Artificial intelligence in capsule endoscopy: development status and future expectations
Abstract
In this review, we aim to illustrate the state-of-the-art artificial intelligence (AI) applications in the field of capsule endoscopy. AI has made significant strides in gastrointestinal imaging, particularly in capsule endoscopy - a non-invasive procedure for capturing gastrointestinal tract images. However, manual analysis of capsule endoscopy videos is labour-intensive and error-prone, prompting the development of automated computational algorithms and AI models. While currently serving as a supplementary observer, AI has the capacity to evolve into an autonomous, integrated reading system, potentially significantly reducing capsule reading time while surpassing human accuracy. We searched Embase, Pubmed, Medline, and Cochrane databases from inception to 06 Jul 2023 for studies investigating the use of AI for capsule endoscopy and screened retrieved records for eligibility. Quantitative and qualitative data were extracted and synthesised to identify current themes. In the search, 824 articles were collected, and 291 duplicates and 31 abstracts were deleted. After a double-screening process and full-text review, 106 publications were included in the review. Themes pertaining to AI for capsule endoscopy included active gastrointestinal bleeding, erosions and ulcers, vascular lesions and angiodysplasias, polyps and tumours, inflammatory bowel disease, coeliac disease, hookworms, bowel prep assessment, and multiple lesion detection. This review provides current insights into the impact of AI on capsule endoscopy as of 2023. AI holds the potential for faster and precise readings and the prospect of autonomous image analysis. However, careful consideration of diagnostic requirements and potential challenges is crucial. The untapped potential within vision transformer technology hints at further evolution and even greater patient benefit.
Keywords
INTRODUCTION
Since its inception in 2001, wireless capsule endoscopy (WCE) has revolutionised the investigation and diagnosis of gastrointestinal (GI) diseases[1]. However, the process of reading WCE images, along with interpreting and diagnosing, is highly labour-intensive and error-prone considering that it is reliant on the expertise of the reader and tens of thousands of video frames collected, of which potentially only a few contain the lesion or pathology to be found. Hence, it is understandable that readers, with their limited attention spans and concentration, may miss pathology or over/underdiagnose lesions which are detected[2]. This is why capsule endoscopy offers a “fertile” field for artificial intelligence (AI) algorithms to be implemented, where AI can significantly streamline the reading process. Several commercial AI systems are already available, such as Quick-View and Express-View, which can recognise potential lesions and remove insignificant video frames. By identifying and selecting images with potential pathology for review and removing those with no suspicion of pathology, these programs decrease the total amount of images the reader is required to view, hence reducing overall reading time. This narrative review aimed to assess and synthesise the current evidence on the AI applications in enhancing the capability and efficiency of capsule endoscopy for investigation of the GI tract and propose future directions for this technology.
METHODS
Methodology for this review was formulated prior to its conduct. Ovid Embase, PubMed (incorporating MEDLINE), and Cochrane databases were searched from database inception to 06 July 2023, with a mixture of Medical Subject Headings (MESH) and free text terms including capsule endoscopy keywords such as “Capsul*”, “Endoscop*”, and “Gastroscop*”, AI-related keywords such as “Artificial Intelligence”, “AI”, “Convolutional Neural Network”, “Deep Learning”, “Computer-Assisted Diagnosis”, “Computer-Assisted Detection”, “Transformer”, and “Vision Transformer”, and common capsule endoscopy findings such as “Ulcer”, “Erosion”, “Vascular Lesion”, “Lesion”, “Gastrointestinal Bleed”, “Dieulafoy”, “Arteriovenous Malformation”, “Inflammatory Bowel Disease”, “Crohn’s Disease”, “Ulcerative Colitis”, “Coeliac Disease”, “Coeliac Sprue”, “Gluten-Sensitive Enteropathy”, “Neoplasm”, “Polyp”, “Cancer”, “Tumour”, and “Bowel Prep”.
Study screening was conducted by three reviewers (A.G., J.K., and J.T.), with disagreements resolved through consensus. Selection criteria were based on their relevance to the research topic of AI for capsule endoscopy. Articles were screened for AI applications, ensuring they focused on one of the sub-categories that were planned a priori: “Active GI Bleeding”, “Erosion and Ulcers”, “Angiodysplasia”, “Polyps and Tumours”, “Inflammatory Bowel Disease”, “Coeliac Disease”, “Hookworm”, and “Other Applications”. Furthermore, they were required to have constructed their own AI tool, including modalities such as support vector machines (SVMs), Multilayer Perceptrons, and convolutional neural networks (CNNs). Furthermore, they were screened for relevance to the field of capsule endoscopy, including domains such as Colon Capsule Endoscopy and Small-Bowel Capsule Endoscopy. Studies were excluded if they were not in English, were conference abstracts, did not report observational data (e.g., review articles), or did not conform to the inclusion criteria listed above.
SEARCH RESULTS
In our search, 824 articles were retrieved, of which 291 duplicates and 31 abstracts were removed. After study screening and full-text review, 106 articles were included for analysis in the present review. Data was synthesised into tabular and narrative formats. For studies with multiple trials, the best result achieved by the models was used.
Additionally, we have designed a modified PRISMA flow-chart [Figure 1].
RESULTS
Active GI bleeding
Automatic haemorrhage detection is one of the largest researched applications of AI for capsule endoscopy. From machine learning models such as the SVM and probabilistic neural network (PNN) methods[3-7], the field has progressed into deep learning models with enhanced efficacy and accuracy. Other models utilising multi-layer perceptrons (MLP)[8] and back-propagation neural networks[4] have also been replaced with deep learning, with this shift appearing to primarily have occurred post-2016. Only four SVM-based models[9-12] were constructed following 2016, compared with eight CNN models[13-20] and two Kernel Neural Networks[21,22]. For example, in 2021, Ghosh et al. constructed a CNN-based deep learning framework via the CNN architecture AlexNet, achieving a sensitivity of 97.51% and specificity of 99.88%, significantly enhanced from the sensitivity of approximately 80% previously mentioned by Girithiran et al.[3,17]. However, SVM models such as that of Rathnamala et al. in 2021 also produced excellent results, with a sensitivity of 99.83% and specificity of 100% reported[12]. More recently, in 2022, Mascarenhas Saraiva et al. constructed a CNN detecting blood and haematic residues in the small blood lumen with a sensitivity and specificity of 98.6% and 98.9%, respectively, with an impressive speed of around 184 frames/s[19]. Based on the current literature for gastrointestinal haemorrhage, incorporating AI significantly improves investigative capability. However, further implementation work is necessary to optimise its accuracy [Table 1].
Table of AI applications in capsule endoscopy for active GI bleeding
Ref. | Application | Year of publication | Study design | Study location | Aim | Training/Validation dataset | AI type | Results |
Giritharan et al.[3] | Active GI bleeding | 2008 | Retrospective | America | Develop a method to re-balance training images | 550 bleeding images | SVM | Sensitivity of 80% |
Li and Meng[8] | Active GI bleeding | 2009 | Retrospective | China | Develop new CAD system utilising colour-texture features and neural network classifier | Training: 1,800 bleeding patches and 1,800 normal patches Testing: 1,800 bleeding patches and 1,800 normal patches | MLP | Sensitivity of 92.6%, specificity of 91% |
Pan et al.[4] | Active GI bleeding | 2009 | Retrospective | China | Use colour-texture features in RGB and HSI as input in BP neural network | Training: 10,000 pixels Testing: 3,172 bleeding images and 11,458 non-bleeding images | BP neural network | Sensitivity of 93%, specificity of 96% |
Pan et al.[7] | Active GI bleeding | 2011 | Retrospective | China | Use colour-texture features in RGB and HSI as input in PNN | Training: 50,000 pairs Testing: 3,172 bleeding images and 11,458 non-bleeding images | PNN | Sensitivity of 93.1%, specificity of 85.8% |
Ghosh et al.[5] | Active GI bleeding | 2014 | Retrospective | Bangladesh | Use RGB colour-texture feature in SVM | Training: 50 bleeding images and 200 non-bleeding images Testing: 400 bleeding and 1,600 non-bleeding images | SVM | Sensitivity of 93.00%, specificity of 94.88% |
Hassan and Haque[6] | Active GI bleeding | 2015 | Retrospective | Bangladesh | Utilise characteristic patterns in frequency spectrum of WCE images | Training: 600 bleeding and 600 non-bleeding frames Testing: 860 bleeding and 860 non-bleeding images | SVM | Sensitivity of 99.41%, specificity of 98.95% |
Yuan et al.[9] | Active GI bleeding | 2016 | Retrospective | China | Construct two-fold system for detection and localisation of bleeding regions | Testing: 400 bleeding frames and 2,000 normal frames | SVM and KNN | Sensitivity of 92%, specificity of 96.5% |
Jia and Meng[13] | Active GI bleeding | 2016 | Retrospective | China | Develop deep neural network that can automatically and hierarchically learn high-level features | Training: 2,050 bleeding and 6,150 non-bleeding images Testing: 800 bleeding, 1,000 non-bleeding | CNN | Sensitivity of 99.20%* |
Jia and Meng[14] | Active GI bleeding | 2017 | Retrospective | China | Combine handcrafted and CNN features for characterisation | Training: 200 bleeding frames and 800 normal frames Testing: 100 bleeding frames and 400 normal frames | CNN | Sensitivity of 91% |
Kundu et al.[21] | Active GI bleeding | 2018 | Retrospective | Bangladesh | Detecting bleeding images based on precise ROI detection in normalised RGB colour plane | Testing: 5 videos, with 100 image frames each | KNN | Sensitivity of 85.7%, specificity of 69.6% |
Ghosh et al.[10] | Active GI bleeding | 2018 | Retrospective | Bangladesh/ Canada | Utilising cluster-based statistical feature extraction for global feature vector construction | Testing: 5 WCE videos | SVM | Sensitivity of 96.5%, specificity of 94.6% |
Xing et al.[22] | Active GI bleeding | 2018 | Retrospective | China | Using SPCH feature based on the principal colour spectrum to discriminate bleeding frames | Training: 340 bleeding frames and 340 normal ones Testing: 160 bleeding frames and 160 normal ones | KNN | Sensitivity of 98.5%, specificity of 99.5% |
Pogorelov et al.[11] | Active GI bleeding | 2019 | Retrospective | Malaysia/ Norway | Combining colour features in RGB and texture features for bleeding detection | Training: 300 bleeding frames and 200 non-bleeding Testing: 500 bleeding and 200 non-bleeding frames | SVM | Sensitivity of 97.6%, specificity of 95.9% |
Hajabdollahi et al.[15] | Active GI bleeding | 2019 | Retrospective | Iran | Developing a low-complexity CNN method | Training and testing on KID[110] | CNN | Sensitivity of 94.8%, specificity of 99.1% |
Kanakatte and Ghose[16] | Active GI bleeding | 2021 | Prospective | India | Proposing compact U-Net model | Training: 700 bleeding and 700 non-bleeding Testing: 50 capsule endoscopy images | CNN | Sensitivity of 99.57%, specificity of 91% |
Rathnamala and Jenicka[12] | Active GI bleeding | 2021 | Retrospective | India | Utilising gaussian mixture model superpixels for bleeding detection | Training: 686 bleeding and 961 non-bleeding images Testing: 487 bleeding images and 1,160 non-bleeding images | SVM | Sensitivity of 99.83%, specificity of 100% |
Ghosh and Chakareski[17] | Active GI bleeding | 2021 | Retrospective | America | Develop CNN-based framework for bleeding identification | Alex-Net training: 1,410 Alex-Net testing: 940 SegNet training: 201 SegNet testing: 134 | CNN | Sensitivity of 97.51%, specificity of 99.88% |
Ribeiro et al.[18] | Active GI bleeding | 2021 | Retrospective | Portugal | Automatic detection and differentiation of vascular lesions | Training: 820 images with red spots, 830 images with angiodysplasia/varices, 7,620 images with normal mucosa Testing: 206 images with red spots, 207 images with angiodysplasia/varices, 1,905 images with normal mucosa | CNN | Sensitivity of 91.8%, specificity of 95.9% |
Mascarenhas Saraiva et al.[19] | Active GI bleeding | 2022 | Retrospective | Portugal | Create CNN-based system for automatic detection of blood or haematic traces in small bowel lumen | Training: 10,808 images containing blood, 6,868 with normal mucosa or other distinct pathological findings Testing; 2,702 images containing blood, 1,717 with normal mucosa or other distinct pathological findings | CNN | Sensitivity of 98.6%, specificity of 98.9% |
Muruganantham and Balakrishnan[20] | Active GI bleeding | 2022 | Retrospective | India | Construct dual branch CNN model with a novel lesion attention map estimator model | Training and testing conducted on bleeding[111] and Kvasir-Capsule dataset[112] Training: 3,430 images Testing: 1,470 images | CNN | No sensitivity and specificity could be found Accuracy of 94.40% for bleeding detection on bleeding dataset Accuracy of 93.18% for ulcer, 93.89% for bleeding, 97.73% for polyp, 96.67% for normal on Kvasir-Capsule dataset |
Erosion and ulcers
Erosions and ulcers are among the most common findings on WCE. These lesions have reduced visual features compared to visibly haemorrhagic lesions, as seen above, and hence, their characterisation is more difficult. Earlier work, as demonstrated by Charisis et al., utilising Bi-dimensional Ensemble Empirical Mode Decomposition and SVMs to identify ulcers obtained a sensitivity and specificity of around 95%[23]. While other MLP and SVM models were created prior to 2014 with similar accuracies[24-26], the earliest study utilising a deep learning framework for the detection of ulcers and erosions is believed to be the work by Fan et al. in 2018, which employed a CNN achieving a sensitivity of 96.80% and 94.79% and specificity of 94.79% and 95.98%, respectively[27]. Since 2018, only two non-deep learning models were retrieved[28,29] in comparison to 14 deep learning models[30-42]. Most recently, in 2023, Nakada et al. published their use of the RetinaNet model to diagnose multiple types of lesions including erosions, ulcers, vascular lesions, and tumours[43]. This study obtained a sensitivity of 91.9% and specificity of 93.6% in the detection of erosions and ulcers [Table 2].
Table of AI applications in capsule endoscopy for erosions and ulcers
Ref. | Application | Year of publication | Study design | Study location | Aim and goals | Training/Validation dataset | AI type | Results |
Li and Meng[24] | Erosions and ulcers | 2009 | Retrospective | China | Utilising chromaticity moment to discriminate normal regions and abnormal region | Training: 1,350 normal samples and 1,350 abnormal samples Testing: 450 normal samples and 450 abnormal samples | MLP | Bleeding: sensitivity of 87.81%, specificity of 88.62% Ulcer: sensitivity of 84.68%, specificity of 92.97% |
Charisis et al.[23] | Erosions and ulcers | 2010 | Retrospective | Greece | Using BEEMD to extract intrinsic mode functions | Dataset: 40 normal and 40 ulcerous images 90% for training, 10% for testing | SVM | Sensitivity of 95%, specificity of 96.5% |
Charisis et al.[25] | Erosions and ulcers | 2012 | Retrospective | Greece | Associate colour with structure information in order to discriminate between healthy and ulcerous tissue | 87 normal images, 50 “easy ulcer case” images, 37 “hard ulcer case” images 90% was used for training, 10% for testing | MLP and SVM | SVM: sensitivity of 98.9%, specificity of 96.9%, for “easy ulcer”; sensitivity of 95.2%, specificity of 88.9%, for “hard ulcer” MLP: sensitivity of 94.6%, specificity of 98.2%, for “easy ulcer”; sensitivity of 82%, specificity of 95.1%, for “hard ulcer” |
Iakovidis and Koulaouzidis[26] | Erosions and ulcers | 2014 | Retrospective | Greece/ United Kingdom | Derive colour feature-based pattern recognition method | Training: 1,233 images Testing: 137 images | SVM | Sensitivity of 95.4%, specificity of 82.9% |
Fan et al.[27] | Erosions and ulcers | 2018 | Retrospective | China | Automatic erosion detection via deep neural network | Ulcer training: 2,000 ulcer images, 2,400 normal images Ulcer testing: 500 ulcer images, 600 normal images Erosion training: 2,720 ulcer images, 3,200 normal images Erosion testing: 690 ulcer images, 800 normal images | CNN | Ulcers: sensitivity of 96.8%, specificity of 94.79% Erosions: sensitivity of 93.67%, specificity of 95.98% |
Khan et al.[28] | Erosions and ulcers | 2019 | Retrospective | Pakistan | Utilising DenseNet CNN for stomach abnormality classification | Training: 2,800 ulcers, 2,800 bleeding, and 2,800 healthy regions Testing: 1,200 ulcers, 1,200 bleeding, and 1,200 healthy regions | MLP | Sensitivity of 99.40%, specificity of 99.20% |
Wang et al.[30] | Erosions and ulcers | 2019 | Retrospective | China | Use deep convolutional neural networks to provide classification confidence score and bounding box marking area of suspected lesion | Training: 15,781 ulcer frames and 17,138 normal frames Testing: 4,917 ulcer frames and 5,007 normal frames | CNN | Sensitivity of 89.71%, specificity of 90.48% |
Aoki et al.[31] | Erosions and ulcers | 2019 | Retrospective | Japan | Develop CNN system based on a single shot multibox detector | Training: 5,360 ulcer and erosion images Testing: 440 ulcer and erosion images, 10,000 normal images | CNN | Sensitivity of 88.2%, specificity of 90.9% |
Ding et al.[32] | Erosions and ulcers | 2019 | Retrospective | China | Characterise SB-CE images as multiple lesion types | Training: 158,235 images from 1,970 patients Testing: 113, 268, 334 images from 5,000 patients | CNN | Sensitivity of 99.90%, specificity of 100% |
Majid et al.[33] | Erosions and ulcers | 2020 | Retrospective | Pakistan | Using multi-type features extraction, fusion, and features selection to detect ulcer, polyp, esophagitis, and bleeding | Training: 6,922 images of bleeding, oesophagitis, polyp, and ulcerative colitis Testing: 2,967 images of bleeding, oesophagitis, polyp, and ulcerative colitis | CNN | Sensitivity of 96.5% |
Kundu et al.[29] | Erosions and ulcers | 2020 | Retrospective | Bangladesh | Employing LDA for ROI separation | Training: 65 bleeding, 31 ulcers, and 30 tumour images Testing: 15 continuous video clips | SVM | Sensitivity of 85.96%, specificity of 92.24% |
Otani et al.[34] | Erosions and ulcers | 2020 | Retrospective | Japan | Multiple lesion detection using RetinaNet | Database of 398 images of erosions and ulcers, 538 images of angiodysplasias, 4,590 images of tumours, and 34,437 normal images for training and testing | Deep neural network | No sensitivity and specificity reported |
Xia et al.[35] | Erosions and ulcers | 2021 | Retrospective | China | Novel CNN and RCNN system to detect 7 types of lesions in MCE imaging | Training: 822,590 images Testing: 201,365 images | CNN, RCNN | Sensitivity of 96.2%, specificity of 76.2% |
Afonso et al.[36] | Erosions and ulcers | 2021 | Retrospective | Portugal | Identify but also differentiate ulcers and erosions based on haemorrhagic potential | Training: 18,976 images Testing: 4,744 images | CNN | Sensitivity of 86.6%, specificity of 95.9% |
Mascarenhas Saraiva et al.[37] | Erosions and ulcers | 2021 | Retrospective | Portugal | Identify various lesions on CE images and differentiate using Saurin’s classification | Training: 42,844 images Testing: 10,711 images | CNN | Sensitivity of 88%, specificity of 99% |
Afonso et al.[38] | Erosions and ulcers | 2022 | Retrospective | Portugal | Identify but also differentiate ulcers and erosions based on haemorrhagic potential | Training: 4,904 images Testing: 379 normal images, 266 erosion, 286 P1 Ulcer images, 295 P2 Ulcer images | CNN | Sensitivity of 90.8%, specificity of 97.1% |
Mascarenhas et al.[39] | Erosions and ulcers | 2022 | Retrospective | Portugal | Develop CNN-based method to detect and distinguish colonic mucosal lesions and luminal blood in CCE imaging | Training: 7,204 images Testing: 1,801 | CNN | Sensitivity of 96.3%, specificity of 98.2% |
Xiao et al.[40] | Erosions and ulcers | 2022 | Retrospective sensitivity of 96.9% and a specificity of 99.9% specific | China | Classify capsule gastroscope images into normal, chronic erosive gastritis, and gastric ulcer categories | Training: 228 images Testing: 912 images | CNN | No sensitivity and specificity, accuracy of 94.81% |
Ribeiro et al.[41] | Erosions and ulcers | 2022 | Retrospective | Portugal | Accurately detect ulcers and erosions in CCE images | Training: 26,869 images Testing: 3,375 normal images, 357 images with ulcers or colonic erosions | CNN | Sensitivity of 96.9%, specificity of 99.9% |
Nakada et al.[43] | Erosions and ulcers | 2023 | Retrospective | Japan | Utilise RetinaNet to diagnose erosions and ulcers, vascular lesions, and tumours in WCE imaging | Training: 6,476 erosion and ulcer images, 1,916 angiodysplasias images, 7,127 tumour images, 14,014,149 normal images Testing: images from 217 patients | Deep neural network | Erosions and ulcers: sensitivity of 91.9%, specificity of 93.6% Vascular lesions: sensitivity of 87.8%, specificity of 96.9% Tumours: sensitivity of 87.6%, specificity of 93.7% |
Raut et al.[42] | Erosions and ulcers | 2023 | Retrospective | India | Use various feature extraction methods in the classification of WCE images as inflammatory, polypoid and ulcer | Training and testing on KID dataset[110] | Deep neural network | Sensitivity of 97.23%, specificity of 52.00% |
Vascular lesions and angiodysplasias
Angiodysplasias, defined as accumulations of dilated, tortuous, and dilated blood vessels in the mucosa and submucosa of the intestinal wall, are common pathologies that can cause small intestinal bleeding. The first record of a software tool for the diagnosis of enteric lesions, including angiodysplasias, was the work by Gan et al. in 2008, which used Image Processing Software to obtain a median sensitivity of 74.2%[44]. Only two non-deep learning models were retrieved in the search: a study by Arieira et al. on evaluating the accuracy of the TOP 100 feature of Rapid Reader™[45] and a 2019 investigation by Vieira et al. on MLP and SVMs which obtained sensitivities above 96%[46]. Since 2019, only deep learning models have been employed in this field[47-53]. In 2018, Leenhardt et al. published their CNN model for detecting gastrointestinal angiodysplasias[54]. An exceptional sensitivity of 100% and specificity of 95.8% were obtained. Moreover, they assisted in constructing a French national database (CAD-CAP) to collect and maintain high-quality capsule endoscopy images for the training and validation of AI assistive tools. Recently, in 2023, Chu et al. published their CNN constructed on Resnet-50 architecture, which obtained a positive predictive value of 94% and negative predictive value of 98%, in addition to the capability of segmenting and recognising an image in 0.6 s[53] [Table 3].
Table of AI applications in capsule endoscopy for vascular lesions and angiodysplasias
Ref. | Application | Year of publication | Study design | Study location | Aim and goals | Training/Validation dataset | AI type | Results |
Gan et al.[44] | Vascular lesions and angiodysplasias | 2008 | Retrospective | China | Develop computer-aided screening and diagnosis for enteric lesions in CE | Dataset of 236 patients with lesion, and 86 without lesion for training and validation | IPS | Median sensitivity of 74.2% |
Leenhardt et al.[54] | Vascular lesions and angiodysplasias | 2018 | Retrospective | France | Utilise CNN for detection of AGD in SB-CE images | Training: 300 normal frames, 300 AGD frames Testing: 300 normal frames, 300 AGD frames | CNN | Sensitivity of 100%, specificity of 96% |
Arieira et al.[45] | Vascular lesions and angiodysplasias | 2019 | Retrospective | Portugal | Evaluate accuracy and efficacy of “TOP 100” feature | Testing: 97 patients | TOP 100 | No sensitivity or specificity. Accuracy of 83.5% for P2 lesions, 95.5% for AGD, 56.7% for ulcers, 100% for active bleeding sites |
Vieira et al.[46] | Vascular lesions and angiodysplasias | 2019 | Retrospective | Portugal | Automatic detection of AGD in WCE videos | Dataset: 27 images from KID database[110], additional 248 AGD images, 550 normal images | MLP and SVM | MLP: sensitivity of 96.60%, specificity of 94.08% SVM: sensitivity of 96.58%, specificity of 92.24% |
Vezakis et al.[47] | Vascular lesions and angiodysplasias | 2019 | Retrospective | Greece | Combining of low-level image analysis, feature detection, and machine learning for AGD detection in WCE images | Training: 350 normal images, 196 bubble images, 75 blood vessel images, 104 AGD images Testing: 3 full-length WCE | CNN | Sensitivity of 92.7%, specificity of 99.5% |
Leenhardt et al.[48] | Vascular lesions and angiodysplasias | 2019 | Retrospective | France | Develop CNN methodology to detect GIA in SB-CE | Training: 300 normal frames, 300 GIA frames GIA Testing: 300 normal frames, 300 GIA frames | CNN | Sensitivity of 100%, specificity of 96% |
Tsuboi et al.[49] | Vascular lesions and angiodysplasias | 2020 | Retrospective | Japan | Development of CNN system based on SSMB for small bowel AGD detection | Training: 2,237 angiodysplasia images Testing: 488 AGD images, 10,000 normal images | CNN | Sensitivity of 98.8%, specificity of 98.4% |
Aoki et al.[50] | Vascular lesions and angiodysplasias | 2021 | Retrospective | Japan | Construct CNN based system for various abnormality detection | Training: 44,684 images of abnormalities and 21,344 normal images Testing: 379 full small-bowel CE videos | CNN | No sensitivity or specificity reported. Accuracy of 100% for mucosal breaks, 97% for AGD, 99% for protruding lesions, and 100% for blood content |
Hwang et al.[51] | Vascular lesions and angiodysplasias | 2021 | Retrospective | Korea | Develop CNN algorithm for categorisation of SBCE videos into haemorrhagic lesions and ulcerative lesions | Training: 11,776 haemorrhagic lesions, 18,448 ulcerative lesions, 30,224 normal images Testing: 5,760 images | CNN | Sensitivity of 97.61%, specificity of 96.04% |
Hosoe et al.[52] | Vascular lesions and angiodysplasias | 2022 | Retrospective | Japan | Detect common findings on SBCE images using CNN framework with aim to reduce false-positive rate | Training: 33 SBCE cases Testing: 35 SBCE cases | CNN | Sensitivity of 93.4%, specificity of 97.8% |
Chu et al.[53] | Vascular lesions and angiodysplasias | 2023 | Retrospective | China | Utilise CNN segmentation method for AGD detection | Training: 178 cases Testing: 200 cases | CNN | No sensitivity or specificity given. Pixel accuracy of 99% |
Polyps and tumours
The significance of detecting polyps and tumours stems from their potential to cause significant morbidity and mortality. A substantial body of research has been devoted to exploring AI-assisted capsule endoscopy for accurate identification and detection of these lesions. Early research in AI-assisted capsule endoscopy for this application includes a study by Li et al. in 2011, which utilised colour texture features to differentiate between normal and tumour-containing images with a sensitivity of 92.33% and a specificity of 88.67%[55]. Multiple other machine learning models utilising Binary Classifiers, SVMs, and MLPs have been utilised to varying accuracies and efficacies[56-61]. Deep learning was integrated into the field with the study by Yuan and Meng in 2017[62], where they utilised a stacked sparse autoencoder method to categorise images into polyps, bubbles, turbid images, and clear images with an overall accuracy of 98.00%. Since then, 12 deep learning applications were used for polyp and tumour detection[63-74]. More recently, a study by Lafraxo et al. in 2023 proposed an innovative model using CNN (Resnet50), where they achieved an accuracy of 99.16% on the MICCAI 2017 WCE dataset[73]. In 2022, the research conducted by Piccirelli et al. investigating the diagnostic accuracy of Express View of IntroMedic achieved a 97% sensitivity and 100% specificity[75]. As AI polyp detection tools are commercially available for colonoscopy, such as FujiFilm’s CADeye[76] and EndoBRAIN (Olympus), the imminent release and usage of AI tools for capsule endoscopy is expected with these promising results, which will likely only be further supported by future research such as the planned multi-centre CESCAIL study[77] [Table 4].
Table of AI applications in capsule endoscopy for polyps and tumours
Ref. | Application | Year of publication | Study design | Study location | Aim and goals | Training/Validation dataset | AI type | Results |
Li et al.[55] | Polyps and tumours | 2011 | Retrospective | China | Utilise textural feature based on multi-scale local binary pattern for tumour detection | Training: 450 normal samples, 450 tumour samples Testing: 150 normal samples, 150 tumour samples | KNN MLP SVM | Best Results: sensitivity of 92.33%, specificity of 88.67% |
Karargyris and Bourbakis[56] | Polyps and tumours | 2011 | Retrospective | America | Utilising log Gabor filters for feature extraction to detect polyps and ulcers | Polyps testing: 10 frames with polyps, 40 normal frames Ulcer testing: 20 ulcer frames, 30 non-ulcer frames | SVM | Ulcer detection: sensitivity of 75.0%, specificity of 73.3% Polyp detection: sensitivity of 100%, specificity of 67.5% |
Barbosa et al.[57] | Polyps and tumours | 2012 | Retrospective | Portugal | Extracting textural features to detect polyps and tumours | Dataset for training and testing: 700 tumour images, 2,300 normal images | MLP | Sensitivity of 93.9%, specificity of 93.1% |
Mamonov et al.[58] | Polyps and tumours | 2014 | Retrospective | USA/Portugal | Development of binary classifier for tumour detection geometrical analysis and texture content | Dataset for training and testing: 230 tumour images, 18,738 normal images | BC | Per frame: sensitivity of 47.4%, specificity of 90.2% Per polyp: sensitivity of 81.25%, specificity of 93.47% |
Liu et al.[59] | Polyps and tumours | 2016 | Retrospective | China | Integrating multi-scale curvelet and fractal technology into textural features for polyp detection | Training: WCE videos of 15 patients Testing: 900 normal frames, 900 tumour frames | SVM | Sensitivity of 97.8%, specificity of 96.7% |
Yuan and Meng[62] | Polyps and tumours | 2017 | Retrospective | China | Construction of SSAEIM for polyp detection | Testing: 1,000 bubble images, 1,000 TIs, 1,000 CIs, 1,000 polyp images | SSAEIM | Polyps: sensitivity of 98%, specificity of 99% Bubbles: sensitivity of 99.5%, specificity of 99.17% TIs: sensitivity of 99%, specificity of 100% CIs: sensitivity of 95.5%, specificity of 99.17% |
Blanes-Vidal et al.[74] | Polyps and tumours | 2019 | Retrospective | Denmark | Developed algorithm to match CCE and colonoscopy polyps and construct CNN for polyp detection | Training: 39,550 images Testing: 8,476 images | CNN | Sensitivity of 97.1%, specificity of 93.3% |
Saito et al.[63] | Polyps and tumours | 2020 | Retrospective | Japan | Constructing CNN model for protruding lesion detection | Training: 30,584 protruding lesion images Testing: 7,507 protruding lesion images, 10,000 normal images | CNN | Sensitivity of 90.7%, specificity of 79.8% |
Yang et al.[60] | Polyps and tumours | 2020 | Retrospective | China | Development of algorithm based on LCDH for polyp detection | Testing: 500 normal, 500 polyp images | SVM | Sensitivity of 95.80%, specificity of 96.20% |
Vieira et al.[61] | Polyps and tumours | 2020 | Retrospective | Portugal | Construction of GMM and ensemble system for tumour detection | Database of 936 tumour images, 3,000 normal images for training and testing | SVM MLP | Best result: sensitivity of 96.1%, specificity of 98.3% |
Yamada et al.[64] | Polyps and tumours | 2021 | Retrospective | Japan | Construction of CNN based on SSMD for colorectal neoplasm detection | Training: 15,933 colorectal neoplasm images Testing: 1,850 colorectal neoplasm images, 2,934 normal colon images | CNN | Sensitivity of 79.0%, specificity of 87% |
Saraiva et al.[65] | Polyps and tumours | 2021 | Retrospective | Portugal | Development of CNN for protruding lesion detection on CCE imaging | Database: 860 protruding lesions images, 2,780 normal mucosa images Training: 2,912 images of database Testing: 728 images of database | CNN | Sensitivity of 90.7%, specificity of 92.6% |
Jain et al.[66] | Polyps and tumours | 2021 | Retrospective | India | Creation of deep CNN based WCENet model for anomaly detection in WCE images | Training and testing on KID database[110] and CVC-clinic database[113] | CNN | Sensitivity of 98% |
Zhou et al.[67] | Polyps and tumours | 2022 | Retrospective | China | Utilising neural network ensembles to improve polyp segmentation | Training: 195 images Testing: 41 images | CNN | No sensitivity and specificity reported |
Mascarenhas et al.[68] | Polyps and tumours | 2022 | Retrospective | Portugal | Construction of CNN for protruding lesion detection on CCE | Training: 1,928 protruding lesion images, 2,644 normal/other finding imagesTesting: 482 protruding lesion images, 661 normal/other finding images | CNN | Sensitivity of 90.0%, specificity of 99.1% |
Gilabert et al.[69] | Polyps and tumours | 2022 | Retrospective | Spain | Comparing AI tool to RAPID Reader Software v9.0 (Medtronic) | Testing: 18 videos | CNN | Sensitivity of 87.8% |
Piccirelli et al.[75] | Polyps and tumours | 2022 | Retrospective | Italy | Testing the diagnostic accuracy of Express View (IntroMedic) | Testing: 126 patients | Express view | Sensitivity of 97%, specificity of 100% |
Liu et al.[70] | Polyps and tumours | 2022 | Retrospective | China | Constructing DBMF fusion network with CNN and transformer for polyp segmentation | Training: 1,450 images Testing: 636 images | DBMF | No sensitivity and specificity given |
Souaidi et al.[71] | Polyps and tumours | 2023 | Retrospective | Morocco | Modifying existing SSMD models for polyp detection | Training: 2,745 images Testing: 784 images | SSMD | No sensitivity and specificity given |
Mascarenhas Saraiva et al.[72] | Polyps and tumours | 2023 | Retrospective | Portugal | Developing CNN for automatic detection of small bowel protruding lesions | Training: 14,900 images Testing: 3,725 images | CNN | Sensitivity of 96.8%, specificity of 96.5% |
Lafraxo et al.[73] | Polyps and tumours | 2023 | Retrospective | Morocco | Proposing novel CNN-based architecture for GI image segmentation | MICCAI2017[114]: training: 2,796 images Testing: 652 images Kvasir-SEG dataset [115]: Training: 800 images Testing: 200 images CVC-ClinicDB dataset[116]: Training: 490 images Testing: 122 images | CNN | No sensitivity or specificity given. Accuracy of 99.16% on MICCAI2017 reported, 97.55% on Kvasir-SEG, and 97.58% on CVC-ClinicDB databases respectively |
Lei et al.[77] | Polyps and tumours | 2023 | Combined prospective/retrospective | United Kingdom | Study is proposed to determine efficacy of AI tools for polyp detection in capsule endoscopy | Study is incomplete | CNN | Study is incomplete |
Inflammatory bowel disease
Potential AI tools to improve the detection and assessment of ulcers and mucosal inflammation caused by Crohn’s disease have been researched for over a decade. In 2012, Kumar et al. published their work using a cascade for classifying CD lesions and quantitatively assessing their severity[78]. The severity assessment given (normal, mild, and severe) by the model was shown to correlate well with those manually assigned by experts. While multiple machine learning models have achieved reasonable sensitivities and specificities in this field[79-81], since 2018, deep learning systems have predominated research[81-92]. In 2022, Ferreira et al. developed a CNN using a total of 8,085 images to detect ulcers and erosions in images from the PillCam™ Crohn’s Capsule, with an overall sensitivity of 90% and specificity of 96%[89]. Higuchi et al. published their work using CNN-based models to automatically classify ulcerative colitis lesion severity based on the Mayo Endoscopic Subscore, achieving an accuracy of 98.3%[90]. While reasonable results have been achieved, ulcers and erosions typically have fewer colour features compared to active bleeding lesions, making their detection and classification generally more difficult [Table 5].
Table of AI applications in capsule endoscopy for inflammatory bowel disease
Ref. | Application | Year of publication | Study design | Study location | Aim and goals | Training/Validation dataset | AI type | Results |
Haji-Maghsoudi et al.[79] | Inflammatory bowel disease | 2012 | Retrospective | Iran | Develop method for the detection of lymphangiodysplasia, xanthoma, CD, and stenosis in WCE image | Stenosis: 45 images CD: 74 images Lymphangiectasia: 32 images Lymphoid hyperplasia: 27 images Xanthoma: 28 images | CED | Crohn’s: sensitivity of 89.32%, specificity of 65.37% Stenosis: sensitivity of 91.27%, specificity of 87.27% Lymphangiectasia: sensitivity of 95.45%, specificity of 94.1% Lymphoid: sensitivity of 87.01%, specificity of 79.71% Xanthoma: sensitivity of 97%, specificity of 97.13% |
Kumar et al.[78] | Inflammatory bowel disease | 2012 | Retrospective | United States of America | Constructing classifier cascade for classifying CD lesions into normal, mild, and severe | Training: 355 images Testing: 212 normal images, 213 mild, 108 severe images | SVM | Sensitivity over 90% was found |
Charisis and Hadjileontiadis[80] | Inflammatory bowel disease | 2016 | Retrospective | Greece | Utilise novel feature extraction method for detecting CD lesions | Database of 466 normal images and 436 CD images | SVM | Sensitivity of 95.2%, specificity of 92.4% |
de Maissin et al.[82] | Inflammatory bowel disease | 2018 | Retrospective | France | Develop CNN for automatic detection of SB CD lesions | Training: 589 images Testing: 73 images | CNN | Sensitivity of 62.18%, specificity of 66.81% |
Klang et al.[83] | Inflammatory bowel disease | 2019 | Retrospective | Israel | Utilise CNN for CD monitoring and diagnosis by SB ulcer detection | Training: 1,090 images Testing: 273 images | CNN | Sensitivity of 96.9%, specificity of 96.6% |
Barash et al.[81] | Inflammatory bowel disease | 2020 | Retrospective | Israel | Automatic severity grading of CD ulcers into grades 1 to 3 | Training: 1,242 images Testing: 248 images | CNN | Sensitivity of 71%, specificity of 34% |
Klang et al.[84] | Inflammatory bowel disease | 2020 | Retrospective | Israel | Construction of CNN to differentiate normal and ulcerated mucosa | Training: 14,112 images Testing: 3,528 images | CNN | Sensitivity of 97.1%, specificity of 96% |
de Maissin et al.[85] | Inflammatory bowel disease | 2021 | Retrospective | France | Assessing importance of annotation quality on CNN | Database of 3,498 images was annotated by different readers for different trials | RANN | Sensitivity of 93%, specificity of 95% |
Klang et al.[86] | Inflammatory bowel disease | 2021 | Retrospective | Israel | Identify intestinal strictures on CE images from CD patients | Database of 1,942 stricture images, 14,266 normal mucosa images, 7,075 mild ulcer images, 2,386 moderate ulcer images, 2,223 severe ulcer images used for training and testing | CNN | Sensitivity of 92%, specificity of 89% |
Klang et al.[87] | Inflammatory bowel disease | 2021 | Retrospective | Israel | Identify NSAID ulcers, which are common differentials for CD ulcers on CE images | Training: 7,391 CD mucosal ulcer images, 10,249 normal mucosa Testing: 980 NSAIDs ulcer images, 625 normal mucosa images | CNN | Sensitivity of 92%, specificity of 95% |
Majtner et al.[88] | Inflammatory bowel disease | 2021 | Retrospective | Denmark | Detection and classifying CD lesions based on severity | Training: 5,419 images Testing: 1,558 images | CNN | Sensitivity of 96.2%, specificity of 100% |
Ferreira et al.[89] | Inflammatory bowel disease | 2022 | Retrospective | Portugal | Automatically detecting ulcers and erosions in the small intestine and colon | Training: 19,740 images Testing: 4,935 images | CNN | Sensitivity of 90%, specificity of 96% |
Higuchi et al.[90] | Inflammatory bowel disease | 2022 | Retrospective | Japan | Classifying ulcerative colitis lesions using MES criteria | Training: 483,644 images Testing: 255,377 images | CNN | No Sensitivity or specificity given. Accuracy of 98.3% on validation |
Kratter et al.[91] | Inflammatory bowel disease | 2022 | Retrospective | Israel | Accurately identify ulcers on capsule endoscopy by combining algorithm viable for two models of capsule endoscope | Database of 15,684 normal mucosa images, 17,416 ulcerated mucosa images used for training and validation | CNN | No Sensitivity or specificity given. Accuracy of 97.4% on validation |
Mascarenhas et al.[92] | Inflammatory bowel disease | 2023 | Retrospective | Portugal | Construct CNN for automatic classification of various types of pleomorphic gastric lesions | Database of 6,844 normal mucosa images, 1,407 protruding lesion images, 994 ulcer and erosion images, 822 vascular lesion images, 2,851 haematic residue images used for training and validation | CNN | Sensitivity of 97.4%, specificity of 95.9% |
Coeliac disease
Currently, there is a comparatively smaller body of research on AI detection and analysis of capsule endoscopy video for coeliac disease. Given the recency of the field, all retrieved articles utilised deep learning in their systems[93-96]. In 2017, Zhou et al. developed a deep learning method using the GoogLeNet model[93]. Impressively, a 100% sensitivity and specificity were found on testing, although only a small number of video clips were used for the study. More recently, in 2021, Li et al. employed principal component analysis (PCA) for feature extraction, including the novel strip PCA (SPCA) method[95]. Using a small database of 460 images, their process was found to have an average accuracy of 93.9% on testing. The small number of studies performed has resulted in a paucity of evidence on the utility of AI tools for this condition [Table 6].
Table of AI applications in capsule endoscopy for coeliac disease
Ref. | Application | Year of publication | Study design | Study location | Aim and goals | Training/Validation dataset | AI type | Results |
Zhou et al.[93] | Coeliac disease | 2017 | Retrospective | China | Develop CNN-based methodology for coeliac disease identification | Training: 6 coeliac disease patient CE videos, 5 control patient CE videos Testing: 5 coeliac disease patient CE videos, 5 control patient CE videos | CNN | Sensitivity of 100%, specificity of 100% |
Wang et al.[94] | Coeliac disease | 2020 | Retrospective | China | Construct novel deep learning recalibration module for the diagnosis of coeliac disease on VCE images | Database of 1,100 normal mucosa images, 1,040 CD mucosa images used for training and testing | CNN SVM KNN LDA | Sensitivity of 97.20%, specificity of 95.63% |
Li et al.[95] | Coeliac disease | 2021 | Retrospective | China | Utilise novel SPCA method for image processing to detect coeliac disease | Training: 184 images Testing: 276 images | KNN SVMCNN | No Sensitivity or specificity given, accuracy of 93.9% |
Chetcuti Zammit et al.[96] | Coeliac disease | 2023 | Retrospective | United Kingdom/ United States of America | Evaluate and compare coeliac disease severity assessment of AI tool and human readers | Training: 444,659 images Testing: 63 VCE videos | MLA | No Sensitivity or specificity given |
Hookworm detection
Among the various pathological conditions that AI diagnostic techniques can identify, research into detecting parasitic infestations such as Hookworms has very little published data available. In 2016, Wu et al. proposed a new method that includes a multi-scale dual matched filter to locate the tubular structure of hookworms and a piecewise parallel region detection method to identify regions potentially containing hookworm bodies on WCE imaging[97]. Testing on a large dataset of 440,000 WCE images demonstrated accuracy, sensitivity, and specificity rates of around 78%. In 2018, He et al. furthered this work by integrating two CNN systems to model the visual appearances and tubular patterns of hookworms concurrently[98]. Testing and validating showcased an impressive accuracy of 88.5%. More recently, in 2021, Gan et al. utilised a deep CNN trained using 11,236 capsule endoscopy images of hookworms[99]. The trained CNN system took 403 s to evaluate 10,529 test images, with sensitivity, specificity, and accuracy of 92.2%, 91.1%, and 91.2%, respectively [Table 7].
Table of AI applications in capsule endoscopy for hookworm detection
Ref. | Application | Year of publication | Study design | Study location | Aim and goals | Training/Validation dataset | AI type | Results |
Wu et al.[97] | Hookworm detection | 2016 | Retrospective | China | Automatically detect hookworm on WCE images | 440,000 images from 11 patients used for training and testing | MLA | Sensitivity of 77.3%, specificity of 77.9% |
He et al.[98] | Hookworm detection | 2018 | Retrospective | China | Utilise deep learning for automatic hookworm detection | 440,000 images from 11 patients used for training and testing | CNN | Sensitivity of 84.6%, specificity of 88.6% |
Gan et al.[99] | Hookworm detection | 2021 | Retrospective | China | Construct CNN for the automatic detection of hookworm on CE images | Training: 11,236 images of hookworm Testing: 531 hookworm images, 9,998 normal images | CNN | Sensitivity of 92.2%, specificity of 91.1% |
Other applications of AI in capsule endoscopy.
Automated calculation of bowel preparation quality
Effective and thorough bowel cleansing is essential for high quality images of the GI tract through capsule endoscopy. The diagnostic potential is reduced when bowel preparation is inadequately performed. Nam et al. created an automated calculation software for small bowel cleansing scores using deep learning algorithms. A five-step scoring system was developed based on mucosal visibility, which was then used to train the deep learning algorithm. The system assigned an average cleansing score (ranging from 1 to 5), which was compared with gradings (A to C) assessed by clinicians. The software was able to provide objective, automated cleansing scores for small bowel preparation, thus potentially allowing its use in the assessment of whether or not appropriate bowel preparation has been achieved for small bowel pathology detection[100] [Table 8].
Table of AI application in capsule endoscopy for bowel prep scoring
Ref. | Application | Year of publication | Study design | Study location | Aim and goals | Training/Validation dataset | AI type | Results |
Nam et al.[100] | Bowel prep scoring | 2021 | Retrospective | Korea | Automatically detect and score bowel prep quality on CE images | Training: 500 images for each score (1-5), totalling 2,500 Testing: 96 CE cases | CNN | Sensitivity of 93%, specificity of 100% At cleansing cut-off value of 3.25 |
Multiple lesion characterisation
A functioning, highly accurate method to detect and characterise a wide range of lesions through the same tool in real time would be the ultimate goal in the foreseeable future for AI research. Various models have so far attempted to achieve this goal[101-107]. Recently, in 2023, Yokote et al. constructed an object detection AI model from a dataset of 18,481 images to detect and characterise into the categories of Angiodysplasia, Erosion, Stenosis, Lymphangiectasis, Lymph follicle, Submucosal tumour, Polyp‐like, Bleeding, Diverticula, Redness, Foreign body, and Venous. The overall sensitivity was 91%[106].
Also, in 2023, Ding et al. developed an AI model to detect various abnormalities on capsule endoscopy imaging, trained on 280,426 images. The AI model showed high sensitivity in detecting various abnormalities: red spots (97.8%), inflammation (96.1%), blood content (96.1%), vascular lesions (94.7%), protruding lesions (95.6%), parasites (100%), diverticulum (100%), and normal variants (96.4%). Furthermore, when junior doctors used the AI model, their overall accuracy increased from 85.5% to 97.9% and became comparable to that of experts who had an accuracy rate of 96.6%[107]. AI tools, which are multi-faceted and have the ability to detect and characterise a variety of common findings, will no doubt revolutionise capsule endoscopy diagnosis [Table 9].
Table of AI applications in capsule endoscopy for multiple lesion detection
Ref. | Application | Year of publication | Study design | Study location | Aim and goals | Training/Validation dataset | AI type | Results |
Park et al.[101] | Multiple lesion detection | 2020 | Retrospective | Korea | Develop CNN model to identify multiple lesions on CE and classify images based on significance | Training: 60,000 significant, 60,000 insignificant Testing: 20 CE videos | CNN | No sensitivity or specificity given; overall detection rate of 81.6% |
Xing et al.[102] | Multiple lesion detection | 2020 | Retrospective | China | Develop AGDN model for WCE image classification | CAD-CAP[54] and KID[110] databases used for training and testing | CNN | Sensitivity of 95.72% for normal, 90.7% for vascular images, 87.44% for inflammatory images |
Zhu et al.[103] | Multiple lesion detection | 2021 | Retrospective | China | Construct new deep learning model for classification and segmentation of WCE images | CAD-CAP[54] and KID[110] databases used for training and testing | Deep neural network | Sensitivity of 97% for normal, 94.17% for vascular images, 92.71% for inflammatory images |
Guo et al.[104] | Multiple lesion detection | 2021 | Retrospective | China | Utilise CNN models for the automatic detection of vascular and inflammatory lesions | Training: 1,440 images Testing: 360 images | CNN | Sensitivity of 96.67% for vascular lesions, sensitivity of 93.33% for inflammatory lesions |
Goel et al.[105] | Multiple lesion detection | 2022 | Retrospective | India | Develop CNN framework to test importance of colour features for lesion detection | Trained and tested on collected 7,259 normal images and 1,683 abnormal images Also trained and tested on KID[110] database | CNN | Sensitivity of 98.06% on collected database, sensitivity of 97% on KID |
Yokote et al.[106] | Multiple lesion detection | 2023 | Retrospective | Japan | Construction of objection detection AI model for classification of 12 types of lesions from CE images | Training: 17,085 images Testing: 1,396 images | CNN | Sensitivity of 91% |
Ding et al.[107] | Multiple lesion detection | 2023 | Retrospective | China | Development of AI tool to detect multiple lesion types on CE | Training: 280,426 images Testing: 240 videos | CNN | Median sensitivity of 96.25%, median specificity of 83.65% |
DISCUSSION
The shifting of utilised AI types over time from traditional machine learning features such as SVMs to deep learning, including CNNs, is associated with an increase in accuracy, sensitivity and specificity of diagnostic results.
Current commercial endoscopes have some algorithm built to assist with interpretation. However, the training of such algorithms are based on traditional supervised learning methods. Given the rise in higher resolution and increase the amount of training images and videos, unsupervised methods will be more efficient and accurate.
Deep learning has shown significant promise in the field of diagnostic capsule endoscopy due to its ability to learn from large volumes of data and make accurate predictions. Current commercial capsule endoscopes have algorithms available to assist with interpretation such as the TOP 100 feature of Rapid Reader[45]. However, the training of these algorithms is based on traditional supervised learning methods. Unlike traditional machine learning algorithms, which require manual feature extraction and selection, deep learning ones can automatically learn and extract features from raw data[108]. CNNs, in particular, are designed to automatically and adaptively learn spatial hierarchies of features from raw data, which makes them well-suited for image classification tasks in capsule endoscopy, as evidenced in the studies above. Given the rise in image resolution and amount of training images and video, unsupervised methods capitalising on these AI systems will become even more efficient and accurate in future.
Despite the advantages of deep learning, it is not without its pitfalls. One of its main criticisms is the “black box” problem. Due to the complexity and depth of these models, it can be challenging to understand and interpret how they make their predictions. This lack of transparency and interpretability can be problematic in medical applications, where understanding the reasoning behind a diagnosis is crucial for patient care and trust[109]. The “black box” problem also raises concerns about the reliability and fairness of deep learning models. If the reasoning behind a model’s prediction is not clear, determining whether the model is making decisions based on relevant features or whether it is being influenced by irrelevant or biased data can be difficult[109]. This is an intrinsic issue with deep learning, and hence, images must be validated prospectively prior to usage in clinical settings. Currently, AI researchers are exploring a concept known as Explainable AI to help understand the logic and decision-making process within a black box.
When training WCE with AI, “images” obtained may not be histologically verified due to an inability to obtain biopsies without invasive enteroscopy. This issue undoubtedly has implications for the reliability of the AI algorithms due to the potential inaccuracy of the training dataset used. This may adversely affect the diagnostic accuracy, causing either false-positive or false-negative results, both of which have significant clinical implications. The issue of data quality can be mitigated by ensuring that the AI models are trained on high-quality, histologically proven images, such as the French-created CAD-CAP. This could involve collaborations with medical institutions and experts to curate and verify the training datasets.
The current AI models used in capsule endoscopy also do not appear to harness the potential of vision transformers (ViTs), a state-of-the-art AI model adapted from natural language processing, which utilises self-attention methods for training. ViTs offer a far superior capacity for data handling compared to other deep learning models, with approximately four times as much capacity as that of traditional CNNs. Moreover, their ability to combine spatial analysis with temporal analysis allows them to demonstrate a much superior performance in image-based tasks. Their employment in capsule endoscopy could open the door to more precise lesion characterisation, thereby enhancing the diagnostic potential of this technology. The lack of current models using ViTs presents a notable gap in the field. However, this is primarily due to the recency of the technology in the medical imaging world. The use of ViT in endoscopy has only been explored very recently in research settings, and more applications are expected in the near future.
However, the potential of AI-assisted capsule endoscopy, particularly for colonoscopy for polyp detection and characterisation, is notable. While capsule endoscopy is quite costly compared to the Faecal Occult Blood Test (FOBT), it could serve as an alternative for patients where FOBT may yield high false positives such as in those with haemorrhoids or who do not wish to partake in FOBT-based screening programs. Furthermore, the non-invasiveness and cost-effectiveness of AI Capsule colonoscopy offer advantages over traditional procedures, making it a promising option for mass screening in the near future. It is expected that AI tools will replace parts of the endoscopy procedure after undergoing further clinical evaluation, especially with examples such as AnX Robotica’s ProScan receiving FDA approval in 2024.
While AI shows high overall accuracy across many studies, it is important to note that overall accuracy alone does not paint a comprehensive picture of model performance in medical applications. For diagnostic models, maintaining a low rate of false negatives is crucial to ensure no diagnoses are missed. While false positives may cause unnecessary worry and additional testing, false negatives can lead to delayed treatment with potentially severe consequences. Additionally, the current body of research is primarily conducted retrospectively, introducing the risk of investigator bias. Hence, future prospective multicentre research on this topic is required.
CONCLUSION AND FUTURE DIRECTIONS
This narrative review provides a comprehensive synthesis on the literature relating to AI in WCE. While integrating AI into capsule endoscopy shows immense promise in reading time reduction and accuracy improvement, there is a potential possibility that the system could independently read images in the future. This path, though, must be navigated carefully, bearing in mind the unique challenges associated with medical data and the specific requirements of diagnostic models. The potential of ViTs is yet to be fully exploited in this field. We anticipate an exciting progression in the coming years as more refined and accurate models are developed.
DECLARATIONS
Authors’ contributions
Study conception and design: Singh R
Data collection: George AA, Tan JL, Kovoor JG, Singh R
Analysis and interpretation of results: George AA, Tan JL, Kovoor JG, George B, Lee A, Stretton B, Gupta AK, Bacchi S, Singh R
Draft manuscript preparation: George AA, Tan JL, Kovoor JG, Singh R
Availability of data and materials
Not applicable.
Financial support and sponsorship
None.
Conflicts of interest
All authors declared that there are no conflicts of interest.
Ethical approval and consent to participate
Not applicable.
Consent for publication
Not applicable.
Copyright
© The Author(s) 2024.
REFERENCES
2. Beg S, Card T, Sidhu R, Wronska E, Ragunath K; UK capsule endoscopy users’ group. The impact of reader fatigue on the accuracy of capsule endoscopy interpretation. Dig Liver Dis 2021;53:1028-33.
3. Giritharan B, Yuan X, Liu J, Buckles B, Oh JH, Tang SJ. Bleeding detection from capsule endoscopy videos. Annu Int Conf IEEE Eng Med Biol Soc 2008;2008:4780-3.
4. Pan G, Yan G, Song X, Qiu X. BP neural network classification for bleeding detection in wireless capsule endoscopy. J Med Eng Technol 2009;33:575-81.
5. Ghosh T, Fattah SA, Shahnaz C, Wahid KA. An automatic bleeding detection scheme in wireless capsule endoscopy based on histogram of an RGB-indexed image. Annu Int Conf IEEE Eng Med Biol Soc 2014;2014:4683-6.
6. Hassan AR, Haque MA. Computer-aided gastrointestinal hemorrhage detection in wireless capsule endoscopy videos. Comput Methods Programs Biomed 2015;122:341-53.
7. Pan G, Yan G, Qiu X, Cui J. Bleeding detection in wireless capsule endoscopy based on probabilistic neural network. J Med Syst 2011;35:1477-84.
8. Li B, Meng MQH. Computer-aided detection of bleeding regions for capsule endoscopy images. IEEE Trans Biomed Eng 2009;56:1032-9.
9. Yuan Y, Li B, Meng MQH. Bleeding frame and region detection in the wireless capsule endoscopy video. IEEE J Biomed Health Inform 2016;20:624-30.
10. Ghosh T, Fattah SA, Wahid KA, Zhu WP, Ahmad MO. Cluster based statistical feature extraction method for automatic bleeding detection in wireless capsule endoscopy video. Comput Biol Med 2018;94:41-54.
11. Pogorelov K, Suman S, Azmadi Hussin F, et al. Bleeding detection in wireless capsule endoscopy videos - Color versus texture features. J Appl Clin Med Phys 2019;20:141-54.
12. Rathnamala S, Jenicka S. Automated bleeding detection in wireless capsule endoscopy images based on color feature extraction from Gaussian mixture model superpixels. Med Biol Eng Comput 2021;59:969-87.
13. Jia X, Meng MQH. A deep convolutional neural network for bleeding detection in wireless capsule endoscopy images. Annu Int Conf IEEE Eng Med Biol Soc 2016;2016:639-42.
14. Jia X, Meng MQH. Gastrointestinal bleeding detection in wireless capsule endoscopy images using handcrafted and CNN features. Annu Int Conf IEEE Eng Med Biol Soc 2017;2017:3154-7.
15. Hajabdollahi M, Esfandiarpoor R, Najarian K, Karimi N, Samavi S, Reza Soroushmehr SM. Low complexity CNN structure for automatic bleeding zone detection in wireless capsule endoscopy imaging. Annu Int Conf IEEE Eng Med Biol Soc 2019;2019:7227-30.
16. Kanakatte A, Ghose A. Precise bleeding and red lesions localization from capsule endoscopy using compact U-net. Annu Int Conf IEEE Eng Med Biol Soc 2021;2021:3089-92.
17. Ghosh T, Chakareski J. Deep transfer learning for automated intestinal bleeding detection in capsule endoscopy imaging. J Digit Imaging 2021;34:404-17.
18. Ribeiro T, Saraiva MM, Ferreira JPS, et al. Artificial intelligence and capsule endoscopy: automatic detection of vascular lesions using a convolutional neural network. Ann Gastroenterol 2021;34:820-8.
19. Mascarenhas Saraiva M, Ribeiro T, Afonso J, et al. Artificial intelligence and capsule endoscopy: automatic detection of small bowel blood content using a convolutional neural network. GE Port J Gastroenterol 2022;29:331-8.
20. Muruganantham P, Balakrishnan SM. Attention aware deep learning model for wireless capsule endoscopy lesion classification and localization. J Med Biol Eng 2022;42:157-68.
21. Kundu AK, Fattah SA, Rizve MN. An automatic bleeding frame and region detection scheme for wireless capsule endoscopy videos based on interplane intensity variation profile in normalized RGB color space. J Healthc Eng 2018;2018:9423062.
22. Xing X, Jia X, Meng MQH. Bleeding detection in wireless capsule endoscopy image video using superpixel-color histogram and a subspace KNN classifier. Annu Int Conf IEEE Eng Med Biol Soc 2018;2018:1-4.
23. Charisis V, Hadjileontiadis LJ, Liatsos CN, Mavrogiannis CC, Sergiadis GD. Abnormal pattern detection in wireless capsule endoscopy images using nonlinear analysis in RGB color space. In: 2010 Annual International Conference of the IEEE Engineering in Medicine and Biology; 2010 Aug 31 - Sep 04; Buenos Aires, Argentina. IEEE; 2010. pp. 3674-7.
24. Li B, Meng MQH. Computer-based detection of bleeding and ulcer in wireless capsule endoscopy images by chromaticity moments. Comput Biol Med 2009;39:141-7.
25. Charisis VS, Hadjileontiadis LJ, Liatsos CN, Mavrogiannis CC, Sergiadis GD. Capsule endoscopy image analysis using texture information from various colour models. Comput Methods Programs Biomed 2012;107:61-74.
26. Iakovidis DK, Koulaouzidis A. Automatic lesion detection in capsule endoscopy based on color saliency: closer to an essential adjunct for reviewing software. Gastrointest Endosc 2014;80:877-83.
27. Fan S, Xu L, Fan Y, Wei K, Li L. Computer-aided detection of small intestinal ulcer and erosion in wireless capsule endoscopy images. Phys Med Biol 2018;63:165001.
28. Khan MA, Sharif M, Akram T, Yasmin M, Nayak RS. Stomach deformities recognition using rank-based deep features selection. J Med Syst 2019;43:329.
29. Kundu AK, Fattah SA, Wahid KA. Multiple linear discriminant models for extracting salient characteristic patterns in capsule endoscopy images for multi-disease detection. IEEE J Transl Eng Health Med 2020;8:3300111.
30. Wang S, Xing Y, Zhang L, Gao H, Zhang H. A systematic evaluation and optimization of automatic detection of ulcers in wireless capsule endoscopy on a large dataset using deep convolutional neural networks. Phys Med Biol 2019;64:235014.
31. Aoki T, Yamada A, Aoyama K, et al. Automatic detection of erosions and ulcerations in wireless capsule endoscopy images based on a deep convolutional neural network. Gastrointest Endosc 2019;89:357-63.e2.
32. Ding Z, Shi H, Zhang H, et al. Gastroenterologist-level identification of small-bowel diseases and normal variants by capsule endoscopy using a deep-learning model. Gastroenterology 2019;157:1044-54.e5.
33. Majid A, Khan MA, Yasmin M, Rehman A, Yousafzai A, Tariq U. Classification of stomach infections: a paradigm of convolutional neural network along with classical features fusion and selection. Microsc Res Tech 2020;83:562-76.
34. Otani K, Nakada A, Kurose Y, et al. Automatic detection of different types of small-bowel lesions on capsule endoscopy images using a newly developed deep convolutional neural network. Endoscopy 2020;52:786-91.
35. Xia J, Xia T, Pan J, et al. Use of artificial intelligence for detection of gastric lesions by magnetically controlled capsule endoscopy. Gastrointest Endosc 2021;93:133-9.e4.
36. Afonso J, Saraiva MJM, Ferreira JPS, et al. Development of a convolutional neural network for detection of erosions and ulcers with distinct bleeding potential in capsule endoscopy. Tech Innov Gastrointest Endosc 2021;23:291-6.
37. Mascarenhas Saraiva MJ, Afonso J, Ribeiro T, et al. Deep learning and capsule endoscopy: automatic identification and differentiation of small bowel lesions with distinct haemorrhagic potential using a convolutional neural network. BMJ Open Gastroenterol 2021;8:e000753.
38. Afonso J, Saraiva MM, Ferreira JPS, et al. Automated detection of ulcers and erosions in capsule endoscopy images using a convolutional neural network. Med Biol Eng Comput 2022;60:719-25.
39. Mascarenhas M, Ribeiro T, Afonso J, et al. Deep learning and colon capsule endoscopy: automatic detection of blood and colonic mucosal lesions using a convolutional neural network. Endosc Int Open 2022;10:E171-7.
40. Xiao P, Pan Y, Cai F, et al. A deep learning based framework for the classification of multi- class capsule gastroscope image in gastroenterologic diagnosis. Front Physiol 2022;13:1060591.
41. Ribeiro T, Mascarenhas M, Afonso J, et al. Artificial intelligence and colon capsule endoscopy: automatic detection of ulcers and erosions using a convolutional neural network. J Gastroenterol Hepatol 2022;37:2282-8.
42. Raut V, Gunjan R, Shete VV, Eknath UD. Gastrointestinal tract disease segmentation and classification in wireless capsule endoscopy using intelligent deep learning model. Comput Method Biomech Biomed Eng Imaging Vis 2023;11:606-22.
43. Nakada A, Niikura R, Otani K, et al. Improved object detection artificial intelligence using the revised RetinaNet model for the automatic detection of ulcerations, vascular lesions, and tumors in wireless capsule endoscopy. Biomedicines 2023;11:942.
44. Gan T, Wu JC, Rao NN, Chen T, Liu B. A feasibility trial of computer-aided diagnosis for enteric lesions in capsule endoscopy. World J Gastroenterol 2008;14:6929-35.
45. Arieira C, Monteiro S, de Castro FD, et al. Capsule endoscopy: is the software TOP 100 a reliable tool in suspected small bowel bleeding? Dig Liver Dis 2019;51:1661-4.
46. Vieira PM, Silva CP, Costa D, Vaz IF, Rolanda C, Lima CS. Automatic segmentation and detection of small bowel angioectasias in WCE images. Ann Biomed Eng 2019;47:1446-62.
47. Vezakis IA, Toumpaniaris P, Polydorou AA, Koutsouris D. A novel real-time automatic angioectasia detection method in wireless capsule endoscopy video feed. Annu Int Conf IEEE Eng Med Biol Soc 2019;2019:4072-5.
48. Leenhardt R, Vasseur P, Li C, et al; The CAD-CAP Database Working Group. A neural network algorithm for detection of GI angiectasia during small-bowel capsule endoscopy. Gastrointest Endosc 2019;89:189-94.
49. Tsuboi A, Oka S, Aoyama K, et al. Artificial intelligence using a convolutional neural network for automatic detection of small-bowel angioectasia in capsule endoscopy images. Dig Endosc 2020;32:382-90.
50. Aoki T, Yamada A, Kato Y, et al. Automatic detection of various abnormalities in capsule endoscopy videos by a deep learning-based system: a multicenter study. Gastrointest Endosc 2021;93:165-73.e1.
51. Hwang Y, Lee HH, Park C, et al. Improved classification and localization approach to small bowel capsule endoscopy using convolutional neural network. Dig Endosc 2021;33:598-607.
52. Hosoe N, Horie T, Tojo A, et al. Development of a deep-learning algorithm for small bowel-lesion detection and a study of the improvement in the false-positive rate. J Clin Med 2022;11:3682.
53. Chu Y, Huang F, Gao M, et al. Convolutional neural network-based segmentation network applied to image recognition of angiodysplasias lesion under capsule endoscopy. World J Gastroenterol 2023;29:879-89.
54. Leenhardt R, Vasseur P, Li Cynthia, et al. 403 A highly sensitive and highly specific convolutional neural network-based algorithm for automated diagnosis of angiodysplasia in small bowel capsule endoscopy. Gastrointest Endosc 2018;87:AB78.
55. Li B, Meng MQH, Lau JYW. Computer-aided small bowel tumor detection for capsule endoscopy. Artif Intell Med 2011;52:11-6.
56. Karargyris A, Bourbakis N. Detection of small bowel polyps and ulcers in wireless capsule endoscopy videos. IEEE Trans Biomed Eng 2011;58:2777-86.
57. Barbosa DC, Roupar DB, Ramos JC, Tavares AC, Lima CS. Automatic small bowel tumor diagnosis by using multi-scale wavelet-based analysis in wireless capsule endoscopy images. Biomed Eng Online 2012;11:3.
58. Mamonov AV, Figueiredo IN, Figueiredo PN, Tsai YHR. Automated polyp detection in colon capsule endoscopy. IEEE Trans Med Imaging 2014;33:1488-502.
59. Liu G, Yan G, Kuang S, Wang Y. Detection of small bowel tumor based on multi-scale curvelet analysis and fractal technology in capsule endoscopy. Comput Biol Med 2016;70:131-8.
60. Yang J, Chang L, Li S, He X, Zhu T. WCE polyp detection based on novel feature descriptor with normalized variance locality-constrained linear coding. Int J Comput Assist Radiol Surg 2020;15:1291-302.
61. Vieira PM, Freitas NR, Valente J, Vaz IF, Rolanda C, Lima CS. Automatic detection of small bowel tumors in wireless capsule endoscopy images using ensemble learning. Med Phys 2020;47:52-63.
62. Yuan Y, Meng MQH. Deep learning for polyp recognition in wireless capsule endoscopy images. Med Phys 2017;44:1379-89.
63. Saito H, Aoki T, Aoyama K, et al. Automatic detection and classification of protruding lesions in wireless capsule endoscopy images based on a deep convolutional neural network. Gastrointest Endosc 2020;92:144-51.e1.
64. Yamada A, Niikura R, Otani K, Aoki T, Koike K. Automatic detection of colorectal neoplasia in wireless colon capsule endoscopic images using a deep convolutional neural network. Endoscopy 2021;53:832-6.
65. Saraiva MM, Ferreira JPS, Cardoso H, et al. Artificial intelligence and colon capsule endoscopy: development of an automated diagnostic system of protruding lesions in colon capsule endoscopy. Tech Coloproctol 2021;25:1243-8.
66. Jain S, Seal A, Ojha A, et al. A deep CNN model for anomaly detection and localization in wireless capsule endoscopy images. Comput Biol Med 2021;137:104789.
67. Zhou JX, Yang Z, Xi DH, et al. Enhanced segmentation of gastrointestinal polyps from capsule endoscopy images with artifacts using ensemble learning. World J Gastroenterol 2022;28:5931-43.
68. Mascarenhas M, Afonso J, Ribeiro T, et al. Performance of a deep learning system for automatic diagnosis of protruding lesions in colon capsule endoscopy. Diagnostics 2022;12:1445.
69. Gilabert P, Vitrià J, Laiz P, et al. Artificial intelligence to improve polyp detection and screening time in colon capsule endoscopy. Front Med 2022;9:1000726.
70. Liu F, Hua Z, Li J, Fan L. DBMF: dual branch multiscale feature fusion network for polyp segmentation. Comput Biol Med 2022;151:106304.
71. Souaidi M, Lafraxo S, Kerkaou Z, El Ansari M, Koutti L. A multiscale polyp detection approach for GI tract images based on improved DenseNet and single-shot multibox detector. Diagnostics 2023;13:733.
72. Mascarenhas Saraiva M, Afonso J, Ribeiro T, et al. Artificial intelligence and capsule endoscopy: automatic detection of enteric protruding lesions using a convolutional neural network. Rev Esp Enferm Dig 2023;115:75-9.
73. Lafraxo S, Souaidi M, El Ansari M, Koutti L. Semantic segmentation of digestive abnormalities from WCE images by using AttResU-Net architecture. Life 2023;13:719.
74. Blanes-Vidal V, Baatrup G, Nadimi ES. Addressing priority challenges in the detection and assessment of colorectal polyps from capsule endoscopy and colonoscopy in colorectal cancer screening using machine learning. Acta Oncol 2019;58:S29-36.
75. Piccirelli S, Mussetto A, Bellumat A, et al. New generation express view: an artificial intelligence software effectively reduces capsule endoscopy reading times. Diagnostics 2022;12:1783.
76. Eluxeo meets artificial intelligence. Available from: https://asset.fujifilm.com/www/uk/files/2021-05/8fbe51b9718df4e16e3e3a545fa5593a/ELUXEO_CADEYE_Brochure.pdf. [Last accessed on 11 Mar 2024].
77. Lei II, Tompkins K, White E, et al. Study of capsule endoscopy delivery at scale through enhanced artificial intelligence-enabled analysis (the CESCAIL study). Colorectal Dis 2023;25:1498-505.
78. Kumar R, Zhao Q, Seshamani S, Mullin G, Hager G, Dassopoulos T. Assessment of Crohn’s disease lesions in wireless capsule endoscopy images. IEEE Trans Biomed Eng 2012;59:355-62.
79. Haji-Maghsoudi O, Talebpour A, Soltanian-Zadeh H, Haji-Maghsoodi N. Segmentation of Crohn, lymphangiectasia, xanthoma, lymphoid hyperplasia and stenosis diseases in WCE. Stud Health Technol Inform 2012;180:143-7.
80. Charisis VS, Hadjileontiadis LJ. Potential of hybrid adaptive filtering in inflammatory lesion detection from capsule endoscopy images. World J Gastroenterol 2016;22:8641-57.
81. Barash Y, Azaria L, Soffer S, et al. Ulcer severity grading in video capsule images of patients with Crohn’s disease: an ordinal neural network solution. Gastrointest Endosc 2021;93:187-92.
82. de Maissin A, Gomez T, Le Berre C, et al. P161 Computer aided detection of Crohn’s disease small bowel lesions in wireless capsule endoscopy. J Crohns Colitis 2018;12:S178-9.
83. Klang E, Barash Y, Margalit R, et al. P285 Deep learning for automated detection of mucosal inflammation by capsule endoscopy in Crohn’s disease. J Crohns Colitis 2019;13:S242.
84. Klang E, Barash Y, Margalit RY, et al. Deep learning algorithms for automated detection of Crohn’s disease ulcers by video capsule endoscopy. Gastrointest Endosc 2020;91:606-13.e2.
85. de Maissin A, Vallée R, Flamant M, et al. Multi-expert annotation of Crohn’s disease images of the small bowel for automatic detection using a convolutional recurrent attention neural network. Endosc Int Open 2021;9:E1136-44.
86. Klang E, Grinman A, Soffer S, et al. Automated detection of Crohn’s disease intestinal strictures on capsule endoscopy images using deep neural networks. J Crohns Colitis 2021;15:749-56.
87. Klang E, Kopylov U, Mortensen B, et al. A convolutional neural network deep learning model trained on CD ulcers images accurately identifies NSAID ulcers. Front Med 2021;8:656493.
88. Majtner T, Brodersen JB, Herp J, Kjeldsen J, Halling ML, Jensen MD. A deep learning framework for autonomous detection and classification of Crohn’s disease lesions in the small bowel and colon with capsule endoscopy. Endosc Int Open 2021;9:E1361-70.
89. Ferreira JPS, de Mascarenhas Saraiva MJQEC, Afonso JPL, et al. Identification of ulcers and erosions by the novel pillcam™ Crohn’s capsule using a convolutional neural network: a multicentre pilot study. J Crohns Colitis 2022;16:169-72.
90. Higuchi N, Hiraga H, Sasaki Y, et al. Automated evaluation of colon capsule endoscopic severity of ulcerative colitis using ResNet50. PLoS One 2022;17:e0269728.
91. Kratter T, Shapira N, Lev Y, et al. Deep learning multi-domain model provides accurate detection and grading of mucosal ulcers in different capsule endoscopy types. Diagnostics 2022;12:2490.
92. Mascarenhas M, Mendes F, Ribeiro T, et al. Deep learning and minimally invasive endoscopy: automatic classification of pleomorphic gastric lesions in capsule endoscopy. Clin Transl Gastroenterol 2023;14:e00609.
93. Zhou T, Han G, Li BN, et al. Quantitative analysis of patients with celiac disease by video capsule endoscopy: a deep learning method. Comput Biol Med 2017;85:1-6.
94. Wang X, Qian H, Ciaccio EJ, et al. Celiac disease diagnosis from videocapsule endoscopy images with residual learning and deep feature extraction. Comput Methods Programs Biomed 2020;187:105236.
95. Li BN, Wang X, Wang R, et al. Celiac disease detection from videocapsule endoscopy images using strip principal component analysis. IEEE/ACM Trans Comput Biol Bioinform 2021;18:1396-404.
96. Chetcuti Zammit S, McAlindon ME, Greenblatt E, et al. Quantification of celiac disease severity using video capsule endoscopy: a comparison of human experts and machine learning algorithms. Curr Med Imaging 2023;19:1455-662.
97. Wu X, Chen H, Gan T, Chen J, Ngo CW, Peng Q. Automatic hookworm detection in wireless capsule endoscopy images. IEEE Trans Med Imaging 2016;35:1741-52.
98. He JY, Wu X, Jiang YG, Peng Q, Jain R. Hookworm detection in wireless capsule endoscopy images with deep learning. IEEE Trans Image Process 2018;27:2379-92.
99. Gan T, Yang Y, Liu S, et al. Automatic detection of small intestinal hookworms in capsule endoscopy images based on a convolutional neural network. Gastroenterol Res Pract 2021;2021:5682288.
100. Nam JH, Hwang Y, Oh DJ, et al. Development of a deep learning-based software for calculating cleansing score in small bowel capsule endoscopy. Sci Rep 2021;11:4417.
101. Park J, Hwang Y, Nam JH, et al. Artificial intelligence that determines the clinical significance of capsule endoscopy images can increase the efficiency of reading. PLoS One 2020;15:e0241474.
102. Xing X, Yuan Y, Meng MQH. Zoom in lesions for better diagnosis: attention guided deformation network for WCE image classification. IEEE Trans Med Imaging 2020;39:4047-59.
103. Zhu M, Chen Z, Yuan Y. DSI-Net: deep synergistic interaction network for joint classification and segmentation with endoscope images. IEEE Trans Med Imaging 2021;40:3315-25.
104. Guo X, Zhang L, Hao Y, Zhang L, Liu Z, Liu J. Multiple abnormality classification in wireless capsule endoscopy images based on EfficientNet using attention mechanism. Rev Sci Instrum 2021;92:094102.
105. Goel N, Kaur S, Gunjan D, Mahapatra SJ. Investigating the significance of color space for abnormality detection in wireless capsule endoscopy images. Biomed Signal Proces 2022;75:103624.
106. Yokote A, Umeno J, Kawasaki K, et al. Small bowel capsule endoscopy examination and open access database with artificial intelligence: the SEE-artificial intelligence project. DEN Open 2024;4:e258.
107. Ding Z, Shi H, Zhang H, et al. Artificial intelligence-based diagnosis of abnormalities in small-bowel capsule endoscopy. Endoscopy 2023;55:44-51.
109. Rudin C. Stop explaining black box machine learning models for high stakes decisions and use interpretable models instead. Nat Mach Intell 2019;1:206-15.
110. Koulaouzidis A, Iakovidis DK, Yung DE, et al. KID project: an internet-based digital video atlas of capsule endoscopy for research purposes. Endosc Int Open 2017;5:E477-83.
111. Deeba F, Islam M, Bui FM, Wahid KA. Performance assessment of a bleeding detection algorithm for endoscopic video based on classifier fusion method and exhaustive feature selection. Biomed Signal Proces 2018;40:415-24.
112. Smedsrud PH, Thambawita V, Hicks SA, et al. Kvasir-capsule, a video capsule endoscopy dataset. Sci Data 2021;8:142.
113. Bernal J, Sánchez FJ, Fernández-Esparrach G, Gil D, Rodríguez C, Vilariño F. WM-DOVA maps for accurate polyp highlighting in colonoscopy: validation vs. saliency maps from physicians. Comput Med Imaging Graph 2015;43:99-111.
114. Coelho P, Pereira A, Leite A, Salgado M, Cunha A. A deep learning approach for red lesions detection in video capsule endoscopies. In: Campilho A, Karray F, ter Haar Romeny B, editors. ICIAR 2018: Image analysis and recognition. Springer, Cham; 2018. pp. 553-61.
115. Jha D, Smedsrud PH, Riegler MA, et al. Kvasir-SEG: a segmented polyp dataset. In: MMM 2020: MultiMedia modeling. Springer, Cham; 2020. pp. 451-62.
Cite This Article
Export citation file: BibTeX | EndNote | RIS
OAE Style
George AA, Tan JL, Kovoor JG, Lee A, Stretton B, Gupta AK, Bacchi S, George B, Singh R. Artificial intelligence in capsule endoscopy: development status and future expectations. Mini-invasive Surg 2024;8:4. http://dx.doi.org/10.20517/2574-1225.2023.102
AMA Style
George AA, Tan JL, Kovoor JG, Lee A, Stretton B, Gupta AK, Bacchi S, George B, Singh R. Artificial intelligence in capsule endoscopy: development status and future expectations. Mini-invasive Surgery. 2024; 8: 4. http://dx.doi.org/10.20517/2574-1225.2023.102
Chicago/Turabian Style
Ashwin A. George, Jin Lin Tan, Joshua G. Kovoor, Alvin Lee, Brandon Stretton, Aashray K. Gupta, Stephen Bacchi, Biju George, Rajvinder Singh. 2024. "Artificial intelligence in capsule endoscopy: development status and future expectations" Mini-invasive Surgery. 8: 4. http://dx.doi.org/10.20517/2574-1225.2023.102
ACS Style
George, AA.; Tan JL.; Kovoor JG.; Lee A.; Stretton B.; Gupta AK.; Bacchi S.; George B.; Singh R. Artificial intelligence in capsule endoscopy: development status and future expectations. Mini-invasive. Surg. 2024, 8, 4. http://dx.doi.org/10.20517/2574-1225.2023.102
About This Article
Special Issue
Copyright
Data & Comments
Data
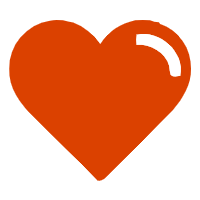
Comments
Comments must be written in English. Spam, offensive content, impersonation, and private information will not be permitted. If any comment is reported and identified as inappropriate content by OAE staff, the comment will be removed without notice. If you have any queries or need any help, please contact us at support@oaepublish.com.