroides thetaiotaomicron affect host immune pathways in a cell-type specific manner that are altered in inflammatory bowel disease. J Extracell Vesicles 2022;11:e12189.
74. Zhang M, Zhou Q, Dorfman RG, et al. Butyrate inhibits interleukin-17 and generates tregs to ameliorate colorectal colitis in rats. BMC Gastroenterol 2016;16:84.
75. Kovács T, Mikó E, Vida A, et al. Cadaverine, a metabolite of the microbiome, reduces breast cancer aggressiveness through trace amino acid receptors. Sci Rep 2019;9:1300.
76. Mikó E, Vida A, Kovács T, et al. Lithocholic acid, a bacterial metabolite reduces breast cancer cell proliferation and aggressiveness. Biochim Biophys Acta Bioenerg 2018;1859:958-74.
77. Corander J, Hanage WP, Pensar J. Causal discovery for the microbiome. Lancet Microbe 2022;3:e881-7.
Cite This Article

How to Cite
Teng, N. M. Y.; Malfettone, A.; Dalby, M. J.; Kiu, R.; Seki, D.; Robinson, T.; Gion, M.; Bermejo, B.; Pérez-García J. M.; Prat, A.; Vázquez R. M.; Llombart-Cussac, A.; Curigliano, G.; Schmid, P.; Barroso-Sousa, R.; Mancino, M.; Shimizu, E.; Rodríguez-Morató J.; Mina, L.; Hall, L. J.; Robinson, S. D.; Cortés J. Profiling the gut and oral microbiota of hormone receptor-positive, HER2-negative metastatic breast cancer patients receiving pembrolizumab and eribulin. Microbiome. Res. Rep. 2025, 4, 4. http://dx.doi.org/10.20517/mrr.2024.49
Download Citation
Export Citation File:
Type of Import
Tips on Downloading Citation
Citation Manager File Format
Type of Import
Direct Import: When the Direct Import option is selected (the default state), a dialogue box will give you the option to Save or Open the downloaded citation data. Choosing Open will either launch your citation manager or give you a choice of applications with which to use the metadata. The Save option saves the file locally for later use.
Indirect Import: When the Indirect Import option is selected, the metadata is displayed and may be copied and pasted as needed.
About This Article
Copyright
Data & Comments
Data
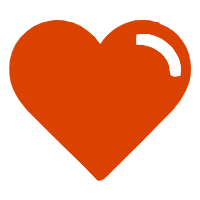
Comments
Comments must be written in English. Spam, offensive content, impersonation, and private information will not be permitted. If any comment is reported and identified as inappropriate content by OAE staff, the comment will be removed without notice. If you have any queries or need any help, please contact us at [email protected].