Forum
Contents
Host
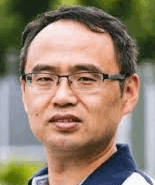
Professor Shujun Zhang
Editor-in-Chief of Microstructures
Institute for Superconducting and Electronic Materials, University of Wollongong, Australia.
Institute for Superconducting and Electronic Materials, University of Wollongong, Australia.
Speaker
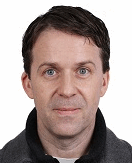
Professor Timon Rabczuk
Institute of Structural Mechanics, Bauhaus-University Weimar, Germany.
Timon did his PhD in January 2002 at University of Karlsruhe. He subsequently worked as postdoctoral fellow in the Computational Mechanics group of Prof. Ted Belytschko at Northwestern University for nearly 4 years, before joining the Technical University of Munich. He was employed as Senior Lecturer at the University of Canterbury from February 2007-2009 before being appointed as Full Professor in Computational Mechanics at Bauhaus University Weimar. Timon’s research interest include computational methods, computational materials design and computational integrated materials engineering.
Special Issue:
Machine Learning for Microstructures and Materials
Special Issue:
Machine Learning for Microstructures and Materials
Introduction
Energy harvesters are an increasingly attractive alternative to conventional batteries. They capture minute amounts of energy from one or more of the surrounding energy sources where a remote application is deployed, and where such energy source is inexhaustible. One of the most common energy harvesters are based on piezoelectricity converting mechanical energy into electrical energy. While most computational studies focus on macro-scale energy harvesters, there are comparatively few studies on nano-scale energy harvesters which promise a significant improvement due to the presence of size effects at the nano-scale. Furthermore, there are only a few studies on flexoelectricity. Flexoelectricity can be considered as an extension of piezoelectricity and is a size dependent phenomenon even in the absence of surface effects.
However, modeling flexoelectricity is challenging due to the C1 requirement of the underlying discretization. This presentation will propose an efficient computational formulation for flexoelectric nano-structures which exploits the higher continuity of the underlying discretization and therefore avoids a complex mixed formulation. Furthermore, an efficient level-set based topology optimization will be presented. The formulation will subsequently be extended to multi-materials and it will be shown that adding soft materials can significantly enhance the energy conversion efficiency of a composite energy harvester.
However, modeling flexoelectricity is challenging due to the C1 requirement of the underlying discretization. This presentation will propose an efficient computational formulation for flexoelectric nano-structures which exploits the higher continuity of the underlying discretization and therefore avoids a complex mixed formulation. Furthermore, an efficient level-set based topology optimization will be presented. The formulation will subsequently be extended to multi-materials and it will be shown that adding soft materials can significantly enhance the energy conversion efficiency of a composite energy harvester.
Moments
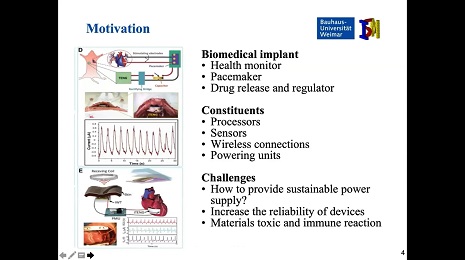
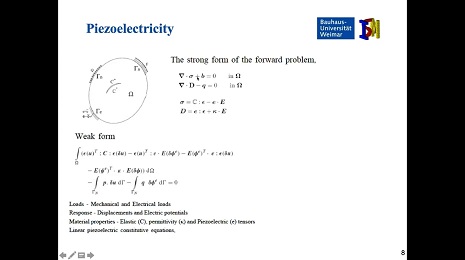
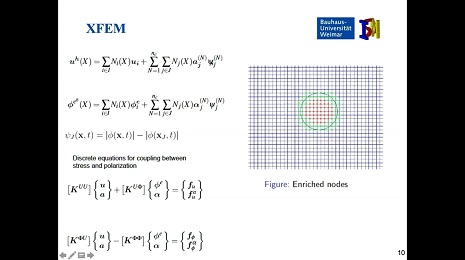
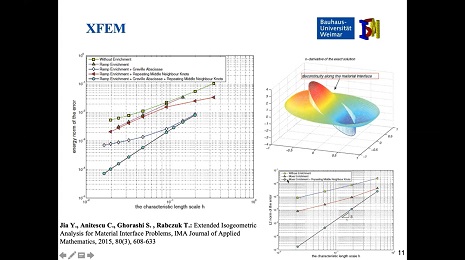
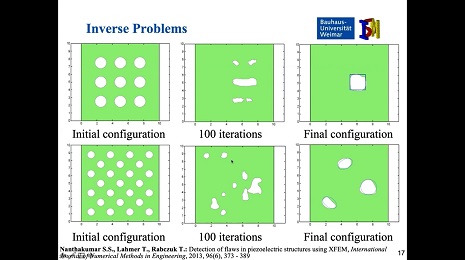
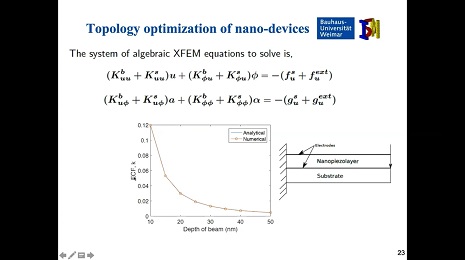
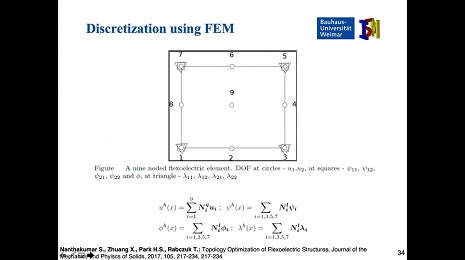
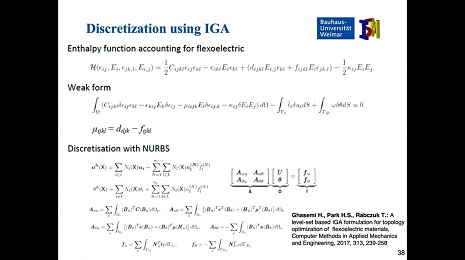
Presentation
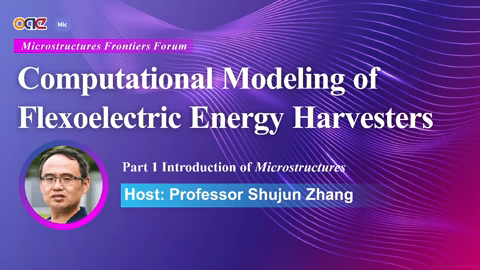
Computational Modeling of Flexoelectric Energy Harvesters: Part 1 Introduction of Microstructures and Speaker
NaN
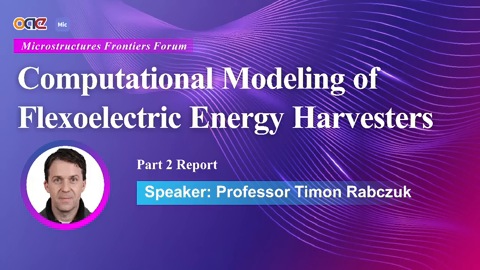
Computational Modeling of Flexoelectric Energy Harvesters: Part 2 Report
NaN
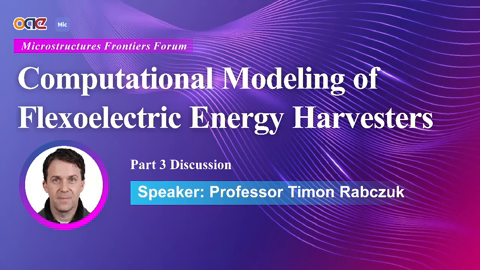
Computational Modeling of Flexoelectric Energy Harvesters: Part 3 Discussion
NaN