Expression characteristics, immune signatures, and prognostic value of the KIF family members identified across cancers
Abstract
Aim: The overexpression of Kinesin superfamily proteins (KIFs) has been increasingly recognized as a critical factor associated with unfavorable prognostic outcomes across a spectrum of cancers. This study aims to elucidate the multifaceted role of KIFs within the tumor immune microenvironment and explore their potential as targets for precision cancer therapy.
Methods: Utilizing comprehensive genomic datasets from the Cancer Genome Atlas and Genotype-Tissue Expression databases, we systematically analyzed KIF expression patterns and their potential oncogenic functions. To investigate the functional impact of KIF3A in hepatocellular carcinoma (HCC), we synthesized siKIF3A and transfected it into HepG2 cells, followed by a series of functional assays. Cell proliferation was meticulously evaluated using EdU incorporation, CCK8, and colony formation assays, while cell migration was assessed through scratch wound healing and Transwell migration assays. Quantitative analysis of gene and protein expression levels was performed using RT-PCR and Western blot techniques, respectively.
Results: Our findings reveal that KIFs exhibit remarkably high mutation frequencies across multiple cancer types. Furthermore, we identified significant genomic and epigenetic modifications of KIFs in various tumors, with specific oncogenic mutations in certain cancers potentially serving as regulatory mechanisms for KIFs expression. Notably, tumor-derived KIFs demonstrate a strong association with immune cell infiltration patterns, suggesting their potential as novel therapeutic targets in cancer immunotherapy. Importantly, the majority of KIF family genes show significant correlations with patient prognosis, underscoring their clinical relevance. Specifically, KIF3A emerges as a promising prognostic marker for HCC, demonstrating significantly higher expression levels in HCC tissues compared to adjacent non-cancerous tissues (P < 0.05). This overexpression strongly correlates with poor survival outcomes and established risk factors. Functional studies reveal that knockdown of KIF3A significantly inhibits the proliferation and migration capabilities of HCC cells (P < 0.05), highlighting its critical role in tumor progression. Our findings suggest that KIF3A not only serves as a valuable prognostic biomarker but also represents a potential therapeutic target for HCC patients, particularly through its involvement in tumor immune regulation mechanisms.
Conclusion: This comprehensive study provides novel insights into the role of KIFs, particularly KIF3A, in cancer biology and offers promising avenues for the development of targeted therapies in hepatocellular carcinoma. The integration of genomic analysis with functional validation underscores the potential of KIFs as both diagnostic markers and therapeutic targets in cancer management.
Keywords
INTRODUCTION
Over the past few decades, conventional cancer treatments such as surgical removal[1], radiation therapy[2], and chemotherapy[3] have been employed. While these methods have shown success in certain cases, they also come with limitations and side effects[4]. In contrast, tumor immunotherapy has drawn extensive attention due to its unique mechanism of action and significant efficacy[5-7]. They utilize specific immune cells, such as T cells and natural killer cells, to enhance their ability to target tumor cells[8-10]. Despite the immense potential of tumor immunotherapy in the field of cancer, it still faces challenges and limitations. These include immunological tolerance, uncertain treatment response rates, and individual variations[11]. Therefore, further research and the development of new biomarkers and therapeutic targets are crucial for advancing tumor treatments.
The kinesin superfamily is a group of motor proteins that play critical roles in intracellular transport, mitosis, and other cellular processes. Dysregulation of Kinesins has been linked to various aspects of tumor development and progression. Kinesin family member 2C[12] (KIF2C) has been shown to promote cancer cell migration and invasion in breast cancer[13]. Other kinesins, such as KIF2A, KIF15, and KIF4A, have also been associated with increased invasiveness and metastasis in various cancer types[14-16]. Moreover,
As part of the current study, we evaluated KIF levels in various tumor types from the TCGA and how they relate to patient prognosis and infiltration rates. A final objective of this study was to determine the effects of silencing KIF3A on HCC cell migration and proliferation. Moreover, a study found that the KIF gene may be a potential target for future cancer therapies due to its role across many cancer types.
METHODS
Database source
The level of KIFs was determined using pan-cancer Genotype-Tissue Expression (GTEx) and The Cancer Genome Atlas (TCGA) data. KIF expression profiling data and clinical pan-cancer data from TCGA and GTEx were collected using the UCSC Xena database (https://xenabrowser.net/datapages/). These data include information about gene expression, copy number variations, and methylation, as well as clinical details. The RNA-seq data are presented in fragments of transcript per kilobase per million mapped reads (FPKM) format. The R package clusterProfiler was then added to the KIFs.
KIF family variation and DNA methylation across cancers
Using the cBioPortal database (http://www.cbioportal.org, version v3.7.9), we assessed the mutation frequency of KIF in 33 tumors. This database is an open resource tool for analyzing and visualizing various cancer genomics data[18]. The collaborative study included 10,519 samples from the TCGA pan-cancer atlas. Gene Set Cancer Analysis (GSCA) (http://bioinfo.life.hust.edu.cn/GSCA/#/) is a database that collects tumor genomic and immune genomic gene set information[19]. We investigated copy number variations (CNVs) of the KIF gene family in different cancers from the TCGA.
KIF protein expression level
The Human Protein Atlas (HPA) database (https://www.proteinatlas.org/) is a freely accessible platform that provides valuable information on protein expression and localization in human tissues, cells, and organs for researchers[20,21]. Using the HPA database, we investigated the protein expression levels of the KIF gene family in various cancers through immunohistochemistry (IHC).
Immunoinfiltration analysis
The xCell, TIMER, and immunedeconv (version 2.0.3) software packages[22] were used to investigate the relationship between tumor-infiltrating immune cells (TICs) and KIF.
Cell culture and transfection
HepG2 HCC cells used in this study were obtained from the Cell Bank of the Chinese Academy of Sciences (Shanghai, China) in RPMI 1640 medium containing 1% penicillin-streptomycin (PS) and 10% fetal bovine serum (FBS), 37 °C, 5% CO2. The siRNA target sequences (Gemma Gene, Suzhou, China) used in this study are listed in Supplementary Table 1.
RT-qPCR assay
Total cellular RNA was extracted using TRIzol® reagent (Invitrogen; Thermo Fisher Scientific, Inc.) according to the manufacturer's instructions. After determining the RNA content using UV light, cDNA was prepared from the extracted total RNA using rapid reverse transcriptase (Vazyme Biotechnology Co., Ltd., China). qRT-PCR was performed using TB Green Premix Ex TaqTM II (Takara Biotechnology Co., Ltd., China). The 2-ΔΔCq method was used to assess the relative mRNA expression level. The primers used were provided by Sangon Biotech, Inc. (Shanghai, China), and the sequences are shown in
Western blot analysis
Cells were lysed with RIPA lysis buffer. Protein content was determined using the BCA protein assay kit (Beijing Sola Biotechnology Co., Ltd.). Then, 15-30 µg of denatured proteins were separated by SDS-PAGE and transferred to a wet PVDF membrane. The membrane was then blocked with QuickBlockTM blocking buffer (Shanghai Bain-Marie Biotechnology Co., Ltd.) for 15 min at 37 °C and incubated overnight with primary antibodies against KIF3A (13930-1-AP, Proteintech) and β-actin (81115-1-RR, Proteintech). The membranes were then rinsed with TBST and incubated with HRP-conjugated goat anti-mouse and anti-rabbit IgG antibodies for 1 h at room temperature. In order to visualize the protein bands, an enhanced chemiluminescence detection kit was employed.
Counting kit-8 assay
A counting kit-8 (CCK8) assay was conducted to measure cell proliferation. HepG2 cells (4,000/well) were inoculated and cultivated in 96-well plates. After inoculation, the plants were cultured in an incubator for 0, 24, 48 h, or
EdU assay
HepG2 cells were cultured in 6-well plates at a density of 5 × 105 cells per well. Following inoculation, the cells were incubated for 0 , 24, 48 h, or 72 h. The BeyoClick™ EdU Cell Proliferation Kit with Alexa Fluor 488 (Beyotime Science & Technology Co. Ltd., China) was used to assess the ability of the cells to synthesize DNA.
Colony formation assay
Three distinct wells were set up for each group, and HepG2 cells (800/well) were inoculated and cultivated on 60 mm2 plates. The cells were rinsed after two weeks and then subjected to 15 min of fixation using 10% formaldehyde at ambient temperature and 15 min of staining using crystal violet. Finally, the colonies were counted under an optical microscope.
Cell wound scratch assay
Inoculate a 6-well plate with the amount of liquid required for 4 × 105 cells/well and add serum culture medium to ensure that each well contains 2 mL of the medium. Remove the triangular ruler and mark the cross direction on the perforated 6-well plate with a 100 µL media pipette tip. After three rounds of washing, serum-free culture medium was added. An image of the cross was then taken under the microscope.
Transwell assay
The cell density was adjusted to 5 × 105 cells/mL. Next, 200 μL of the cell suspension was added to the Transwell chamber. The cells were cultured conventionally for 12-48 h. Then, the wells were fixed using methanol for 30 min. Subsequently, the fixed cells were stained with 0.1% crystal violet for 20 min. Next, the cells were observed in five fields under a microscope at 200× magnification. In addition, their counts were recorded.
Statistical analysis
For all the statistical analyses, R (version 4.0.3) was used. Wilcoxon rank sum test and paired samples t-test were used to determine the statistical significance of KIF expression in unpaired and paired tissues, respectively. Wilcoxon rank sum test and logistic regression were used to investigate the relationship between clinical characteristics and KIF expression. Furthermore, all tests were two-sided, and P values less than 0.05 were considered to indicate statistical significance.
RESULTS
Expression of KIFs in cancer types
The mRNA expression levels of KIF family genes were examined in tumor tissues compared to normal tissues using a combination of TCGA samples and normal tissue samples from the GTEx database. Overall, KIFs were found to be highly expressed in most tumor tissues, such as THCA, KICH, PRAD, HNSC, KIRC, COAD, STAD, BLCA, KIRP, ESCA, BRCA, LUSC, LUAD, and LIHC [Figure 1A]. The full names of all abbreviated cancers within text in the Supplementary Table 3. Furthermore, when paired samples of tumor tissue and adjacent normal tissue were analyzed in TCGA, the majority of KIF family genes were found to be upregulated in CHOL, LIHC, and LUAD [Figure 1B]. In terms of protein levels, the expression of KIFs was analyzed using the Human Protein Atlas (HPA) database, which showed moderate to strong staining of KIF2A/KIF3B/KIF4A mainly in most cancers. Additionally, KIF2C expression was found to be moderate to high in glioma, renal cancer, urothelial cancer, cervical cancer, endometrial cancer, and lymphoma. KIF3A expression was found to be moderate to high in lung cancer, colorectal cancer, carcinoid, pancreatic cancer, testis cancer, endometrial cancer, ovarian cancer, melanoma, and lymphoma [Figure 1C].
Figure 1. The expression levels of KIF family members in various types of cancer were analyzed using different approaches. (A) The differential mRNA expression of KIFs in tumor and normal tissues was evaluated by combining data from the TCGA and GTEx databases. (B) Pan cancer data from the TCGA and GTEx databases show the levels of KIFs in tumor samples and corresponding non-cancerous samples. (C) Protein expression patterns of KIFs in different types of cancer were investigated using HPA data. 9D KIFs in tumor samples and corresponding non-cancerous samples are shown in the HPA data. (*P < 0.05, **P < 0.01, ***P < 0.001).
Genetic alterations, variation, and methylation of KIFs in different cancers
The mutation of KIFs in various cancers was investigated using cBioPortal. The results indicated a relatively high frequency of variations in KIF2C, KIF3A, and KIF3B. These variations were common in lung cancer, endometrial cancer, and hepatobiliary cancer [Figure 2A]. The differences in methylation between tumor and normal samples are summarized in Figure 2B. The present study also examined the percentage of copy number variations (CNVs) and their contribution to KIF expression in each cancer type. ACC, KIRC, KLRC, SARC, OCS, LIHC, LUSC, KICH, TGCT, ESCA, BLCA, HNSC, OV, STAD, LUAD, CHOL, UCEC, BRCA, SKCM, READ, COAD, GESC, and GBM exhibited a greater percentage of CNVs [Figure 2C]. Additionally, a correlation analysis was performed to explore the impact of DNA methylation in the promoter region on KIF expression. Methylation significantly suppressed the mRNA expression of KIF2A in STAD, HNSC, KIRC, LIHC, PKAD, and THCA; KIF2C in LGG; TGCT, KIRP, and SARC; KIF3A in LGG; TGCT, PCPG, and SKCM; and KIF3B in THYM, ESCA, STAD, and LUSC; and KIF4A in BRCA, LUAD, and LIHC [Figure 2D].
Figure 2. The variation and DNA methylation of the KIF family in pan-cancer were investigated using multiple methods. (A) The alteration frequency of KIFs across different types of cancer was analyzed using cBioPortal. (B) Methylation differences between paired tumor and paracancer samples were examined; grey dots represent no statistical significance. (C) Copy number variation (CNV) of KIFs in cancers was assessed by GSCA. (D) The correlation between DNA methylation and mRNA expression of KIFs was determined using TCGA data.
Relationship between KIFs and immune infiltration
KIFs and immune infiltration were comprehensively assessed using the xCell method, and the results showed a consistent pattern with the ESTIMATE score. In ACC, BCCA, CESC, HNSC, KIRP, LAUC, LIHC, SKCM and TGCT, KIF2A was associated with most immune cells. Similarly, KIF2C, KIF3A, KIF3B, and KIF4A were positively associated with infiltrating immune cells in almost all cancers [Figure 3].
KIF3A levels correlate with prognosis in HCC patients
In order to ascertain whether KIF levels have predictive significance, we also looked into the connection between KIF expression and overall survival (OS) using information from the TCGA database. Overexpression of KIF predicted poor prognosis in HCC patients [Figure 4], suggesting that KIF may play an oncogenic role in HCC.
Figure 4. The KIF family members have shown promising clinical significance in various types of cancers. (A) Diagnostic receiver operating characteristic (ROC) analysis was performed to evaluate the potential of KIFs as biomarkers for distinguishing between HCC and normal tissue samples. (B-F) OS curve analysis of KIFs was conducted by integrating HCC datasets.
Additionally, utilizing data from the TCGA database, correlations between KIF3A expression and overall survival in patients belonging to various HCC subgroups were examined [Figure 5]. The gene signature in the TCGA dataset was subjected to K-M survival analysis [Figure 6A]. To compare several groups, the log-rank test was employed. The high expression group's risk coefficient in relation to the low expression group is shown by the Hazard Ratio (HR). A gene's risk factor status is indicated by an HR > 1, where a higher expression level translates into a worse prognosis. As shown in Figure 6B, we discovered that KIF3A’s HR was 1.591, suggesting that this gene is a risk factor. To see the projected OS probability over the next year, three years, and five years, we made a nomogram. When comparing the 3-year and 5-year survival rates to the actual outcomes, our model showed the highest predicted accuracy. Furthermore, Figure 6C illustrates how well the model matches the 1-year survival rates.
Figure 5. Kaplan-Meier analysis of the prognostic value of KIF3A expression in different HCC subgroups. (A-F) Comparison of OS curves for T1 and T2, N0 and N1, M0 and M1, pathological stage I and II, age > 60 years and vascular invasion subgroups in patients with high and low KIF3A expression in HCC.
Figure 6. Nomograms and calibrations for the prediction of 1-year, 3-year, and 5-year overall survival in patients with HCC. (A) Gene expression, survival time, and status of patients in the TCGA dataset. (B) The low-expression sample's hazard ratio (HR) in comparison to the high-expression sample was ascertained. (C) The ROC curve of the genes.
Correlation analysis and enrichment
We collected the genes contained in the corresponding path and analyzed them with R software GSVA encapsulation. The parametric method “ssgsea” is selected. Paswell scores and genes were correlated using Spearman correlation. The activation of KIF3A is associated with hyperactivation of a number of cancer-related pathways within HCC, such as angiogenesis, apoptosis, DNA replication, tumor proliferation, MYC targeting, EMT markers, DNA repair, and the p53 pathway [Figure 7].
Figure 7. Spearman's correlations were used to examine the relationships between individual genes and pathway scores. (A-I) Apoptosis, DNA replication, tumor proliferation, G2/M checkpoint, MYC targets, p53 pathway, EMT markers, ECM-related genes, and DNA repair pathways are shown. Horizontal coordinates indicate gene expression distribution and vertical coordinates indicate pathway score distribution. Top values indicate correlation P-values, correlation coefficients, and how the correlation was calculated.
KIF3A promotion of HCC cell proliferation and migration
Western blotting was used to detect KIF3A protein expression levels in normal hepatocytes LX-2 and HepG2 cells. KIF3A protein expression was significantly greater in HepG2 cells than in LX-2 cells [Figure 8A]. The HCC cell line HepG2 with KIF3A knockdown was constructed by liposome transfection, and the knockdown effect of KIF3A was detected by real-time PCR and Western blotting. The results showed that the expression of KIF3A in the HepG2 cells transfected with siKIF3A was significantly lower than that in the negative control cells [Figure 8B]. Using CCK-8, plate cloning, and EdU experiments, KIF3A promoted HCC cell proliferation [Figure 8C-E]. In scratch and transwell assays, KIF3A enhanced HCC cell migration and invasion [Figure 8F and G].
Figure 8. Effects of KIF3A on the proliferation and migration of HCC. (A) Western blot analysis was used to analyze KIF3A expression in LX-2 and HepG2 cells. (B) The knockdown efficiency of KIF3A in HepG2 cells was detected by real-time PCR and Western blotting. (C) CCK-8 assay to detect the proliferation of HepG2 cells after the knockdown of KIF3A. (D and d) A plate colony formation assay was used to detect the colony formation ability of cells after the knockdown of KIF3A. (E and e) BeyoClick™ EdU-488 assay to detect cellular DNA synthesis after the knockdown of KIF3A. (F and f) The migration ability of cells after KIF3A knockdown was detected by a scratch test. (G and g) The invasion ability of cells after KIF3A knockdown was detected by Transwell assay (*P < 0.05, **P< 0.01,
DISCUSSION
The Kinesin superfamily is classified into various subfamilies based on their structural and functional characteristics[23]. KIFs is an important molecular motor protein, which is responsible for key processes such as intracellular material transport, cell function and signal transduction[24]. In pan-cancer research, KIFs can quickly and accurately transport proteins, RNA, organelles, etc. to specific locations to ensure the normal functioning of cell functions. In cancer cells, this efficient transport may support rapid proliferation and invasion of tumor cells. KIFs play a key role in spindle formation and chromosome separation, ensuring the accuracy of cell replication. In cancer, abnormal expression of KIFs may lead to chromosomal instability and promote tumorigenesis. KIFs can respond to signal changes in the tumor microenvironment, such as hypoxia and acidosis, to help cancer cells adapt to the harsh environment and survive. Mutations or dysregulation of Kinesin superfamily genes have been associated with various diseases and disorders. Dysfunction of Kinesin superfamily genes has also been implicated in cancer metastasis, neurodegenerative diseases, and developmental disorders[25,26]. Studies have shown that abnormal expression of KIF2A[14,27], KIF2C, KIF3B[28,29], KIF3A, and KIF4A are associated with the development and progression of several tumors, including breast, colon, and lung cancer. The overexpression of KIF2A, KIF2C, KIF3B, KIF3A, and KIF4A may promote the proliferation and invasion of tumor cells and is also associated with poor prognosis. Therefore, inhibiting the function of KIFs may be a potential strategy for treating tumor invasion and metastasis. Understanding the function and mechanisms of kinesin superfamily genes is essential for understanding the complexity of intracellular trafficking and its impact on health and disease. The precise mechanisms underlying their actions are not yet fully understood. In this study, we found that KIFs are associated with immune regulation, which provides a basis for exploring their involvement in the tumor microenvironment (TME).
The TME plays an important role in tumor development, angiogenesis and immune escape[30,31]. KIFs may be involved in regulating a variety of cell types and processes in the TME[32-34]. First, in tumor angiogenesis, these proteins may regulate the migration and proliferation of endothelial cells and play a regulatory role in angiogenesis. Second, in terms of immune cell infiltration and activation[35], these proteins may affect the migration, proliferation, and function of tumor-associated immune cells. In addition, they may affect the proliferation and secretion of tumor-associated stromal cells, further altering the composition of cytokines and the extracellular matrix in the TME[36]. Researchers have begun exploring the use of these proteins as potential therapeutic targets to regulate the TME[37]. By inhibiting or activating the functions of KIF2A, KIF2C, KIF3B, KIF3A, and KIF4A, cell interactions and signaling pathways in the TME can be disrupted, thereby affecting tumor growth and progression. Furthermore, inhibiting these proteins may suppress tumor angiogenesis, reduce immune escape, and decrease tumor-associated mesenchymal activity, thereby improving the efficacy of immunotherapy.
Tumor immunotherapy is an emerging therapeutic strategy that involves activating a patient's own immune system to attack and clear tumor cells[38]. Recent studies suggest that KIF2A, KIF2C, KIF3B, KIF3A, and KIF4A may play important roles in tumor immunotherapy. First, the overexpression of these proteins in tumor cells is associated with tumor invasion and metastasis, which may lead to tumor escape from immune cells[39]. Therefore, inhibiting the expression or function of these proteins may help boost immune cells’ ability to attack tumors. Second, these proteins may serve as potential targets in tumor immunotherapy. It is possible to reduce the resistance of tumor cells to immune checkpoint inhibitors, thereby improving the efficacy of immunotherapy by inhibiting the function of KIF2A, KIF2C, KIF3B, KIF3A, and KIF4A. KIFs enhances the motility of HCC cells and promotes invasion and metastasis by regulating cytoskeletal rearrangement and vesicular transport. For example, KIF14 and KIF23 are highly expressed in HCC and are associated with tumor aggressiveness and poor prognosis. KIFs promotes the rapid proliferation of HCC cells by transporting cell cycle-related proteins and regulating mitosis[40]. KIF11 and KIF20A are highly expressed in HCC and are closely related to tumor growth. KIFs may promote immune escape from HCC by transporting immunomodulatory molecules or cytokines that affect immune cell infiltration and function in the tumor microenvironment. KIF3A expression was greater in many tumors than in non-carcinoma samples in this study, highlighting the role of KIF3A as a novel oncogene in these tumors. A subsequent investigation disclosed that silencing KIF3A suppressed the proliferation and migration of HepG2 cells. Furthermore, KIF3A expression was related to M2 macrophage and T cell infiltration levels in HCC, suggesting that KIF3A plays a role in tumor immunology. The relevant mechanisms ought to be further investigated to enhance the diagnosis and treatment of HCC.
CONCLUSION
In summary, the kinase protein superfamily plays a multifaceted role in cancer biology, including cell division, intracellular transport, migration, and invasion [Figure 9]. Dysregulation of specific motor proteins can promote the occurrence and progression of tumors. Understanding the exact mechanisms by which they are involved in cancer could lead to the development of new treatment strategies to fight the disease. KIF3A was also found to be a potential prognostic factor affecting HCC development. Although further studies are needed to explore and confirm the mechanism of HCC development, we speculate that KIF3A is a new target for HCC diagnosis and treatment.
LIMITATION
The study does not include in vivo experiments to assess the effects of KIF3A knockdown on tumor growth and metastasis in animal models. Such studies are crucial for understanding the therapeutic potential of targeting KIF3A in a more physiologically relevant context. Although the study suggests that KIFs are associated with immune cell infiltration, the exact mechanisms by which KIFs influence the tumor immune microenvironment are not fully elucidated. Further studies are needed to dissect these interactions and their implications for cancer immunotherapy. The study identifies KIF3A as a potential prognostic biomarker and therapeutic target, but the translation of these findings into clinical practice remains uncertain. More clinical trials are required to validate the prognostic value of KIF3A and to assess the efficacy of KIF3A-targeted therapies in patients.
DECLARATIONS
Authors’ contributions
Designed the study: Zhang X
Carried out the study and drafted the manuscript: Zhang X, Zhang Y
Assisted in the methods section: Zheng G
Guidance: Yang R
All authors read and approved the final version.
Availability of data and materials
The single‐gene bioinformatics datasets analyzed in the present study are available in the TCGA, GSEA and KEGG databases (https://portal.gdc.cancer.gov, https://www.gsea-msigdb.org/gsea/msigdb, https://www.genome.jp/kegg/).
Financial support and sponsorship
The manuscript was supported in part by Guangdong Basic and Applied Basic Research Foundation (2022A1515012160).
Conflicts of interest
All authors declared that there are no conflicts of interest.
Ethical approval and consent to participate
Not applicable.
Consent for publication
Not applicable.
Copyright
© The Author(s) 2025.
Supplementary Materials
REFERENCES
1. Chen Z, Zhang P, Xu Y, et al. Surgical stress and cancer progression: the twisted tango. Mol Cancer. 2019;18:132.
2. Grewal AS, Jones J, Lin A. Palliative radiation therapy for head and neck cancers. Int J Radiat Oncol Biol Phys. 2019;105:254-66.
3. Ippolito MR, Martis V, Martin S, et al. Gene copy-number changes and chromosomal instability induced by aneuploidy confer resistance to chemotherapy. Dev Cell. 2021;56:2440-54.e6.
4. Tímár J, Uhlyarik A. On-target side effects of targeted therapeutics of cancer. Pathol Oncol Res. 2022;28:1610694.
5. Zhang Y, Zhang Z. The history and advances in cancer immunotherapy: understanding the characteristics of tumor-infiltrating immune cells and their therapeutic implications. Cell Mol Immunol. 2020;17:807-21.
6. Zeng D, Wu J, Luo H, et al. Tumor microenvironment evaluation promotes precise checkpoint immunotherapy of advanced gastric cancer. J Immunother Cancer. 2021;9:e002467.
7. Huang H, Nie CP, Liu XF, et al. Phase I study of adjuvant immunotherapy with autologous tumor-infiltrating lymphocytes in locally advanced cervical cancer. J Clin Invest. 2022;132:e157726.
8. Mailankody S, Devlin SM, Landa J, et al. GPRC5D-targeted CAR T cells for myeloma. N Engl J Med. 2022;387:1196-206.
9. Walle T, Kraske JA, Liao B, et al. Radiotherapy orchestrates natural killer cell dependent antitumor immune responses through CXCL8. Sci Adv. 2022;8:eabh4050.
10. Chesney J, Lewis KD, Kluger H, et al. Efficacy and safety of lifileucel, a one-time autologous tumor-infiltrating lymphocyte (TIL) cell therapy, in patients with advanced melanoma after progression on immune checkpoint inhibitors and targeted therapies: pooled analysis of consecutive cohorts of the C-144-01 study. J Immunother Cancer. 2022;10:e005755.
11. Li S, Yu W, Xie F, et al. Neoadjuvant therapy with immune checkpoint blockade, antiangiogenesis, and chemotherapy for locally advanced gastric cancer. Nat Commun. 2023;14:8.
12. Duan H, Zhang X, Wang FX, et al. KIF-2C expression is correlated with poor prognosis of operable esophageal squamous cell carcinoma male patients. Oncotarget. 2016;7:80493-507.
13. Cai Y, Mei J, Xiao Z, et al. Identification of five hub genes as monitoring biomarkers for breast cancer metastasis in silico. Hereditas. 2019;156:20.
14. Wang F, Li J, Li L, et al. Circular RNA circ_IRAK3 contributes to tumor growth through upregulating KIF2A via adsorbing miR-603 in breast cancer. Cancer Cell Int. 2022;22:81.
15. Huang J, Sun X, Diao G, Li R, Guo J, Han J. KIF15 knockdown inhibits the development of endometrial cancer by suppressing epithelial-mesenchymal transition and stemness through Wnt/β-catenin signaling. Environ Toxicol. 2023;1824:34.
16. Zeng S, Wang Q, Zhou R, Wang D. KIF4A functions as a diagnostic and prognostic biomarker and regulates tumor immune microenvironment in skin cutaneous melanoma. Pathol Res Pract. 2024;254:155166.
17. Zhong M, Gong L, Li N, et al. Pan-cancer analysis of kinesin family members with potential implications in prognosis and immunological role in human cancer. Front Oncol. 2023;13:1179897.
18. Cerami E, Gao J, Dogrusoz U, et al. The cBio cancer genomics portal: an open platform for exploring multidimensional cancer genomics data. Cancer Discov. 2012;2:401-4.
19. Liu CJ, Hu FF, Xie GY, et al. GSCA: an integrated platform for gene set cancer analysis at genomic, pharmacogenomic and immunogenomic levels. Brief Bioinform. 2023;24:bbac558.
20. Uhlen M, Zhang C, Lee S, et al. A pathology atlas of the human cancer transcriptome. Science. 2017;357:eaan2507.
21. Thul PJ, Åkesson L, Wiking M, et al. A subcellular map of the human proteome. Science. 2017;356:eaal3321.
22. Sturm G, Finotello F, Petitprez F, et al. Comprehensive evaluation of transcriptome-based cell-type quantification methods for immuno-oncology. Bioinformatics. 2019;35:i436-45.
23. Li X, Tai Y, Liu S, et al. Bioinformatics analysis: relationship between adrenocortical carcinoma and KIFs. Biotechnol Genet Eng Rev. 2023;39:575-85.
24. Xiao KH, Teng K, Ye YL, et al. Kinesin family member C1 accelerates bladder cancer cell proliferation and induces epithelial-mesenchymal transition via Akt/GSK3β signaling. Cancer Sci. 2019;110:2822-33.
25. Wang J, Wang D, Fei Z, et al. KIF15 knockdown suppresses gallbladder cancer development. Eur J Cell Biol. 2021;100:151182.
26. Hu ZD, Jiang Y, Sun HM, et al. KIF11 promotes proliferation of hepatocellular carcinoma among patients with liver cancers. Biomed Res Int. 2021;2021:2676745.
27. Xie T, Li X, Ye F, et al. High KIF2A expression promotes proliferation, migration and predicts poor prognosis in lung adenocarcinoma. Biochem Biophys Res Commun. 2018;497:65-72.
28. Gu R, Li X, Yan X, Feng Z, Hu A. Circular RNA circ_0032462 enhances osteosarcoma cell progression by promoting KIF3B expression. Technol Cancer Res Treat. 2020;19:1533033820943217.
29. Joseph NF, Grinman E, Swarnkar S, Puthanveettil SV. Molecular motor KIF3B acts as a key regulator of dendritic architecture in cortical neurons. Front Cell Neurosci. 2020;14:521199.
30. Duan Y, Xu X. A signature based on anoikis-related genes for the evaluation of prognosis, immunoinfiltration, mutation, and therapeutic response in ovarian cancer. Front Endocrinol. 2023;14:1193622.
31. Qin H, Lu H, Qin C, et al. Pan-cancer analysis suggests that LY6H is a potential biomarker of diagnosis, immunoinfiltration, and prognosis. J Cancer. 2024;15:5515-39.
32. Guo X, Zhou L, Wu Y, Li J. KIF11 As a potential pan-cancer immunological biomarker encompassing the disease staging, prognoses, tumor microenvironment, and therapeutic responses. Oxid Med Cell Longev. 2022;2022:2764940.
33. Qiu MJ, Wang QS, Li QT, et al. KIF18B is a prognostic biomarker and correlates with immune infiltrates in pan-cancer. Front Mol Biosci. 2021;8:559800.
34. Cao Z, Guan M, Cheng C, et al. KIF20B and MET, hub genes of DIAPHs, predict poor prognosis and promote pancreatic cancer progression. Pathol Res Pract. 2024;254:155046.
35. Li T, Fu J, Zeng Z, et al. TIMER2.0 for analysis of tumor-infiltrating immune cells. Nucleic Acids Res. 2020;48:W509-14.
36. Quail DF, Joyce JA. Microenvironmental regulation of tumor progression and metastasis. Nat Med. 2013;19:1423-37.
37. Leong SP, Aktipis A, Maley C. Cancer initiation and progression within the cancer microenvironment. Clin Exp Metastasis. 2018;35:361-7.
38. Li Q, Chen Q, Klauser PC, et al. Developing covalent protein drugs via proximity-enabled reactive therapeutics. Cell. 2020;182:85-97.e16.
39. Spitzer MH, Carmi Y, Reticker-Flynn NE, et al. Systemic immunity is required for effective cancer immunotherapy. Cell. 2017;168:487-502.e15.
Cite This Article

How to Cite
Download Citation
Export Citation File:
Type of Import
Tips on Downloading Citation
Citation Manager File Format
Type of Import
Direct Import: When the Direct Import option is selected (the default state), a dialogue box will give you the option to Save or Open the downloaded citation data. Choosing Open will either launch your citation manager or give you a choice of applications with which to use the metadata. The Save option saves the file locally for later use.
Indirect Import: When the Indirect Import option is selected, the metadata is displayed and may be copied and pasted as needed.
About This Article
Copyright
Data & Comments
Data
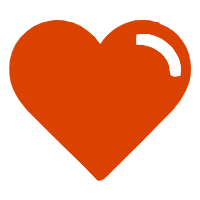
Comments
Comments must be written in English. Spam, offensive content, impersonation, and private information will not be permitted. If any comment is reported and identified as inappropriate content by OAE staff, the comment will be removed without notice. If you have any queries or need any help, please contact us at [email protected].