Figure1
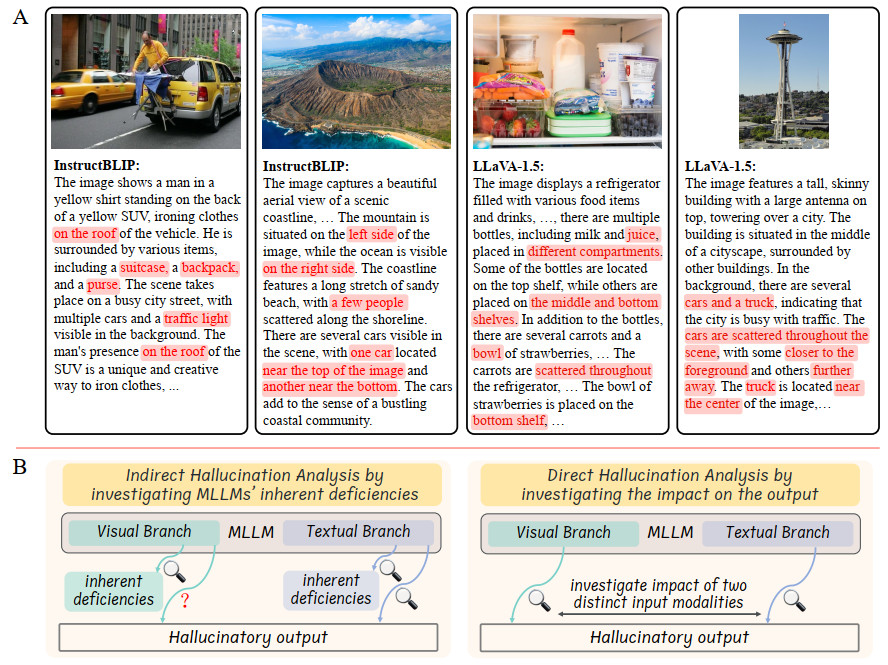
Figure 1. (A) An illustration of visual hallucinations in MLLMs' responses. Leading MLLMs, such as InstructBLIP and LLaVA-1.5, often produce image descriptions that contain inaccuracies, commonly referred to as visual hallucinations. These visual hallucinations can include incorrect object categories (nouns), incorrect spatial relationships (prepositions and adverbs), and inappropriate visual attributes (adjectives). Such errors can diminish the reliability of MLLMs in real-world applications. The present samples are from the LLaVA-bench-in-the-wild dataset, with inaccurate content highlighted in red. (B) Previous research has identified inherent deficiencies in MLLMs, but has not explored how these deficiencies contribute to the hallucinatory responses. While recent studies have explored the impact of the textual branch of MLLMs on hallucinations, the influence of the visual branch has been overlooked. This study addresses this gap by conducting a direct analysis of hallucinations, examining the respective contributions of both the visual and textual branches to hallucinatory responses. MLLMs: Multi-modal large language models.