Cross-scale backscattered-electron imaging and its application in revealing the microstructure-property relations
Abstract
Scanning electron microscopy (SEM) has been widely utilized in the field of materials science due to its significant advantages, such as large depth of field, wide field of view, and excellent stereoscopic imaging. However, at high magnification, the limited imaging range in SEM cannot cover all the possible inhomogeneous microstructures. In this research, we propose a novel approach for generating high-resolution SEM images across multiple scales, enabling a single image to capture physical dimensions at the centimeter level while preserving submicron-level details. We adopted the SEM imaging on the AlCoCrFeNi2.1 eutectic high entropy alloy as an example. SEM videos and image stitching are combined to fulfill this goal, and the video-extracted low-definition images are clarified by a well-trained denoising model. Furthermore, we segment the macroscopic image of the eutectic high entropy alloy, and the area of various microstructures is distinguished. By combining the segmentation results and hardness experiments, we found that the hardness is positively correlated with the content of the body-centered cubic phase and negatively correlated with the lamella width. The whole procedure is also applied to a thermoelectric gradient material (PbSe-SrSe). Our work provides a feasible solution to generate macroscopic images based on SEM for further analysis of the correlations between the microstructures and spatial distribution, and can be widely applied to other types of microscopes.
Keywords
INTRODUCTION
Microstructure of a material refers to its local composition, grains, phases, and other structural features at a microscopic scale. It plays a crucial role in determining the material’s properties, such as mechanical strength, electrical conductivity, thermal conductivity, corrosion resistance, and more. One classical example is material strengthening through manipulation of structural heterogeneity at various scales such as impurity atoms, dislocations, twinning, grain boundary, precipitation/dispersion phases, composites, etc. Therefore, researchers have devoted considerable effort over an extended period to the characterization and design of microstructures that are intricately tied to the manufacturing process. Significant progress has been made in the fabrication of metals with various microstructures using different compositions, manufacturing methods and parameters, and optimum microstructures could be determined by comparing the corresponding properties[1-3]. On the other hand, gradient materials were intentionally designed to establish the local microstructure-property correlation to screen the microstructure that exhibits the desired properties[4,5]. Regardless of the approach taken, it is crucial to accurately determine the relationship between the local microstructural characteristics and its properties throughout the entire sample. Even in the first approach, microstructural gradient or structural non-uniformity can exist within a single ingot due to local variations in parameters during fabrications[6,7]. This phenomenon of structural non-uniformity and anisotropy is more common in materials prepared by additive manufacturing, which builds parts by adding material one layer at a time and involves complex cyclic thermal history[8,9]. Therefore, characterizing the structure of micro-regions to understand the performance of the entire sample may be inaccurate and far from sufficient. High-throughput characterization method to reveal the microstructures over large length scales is indispensable to illustrate the structural heterogeneity within the ingot, understand the local microstructure-property correlation, and screen the superior microstructure in a rapid way.
We take the common material characterization technique, scanning electron microscopy (SEM), as an example. SEM plays an extremely significant role in the characterization of microstructures, providing detailed imaging and analysis of surface morphology, grain boundaries, phase distribution with high-definition (HD)[10] and magnification. Definition refers to the clarity of an image and its capacity to present fine details; HD images feature a high signal-to-noise ratio while low-definition (LD) images have a low ratio. However, obtaining cross-scale images through SEM is often an impossible task due to limitations in resolution and working distance. Higher-resolution imaging often requires shorter working distances, limiting the field of view and the ability to image large areas. Therefore, it poses a challenge in simultaneously optimizing imaging resolution, field of view, and imaging speed. One possible solution is super-resolution methods[11-16] based on deep learning, which have shown tremendous potential in enhancing image resolution. These methods utilize deep neural networks to learn the mapping between low-resolution (LR) and high-resolution (HR) images, thereby generating HR images from LR inputs. The super-resolution convolutional neural network (SRCNN) developed by Dong et al. is considered the seminal work in image super-resolution reconstruction based on deep learning[17]. The very deep super resolution (VDSR) method presented by Kim et al. introduced residual learning[18]. Ledig et al. utilized generative adversarial networks for super-resolution[19]. Zhang et al. enhanced feature learning with channel attention and proposed the residual in residual structure[20]. Liang et al. utilized Swin Transformer for image super-resolution, combining Transformer with convolutional neural networks (CNN)[21]. These methods have been successfully applied in various fields, including medical imaging[22-25] and remote sensing[26-28]. In the field of electron microscopy, Fura et al. employed generative adversarial networks to enhance the resolution of SEM images of fractured cathode materials[29]. Jangid et al. found that incorporating domain knowledge into the training process of super-resolution models improves their performance on electron microscopy image datasets[30]. However, existing work has limitations in resolution enhancement while maintaining the original field of view, typically achieving 2×, 4×, 8×, or 16× enhancements. As magnification increases, the “authenticity” of the images diminishes. Even with a 16× magnification, it falls short of the requirements for cross-scale imaging. Moreover, limited magnification may not meet the requirements when characterizing materials at the centimeter scale while preserving microscale details, which cannot be solely addressed by conventional super-resolution techniques. Another potential solution for cross-scale imaging is image stitching. Yin et al. have developed an all-weather continuous autonomous imaging system for transmission electron microscopy, enabling high-throughput image acquisition at the petabyte scale through parallelization and automation[31]. This work achieved high-throughput imaging and stitching to obtain extremely large images. However, it suffers from fatal drawbacks such as equipment modifications and excessively long acquisition times.
To overcome these challenges, we propose a novel method for generating cross-scale HR-SEM images, i.e., macroscopic ones with submicron-level details. The required equipment is simply a standard electron microscope with video recording capability, along with our plug-and-play system called the Cross-Scale Electron Microscopy Image Generation System (CEMI), as shown in Figure 1. Given a LD SEM video as input, CEMI extracts consecutive LD frames, and feeds them into a pre-trained denoising model to generate corresponding HD images. The images are then stitched together using an image stitching module. This technique holds paramount significance for materials research. Firstly, our proposed method addresses the limited imaging range issue of traditional SEM techniques, and it has no magnification limitations while preserving microscale details in comparison with super-resolution methods, allowing researchers to obtain images over a broader range. By generating large-scale HR images, researchers can better observe the microstructure and properties of materials, providing a more comprehensive understanding in the field of materials science. Secondly, we greatly reduce the cost of acquiring HD SEM images based on LD ones at a much faster scanning speed. Furthermore, we explore analytical methods for the cross-scale image to gain better insights into the microstructure and properties of materials. Due to the enormous imaging range of cross-scale images, it is challenging for the human eye to derive meaningful conclusions directly. Therefore, we employed image segmentation techniques to segment the microstructures of interest. In this work, we conducted a statistical analysis of the distribution of three microstructural components in AlCoCrFeNi2.1 eutectic high entropy alloys (EHEA). These components include lamellar structures, lamellar width, and the body-centered cubic (BCC) phase. Furthermore, we analyzed their correlation with material hardness. Another example presented in this work is the application of the CEMI to thermoelectric gradient material. CEMI is not only applicable to SEM but also easily integrable with various types of microscopes, making it a valuable tool for researchers across multiple scientific disciplines.
RESULTS
Image capturing
Conventionally, a series of high-magnification photographs with partial (typically 30%-60%) overlaps must be captured to serve as input data for image stitching over large length scales[32-37]. This is also true for the commercial software Thermo Scientific Maps, which is only compatible with its own facilities. However, shooting HD images is very time-consuming and labor-intensive. Even for skilled operators, taking a HD SEM image requires 1-2 min (including locating the local region, focusing, scanning and saving). By contrast, CEMI offers flexibility to deal with either low-quality videos or LD images directly. Compared with HD image (cycle time = 26.2 s), it only takes one-tenth of the corresponding time to acquire the LD image (cycle time = 2.7 s). Furthermore, we can continue to save time in data acquisition and free up manpower by using the automatic sample stage translation and video capture function. By setting an appropriate movement velocity, a low-quality video containing structural information throughout the lateral x-axis could be obtained. In this way, we finally collected 18 videos, from which 3,902 frames were automatedly extracted by setting appropriate extraction intervals, covering the specimen with a macro size of 2.75 cm × 0.175 cm as shown in Figure 2A. To facilitate subsequent stitching, the extracted adjacent video frames have a certain degree of overlap (which can be cumbersome to manually control). In fact, we can further magnify the image to capture videos, obtaining more photographs and finer structural information as well as maintaining the same level of definition. After preprocessing by the image acquisition module, the LD image is sent to the denoising module, where it is processed to obtain the corresponding HD image.
Image denoising
The overall structure of the denoising model is inspired by ESRGAN[38], which can be used for image denoising when the magnification factor is 1, and utilizes an adversarial neural network[39] consisting of a generator and a discriminator. As shown in Figure 2B, the generator consists of four components: shallow feature extraction, deep feature extraction, upsampling module, and reconstruction module. The deep feature extraction consists of multiple basic blocks, which are implemented based on the Residual-in-Residual Dense Block[38]. Each basic block contains three dense blocks, and each dense block consists of five convolutional layers (with ReLU activation applied after the first four layers). The discriminator employs a U-Net[40] network with spectral normalization regularization[41], which helps stabilize the training process. We trained the model using 100 pairs of high-low definition images of high-entropy alloy samples (detailed shooting methods can be found in the Section "Methods"). Due to the instability of adversarial training, we first trained the generator separately using L1 loss. The resulting denoising model is referred to as SEMNET. Then, we used the trained SEMNET as the initialization for the generator in the adversarial neural network and combined L1 loss, content perception loss, and adversarial loss to obtain the final denoising model, named SEMGAN. The results are shown in Figure 3A. Overall, images generated by SEMNET tend to be relatively smooth, with some loss of fine details compared to HD real images. In contrast, SEMGAN performs better in this regard and is therefore used as the denoising model in CEMI. The so-obtained HD images show better similarity with the ground-truth (GT) HD images, than the LD images. For example, we evaluated the numerical disparities in BCC phase fractions (details for this microstructure will be discussed later in this work) between the output images from the denoising model and the real 100 HD-LD image pairs in the training set [Supplementary Figure 1]. The results of the denoising model’s output images closely align with those of real HD images, while real LD images show larger disparities from the other two. Furthermore, when only HD images are available, we can use a degradation model to obtain the corresponding LD images, which can then be used for training the denoising model. You can find more detailed information in the Section "Discussion".
Figure 3. Denoising model performance demonstration. (A) LD represents the low-definition input image to the model, followed by the HD images generated by SEMNET and SEMGAN. GT denotes the GT HD image. (B) The image shows a large-scale image created by stitching together 3,902 images. It has a size of 123,672 × 7,848 pixels and a physical size of 2.75 cm × 0.175 cm. The actual length of the material is approximately 28 mm.
The denoising module is an indispensable component of CEMI in this EHEA case for two main reasons. Firstly, for the purpose of rapidly and cost-effectively generating cross-scale images, LD images obtained through the image capture module must undergo the denoising module before being used for subsequent stitching. Secondly, the denoising module can be used independently, reducing the cost of acquiring HD images. Furthermore, as per our knowledge, there is currently no high and LD SEM image data set for training denoising models. We have produced and made the above data public, which will help promote the research of denoising models dedicated to the SEM area.
Image stitching
By using the trained denoising model, we can input LD images obtained from the image acquisition module and generate corresponding HD images. Subsequently, we employ image stitching methods to merge the generated HD images and create cross-scale images. The stitching process takes time proportional to the number of images being stitched, with approximately 3 h needed for the 3,902 images. In our experiments, we found that LD images are not suitable for large-scale image stitching due to their limited feature points. Even when combining two LD images, there is still a possibility of stitching failure. Therefore, LD images are not usable when the number of images for stitching reaches thousands. As shown in Figure 3B, we generated a large-sized image of 123,672 × 7,848 pixels using the 3,902 images, which corresponds to a physical size of 2.75 cm × 0.175 cm. An animation of the zooming-in process on the cross-scale image is demonstrated in Supplementary Gif 1. For ease of description in the following text, we will refer to the horizontal direction as the x-direction and the vertical direction as the y-direction. Remarkably, even at this scale, we were able to observe details at the submicron level. The amplification level in this work is equivalent to reading a textbook with a font size of 10 from a distance of 300 m.
The significance of cross-scale images lies in their ability to capture a wide range of information that is not easily obtained through conventional imaging techniques. In our experiments, we utilized SEM, but any microscope capable of recording videos can leverage CEMI to generate cross-scale images that were previously unattainable. From a material application perspective, an overwhelming amount of information provided by cross-scale images makes manual analysis practically impossible. Consequently, there is a need to develop CEMI plugins for automated cross-scale image analysis. In the following section, we demonstrate the scientific value of CEMI by showcasing a plugin for image segmentation in high-entropy alloys.
Applications of cross-scale image
In the context of cross-scale images generated through stitching, a more in-depth exploration of analytical methods has been undertaken. The sample we adopted here was AlCoCrFeNi2.1 EHEA, in which fine, intricately spaced phases provide exceptional mechanical properties, making it gain significant attention in aerospace, automotive, and other applications in various industries. Prior investigations indicated that hardness, strength, and ductility are correlated with some factors such as the contents of lamellar eutectic structures, BCC phases, and size of eutectic structures[42-45]. In order to illustrate the structural heterogeneity within the ingot and determine local microstructure-property correlation in a high throughput manner, we used CEMI to automatically image the microstructure with a high resolution throughout the entire ingot. The ingot of AlCoCrFeNi2.1 EHEA was manufactured by arc melting method, with a diameter of ~ 3 cm. To quantitatively obtain the distribution of different types of microstructural features, the cross-scale image has been partitioned into a grid of 19 × 243 smaller images, each measuring 508 × 413 pixels. Subsequently, dedicated procedures have been applied to these smaller images, encompassing lamellar structure segmentation, lamellar width quantification, and BCC phase proportion estimation. The outcomes of these operations are then visualized on a 19 × 243 matrix, where darker shades indicate higher numerical values in the respective regions. The so-obtained distribution of microstructures serves as a quantitative tool to eliminate the bias of inhomogeneity, and such information cannot be obtained from single SEM (or other type) images.
For lamellar structure segmentation, an image segmentation model has been employed. In recent years, image segmentation networks[46-51] based on deep learning have experienced rapid development. In this work, the U-net[40] architecture, known for its robust segmentation capabilities, specifically a U-net++[52] variation, has been utilized for the lamellar segmentation task, as illustrated in Figure 4A. Based on the results of lamellar structure segmentation, the widths of the lamellar structures have been quantified, as demonstrated in Figure 4B. This was achieved by determining the minimum bounding rectangle for each lamellar region within the smaller images. The width of each lamellar region (represented by the length of the green line segment) and the count of lamellar structures (indicated by the number of black lines intersected by the green line segment) were computed. It is important to note that due to the presence of multiple lamellar regions within each smaller image, the width of lamellar structures was calculated separately for each region, and the average value was considered as the lamellar width within the respective smaller image. In the case of BCC phase proportion estimation, the original image was first transformed into a grayscale representation. Subsequently, a binary image was created through a thresholding procedure[53], enabling the quantification of the BCC phase’s respective proportion (represented by the black regions in the binary image), as depicted in Figure 4C. The comprehensive distribution of these three microstructural characteristics is illustrated in Figure 4D-F. The proportion of lamellar structures is relatively low on the far right, with some degree of fluctuation in other parts. Lamellar width is higher at both ends and lower in the middle. The BCC phase exhibits a distinct feature of being higher in the middle and lower at both ends. The x and y axes in the distribution graph are the corresponding dimensions of the sample, allowing for a direct comparison with real measurements. This approach facilitates direct observation of the distribution of these microstructural features across different regions, thereby aiding subsequent analyses.
Figure 4. Visualization and analysis of microstructures in cross-scale images. (A) The portion enclosed by green lines represents segmented lamellar components. (B) Lamellar widths are calculated based on the lamellar segmentation results. (C) Image binarization; black regions denote the BCC phase. (D) Distribution of lamellar structures. (E) Distribution of lamellar structure widths. (F) Distribution of BCC phase composition.
Subsequently, we quantified the microstructural information at corresponding positions. This information was condensed into one dimension by averaging along the y-axis, as illustrated in Figure 5A-C. The overall distribution of lamellar width ranges from 550 to 750 nanometers, predominantly concentrated around 600 nanometers. The overall distribution of the lamellar structure proportion ranges from 15% to 33%, with a main concentration near 26%. The distribution of the BCC phase ranges from 33% to 35%, predominantly centered around 34.5%, in accord with the phase fraction reported in the literature[54,55]. To evaluate the local mechanical properties varying with the structural features, nanoindentation experiments, a powerful method to investigate the surface mechanical properties, were carried out along the lateral x-axis to determine the hardness of small volume with small load and small tip size[56-58]. As depicted in Figure 5D, the hardness changes with the length along the x-axis, initially increasing from 450 to 550 HV and subsequently descending back to approximately 450 HV. Maximum harness is achieved in the range of 1.1-1.5 cm, that is, the middle section of the ingot with a smaller lamella width of 600 nanometers, lamella content of 26% and maximum BCC content of 35%. Based on the data from Figure 5A-C, we calculated the Pearson correlation coefficients between hardness and each of the three microstructural characteristics, resulting in values of
DISCUSSION
One of the key points of our work is the denoising model trained from 100 pairs of high-low definition images. However, taking pairs of high-low definition images has complicated post-processing operations such as image alignment, which will limit the generalization of CEMI. In addition, we found that many electron microscopy laboratories have accumulated a lot of HD SEM images, but there are no corresponding LD images. To address the above situation, we explored the feasibility of using only HD images, and the LD images are generated by degradation model, and then used for denoising model training. The relationship between HD and LD images can be modeled using Equation (1), where x represents the LD image, y represents the existing HD image, k is the blur kernel, r is the downscaling factor, and n is the noise. The degradation model is complex and irreversible. Although classic degradation models can represent the degradation process, directly using them to generate LD images leads to limited diversity in the generated LD images. Inspired by Real-ESRGAN[13], we mix the degradation processes, as given in Equation (2), randomly combining blur, down-sampling, and noise operations twice, with the possibility of skipping each step (For further details, please refer to the Section "Methods"). The model trained using the degradation data is referred to as the “synthetic model”, while the one trained with paired images is termed the “pairs model”. As shown in Figure 6, the synthetic model effectively recovers primary textural details, but it may overlook smaller black point-like areas. The Learned Perceptual Image Patch Similarity (LPIPS)[59] values for the synthetic and pairs models vs. the HD image in the testing dataset are 0.3363 and 0.2772, respectively. Lower LPIPS values indicate greater similarity between two images, while higher values indicate greater dissimilarity. While there is indeed some difference between the synthetic and pairs models, the synthetic model remains suitable for the denoising module in CEMI. We replicated the process illustrated in Figure 1 using the synthetic model and conducted a statistical analysis of the BCC phase. As shown in Supplementary Figure 3, despite differences in values, the overall distribution trend is very similar to that in Figure 5C. The suitability of the synthetic model in CEMI is because subsequent applications and analyses primarily focus on the texture structure of the images rather than fine-grained pixel-level differences. After image processing, we calculated the average LPIPS between 100 HD images and the processed versions from the original LD images, resulting in 0.119. Our LPIPS score of 0.119 demonstrates the authenticity of the processed images, aligning with results from other image-denoising studies[60,61], where tested methods achieved LPIPS scores ranging from 0.1 to 0.17 and 0.08 to 0.287.
Figure 6. Comparison of denoising results. The performance of SEMGAN trained on real paired data and degradation data is demonstrated. The “Pairs” section shows the results obtained with real paired data, while the “Synthetic” section presents the results obtained with degradation data.
In addition to the alloy materials studied in this work, CEMI can also be used to generate cross-scale images for other types of materials, such as thermoelectrics. We synthesized a series of gradient materials
Figure 7. Stitched image of PbSe-SrSe and distribution of Sr-rich phase. (A) The Electron Microscopy Image Stitching of PbSe-SrSe. It has a size of 9,096 × 608 pixels and a physical size of 2,165.75 μm × 145.3 μm. (B) The distribution of Sr-rich phase.
In this research, we have developed a cross-scale electron microscope image generation system based on computer vision techniques. Through the utilization of video frame extraction, denoising models (based on 100 pairs of LD and HD images), and image stitching, we have successfully generated electron microscope images ranging from centimeter to submicrometer scales. The overall function of CEMI is to generate cross-scale images, which can be applied when the horizontal axis has a meaningful physical scale, such as in the case of gradient structural materials. On the other hand, each module of CEMI can also be used individually to address the issue of material structural heterogeneity. For instance, one can directly capture a certain number of LD images, apply the denoising module in CEMI, and then perform image stitching to obtain the cross-scale image containing micrometer-level microstructures. We then explore methods for cross-scale image analysis using an image segmentation model. Three different microstructures are examined, and the proportion of the BCC phase and the width of lamellar structures significantly influence hardness. The primary benefit of CEMI is its ability to rapidly and automatically generate cross-scale images with spatial distribution, which is of great significance in materials science research. Understanding the structure and properties of materials at different scales is crucial for developing new materials and improving existing ones. This technology aids in identifying and analyzing material defects and studying the effects of processing and treatment on material performance. Furthermore, its modular design ensures that CEMI can be continuously improved by incorporating the latest denoising, stitching, and segmentation techniques as deep learning technology advances.
METHODS
Materials preparing
The AlCoCrFeNi2.1 EHEA was prepared via arc melting high purity elements with purity > 99.99% in argon atmosphere[63,64]. Briefly, the metal blocks were mechanically ground to remove the surface oxide layer, cleaned by anhydrous ethanol solutions within an ultrasonic cleaning machine, and then dried with cold air before weighing. The alloy elements were sequentially placed in a crucible within the furnace based on their melting points. High-purity argon gas was adopted for purging and protecting before and during manufacturing. The melting current was set to about 150 A.
Sample polishing
The AlCoCrFeNi2.1 EHEA ingot was cut as shown by the schematic in Supplementary Figure 4A. Prior to the microstructural characterizations, the ingot was respectively ground with 1,000, 2,000, 3,000, 5,000, and 7,000 grit SiC papers. The polishing process continued until all unidirectional scratches on the surface became invisible. Subsequently, vibration polishing was performed using a VibroMet2 vibration polishing device from Buehler, USA, operating at 20% power for approximately 5 to 10 min. Finally, the AlCoCrFeNi2.1 EHEA specimen was ultrasonicated in ethanol, and dried in air to acquire clean and fresh surfaces, as shown in Supplementary Figure 4B.
Image datasets and video shooting
Microstructural heterogeneity of the AlCoCrFeNi2.1 EHEA specimen was characterized within a field-emission scanning electron microscope (SEM, model G300, Carl Zeiss, Germany) at 500× magnification, with a 60 μm aperture size, contrast information set at 49.5%, and brightness at 68.8%. The backscattered electron detection (BSD) mode was utilized, and low, HD image data shots of the training set (containing 100 LD images and 100 HD images) were captured using Pixel Avg mode with a scan speed of 5
Nanoindentation experiment
The microhardness of AlCoCrFeNi2.1 EHEA was assessed using a nanoindentation instrument (model iMicro, KLA), with maximum indentation depth of 5,000 nm, and maximum load of 1 N. To ensure accurate results, a minimum of three indentations were made at specific x-position, with sufficient distance between each indentation to avoid overlapping effects.
Denoising model
We employed an adversarial neural network approach, where the generator’s detailed structure is depicted in the provided diagram [Supplementary Figure 5]. The discriminator utilized a U-Net architecture. During training, the upsampling module had a magnification factor of 1, ensuring consistent input and output sizes. For the SEMNET training stage, we employed L1Loss, and for training SEMGAN, we initialized the parameters using the pre-trained SEMNET model. The loss function comprised adversarial loss, content perception loss, and L1 loss[14].
Image degradation
In our study, we employed Gaussian noise and Poisson noise with probabilities of 0.5 each to obtain LD images from the HD counterparts. The noise sigma range was set between 1 and 30, while the Poisson noise scale ranged from 0.05 to 3. For the second degradation process, the noise sigma range was adjusted to 1-25, and the Poisson noise scale was set between 0.05 and 2.5.
Image stitching
To merge the captured images seamlessly, we utilized PanoramaStudio 3.6.7 Pro for the image stitching process.
Image segmentation model
Our segmentation model was trained using a dataset of 21 HD images for training and three for validation. We adopted the U-net++ architecture with the se_resnext50_32x4d encoder for the segmentation model.
Training details
We incorporated transfer learning in our denoising model training. Initially, we fine-tuned the SEMNET model based on the pre-trained ESRGAN[38] model to achieve faster convergence. Subsequently, we trained the SEMGAN model based on the improved SEMNET. During training, the batch size was set to 18, and we utilized Adam[66] optimizer with a learning rate of 1e-4. All models were trained for a total of 40,000 iterations. For implementation details not mentioned in the paper, we followed the guidelines provided by the ESRGAN[38]; this module is developed based on the BasicSR[67] framework. The segmentation model employed a pre-trained model based on the imagenet[68] dataset and utilized Dice Loss[69] as the loss function. The optimizer used was Adam with a learning rate of 0.0001, and the training was conducted for 80 epochs. All training of deep neural networks was performed on a machine equipped with an NVIDIA RTX 3090 GPU.
DECLARATIONS
Acknowledgments
This work is supported by the Shanghai Technical Service Center of Science and Engineering Computing, Shanghai University. Jiang, Y. acknowledges the support from the Hefei Advanced Computing Center and Shanghai Engineering Research Center for Integrated Circuits and Advanced Display Materials. Jiang, Y. acknowledges the support of Dr. Shi PJ at Shanghai University for the alloy preparation.
Authors’ contributions
Writing - review & editing, writing - original draft, visualization, validation, methodology, investigation, formal analysis, software, data curation: Lang, Z.
Writing - review & editing, writing - original draft, methodology, visualization, validation, software, investigation, formal analysis, data curation, conceptualization: Zhang, Z.
Data curation, writing - original draft, methodology: Wang, L.
Data curation: Liu, Y.; Qian, W.; Zhou, S.
Resources: Zhang, J.
Supervision, resources, project administration, methodology, investigation, formal analysis, data curation, conceptualization: Jiang, Y.
Supervision: Zhang, T.
writing - review & editing, supervision, resources, project administration, methodology, investigation, funding acquisition, formal analysis, data curation, conceptualization: Yang, J.
Availability of data and materials
Python source codes are available at https://github.com/lzy24601/CEMI. Other data that support the findings of this study are available in the Supplementary Materials of this article.
Financial support and sponsorship
This work was supported by the National Natural Science Foundation of China (Grant Nos. 52172216 and 92163212).
Conflicts of interest
All authors declared that there are no conflicts of interest.
Ethical approval and consent to participate
Not applicable.
Consent for publication
Not applicable.
Copyright
© The Author(s) 2025.
Supplementary Materials
REFERENCES
1. Li, X.; Wei, Y.; Lu, L.; Lu, K.; Gao, H. Dislocation nucleation governed softening and maximum strength in nano-twinned metals. Nature 2010, 464, 877-80.
2. Shi, P.; Li, R.; Li, Y.; et al. Hierarchical crack buffering triples ductility in eutectic herringbone high-entropy alloys. Science 2021, 373, 912-8.
3. Mu, Y.; He, L.; Deng, S.; et al. A high-entropy alloy with dislocation-precipitate skeleton for ultrastrength and ductility. Acta. Mater. 2022, 232, 117975.
4. Nie, J.; Wei, L.; Li, D.; Zhao, L.; Jiang, Y.; Li, Q. High-throughput characterization of microstructure and corrosion behavior of additively manufactured SS316L-SS431 graded material. Addit. Manuf. 2020, 35, 101295.
5. Pegues, J. W.; Melia, M. A.; Puckett, R.; Whetten, S. R.; Argibay, N.; Kustas, A. B. Exploring additive manufacturing as a high-throughput screening tool for multiphase high entropy alloys. Addit. Manuf. 2021, 37, 101598.
6. Fang, T. H.; Li, W. L.; Tao, N. R.; Lu, K. Revealing extraordinary intrinsic tensile plasticity in gradient nano-grained copper. Science 2011, 331, 1587-90.
7. Cheng, Z.; Zhou, H.; Lu, Q.; Gao, H.; Lu, L. Extra strengthening and work hardening in gradient nanotwinned metals. Science 2018, 362, eaau1925.
8. Kelly, S. M.; Kampe, S. L. Microstructural evolution in laser-deposited multilayer Ti-6Al-4V builds: Part II. Thermal modeling. Metall. Mater. Trans. A. 2004, 35, 1869-79.
9. Kok, Y.; Tan, X.; Wang, P.; et al. Anisotropy and heterogeneity of microstructure and mechanical properties in metal additive manufacturing: a critical review. Mater. Des. 2018, 139, 565-86.
10. Wang, Z.; Wang, L.; Duan, S.; Li, Y. An image denoising method based on deep residual GAN. J. Phys. Conf. Ser. 2020, 1550, 032127.
11. Singla, K.; Pandey, R.; Ghanekar, U. A review on single image super resolution techniques using generative adversarial network. Optik 2022, 266, 169607.
12. Yang, W.; Zhang, X.; Tian, Y.; Wang, W.; Xue, J.; Liao, Q. Deep learning for single image super-resolution: a brief review. IEEE. Trans. Multimedia. 2019, 21, 3106-21.
13. Chauhan, K.; Patel, S. N.; Kumhar, M.; et al. Deep learning-based single-image super-resolution: a comprehensive review. IEEE. Access. 2023, 11, 21811-30.
14. Chen, H.; He, X.; Qing, L.; et al. Real-world single image super-resolution: a brief review. Inform. Fusion. 2022, 79, 124-45.
15. Li, K.; Yang, S.; Dong, R.; Wang, X.; Huang, J. Survey of single image super-resolution reconstruction. IET. Image. Process. 2020, 14, 2273-90.
16. Lepcha, D. C.; Goyal, B.; Dogra, A.; Goyal, V. Image super-resolution: a comprehensive review, recent trends, challenges and applications. Inform. Fusion. 2023, 91, 230-60.
17. Dong, C.; Loy, C. C.; He, K.; Tang, X. Image super-resolution using deep convolutional networks. IEEE. Trans. Pattern. Anal. Mach. Intell. 2016, 38, 295-307.
18. Kim, J.; Lee, J. K.; Lee, K. M. Accurate image super-resolution using very deep convolutional networks. In Proceedings of the 2016 IEEE Conference on Computer Vision and Pattern Recognition (CVPR); 27-30 June 2016; Las Vegas, NV, USA.
19. Ledig, C.; Theis, L.; Huszár, F.; Caballero, J.; Cunningham, A.; Acosta, A. Photo-realistic single image super-resolution using a generative adversarial network. In Proceedings of the 2017 IEEE Conference on Computer Vision and Pattern Recognition (CVPR); 21-26 July 2017; Honolulu, HI, USA.
20. Zhang, Y.; Li, K.; Li, K.; Wang, L.; Zhong, B.; Fu, Y. Image super-resolution using very deep residual channel attention networks. In: Ferrari V, Hebert M, Sminchisescu C, Weiss Y, editors, Computer Vision - ECCV 2018, Lecture Notes in Computer Science. Cham: Springer International Publishing; 2018. pp. 294-310.
21. Liang, J.; Cao, J.; Sun, G.; Zhang, K.; Van, G. L.; Timofte, R. SwinIR: image restoration using swin transformer. In Proceedings of the 2021 IEEE/CVF International Conference on Computer Vision Workshops (ICCVW); 11-17 October 2021; Montreal, BC, Canada.
22. Zhang, S.; Liang, G.; Pan, S.; Zheng, L. A fast medical image super resolution method based on deep learning network. IEEE. Access. 2019, 7, 12319-27.
23. Li, Y.; Sixou, B.; Peyrin, F. A review of the deep learning methods for medical images super resolution problems. IRBM. 2021, 42, 120-33.
24. Sood, R.; Topiwala, B.; Choutagunta, K.; Sood, R.; Rusu, M. An application of generative adversarial networks for super resolution medical imaging. In Proceedings of the 2018 17th IEEE International Conference on Machine Learning and Applications (ICMLA); 17-20 December 2018; Orlando, FL, USA.
25. Liu, H.; Xu, J.; Wu, Y.; Guo, Q.; Ibragimov, B.; Xing, L. Learning deconvolutional deep neural network for high resolution medical image reconstruction. Inf. Sci. 2018, 468, 142-54.
26. Wang, P.; Bayram, B.; Sertel, E. A comprehensive review on deep learning based remote sensing image super-resolution methods. Earth-Sci. Rev. 2022, 232, 104110.
27. Li, Z.; Shen, H.; Cheng, Q.; Liu, Y.; You, S.; He, Z. Deep learning based cloud detection for medium and high resolution remote sensing images of different sensors. ISPRS. J. Photogramm. Remote. Sens. 2019, 150, 197-212.
28. Lei, S.; Shi, Z.; Zou, Z. Super-resolution for remote sensing images via local-global combined network. IEEE. Geosci. Remote. Sensing. Lett. 2017, 14, 1243-7.
29. Furat, O.; Finegan, D. P.; Yang, Z.; Kirstein, T.; Smith, K.; Schmidt, V. Super-resolving microscopy images of Li-ion electrodes for fine-feature quantification using generative adversarial networks. NPJ. Comput. Mater. 2022, 8, 749.
30. Jangid, D. K.; Brodnik, N. R.; Goebel, M. G.; et al. Adaptable physics-based super-resolution for electron backscatter diffraction maps. NPJ. Comput. Mater. 2022, 8, 924.
31. Yin, W.; Brittain, D.; Borseth, J.; et al. A petascale automated imaging pipeline for mapping neuronal circuits with high-throughput transmission electron microscopy. Nat. Commun. 2020, 11, 4949.
32. Ma, B.; Ban, X.; Huang, H.; et al. A fast algorithm for material image sequential stitching. Comput. Mater. Sci. 2019, 158, 1-13.
33. Yang, F.; Deng, Z. S.; Fan, Q. H. A method for fast automated microscope image stitching. Micron 2013, 48, 17-25.
34. Kaynig, V.; Fischer, B.; Müller, E.; Buhmann, J. M. Fully automatic stitching and distortion correction of transmission electron microscope images. J. Struct. Biol. 2010, 171, 163-73.
35. Chalfoun, J.; Majurski, M.; Blattner, T.; et al. MIST: accurate and scalable microscopy image stitching tool with stage modeling and error minimization. Sci. Rep. 2017, 7, 4988.
36. Singla, A.; Lippmann, B.; Graeb, H. Recovery of 2D and 3D layout information through an advanced image stitching algorithm using scanning electron microscope images. In Proceedings of the 2020 25th International Conference on Pattern Recognition (ICPR); 10-15 January 2021; Milan, Italy.
37. Ma, B.; Zimmermann, T.; Rohde, M.; et al. Use of autostitch for automatic stitching of microscope images. Micron 2007, 38, 492-9.
38. Wang, X.; Yu, K.; Wu, S.; et al. ESRGAN: enhanced super-resolution generative adversarial networks. In: Leal-Taixé L, Roth S, editors, Computer Vision - ECCV 2018 Workshops, Lecture Notes in Computer Science. Cham: Springer International Publishing; 2019. pp. 63-79.
39. Goodfellow, L. J.; Pouget-Abadie, J.; Mirza, M.; et al. Generative adversarial nets. Available from: https://papers.nips.cc/paper_files/paper/2014/file/5ca3e9b122f61f8f06494c97b1afccf3-Paper.pdf [Last accessed on 3 Jan 2025].
40. Ronneberger, O.; Fischer, P.; Brox, T. U-net: convolutional networks for biomedical image segmentation. In: Navab N, Hornegger J, Wells WM, Frangi AF, editors, Medical Image Computing and Computer-Assisted Intervention – MICCAI 2015, Lecture Notes in Computer Science. Cham: Springer International Publishing; 2015. pp. 234-41.
41. Miyato, T.; Kataoka, T.; Koyama, M.; Yoshida, Y. Spectral normalization for generative adversarial networks. In Proceedings of the International Conference on Learning Representations. arXiv2018.
42. Lu, Y.; Dong, Y.; Guo, S.; et al. A promising new class of high-temperature alloys: eutectic high-entropy alloys. Sci. Rep. 2014, 4, 6200.
43. Gao, X.; Lu, Y.; Zhang, B.; et al. Microstructural origins of high strength and high ductility in an AlCoCrFeNi2.1 eutectic high-entropy alloy. Acta. Mater. 2017, 141, 59-66.
44. Yan, P.; Chang, J.; Wang, W.; Zhu, X.; Lin, M.; Wei, B. Eutectic growth kinetics and microscopic mechanical properties of rapidly solidified CoCrFeNiMo0.8 high entropy alloy. Acta. Mater. 2022, 237, 118149.
45. Vikram, R.; Gupta, K.; Suwas, S. Design of a new cobalt base nano-lamellar eutectic high entropy alloy. Scr. Mater. 2021, 202, 113993.
46. Wischi, M.; Campo, K.; Starck, L.; da, F. E.; Lopes, É.; Caram, R. Microstructure and mechanical behavior of the directionally solidified AlCoCrFeNi2.1 eutectic high-entropy alloy. J. Mater. Res. Technol. 2022, 20, 811-20.
47. Shelhamer, E.; Long, J.; Darrell, T. Fully convolutional networks for semantic segmentation. IEEE. Trans. Pattern. Anal. Mach. Intell. 2017, 39, 640-51.
48. He, K.; Gkioxari, G.; Dollar, P.; Girshick, R. Mask R-CNN. In Proceedings of the 2017 IEEE International Conference on Computer Vision (ICCV); 22-29 October 2017; Venice, Italy.
49. Badrinarayanan, V.; Kendall, A.; Cipolla, R. SegNet: a deep convolutional encoder-decoder architecture for image segmentation. IEEE. Trans. Pattern. Anal. Mach. Intell. 2017, 39, 2481-95.
50. Wen, Q.; Yang, J.; Yang, X.; Liang, K. PatchDCT: patch refinement for high quality instance segmentation. arXiv 2023.
51. Bochkovskiy, A.; Wang, C. Y.; Liao, H. Y. M. YOLOv4: optimal speed and accuracy of object detection. arXiv 2024.
52. Zhou, Z.; Rahman, S. M. M.; Tajbakhsh, N.; Liang, J. UNet++: a nested U-net architecture for medical image segmentation. In: Stoyanov D, Taylor Z, Carneiro G, et al., editors. Deep learning in medical image analysis and multimodal learning for clinical decision support. Cham: Springer International Publishing; 2018. pp. 3-11.
53. Bradley, D.; Roth, G. Adaptive thresholding using the integral image. J. Grap. Tools. 2007, 12, 13-21.
54. Wani, I. S.; Bhattacharjee, T.; Sheikh, S.; et al. Ultrafine-grained AlCoCrFeNi2.1 eutectic high-entropy alloy. Mater. Res. Lett. 2016, 4, 174-9.
55. Nassar, A.; Mullis, A.; Cochrane, R.; Aslam, Z.; Micklethwaite, S.; Cao, L. Rapid solidification of AlCoCrFeNi2.1 high-entropy alloy. J. Alloys. Compd. 2022, 900, 163350.
57. Karimzadeh, A.; Koloor, S. S. R.; Ayatollahi, M. R.; Bushroa, A. R.; Yahya, M. Y. Assessment of nano-indentation method in mechanical characterization of heterogeneous nanocomposite materials using experimental and computational approaches. Sci. Rep. 2019, 9, 15763.
58. Miller, M.; Bobko, C.; Vandamme, M.; Ulm, F. Surface roughness criteria for cement paste nanoindentation. Cement. Concrete. Res. 2008, 38, 467-76.
59. Zhang, R.; Isola, P.; Efros, A. A.; Shechtman, E.; Wang, O. The unreasonable effectiveness of deep features as a perceptual metric. In Proceedings of the 2018 IEEE/CVF Conference on Computer Vision and Pattern Recognition; 18-23 June 2018; Salt Lake City, UT, USA.
60. Jiang, K.; Wang, R.; Xiao, Y.; Jiang, J.; Xu, X.; Lu, T. Image enhancement via associated perturbation removal and texture reconstruction learning. IEEE/CAA. J. Autom. Sin. 2024, 11, 2253-69.
61. Tian, C.; Zheng, M.; Lin, C.; Li, Z.; Zhang, D. Heterogeneous window transformer for image denoising. IEEE. Trans. Syst. Man. Cybern. Syst. 2024, 54, 6621-32.
62. He, S.; Yang, Y.; Li, Z.; et al. A general strategy for high-throughput experimental screening of promising bulk thermoelectric materials. Sci. China. Mater. 2021, 64, 1751-60.
63. Shi, P.; Zhong, Y.; Li, Y.; et al. Multistage work hardening assisted by multi-type twinning in ultrafine-grained heterostructural eutectic high-entropy alloys. Mater. Today. 2020, 41, 62-71.
64. Shi, P.; Ren, W.; Zheng, T.; et al. Enhanced strength-ductility synergy in ultrafine-grained eutectic high-entropy alloys by inheriting microstructural lamellae. Nat. Commun. 2019, 10, 489.
65. Wada, K.; Mpitid; Buijs, M. wkentaro/labelme: v4.6.0. Available from: https://zenodo.org/records/5711226 [Last accessed on 21 Jan 2025].
67. BasicSR: open source image and video restoration toolbox; 2022. Available from: https://github.com/XPixelGroup/BasicSR [Last accessed on 3 Jan 2025].
68. Russakovsky, O.; Deng, J.; Su, H.; et al. ImageNet large scale visual recognition challenge. Int. J. Comput. Vis. 2015, 115, 211-52.
Cite This Article
How to Cite
Download Citation
Export Citation File:
Type of Import
Tips on Downloading Citation
Citation Manager File Format
Type of Import
Direct Import: When the Direct Import option is selected (the default state), a dialogue box will give you the option to Save or Open the downloaded citation data. Choosing Open will either launch your citation manager or give you a choice of applications with which to use the metadata. The Save option saves the file locally for later use.
Indirect Import: When the Indirect Import option is selected, the metadata is displayed and may be copied and pasted as needed.
About This Article
Special Issue
Copyright
Data & Comments
Data
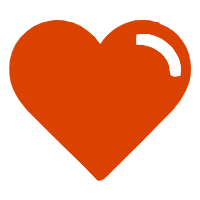
Comments
Comments must be written in English. Spam, offensive content, impersonation, and private information will not be permitted. If any comment is reported and identified as inappropriate content by OAE staff, the comment will be removed without notice. If you have any queries or need any help, please contact us at [email protected].