Current status and challenges of 3D navigation in partial nephrectomy
Abstract
Partial nephrectomy, a standard treatment for small renal cancers, has evolved through minimally invasive procedures such as laparoscopic and robot-assisted partial nephrectomy. The use of three-dimensional (3D) kidney models derived from preoperative computed tomography (CT) images has been investigated to improve surgical outcomes. This review explores various navigation techniques, such as 3D printing, virtual reality (VR), and augmented reality (AR), to address organ movement and deformation challenges during surgery. Despite the promising positive impact of these methods, as revealed by a systematic review in 2022, achieving the desired navigation accuracy remains elusive. The use of Virtual Reality and Augmented Reality, capable of overlaying the 3D model onto the surgical image in real-time, has shown potential. Still, we need advanced techniques, for instance, non-rigid 3D models employing nonlinear parametric deformation, to adapt to organ deformation. Additionally, the application of deep learning from artificial intelligence for high accuracy 3D navigation is an emerging area of interest. Although considerable progress has been achieved, a comprehensive, widely adoptable solution has yet to be discovered. The paper underscores the necessity for ongoing research and development in 3D navigation methods, anticipating their substantial contribution to future surgical procedures.
Keywords
INTRODUCTION
In the field of small renal cell carcinoma, partial nephrectomy (PN) has been established as a standard treatment strategy[1,2] and is recommended by the EAU Guidelines[3]. To achieve the goal of preserving renal function in PN, techniques such as the zero-ischemia approach are being researched[4]. As minimally invasive surgical techniques advance, both laparoscopic (LPN) and robot-assisted PN (RAPN) have become increasingly common for treating cT1 and some cT2 renal tumors, with good outcomes reported[5-8].
In cases where a tumor is primarily located within the kidney (endophytic tumor), it can be difficult to visually locate the tumor and determine the extent of resection required. This increases the complexity of the surgical technique and the risk of complications[9]. Surgeons typically use preoperative computed tomography (CT) scans to understand the anatomical characteristics of the tumor and vascular system, but in cases where it is challenging to visually identify the tumor, intraoperative ultrasound is an effective and common method for confirming its location[10].
With the advancements in technology, it has become easier to reconstruct three-dimensional (3D) images from the two-dimensional (2D) images taken from preoperative CT scans[11]. Using these reconstructed 3D kidney models can improve the accuracy of locating difficult-to-see tumors and blood vessels, leading to research into navigation systems for LPN and RAPN that utilize these models. This 3D navigation technology has the potential to improve treatment outcomes of PN and expand its indications even in challenging cases such as endophytic renal masses[12]. This review will discuss the use of 3D kidney models in navigation for LPN and RAPN.
CURRENT STATE OF 3D NAVIGATION IN MINIMALLY INVASIVE PN
Three main navigation methods using 3D models include 3D printed models, augmented reality (AR), and virtual reality (VR)[13]. A systematic review conducted in 2022 has reported significant improvement in surgical outcomes when using these techniques for navigation during RAPN surgeries[14]. However, while their utility is acknowledged, some opinions suggest that there is insufficient evidence to demonstrate improvement in surgical outcomes[15], and there may still be room for debate regarding sufficient scientific evidence for their effectiveness. When conducting navigation using 3D models, information about the renal tumor and blood vessels is first extracted from contrast-enhanced CT scans, and a 3D model is then created. Information captured in contrast-enhanced CT scans is processed through segmentation and then reconstructed into 3D. It is further optimized for medical purposes, and then navigation is performed using 3D printers, AR, or VR[11] [Figure 1].
Figure 1. (A) Generation of a 3D kidney model through 3D reconstruction from CT scans; (B) 3D printed model, which is fabricated from the kidney’s 3D model using a 3D printer, aids in understanding anatomical features such as the kidney, tumor, and blood vessels; (C) 3D navigation via VR. The 3D model of the kidney can be viewed from various angles using devices such as a head-mounted display; (D) 3D navigation via AR. By overlaying the 3D kidney model onto the actual surgical view, detailed anatomical features can be grasped during surgery. No direct patient identifiers are included in this image. 3D: Three-dimensional; CT: computed tomography; VR: virtual reality; AR: augmented reality.
3D printed model
For patient education, 3D printed models tend to be preferred[13], and there have been reports of improved patient understanding of their anatomical structure and surgical approach[16]. In navigation for PN, these models have been used for preoperative training and surgical simulations, with reports indicating significantly less blood loss compared to when navigation was not performed[17]. There have also been reports of a significant decrease in warm ischemia time when 3D printed models were employed for preoperative planning and intraoperative navigation[18]. In addition, it has been reported that they are not only useful for patient education but also for resident education, and they are beneficial in achieving the trifecta during the initial implementation of RAPN[19,20].
VR
VR, a technology that provides an independent virtual world, is used for preoperative simulation and training in surgical navigation[21]. When used during surgery, 3D models are displayed side-by-side on surgical or head-mounted displays to provide surgeons with navigation[22-24]. In VR navigation, there is no overlay (registration) of 3D models onto the surgical view.
AR
AR is a method that allows surgeons to visualize organ details by overlaying a 3D model created from CT images onto the surgical view. Due to this feature, it is often preferred over VR for intraoperative navigation[13]. The use of 3D models derived from CT for AR in intraoperative navigation for PN was first reported by Ukimura et al. in 2008[25]. Since then, numerous reports have followed about its application in actual surgery[26-32]. Furthermore, studies have also reported that 3D navigation using AR can display the blood flow of the kidney in segments and enhance the outcomes of selective clamping strategies[33]. Although not as widely adopted as echo guidance, 3D navigation has become more accessible thanks to software programs (such as SYNAPSE VINCENT and others) that can create 3D models from CT scans.
CHALLENGES
As discussed in the section on the current state of 3D navigation in minimally invasive PN, the proof of 3D navigation effectiveness in PN remains a subject of debate. In fact, 3D navigation in PN has not become as widespread as the navigation systems used in other specialties such as otolaryngology, dentistry, and orthopedics[34,35]. This discrepancy is largely due to the unique challenges presented by the surgical area. For instance, the organs dealt with in otolaryngology undergo very little movement or deformation, making the application of preoperative CT information to the surgical site straightforward, with navigation accuracy reaching under 1 mm[36]. In contrast, organs involved in urology or gastrointestinal surgery are subject to intraoperative manipulations and insufflation, resulting in movement and deformation that make navigation challenging[37]. Even in the case of the rectum, an organ relatively immobile within the pelvic cavity, errors of around 19mm have been reported when attempting 3D navigation[38]. This confirms the difficulty of high-precision navigation.
Various methods have been researched to adjust navigation to organ movement and deformation. However, most reports involving 3D navigation during renal PN use rigid models, which do not accommodate deformation. Reports that employ deformable, non-rigid models are rare[14]. Even fifteen years after Ukimura et al. reported in 2008 about navigation for renal PN using a 3D model[25], manual navigation using a rigid model remains the norm. To achieve more accurate 3D navigation, it is crucial that methods able to cope with organ deformation and movement become widely available.
Despite most methods reported for actual renal PN using rigid models, a multitude of research has been done to accommodate organ deformation and movement. From here, we will focus on AR, where the most significant technological advancements are expected, and introduce its research contents [Figure 2].
Figure 2. Overview of the technique for overlaying the 3D model and the surgical view in AR. No direct patient identifiers are included in this image. AI: Artificial intelligence; CNN: convolutional neural network; ICP: iterative closest point; CPD: coherent point cloud; SfM: structure from motion; SGBM: semi-global block matching; Mask R-CNN: Mask Region-based CNN; FEM: finite element method; 3D: Three-dimensional; AR: augmented reality.
Manual registration
The simplest method is manual registration, adopted from early stages due to its low technological barrier[25]. There have been reports proving its effectiveness in actual surgery[14,39,40]. Most of these studies lack a comparative counterpart, but Porpiglia et al. conducted a retrospective study comparing an AR group of 48 cases with a control group of 43 cases guided by ultrasound[27]. They demonstrated a significant reduction in the total arterial clamping rate, a reduction in the urinary tract opening rate, and a similar complication rate.
Fiducial-based registration
Fiducial markers serve as landmarks for registration, commonly used in 3D navigation in fields such as otolaryngology. For navigation during renal PN, these markers placed in the kidney can accommodate organ movement and deformation. Unlike in otolaryngology, kidneys move, so markers need to be placed in the organ itself rather than fixed landmarks such as the body surface. Animal experiments with pigs have reported kidney bleeding due to markers embedded directly into the kidneys to prevent movement[41]. Conversely, studies reported safe execution of AR navigation using fiducial markers in LPN[28,31]. Adhesive markers have also been proposed to allow non-invasive fiducial-based registration without inserting markers into the target organ[42,43].
Additional methods utilized in actual surgeries
Porpiglia et al. implemented surgical navigation with nonlinear parametric deformation and reported its effectiveness compared to a group guided only by 2D ultrasound[27]. Nonlinear parametric deformation can accommodate complex operations such as bending, stretching, and twisting, allowing for more realistic and natural movements and shape changes. While registration was performed manually, using this technique in 3D navigation for RAPN significantly improved surgical outcomes compared to the group guided only by 2D ultrasound. Kobayashi et al. reported a method using an infrared reflective marker placed on the camera and tracked with an optical sensor in 2020[44]. Initially, the surgical scene was manually registered with the 3D model, and then the camera movement was tracked to maintain navigation accuracy. This method reportedly yielded effective treatment results in actual RAPN procedures. Amparore et al. have reported on effectively using selective clamping of blood vessels by evaluating kidney blood flow using indocyanine green (ICG) and combining this with AR[30]. Blood flow areas of the 3D kidney model, created from CT, are color-coded using a Voronoi diagram, and compared with blood flow areas confirmed intraoperatively with ICG injection. This method does not directly enhance registration accuracy in response to organ deformation or movement but successfully improves navigation accuracy by enhancing the precision of blood flow evaluation.
Other registration innovations
Despite the potential of fiducial-based navigation, a key drawback is the time-consuming correction process in case of significant misalignment. Without fiducial markers, a registration method utilizing affine transformations was proposed in 2013 to accommodate organ movement[45]. Affine transformations manipulate geometric objects, enabling translation, rotation, scaling, and shearing. Yet, its major limitation is the inability to realize bending deformation. B-spline curves, capable of complex nonlinear deformation such as bending and twisting, have been used in some studies[46], but accuracy drops when deformation is significant. Recent research has explored deep learning-based registration methods. In 2021, Jia et al. reported that using SiamMask, a deep learning method for object tracking and segmentation, they substantially improved SLAM accuracy and accommodated organ movement and deformation[47]. In 2022, Padovan et al. presented a study using two convolutional neural networks (CNNs) to identify organ positions from RGB images captured by a camera and register these with a CT model[48].
All the aforementioned 3D navigation techniques involve overlaying 3D models derived from CT onto 2D images. If the surgical scene could be reconstructed in 3D and then superimposed with the 3D CT-derived model, it would possibly lead to more precise navigation. However, the technical hurdles are high, and there are currently no reports of its effective use in actual surgeries. This section will overview research on PN navigation using 3D-3D registration.
To perform 3D-3D registration, not only is it necessary to reconstruct the kidney model in 3D from CT images, but also to reconstruct the surgical scene in 3D. Furthermore, 3D point cloud registration methods differ from those of 2D-3D registration. The first method of 3D-3D navigation in PN was reported by Su et al.[49]. They reconstructed the surgical scene in 3D from the disparity of a stereo camera and manually registered it with the 3D model of the kidney derived from CT using the Iterative Closest Point (ICP) algorithm. However, while ICP is a common 3D registration method, it is incomplete due to its requirement for the point clouds to be somewhat close together and its difficulty registering deformation models. Zampokas et al. used a strategy known as Quasi-dense matching, combining sparse and dense matching, to create a 3D point cloud in 2018[50]. A subsequent study in 2022 used the DynamicFusion method for the registration of this 3D point cloud with the 3D kidney model[51]. There has also been a report of extracting feature points from the surgical field, capturing them as a 3D point cloud through Structure from Motion (SfM), and tracking them, but no mention was made of registration with the 3D kidney model[52]. Zhang
Other research exists that, while not strictly creating a 3D point cloud, captures the position and posture of the kidney in real time using particle filtering and then registers it with the 3D model derived from CT[54]. This study compared it with registration via Coherent Point Drift (CPD), showing that particle filtering was faster in terms of calculation speed in real-time intraoperative navigation.
Various studies have also been conducted on methods for creating deformable 3D models for high-precision registration with deforming organs. One approach combined shape matching with cluster-based deformation to create a non-rigid model[55]. The effectiveness of this method was confirmed by creating a model from a pig kidney captured by CT and conducting experiments. Another study used a finite element method (FEM) model as a non-rigid model, reproducing organ deformation and movement in conjunction with the positional change of fiducial markers attached to the kidney[41].
CONCLUSION
Numerous studies have aimed to achieve high-precision 3D navigation, and some attempts in actual surgeries have yielded certain effects. However, none have led to a complete solution that could be widely generalized. Further advancements in 3D navigation research capable of accommodating deforming organs are expected to contribute to surgical procedures in the future.
DECLARATIONS
Authors’ contributions
Made substantial contributions to conception and design of the study and performed data analysis and interpretation: Magaribuchi T
Managed acquired data and drafted the manuscript: Masui K, Goto T, Saito R, Kobayashi T
Availability of data and materials
Not applicable.
Financial support and sponsorship
None.
Conflicts of interest
All authors declared that there are no conflicts of interest.
Ethical approval and consent to participate
Not applicable.
Consent for publication
Not applicable.
Copyright
© The Author(s) 2024.
REFERENCES
1. Marchioni M, Preisser F, Bandini M, et al. Comparison of partial versus radical nephrectomy effect on other-cause mortality, cancer-specific mortality, and 30-day mortality in patients older than 75 years. Eur Urol Focus 2019;5:467-73.
2. Zini L, Perrotte P, Capitanio U, et al. Radical versus partial nephrectomy: effect on overall and noncancer mortality. Cancer 2009;115:1465-71.
3. Ljungberg B, Albiges L, Abu-Ghanem Y, et al. European association of urology guidelines on renal cell carcinoma: the 2022 update. Eur Urol 2022;82:399-410.
4. Fong KY, Gan VHL, Lim BJH, et al. Off-clamp vs on-clamp robot-assisted partial nephrectomy: a systematic review and meta-analysis. BJU Int 2024;133:375-86.
5. Bertolo R, Autorino R, Simone G, et al. Outcomes of robot-assisted partial nephrectomy for clinical T2 renal tumors: a multicenter analysis (ROSULA Collaborative Group). Eur Urol 2018;74:226-32.
6. Delto JC, Paulucci D, Helbig MW, et al. Robot-assisted partial nephrectomy for large renal masses: a multi-institutional series. BJU Int 2018;121:908-15.
7. Vartolomei MD, Remzi M, Fajkovic H, Shariat SF. Robot-assisted partial nephrectomy mid-term oncologic outcomes: a systematic review. J Clin Med 2022;11:6165.
8. Springer C, Hoda MR, Fajkovic H, et al. Laparoscopic vs open partial nephrectomy for T1 renal tumours: evaluation of long-term oncological and functional outcomes in 340 patients. BJU Int 2013;111:281-8.
9. Mullerad M, Kastin A, Adusumilli PS, Moskovitz B, Sabo E, Nativ O. Comparison of nephron-sparing surgery in central versus peripheral renal tumors. Urology 2005;65:467-72.
10. Qin B, Hu H, Lu Y, et al. Intraoperative ultrasonography in laparoscopic partial nephrectomy for intrarenal tumors. PLoS One 2018;13:e0195911.
11. Checcucci E, Piazza P, Micali S, et al. Three-dimensional model reconstruction: the need for standardization to drive tailored surgery. Eur Urol 2022;81:129-31.
12. Fan G, Li J, Li M, et al. Three-dimensional physical model-assisted planning and navigation for laparoscopic partial nephrectomy in patients with endophytic renal tumors. Sci Rep 2018;8:582.
13. Amparore D, Pecoraro A, Checcucci E, et al. 3D imaging technologies in minimally invasive kidney and prostate cancer surgery: which is the urologists’ perception? Minerva Urol Nephrol 2022;74:178-85.
14. Piramide F, Kowalewski KF, Cacciamani G, et al. European Association of Urology Young Academic Urologists and the European Section of Uro-Technology. Three-dimensional model-assisted minimally invasive partial nephrectomy: a systematic review with meta-analysis of comparative studies. Eur Urol Oncol 2022;5:640-50.
15. Bertolo R, Kuusk T, Mir MC. Kidney Cancer EAU Edu Platform UROONCO. Three-dimensional models-assisted minimally-invasive partial nephrectomy: looking forward to more evidence. Minerva Urol Nephrol 2023;75:665-6.
16. Porpiglia F, Bertolo R, Checcucci E, et al; ESUT Research Group. Development and validation of 3D printed virtual models for robot-assisted radical prostatectomy and partial nephrectomy: urologists’ and patients’ perception. World J Urol 2018;36:201-7.
17. Maddox MM, Feibus A, Liu J, Wang J, Thomas R, Silberstein JL. 3D-printed soft-tissue physical models of renal malignancies for individualized surgical simulation: a feasibility study. J Robot Surg 2018;12:27-33.
18. Fan G, Meng Y, Zhu S, et al. Three-dimensional printing for laparoscopic partial nephrectomy in patients with renal tumors. J Int Med Res 2019;47:4324-32.
19. Yamazaki M, Takayama T, Fujita A, et al. 3D printed kidney model could be an important educational tool for residents. Asian J Endosc Surg 2023;16:197-202.
20. Fujisaki A, Takayama T, Yamazaki M, et al. Utilization of a three-dimensional printed kidney model for favorable TRIFECTA achievement in early experience of robot-assisted partial nephrectomy. Transl Androl Urol 2020;9:2697-704.
21. Moglia A, Ferrari V, Morelli L, Ferrari M, Mosca F, Cuschieri A. A systematic review of virtual reality simulators for robot-assisted surgery. Eur Urol 2016;69:1065-80.
22. Michiels C, Khene ZE, Prudhomme T, et al. 3D-Image guided robotic-assisted partial nephrectomy: a multi-institutional propensity score-matched analysis (UroCCR study 51). World J Urol 2023;41:303-13.
23. Kihara K, Saito K, Komai Y, Fujii Y. Integrated image monitoring system using head-mounted display for gasless single-port clampless partial nephrectomy. Wideochir Inne Tech Maloinwazyjne 2014;9:634-7.
24. Wu X, Jiang C, Wu G, et al. Comparison of three dimensional reconstruction and conventional computer tomography angiography in patients undergoing zero-ischemia laparoscopic partial nephrectomy. BMC Med Imaging 2020;20:47.
25. Ukimura O, Gill IS. Imaging-assisted endoscopic surgery: Cleveland Clinic experience. J Endourol 2008;22:803-10.
26. Li L, Zeng X, Yang C, Un W, Hu Z. Three-dimensional (3D) reconstruction and navigation in robotic-assisted partial nephrectomy (RAPN) for renal masses in the solitary kidney: a comparative study. Int J Med Robot 2022;18:e2337.
27. Porpiglia F, Checcucci E, Amparore D, et al. Three-dimensional augmented reality robot-assisted partial nephrectomy in case of complex tumours (PADUA ≥10): a new intraoperative tool overcoming the ultrasound guidance. Eur Urol 2020;78:229-38.
28. Teber D, Guven S, Simpfendörfer T, et al. Augmented reality: a new tool to improve surgical accuracy during laparoscopic partial nephrectomy? Preliminary in vitro and in vivo results. Eur Urol 2009;56:332-8.
29. Komai Y, Sakai Y, Gotohda N, Kobayashi T, Kawakami S, Saito N. A novel 3-dimensional image analysis system for case specific kidney anatomy and surgical simulation to facilitate clampless partial nephrectomy. Urology 2014;83:500-6.
30. Amparore D, Piramide F, Verri P, et al. New generation of 3D virtual models with perfusional zones: perioperative assistance for the best pedicle management during robotic partial nephrectomy. Curr Oncol 2023;30:4021-32.
31. Simpfendörfer T, Gasch C, Hatiboglu G, et al. Intraoperative computed tomography imaging for navigated laparoscopic renal surgery: first clinical experience. J Endourol 2016;30:1105-11.
32. Schiavina R, Bianchi L, Chessa F, et al. Augmented reality to guide selective clamping and tumor dissection during robot-assisted partial nephrectomy: a preliminary experience. Clin Genitourin Cancer 2021;19:e149-55.
33. Amparore D, Piramide F, Checcucci E, et al. Three-dimensional virtual models of the kidney with colored perfusion regions: a new algorithm-based tool for optimizing the clamping strategy during robot-assisted partial nephrectomy. Eur Urol 2023;84:418-25.
34. Tzelnick S, Rampinelli V, Sahovaler A, et al. Skull-base surgery-a narrative review on current approaches and future developments in surgical navigation. J Clin Med 2023;12:2706.
35. Shenaq DS, Matros E. Virtual planning and navigational technology in reconstructive surgery. J Surg Oncol 2018;118:845-52.
36. Grauvogel TD, Engelskirchen P, Semper-Hogg W, Grauvogel J, Laszig R. Navigation accuracy after automatic- and hybrid-surface registration in sinus and skull base surgery. PLoS One 2017;12:e0180975.
37. Zhang W, Yin D, Chen X, et al. Morphologic change of in vivo porcine liver under 13 mmHg pneumoperitoneum pressure. Surg Laparosc Endosc Percutan Tech 2021;31:679-84.
38. Okada T, Kawada K, Sumii A, et al. Stereotactic navigation for rectal surgery: comparison of 3-dimensional C-arm-based registration to paired-point registration. Dis Colon Rectum 2020;63:693-700.
39. Chen Y, Li H, Wu D, Bi K, Liu C. Surgical planning and manual image fusion based on 3D model facilitate laparoscopic partial nephrectomy for intrarenal tumors. World J Urol 2014;32:1493-9.
40. Amparore D, Pecoraro A, Checcucci E, et al. Three-dimensional virtual models’ assistance during minimally invasive partial nephrectomy minimizes the impairment of kidney function. Eur Urol Oncol 2022;5:104-8.
41. Kong SH, Haouchine N, Soares R, et al. Robust augmented reality registration method for localization of solid organs’ tumors using CT-derived virtual biomechanical model and fluorescent fiducials. Surg Endosc 2017;31:2863-71.
42. Joeres F, Mielke T, Hansen C. Laparoscopic augmented reality registration for oncological resection site repair. Int J Comput Assist Radiol Surg 2021;16:1577-86.
43. Wild E, Teber D, Schmid D, et al. Robust augmented reality guidance with fluorescent markers in laparoscopic surgery. Int J Comput Assist Radiol Surg 2016;11:899-907.
44. Kobayashi S, Cho B, Mutaguchi J, et al. Surgical navigation improves renal parenchyma volume preservation in robot-assisted partial nephrectomy: a propensity score matched comparative analysis. J Urol 2020;204:149-56.
45. Puerto-Souza GA, Cadeddu JA, Mariottini GL. Toward long-term and accurate augmented-reality for monocular endoscopic videos. IEEE Trans Biomed Eng 2014;61:2609-20.
46. Roberts S, Desai A, Checcucci E, et al. “Augmented reality” applications in urology: a systematic review. Minerva Urol Nephrol 2022;74:528-37.
47. Jia T, Taylor ZA, Chen X. Long term and robust 6DoF motion tracking for highly dynamic stereo endoscopy videos. Comput Med Imaging Graph 2021;94:101995.
48. Padovan E, Marullo G, Tanzi L, et al. A deep learning framework for real-time 3D model registration in robot-assisted laparoscopic surgery. Int J Med Robot 2022;18:e2387.
49. Su LM, Vagvolgyi BP, Agarwal R, Reiley CE, Taylor RH, Hager GD. Augmented reality during robot-assisted laparoscopic partial nephrectomy: toward real-time 3D-CT to stereoscopic video registration. Urology 2009;73:896-900.
50. Zampokas G, Tsiolis K, Peleka G, Mariolis I, Malasiotis S, Tzovaras D. Real-time 3D reconstruction in minimally invasive surgery with quasi-dense matching. In:2018 IEEE International Conference on Imaging Systems and Techniques (IST); 2018 Oct 16-18; Krakow, Poland. IEEE; 2018. pp. 1-6.
51. Zampokas G, Peleka G, Tsiolis K, Topalidou-Kyniazopoulou A, Mariolis I, Tzovaras D. Real-time stereo reconstruction of intraoperative scene and registration to preoperative 3D models for augmenting surgeons’ view during RAMIS. Med Phys 2022;49:6517-26.
52. Yip MC, Lowe DG, Salcudean SE, Rohling RN, Nguan CY. Tissue tracking and registration for image-guided surgery. IEEE Trans Med Imaging 2012;31:2169-82.
53. Zhang X, Otoo EM, Fan Y, Tao C, Wang T, Rhode K. Autostereoscopic 3D augmented reality navigation for laparoscopic surgery: a preliminary assessment. IEEE Trans Biomed Eng 2023;70:1413-21.
54. Kokko MA, Van Citters DW, Seigne JD, Halter RJ. A particle filter approach to dynamic kidney pose estimation in robotic surgical exposure. Int J Comput Assist Radiol Surg 2022;17:1079-89.
Cite This Article
Export citation file: BibTeX | EndNote | RIS
OAE Style
Magaribuchi T, Masui K, Goto T, Saito R, Kobayashi T. Current status and challenges of 3D navigation in partial nephrectomy. Mini-invasive Surg 2024;8:6. http://dx.doi.org/10.20517/2574-1225.2023.81
AMA Style
Magaribuchi T, Masui K, Goto T, Saito R, Kobayashi T. Current status and challenges of 3D navigation in partial nephrectomy. Mini-invasive Surgery. 2024; 8: 6. http://dx.doi.org/10.20517/2574-1225.2023.81
Chicago/Turabian Style
Toshihiro Magaribuchi, Kimihiko Masui, Takayuki Goto, Ryoichi Saito, Takashi Kobayashi. 2024. "Current status and challenges of 3D navigation in partial nephrectomy" Mini-invasive Surgery. 8: 6. http://dx.doi.org/10.20517/2574-1225.2023.81
ACS Style
Magaribuchi, T.; Masui K.; Goto T.; Saito R.; Kobayashi T. Current status and challenges of 3D navigation in partial nephrectomy. Mini-invasive. Surg. 2024, 8, 6. http://dx.doi.org/10.20517/2574-1225.2023.81
About This Article
Special Issue
Copyright
Data & Comments
Data
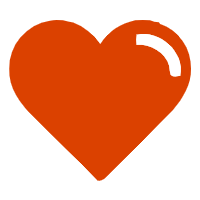
Comments
Comments must be written in English. Spam, offensive content, impersonation, and private information will not be permitted. If any comment is reported and identified as inappropriate content by OAE staff, the comment will be removed without notice. If you have any queries or need any help, please contact us at support@oaepublish.com.