Non-targeted screening of urban PM2.5 components based on cellular effects: influence from molecular features and sources
Abstract
Chemical composition plays a crucial role in the adverse health effects of fine particulate matter (PM2.5), yet traditional methods fail to comprehensively identify the toxic components. In this study, we developed a non-targeted approach using gas chromatography and high-resolution mass spectrometry, and obtained a database of 2,138 organic components from PM2.5 samples collected in urban Beijing between 2016 and 2018. Meanwhile, we exposed PM2.5 extracts to a human lung epithelial cell line and measured intracellular reactive oxygen species (ROS) and extracellular cytokines as indicators of cellular response. A combination of single pollutant model, partial least squares regression, and Bayesian kernel machine regression was applied and filtered 165 robust components in the database. Beyond 110 classic polycyclic aromatic compounds (PACs), we also identified 14 aromatic anhydrides and 21 nitrated compounds as contributors to toxicity. Molecular features such as unsaturation and volatility were found to be the most significant factors influencing cellular effects. Source apportionment analysis demonstrated distinct seasonal patterns in toxicological impacts. During the heating season, biomass burning emerged as the predominant pollution source, with its two-fold intensity elevation correlating with a significant 2.0% increase in tumor necrosis factor (TNF)-α, accompanied by 6.0% and 7.6% decreases in vascular endothelial growth factor (VEGF) and interleukin (IL)-8, respectively. In contrast, non-heating season observations revealed vehicular emission as the principal contributor, inducing a pronounced 11.0% elevation in ROS levels. This study introduces an effect-oriented approach for screening toxic components in ambient PM2.5, offering valuable insights to support the formulation of public health protection strategies.
Keywords
INTRODUCTION
Fine particulate matter (PM2.5) is a major air pollutant that poses a significant risk to public health. According to the latest Global Burden of Disease Study, PM2.5 pollution was the third leading global risk factor in 2021, responsible for 8.08 million premature deaths worldwide, with 2.35 million occurring in China alone[1]. PM2.5 can penetrate the human respiratory system, leading to adverse effects on both the respiratory and cardiovascular systems through various mechanisms, including oxidative stress, inflammation, vascular dysfunction, thrombosis, arrhythmias, and atherosclerosis[2]. As the primary barrier to PM2.5 exposure, the lungs play a crucial role in modulating systemic responses through localized oxidative stress and inflammation[3].
Compared to mass concentration, numerous studies have suggested that the composition of PM2.5 plays a more critical role in explaining its health effects[4]. While inorganic components such as sulfates, nitrates, ammonium salts, black carbon, and crustal elements are well-documented, organic components are far more complex, exhibiting potent toxic effects and accounting for substantial proportions (30.5%-48.0%) of PM2.5[5]. Studies have revealed significant variability in the toxicity levels of organic components[6], primarily attributed to differences in their chemical properties and molecular structures[7]. However, a deeper determinant of their toxicity lies in their diverse emission sources and atmospheric transformation processes[8].
Humans are exposed to an extraordinarily complex and often poorly understood array of organic components[9]. Traditional targeted methods typically fail to effectively identify the intricate components of PM2.5. With the advancement in high-resolution mass spectrometry, non-targeted detection techniques have emerged, offering the potential to comprehensively detect and identify both known and unknown components in PM2.5[10]. By constructing an organic component database, a health-effect-oriented approach can be developed to screen and identify key toxic species[11]. However, these techniques typically generate vast amount of data characterized by high-dimensionality and multicollinearity, with potential interactions and nonlinear effects[12], posing great challenges for identifying key toxic components.
To address these limitations, our study introduces three methodological innovations:
(1) Effect-driven prioritization. We developed an effect-oriented approach to identify key components in PM2.5 linked to cellular oxidative stress and inflammation, utilizing high-sensitivity, high-resolution gas chromatography and time-of-flight mass spectrometry (GC-ToF-MS). Multiple statistical methods were strategically integrated to address the complexity of the data.
(2) Machine learning-powered resolution. Molecular characteristics of key effect components were examined using extreme gradient boosting tree model, to decode nonlinear relationships and interaction effects among more than 1,000 organic components.
(3) Source-to-health bridging. By establishing PM2.5 effect components as intermediates, we aimed to directly connect emission sources to health outcomes, providing scientific evidence to support the precise prevention and control of PM2.5 pollution.
EXPERIMENTAL
Chemical analyses of PM2.5
PM2.5 samples were collected at the Peking University Urban Atmosphere Environment Monitoring Station (PKUERS; 39.99° N, 116.31° E), a representative urban background site atop an academic building 30 meters off the ground. We used a high-flow sampler (flow rate 1.13 m3/min; HIVOL-CABLD, ThermoFisher Scientific, Waltham, MA, USA) on pre-baked quartz filters (203 × 254 mm2; Whatman, Hillsboro, OR, USA). Sampling was conducted for 23.5 h every six days from March 2016 to March 2018, resulting in a total of 122 samples[13].
For the chemical characterization of PM2.5, a 50 × 75 mm2 aliquot from each filter was Soxhlet-extracted with 200 mL n-hexane/dichloromethane mixture (1:1, v/v) for 22 h. The extract was concentrated and purified using a silica gel chromatography column (0.6 cm i.d., 6 cm height, topped with anhydrous sodium sulfate), with 8 mL dichloromethane as the elution solvent. Deuterated-labeled anthracene and 9-nitroanthracene were spiked as internal standards prior to instrumental analyses. Non-targeted detection of organic components in the PM2.5 extract was performed using an Agilent 7890B-7200 GC-ToF-MS equipped with electron ionization (EI) and electron capture negative ionization (ECNI) sources. Additionally, targeted pollutants, including polycyclic aromatic hydrocarbons (PAHs), alkyl-PAHs (APAHs), oxygenated PAHs (OPAHs), and nitro-PAHs (NPAHs), were quantified using an Agilent 8890-7010 GC and triple quadrupole MS (GC-QQQ-MS) [Supplementary Figure 1]. Further details of the instrumental analyses are provided in the Supplementary Materials.
Raw data from GC-ToF-MS were subjected to quality control, deconvolution with Unknowns Analysis software (version 10.0, Agilent), and peak alignment with Mass Profiler Professional (version B 14.9.2, Agilent). Molecular calculators were then employed to determine accurate masses and molecular formulas. Quantitative Analysis software (version 10.0, Agilent) was used to integrate the target substances, providing semi-quantitative information (relative intensity concentration). Species identification was performed using the NIST library for EI spectrums and characteristic fragmentation patterns of fragment ions. Further details are provided in the Supplementary Materials.
Cellular effect characterization
The Bronchial Epithelium transformed with Ad12-SV40 2B (BEAS-2B) human lung epithelial cell line was used as a model to investigate the effects of PM2.5 components[14]. Briefly, the PM2.5 extracts were dried by nitrogen blowing and then re-dissolved in the culture medium containing 0.5% DMSO. The BEAS-2B cells were cultured and exposed in RPMI-1640 medium with 10% fetal bovine serum at 37 °C in a 5% CO2 atmosphere. Cell viability and membrane permeability were evaluated to establish the exposure conditions for the PM2.5 extracts, which were set at 100 μg/mL for 12 h. After exposure, intracellular reactive oxygen species (ROS) were measured by flow cytometry (FACSVerse; BD Biosciences, CA, USA) with dichlorodihydrofluorescein diacetate (DCFH-DA) as a fluorescence probe. In parallel, extracellular levels of five cytokines - interleukin (IL)-1β, IL-6, IL-8, tumor necrosis factor (TNF)-α, and vascular endothelial growth factor (VEGF) - were quantified using a cytometric bead array (CBA) approach.
Statistical analyses
To identify components with robust effects from large datasets characterized by high-dimensionality, multicollinearity, potential interactions, and nonlinear effects, we integrated multiple models, including single pollutant model (SPM), partial least squares regression (PLS), and Bayesian kernel machine regression (BKMR). Preliminary screening was performed by a conventional SPM. Components identified as significant in the SPM were incorporated into both PLS and BKMR models. Species that were significantly correlated with more than three effect markers in both models were considered robust effect components [Supplementary Figure 2]. PLS resolves multicollinearity in linear associations, while BKMR characterizes nonlinear dose-response relationships and interaction effects; the synergistic implementation of these two approaches compensates for individual limitations while enabling systematic cross-validation. Furthermore, the mutual corroboration among the three models reduces false positives in identifying robust effect components and enhances the reliability of the findings.
Extreme gradient boosting trees (XGBoost) were used to explore the influence of molecular features on cellular effects[15,16]. For organic components with known molecular formulas, the retention indices (RI) were calculated based on chromatography results. The molecular formulas of 1,260 species were calculated for eight molecular features: hydrogen-to-carbon ratio (H/C), oxygen-to-carbon ratio (O/C), nitrogen-to-carbon ratio (N/C), double bond equivalent (DBE), aromaticity index (AI), carbon oxidation state (OSC), and octanol-water partition coefficient (logKow). The contribution of each organic component to cellular effects was ranked in both the PLS and BKMR models, with effect equivalents summed to derive the overall effect equivalent for each species. These molecular features and effect equivalents were then input into the extreme gradient boosting tree model. Details are given in the Supplementary Materials.
The positive matrix factorization (PMF) model was used to perform source apportionment for organic components in PM2.5. Considering both sample size and species number, 186 species that showed significant associations with more than two cellular effects were included in the analysis [Supplementary Text 3]. Both linear regression and stepwise regression models were applied to explore the correlation between sources and cellular effects. Seasonal differences were considered by distinguishing the heating season (November 15 to March 15 of the following year) from the non-heating season to assess the impact of seasonal variation.
RESULTS AND DISCUSSION
Screening of effect components
A database of 2,138 organic components was constructed from 122 PM2.5 samples collected over two years, including 1,163 compounds with molecular formulas identified primarily through an accurate mass-to-charge (m/z) ratio. Among these, 129 compounds were validated using standard reference materials, representing various categories such as PAHs, OPAHs, and nitrated aromatic compounds (NACs).
BEAS-2B cells exposed to PM2.5 extracts exhibited increased ROS and altered cytokine profiles. ROS, IL-1β, and TNF-α levels were positively correlated with the concentrations of organic components, while VEGF and IL-8 exhibited a dose-dependent negative response at high concentrations [Supplementary Figure 4]. Since no significant association was found between IL-6 and any component in the SPM [Supplementary Figure 3], it was excluded from further analysis. In multiple model analysis, using ROS as an example [Figure 1], 165 components with robust effects were identified, including 58 PAHs with 3-6 rings, 52 OPAHs with 3-5 rings, 14 aromatic anhydrides, and 21 NACs with 2-4 rings (details in Supplementary Table 1).
Figure 1. Effect-oriented screening of robust effect components. (A) Substances selected by the SPM were indicated as red points; (B) Further screening through the PLS and BKMR models to identify key effect components; (C) Substances exhibiting significant correlations with more than three cellular effects are identified as robust effect components, as indicated by the species count. SPM: Single pollutant model; PLS: partial least squares regression; BKMR: Bayesian kernel machine regression.
Among PAHs, phenanthrene, pyrene, chrysene, and indeno[1,2,3-cd]pyrene are priority pollutants by the US EPA. Newly identified components also included PAHs with five rings. Single oxygen-containing OPAHs included benzo[b]naphtho[2,3-d]furan, benzo[de]anthraquinone-7-ketone and its homologs, all featuring a five-ring structure. Additionally, 20 OPAHs containing two oxygen atoms were identified, including 5,12-naphtho[1,2-d]naphthoquinone and its homologs, as well as those with a basic benzo-naphthoquinone structure.
In addition to PAHs, which are known to trigger adverse health effects[17-19], OPAHs took the largest proportion (32%) of the robust effect components. Once inhaled, these compounds can be converted into their hydroxyl derivatives in biological systems[20], disrupting homeostasis by increasing ROS and inflammatory cytokines including TNF-α, VEGF, IL-1β, and IL-8[11,21-23]. Their ability to generate hydroxyl (OH) radicals, either directly or through activation, can cause severe damage to biological molecules, leading to oxidative stress, genetic instability, inflammation, proliferation, reduced antioxidant levels, apoptosis, and angiogenesis[24]. OPAHs may induce stronger respiratory toxicity and inflammatory responses than the precursor PAHs[24,25].
In addition to PAHs and OPAHs, new compounds were identified, including aromatic anhydrides, nitriles, and nitrated compounds. Fourteen robust effect components were determined as aromatic anhydrides, likely including phthalic, naphthalic, and anthraquinone anhydrides. Meanwhile, 21 NACs were identified, comprising nitrobiphenyl, nitronaphthalene, nitrofluorene, nitrophenanthrene, nitroanthracene, and hydroxy-nitroanthracene. Among NACs, nitropyrenol, an atmospheric oxidation product from nitropyrene and known for its endocrine-disrupting effects[26], showed significant correlations with all five cellular effects. These identified robust effect components offer valuable data for future toxicological and epidemiological studies.
Key molecular features
To explore molecular characteristics associated with cellular effects across a broader range of unknown components, the relationship between molecular features and effect equivalency was modeled. As illustrated in Figure 2, RI, DBE, AI, OSC, and logKow exhibited positive correlations with the effect equivalency of the five biomarkers, suggesting that species with low volatility, high unsaturation, high aromaticity, elevated oxidation states, and high lipophilicity are more likely to induce oxidative stress, inflammatory response, and endothelial dysfunction. In contrast, H/C, O/C, and N/C ratios showed negative correlations in relative importance, indicating that these features contribute less to toxicity. This implies that, compared to anhydrides and cyanides, aromatic compounds demonstrated higher potential toxicity. These results align with the identified robust effect components and are consistent with previous research[27].
Figure 2. Importance of organic components’ molecular features associated with the effect equivalency of (A) ROS, (B) IL-1β, (C) TNF-α, (D) VEGF, and (E) IL-8 in the XGBoost model. Red and green indicate positive and negative correlations, respectively. The error bars represent 95%CI. ROS: Reactive oxygen species; IL: interleukin; TNF: tumor necrosis factor; VEGF: vascular endothelial growth factor; XGBoost: extreme gradient boosting; 95%CI: the 95% confidence interval.
Unsaturation (DBE) and volatility (RI) emerged as the most critical molecular features influencing effect equivalency. Specifically, components with DBE values between 10-13 and volatility corresponding to
Cellular effects with sources
Emission sources play a fundamental role in determining the cellular effects of PM2.5 components. Using the PMF model, we resolved five contributing factors attributed to coal combustion, biomass burning, vehicle emission, atmospheric oxidation, and atmospheric nitration, with the assistance of typical source tracers
The relationship between sources and cellular effects was further quantified. By combining the results from linear and stepwise regression models, as shown in Figure 3, we found that, on an annual scale, coal combustion is a major source influencing IL-1β, TNF-α, VEGF, and IL-8. In contrast, atmospheric oxidation and nitration were identified as key sources driving oxidative stress (ROS) and the rapid release of inflammatory factors IL-1β and TNF-α. These results aligned with previous studies[11].
Figure 3. Quantified associations between sources and cellular effects across the entire year (top), non-heating season (middle), and heating season (bottom) once the intensity of each source doubles. The error bars represent 95%CI, and * indicates significant associations in the stepwise regression model. 95%CI: The 95% confidence interval.
The promotion of ROS, IL-1β, and TNF-α induced by pollution sources coexists with the inhibition of VEGF and IL-8, highlighting the multi-pathway impact of the complex chemical composition of PM2.5. For example, the release of ROS and TNF-α by pulmonary epithelial cells is driven by the activation of the PI3K/Akt pathway, which is triggered by NPAHs[31]. PAHs (such as benzopyrene) downregulate VEGF expression while upregulating IL-1β in a manner dependent on the aryl hydrocarbon receptor[32,33]. Additionally, IL-8 levels significantly increase in a human epidermis model upon PM2.5 exposure, potentially involving oxidative stress and signal transducer and activator of transcription (STAT) pathways[34]. These variations in pathway specificity suggest that the biological effects of individual pollution sources should be further elucidated by multi-omics integration analyses.
When seasonal variations were considered, vehicle emission and biomass burning were newly identified as sources significantly associated with cellular effects. During the non-heating season, when the source intensity of vehicle emission doubled, ROS levels increased by as much as 11.0% (95%CI: 4.8%-17.6%), with no such strong correlation observed elsewhere. This finding is consistent with previous studies[35,36] and underscores the importance of controlling traffic emissions. In contrast, during the heating season, a two-fold increase in the source intensity of biomass burning led to significant changes in TNF-α, VEGF, and
Studies have demonstrated that exposure to vehicle emissions triggers significant inflammatory responses in the respiratory system and other parts of the body. For instance, professional drivers exposed to traffic pollution exhibited elevated levels of TNF-α and C-reactive protein (CRP), which are correlated with PM2.5 exposure[37]. Similarly, PM2.5 from vehicular emissions induces dose-dependent increases in oxidative stress and inflammatory markers, such as TNF-α, in lung cells[38].
Exposure to biomass burning has significant health impacts, particularly on the respiratory and cardiovascular systems. Short-term exposure to biomass smoke extract induces airway inflammation, elevating inflammatory markers such as IL-8, TNF-α, and granulocyte-macrophage colony-stimulating factor (GM-CSF) in human bronchial epithelial cells and lung tissue[39]. Multiple mechanisms contribute to the toxicity of PM components from biomass burning, leading to harmful effects such as ROS generation, inflammation, genotoxicity, and tissue-specific damage[40].
CONCLUSIONS
A PM2.5 organic component database was constructed using high-sensitivity and high-resolution mass spectrometry. A total of 165 components with robust effects were identified out of 2,138 organic components, including 58 PAHs with 3-6 rings, 52 OPAHs with 3-5 rings, 14 aromatic anhydrides, and 21 NACs with 2-4 rings. Through an effect-directed approach, we identified a list of robust effect components critical for future research, and provided a paradigm for identifying toxic pollutants in complex mixtures, tackling the challenges from massive data.
Leveraging the detection data from this database, we determined key molecular features, such as unsaturation and volatility, as significant factors influencing effect equivalents. Specifically, components with DBE values ranging from 10 to 13 and volatility indices equivalent to C19–C25 n-alkanes exhibited higher effect equivalency. Such knowledge provides a reference for predicting the toxic potential of unknown substances.
Source analysis highlighted the importance of controlling traffic emissions during the non-heating season and coal combustion and biomass burning during the heating season to mitigate air pollution and associated health risks. In the non-heating season, a two-fold intensification of vehicular emission induced statistically significant elevation in ROS levels (11.0%, 95%CI: 4.8%-17.6%). Conversely, heating season biomass burning enhancements demonstrated a significant increase in TNF-α (2.0%, 95%CI: 0.1%-3.9%), accompanied by significant reductions in VEGF (-6.0%, 95%CI: -9.7% to -2.1%) and IL-8 (-7.6%, 95%CI:
However, the study has certain limitations. The effects of robust components on cellular responses and human health require further validation through toxicological experiments and epidemiological studies to elucidate the underlying mechanisms.
DECLARATIONS
Authors’ contributions
Designed the study: Qiu, X.; Zhu, T.
Performed the sample collection: Zhong, P.; Liu, J.
Conducted the laboratory analysis: Zhong, P.; Liu, J.; Jiang, X.
Conducted the data analysis: Zhong, P.; Liu, J.
Wrote the manuscript: Zhong, P.; Liu, J.; Qiu, X.
Availability of data and materials
Supplementary data are reported in Supplementary Materials or can be made available upon reasonable request to the corresponding author.
Financial support and sponsorship
This research was supported by the National Key Research and Development Program of China (No. 2022YFC3702704), the National Natural Science Foundation of China grant (NSFC; 42293324 and 41961134034), the 111 Center of Urban Air Pollution and Health Effects (B20009), and the International Joint Laboratory for Regional Pollution Control, Ministry of Education.
Conflicts of interest
Qiu, X. is an Editorial Board member of Journal of Environmental Exposure Assessment and Guest Editor of the Special Issue of “Global Perspectives on Air Pollution Exposure and Human Health Implications”. He is not involved in any steps of editorial processing, notably including reviewer selection, manuscript handling, and decision making. The other authors declared that there are no conflicts of interest.
Ethical approval and consent to participate
Not applicable.
Consent for publication
Not applicable.
Copyright
© The Author(s) 2025.
Supplementary Materials
REFERENCES
1. GBD 2021 Risk Factors Collaborators. Global burden and strength of evidence for 88 risk factors in 204 countries and 811 subnational locations, 1990-2021: a systematic analysis for the Global Burden of Disease Study 2021. Lancet 2024, 403, 2162-203.
2. Garcia, A.; Santa-Helena, E.; De Falco, A.; de Paula Ribeiro, J.; Gioda, A.; Gioda, C. R. Toxicological effects of fine particulate matter (PM2.5): health risks and associated systemic injuries-systematic review. Water. Air. Soil. Pollut. 2023, 234, 346.
3. Zhai, X.; Wang, J.; Sun, J.; Xin, L. PM2.5 induces inflammatory responses via oxidative stress-mediated mitophagy in human bronchial epithelial cells. Toxicol. Res. 2022, 11, 195-205.
4. Li, H.; Zhao, Z.; Luo, X. S.; et al. Insight into urban PM2.5 chemical composition and environmentally persistent free radicals attributed human lung epithelial cytotoxicity. Ecotoxicol. Environ. Saf. 2022, 234, 113356.
5. Chowdhury, S.; Pozzer, A.; Haines, A.; et al. Global health burden of ambient PM2.5 and the contribution of anthropogenic black carbon and organic aerosols. Environ. Int. 2022, 159, 107020.
6. Alves, C.; Evtyugina, M.; Vicente, E.; et al. PM2.5 chemical composition and health risks by inhalation near a chemical complex. J. Environ. Sci. 2023, 124, 860-74.
7. Qi, Z.; Zhang, Y.; Chen, Z. F.; et al. Chemical identity and cardiovascular toxicity of hydrophobic organic components in PM2.5. Ecotoxicol. Environ. Saf. 2020, 201, 110827.
8. Yan, R. H.; Peng, X.; Lin, W.; et al. Trends and challenges regarding the source-specific health risk of PM2.5-bound metals in a Chinese megacity from 2014 to 2020. Environ. Sci. Technol. 2022, 56, 6996-7005.
9. Johnston, M. V.; Kerecman, D. E. Molecular characterization of atmospheric organic aerosol by mass spectrometry. Annu. Rev. Anal. Chem. 2019, 12, 247-74.
10. Hites, R. A. Mass spectrometric identification of pollutants in the environment: a personal and bibliometric perspective. J. Am. Soc. Mass. Spectrom. 2022, 33, 620-6.
11. Jiang, X.; Xu, F.; Qiu, X.; et al. Hydrophobic organic components of ambient fine particulate matter (PM2.5) associated with inflammatory cellular response. Environ. Sci. Technol. 2019, 53, 10479-86.
12. Yu, L.; Liu, W.; Wang, X.; et al. A review of practical statistical methods used in epidemiological studies to estimate the health effects of multi-pollutant mixture. Environ. Pollut. 2022, 306, 119356.
13. Shi, X.; Qiu, X.; Jiang, X.; Rudich, Y.; Zhu, T. Comprehensive detection of nitrated aromatic compounds in fine particulate matter using gas chromatography and tandem mass spectrometry coupled with an electron capture negative ionization source. J. Hazard. Mater. 2021, 407, 124794.
14. Jia, Y. Y.; Wang, Q.; Liu, T. Toxicity research of PM2.5 compositions in vitro. Int. J. Environ. Res. Public. Health. 2017, 14, 232.
15. Zhang, Y.; Zhang, R.; Ma, Q.; et al. A feature selection and multi-model fusion-based approach of predicting air quality. ISA. Trans. 2020, 100, 210-20.
16. Sheridan, R. P.; Wang, W. M.; Liaw, A.; Ma, J.; Gifford, E. M. Extreme gradient boosting as a method for quantitative structure-activity relationships. J. Chem. Inf. Model. 2016, 56, 2353-60.
17. Låg, M.; Øvrevik, J.; Refsnes, M.; Holme, J. A. Potential role of polycyclic aromatic hydrocarbons in air pollution-induced non-malignant respiratory diseases. Respir. Res. 2020, 21, 299.
18. Øvrevik, J.; Refsnes, M.; Låg, M.; Holme, J. A.; Schwarze, P. E. Activation of proinflammatory responses in cells of the airway mucosa by particulate matter: oxidant- and non-oxidant-mediated triggering mechanisms. Biomolecules 2015, 5, 1399-440.
19. Rojas, G. A.; Saavedra, N.; Saavedra, K.; et al. Polycyclic aromatic hydrocarbons (PAHs) exposure triggers inflammation and endothelial dysfunction in BALB/c mice: a pilot study. Toxics 2022, 10, 497.
20. Niu, X.; Ho, S. S. H.; Ho, K. F.; et al. Atmospheric levels and cytotoxicity of polycyclic aromatic hydrocarbons and oxygenated-PAHs in PM2.5 in the Beijing-Tianjin-Hebei region. Environ. Pollut. 2017, 231, 1075-84.
21. Honda, A.; Inoue, K. I.; Higashihara, M.; Ichinose, T.; Ueda, K.; Takano, H. Differential pattern of cell death and ROS production in human airway epithelial cells exposed to quinones combined with heated-PM2.5 and/or Asian sand dust. Int. J. Mol. Sci. 2023, 24, 10544.
22. Jiang, X.; Han, Y.; Qiu, X.; et al. Personal exposure to electrophilic compounds of fine particulate matter and the inflammatory response: the role of atmospheric transformation. J. Hazard. Mater. 2022, 432, 128559.
23. Gao, R.; Jiang, Z.; Wu, X.; Cai, Z.; Sang, N. Metabolic regulation of tumor cells exposed to different oxygenated polycyclic aromatic hydrocarbons. Sci. Total. Environ. 2024, 907, 167833.
24. Idowu, O.; Semple, K. T.; Ramadass, K.; O’Connor, W.; Hansbro, P.; Thavamani, P. Beyond the obvious: environmental health implications of polar polycyclic aromatic hydrocarbons. Environ. Int. 2019, 123, 543-57.
25. Honda, A.; Inoue, K.; Takai, S.; Kameda, T.; Ueda, K.; Takano, H. Effects of oxidized pyrenes on the biological responses in the human bronchial epithelial cells. Appl. Sci. 2022, 12, 9664.
26. Kameda, T.; Akiyama, A.; Toriba, A.; Tang, N.; Hayakawa, K. Atmospheric formation of hydroxynitropyrenes from a photochemical reaction of particle-associated 1-nitropyrene. Environ. Sci. Technol. 2011, 45, 3325-32.
27. Tuet, W. Y.; Chen, Y.; Fok, S.; et al. Chemical and cellular oxidant production induced by naphthalene secondary organic aerosol (SOA): effect of redox-active metals and photochemical aging. Sci. Rep. 2017, 7, 15157.
28. Yang, B.; Wang, Y.; Fang, C.; Song, E.; Song, Y. Polybrominated diphenyl ether quinone exposure leads to ROS-driven lysosomal damage, mitochondrial dysfunction and NLRP3 inflammasome activation. Environ. Pollut. 2022, 311, 119846.
29. Liu, J.; Fu, M.; Miao, J.; et al. The toxicity of cooking oil fumes on human bronchial epithelial cells through ROS-mediated MAPK, NF-κB signaling pathways and NLRP3 inflammasome. Environ. Toxicol. 2022, 37, 1071-80.
30. Kim, M. J.; Pelloux, V.; Guyot, E.; et al. Inflammatory pathway genes belong to major targets of persistent organic pollutants in adipose cells. Environ. Health. Perspect. 2012, 120, 508-14.
31. Shang, Y.; Zhou, Q.; Wang, T.; et al. Airborne nitro-PAHs induce Nrf2/ARE defense system against oxidative stress and promote inflammatory process by activating PI3K/Akt pathway in A549 cells. Toxicol. In. Vitro. 2017, 44, 66-73.
32. Ichihara, S.; Yamada, Y.; Gonzalez, F. J.; Nakajima, T.; Murohara, T.; Ichihara, G. Inhibition of ischemia-induced angiogenesis by benzo[a]pyrene in a manner dependent on the aryl hydrocarbon receptor. Biochem. Biophys. Res. Commun. 2009, 381, 44-9.
33. Kohno, R.; Nagata, Y.; Ishihara, T.; et al. Benzo[a]pyrene induces NLRP1 expression and promotes prolonged inflammasome signaling. Front. Immunol. 2023, 14, 1154857.
34. Kono, M.; Okuda, T.; Ishihara, N.; et al. Chemokine expression in human 3-dimensional cultured epidermis exposed to PM2.5 collected by cyclonic separation. Toxicol. Res. 2023, 39, 1-13.
35. Lei, Y.; Wang, Z.; Xu, H.; et al. Characteristics and health risks of parent, alkylated, and oxygenated PAHs and their contributions to reactive oxygen species from PM2.5 vehicular emissions in the longest tunnel in downtown Xi’an, China. Environ. Res. 2022, 212, 113357.
36. Liu, B.; Shen, L. J.; Zhao, T. X.; et al. Automobile exhaust-derived PM2.5 induces blood-testis barrier damage through ROS-MAPK-Nrf2 pathway in sertoli cells of rats. Ecotoxicol. Environ. Saf. 2020, 189, 110053.
37. Riaz, H.; Syed, B. M.; Laghari, Z.; Pirzada, S. Analysis of inflammatory markers in apparently healthy automobile vehicle drivers in response to exposure to traffic pollution fumes. Pak. J. Med. Sci. 2020, 36, 657-62.
38. Niu, X.; Yu, J.; Zhang, N.; et al. Human pulmonary cytotoxicity of vehicular derived PM2.5: a study from three characterized tunnels. Atmos. Environ. 2024, 326, 120481.
39. Kc, B.; Mahapatra, P. S.; Henry, A.; et al. Inflammatory effects of short-term exposure to smoke emissions from biomass fuels. In ISES-ISEE 2018 Joint Annual Meeting: Addressing Complex Local and Global Issues in Environmental Exposure and Health, 2018.
Cite This Article

How to Cite
Download Citation
Export Citation File:
Type of Import
Tips on Downloading Citation
Citation Manager File Format
Type of Import
Direct Import: When the Direct Import option is selected (the default state), a dialogue box will give you the option to Save or Open the downloaded citation data. Choosing Open will either launch your citation manager or give you a choice of applications with which to use the metadata. The Save option saves the file locally for later use.
Indirect Import: When the Indirect Import option is selected, the metadata is displayed and may be copied and pasted as needed.
About This Article
Special Issue
Copyright
Data & Comments
Data
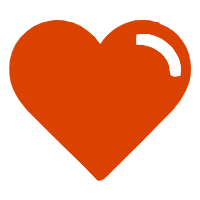
Comments
Comments must be written in English. Spam, offensive content, impersonation, and private information will not be permitted. If any comment is reported and identified as inappropriate content by OAE staff, the comment will be removed without notice. If you have any queries or need any help, please contact us at [email protected].