Figure2
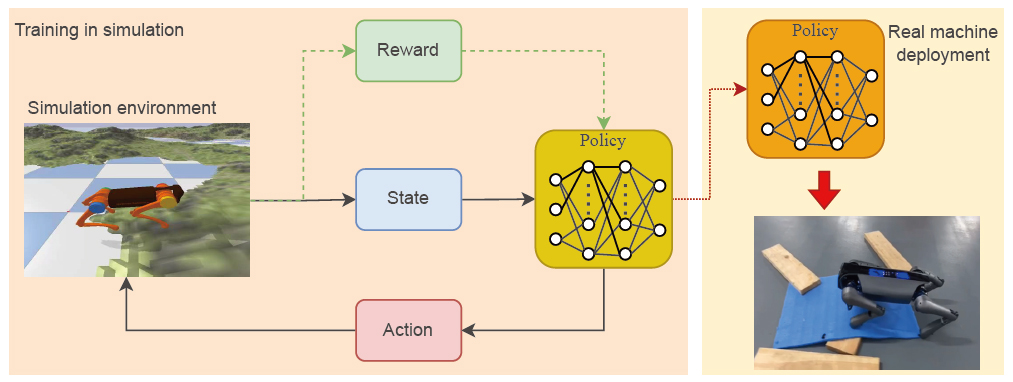
Figure 2. A common paradigm for DRL-based quadrupedal locomotion research. This paradigm is mainly divided into training and testing phases. The policy interacts with the simulated environment and collects data for iterative updates, and then the trained policy is deployed to the real robot.