Transformative strategies in photocatalyst design: merging computational methods and deep learning
Abstract
Photocatalysis is a unique technology that harnesses solar energy through in-situ processes, operating without the need for external energy inputs. It is integral to advancing environmental, energy, chemical, and carbon-neutral objectives, promoting the dual goals of pollution control and carbon reduction. However, the conventional approach to photocatalyst design faces challenges such as inefficiency, high costs, and low success rates, highlighting the need for integrating modern technologies and seeking new paradigms. Here, we demonstrate a comprehensive overview of transformative strategies in photocatalyst design, combining computational materials science with deep learning technologies. The review covers the fundamental principles of photocatalyst design, followed by a comprehensive examination of computational methods and the workflow for deep-learning-assisted design. Deep learning approaches are extensively reviewed, focusing on the discovery of novel photocatalysts, microstructure design, property optimization, novel design approaches, application exploration, and mechanistic insights into photocatalysis. Finally, we highlight the synergy between multidimensional computation and deep learning, while discussing the challenges and future directions in photocatalyst development. This review offers a comprehensive summary of deep-learning-assisted photocatalyst design, offering transformative insights that not only enhance the development of photocatalytic technologies but also expand the practical applications of photocatalysis in various domains.
Keywords
INTRODUCTION
Photocatalysis offers a unique advantage by directly harnessing solar energy through in-situ processes[1], making it a highly sustainable technology that operates without external energy inputs[2]. By utilizing abundant and renewable sunlight, photocatalytic reactions significantly reduce reliance on fossil fuels and minimize greenhouse gas emissions[3]. One of the major environmental benefits of this technology is its ability to degrade a wide range of pollutants, such as organic compounds[4,5] and heavy metals[6], thus promoting cleaner ecosystems. In the energy domain, it plays a pivotal role in solar fuel generation, particularly through hydrogen production via water splitting, offering a clean and renewable energy source[7-9]. Additionally, it enhances chemical processes by enabling selective reactions with greater efficiency, reducing the need for hazardous chemicals and lowering operational costs[10]. With the global focus on carbon neutrality, photocatalysis contributes to carbon reduction efforts by converting CO2 into valuable fuels and chemicals, thus advancing the circular carbon economy[11]. The key to unlocking the full potential of this technology lies in the design of advanced photocatalysts, which optimize light absorption, charge separation, and photocatalytic efficiency.
Traditional approaches to photocatalyst design are fraught with inefficiencies, high costs, and low success rates, which significantly hinder progress in this field[12]. These methods often depend on laborious trial-and-error experimentation[13-15], consuming substantial time and resources while offering limited predictive power for real-world applications. Key photocatalytic properties, such as charge separation efficiency[16] and reaction kinetics[17,18], remain difficult to optimize, resulting in suboptimal performance and limited advancement. The high costs associated with synthesizing and characterizing photocatalysts further compound these issues, limiting scalability and delaying the transition from laboratory research to industrial implementation[19]. Surface reactivity, adsorption dynamics, and charge transfer mechanisms[20,21] are not well understood through conventional methods, hindering systematic improvements in reactivity and stability. Consequently, many photocatalysts designed through traditional approaches fail to achieve the required durability, selectivity, and efficiency needed for practical applications in energy conversion and environmental remediation. To overcome these challenges, there is an urgent need to adopt advanced methodologies, such as computational modeling and deep learning, to develop new paradigms that can accelerate the discovery process and enhance the overall performance of photocatalytic systems.
The rise of computational science and deep learning has revolutionized materials design by offering predictive power, accelerated discovery, and improved optimization capabilities[22]. Advanced computational techniques, such as density functional theory (DFT)[23,24] and molecular dynamics[25], offer precise insights into electronic structures and reaction mechanisms that are challenging to capture through experimental methods alone. High-throughput screening (HTS) enables the evaluation of vast libraries of potential photocatalysts, facilitating the identification of promising candidates based on theoretical performance metrics before engaging in resource-intensive experimental validation[26]. Deep learning algorithms[27], trained on extensive datasets of material properties and photocatalytic performance, reveal intricate correlations between structural characteristics and photocatalytic activity, providing a data-driven approach to more effective photocatalyst design. It further enables the prediction of photocatalyst behavior under diverse environmental conditions, thereby advancing the development of durable and high-performance photocatalysts for real-world applications. The integration of machine learning with computational methodologies establishes a dynamic and iterative framework, allowing continuous refinement and optimization of photocatalytic systems. This integrated approach of computational modeling and deep learning accelerates the design process and significantly elevates photocatalyst performance, addressing the fundamental limitations of traditional methodologies.
Here, this review presents a transformative approach to photocatalyst design by integrating computational science with deep learning technologies. Fundamental principles of photocatalysis are explored, offering a comprehensive foundation for understanding the key factors that drive efficiency in photocatalytic systems. A detailed examination of computational methodologies follows, focusing on first-principles calculations and HTS, which play a critical role in optimizing photocatalytic performance and accelerating the discovery of efficient photocatalysts. The workflow for deep-learning-assisted photocatalyst design is outlined, emphasizing how data collection, feature engineering, model training, and validation are integrated with machine learning models to guide experimental validation and enhance predictive capabilities. Deep learning approaches are thoroughly investigated, with attention to the discovery of novel photocatalysts, microstructural design, property optimization, innovative design frameworks, application exploration, and mechanistic insights into photocatalysis. The synergy between multidimensional computations and deep learning is examined, demonstrating how this integrated framework addresses the inherent challenges of traditional design and fosters breakthroughs in photocatalyst development. This review offers transformative insights that not only advance the design of high-performance photocatalysts but also expand their practical applications across various fields, contributing to the broader goals of environmental sustainability and energy efficiency.
DESIGN OF PHOTOCATALYSTS
General principle of photocatalysis
Photocatalysis has emerged as a crucial technology with applications spanning environmental remediation, energy conversion, and sustainable chemical production[28]. Its significance lies in its ability to harness solar energy to drive chemical reactions, contributing to pollution reduction, carbon neutrality, and green energy generation. One of the primary advantages of photocatalysis is its ability to operate in-situ without the need for external energy inputs, relying solely on solar energy[29]. For example, in environmental remediation, photocatalysis has been extensively demonstrated under natural sunlight conditions[30]. Furthermore, it supports simultaneous pollution reduction and carbon mitigation[31]. This is evidenced by the photocatalytic reduction of CO2 into useful fuels or chemicals, promoting both environmental sustainability and carbon neutrality[32].
The fundamental mechanism[33-35] of photocatalysis involves the excitation of electrons within a semiconductor upon absorption of photons with energy equal to or greater than its band gap. This excitation promotes electrons from the valence to the conduction band, leaving corresponding holes in the valence band. The photogenerated charge carriers (electrons and holes) then migrate to the surface, where they participate in redox reactions. Electrons predominantly participate in reduction reactions, where they are transferred to oxygen molecules (O2) adsorbed on the photocatalyst surface, leading to the formation of superoxide radicals (•O2-). These superoxide radicals can subsequently react with protons to produce hydrogen peroxide (H2O2) or other reactive oxygen species (ROS), which are highly effective in degrading organic pollutants and reducing heavy metals. Conversely, the holes (h+) facilitate oxidation reactions by oxidizing water (H2O) molecules adsorbed on the photocatalyst surface, generating hydroxyl radicals
Figure 1. Fundamental mechanism of photocatalysis[37]. Copyright 2022, Elsevier.
The core of photocatalysis lies in the photocatalysts. Traditional photocatalysts are typically bulk semiconductors[38] that harness light to drive photocatalytic reactions, but they often suffer from low efficiency due to limited surface area and rapid recombination of charge carriers. Nanostructured photocatalysts[39], on the other hand, offer significantly enhanced performance due to their increased surface area and improved charge carrier dynamics, which help reduce recombination and enhance photocatalytic activity. Heterojunction systems[40,41] combine two or more semiconductors with different band structures, creating interfaces that facilitate more efficient charge separation and transfer, thus improving overall efficiency. Low-dimensional systems, such as quantum dots[42], one-dimensional[43], and two-dimensional (2D) structures[44,45], exhibit unique quantum confinement effects that significantly alter their electronic properties. Quantum dots have tunable band gaps due to their nanoscale dimensions, enabling enhanced light absorption and charge separation[46]. One-dimensional structures, including nanowires or nanotubes, provide high charge carrier mobility along their axial direction to improve performances[47]. 2D systems feature high surface-to-volume ratios and exceptional electronic properties, offering more active sites for photocatalytic reactions[48].
The performance of photocatalysts is critically determined by several key characteristics, each influencing different aspects of photocatalytic efficiency. (1) The microstructure, including grain size, specific surface area, and pore size, directly affects the availability of active sites and the overall surface reactivity; (2) Architecture factors, such as quantum dots, nanowires, and heterojunctions, influence light absorption and charge separation, with more advanced structural designs often improving charge transportation; (3) Composition, including stoichiometry, doping elements, and the presence of defects, plays a pivotal role in tuning electronic properties, thereby optimizing light absorption, charge carrier dynamics, and redox processes; (4) The electronic structure, particularly the band gap, density of states (DOS), and electron affinity, dictates the ability to absorb irradiation energy and generate charge carriers, which are essential for driving photocatalytic reactions; (5) Photoelectric properties, such as carrier mobility, carrier lifetime, and quantum yield, determine the effectiveness of converting absorbed light into chemical energy. Higher mobility and longer carrier lifetimes facilitate more efficient charge separation and reduce recombination. Table 1 lists the key characteristics of photocatalysts and their typical characterization techniques.
Key characteristics of photocatalysts and typical characterization techniques
Characteristics | Typical characterization technique | |
Microstructure | Grain size | SEM TEM DLS AFM |
Specific surface area | BET BJH MIP | |
Pore size | BET SAXS | |
Facet | XRD TEM | |
Lattice symmetry | XRD Neutron diffraction | |
Space group | XRD Electron diffraction | |
Architecture | Quantum dot | PL HRTEM AC-TEM |
Nanowire | SEM TEM AFM | |
Single few-layer nanostructure | AFM HRTEM | |
Heterojunction | EELS STEM | |
Composition | Stoichiometry | EDS ICP-MS ICP-OES XPS |
Doping element | XPS EELS | |
Defect | PALS HRTEM | |
Functional group | FTIR XPS SIMS | |
Electronic structure | Band structure | XPS ARPES |
DOS | XPS | |
Electron affinity | UPS | |
Electron spin | ESR EPR XMCD | |
Band gap | UV-Vis PL | |
VBM | XPS UPS | |
CBM | XPS | |
Photoelectric property | Absorption | UV-Vis DRS |
Carrier mobility | Hall effect analysis Seebeck coefficient | |
Carrier lifetime | TRPL | |
Photocurrent density | LSV j-V curve | |
Quantum yield | PL |
Common modification strategies for photocatalysts include doping[49], cocatalyst loading[50] and incorporating single-atom catalysts[51,52]. Latest modification mechanisms, such as defect engineering, band structure engineering, and interfacial engineering, enable precise control at the atomic, electronic, and chemical bond levels, significantly enhancing photocatalytic performance[53,54]. Recently, constructing heterojunctions[55,56] remains a major focus of research, with types including Type I, Type II, Z-scheme, and S-scheme heterojunctions being extensively studied. In particular, S-scheme heterojunctions offer distinct advantages[57,58], such as optimized band alignment and enhanced charge separation, which contribute to superior redox capabilities and reduced electron-hole recombination, making them highly promising for practical applications[59]. For instance, in ribbon-based water purification, these modification strategies have been instrumental in enhancing photocatalytic degradation efficiency by improving charge carrier dynamics, optimizing active sites, and facilitating the breakdown of complex organic pollutants, thereby demonstrating significantly superior performance[60].
Traditional procedure of photocatalyst design
Traditional photocatalyst design has predominantly relied on empirical methods, where photocatalysts are synthesized and evaluated through trial-and-error experimentation. The process typically begins with selecting semiconductors based on properties such as band gap and chemical stability, which indicate potential photocatalytic activity. Techniques such as sol-gel synthesis[61], hydrothermal methods[62], or chemical vapor deposition[63,64] are commonly used to fabricate photocatalysts. After synthesis, thorough characterization is conducted using methods such as transmission electron microscopy (TEM), X-ray photoelectron spectroscopy (XPS), and ultraviolet-visible absorbance spectra (UV-Vis) spectroscopy to assess key features such as morphology, electronic structure, and light absorption properties. The photocatalysts are then tested under controlled laboratory conditions to determine their efficiency in processes such as pollutant degradation or water splitting.
Despite its long-standing use, this traditional approach presents several inherent limitations. The trial-and-error nature of the process is highly time-consuming and resource-intensive, requiring extensive experimentation without a guarantee of success. Additionally, the complexity of photocatalytic mechanisms, such as charge carrier dynamics, surface reactions and photon absorption, cannot be fully understood or optimized using experimental methods alone. As a result, many promising photocatalysts may go undiscovered, and the development of highly efficient systems is often impeded by the lack of predictive capability in traditional design approaches. These limitations have driven growing interest in computational techniques and data-driven methodologies to accelerate the discovery and optimization of photocatalysts[65].
Progress of deep learning in photocatalyst design
Machine learning was first applied in materials science to predict properties and accelerate the discovery of new materials[66,67]. Early models, including decision trees[68] and support vector machines[69], were employed to analyze datasets and detect patterns, thereby improving the efficiency of material screening and optimization processes. The transition from traditional machine learning to deep learning represented a paradigm shift in the field[70], as deep learning, particularly through neural networks, allowed for the extraction of more complex, high-dimensional relationships from large datasets. This development significantly enhanced predictive accuracy and deepened our understanding of the intricate behaviors governing material properties. The integration of deep learning in photocatalyst design has experienced remarkable growth, as evidenced by the sharp increase in publications and citations from 2012 to 2024 [Figure 2A]. This upward trend underscores the expanding role of data-driven approaches in optimizing photocatalyst properties, highlighting the continued momentum of deep learning in transforming materials design. Table 2 summarizes notable research teams worldwide applying deep learning to design photocatalysts, highlighting diverse global efforts that underscore the expanding role of data-driven approaches in advancing photocatalysis.
Figure 2. General description of deep-learning-assisted photocatalyst design. (A) Trends of publications and citations in 2012-2024; (B) Clustering diagram of keywords; (C) Contribution from top 20 journals.
International efforts in deep learning-based photocatalyst design
Researcher | Institution | Country/Region |
Yang Liu, Zhenhui Kang | Soochow University | China P.R. |
Yong-Bin Zhuang, Jun Cheng | Xiamen University | China P.R. |
Yunjin Yu, Yadong Wei | Shenzhen University | China P.R. |
Ting Ren, Xin Li | Institute of Electrical Engineering, CAS | China P.R. |
Jianmei Yuan, Yuliang Mao | Xiangtan University | China P.R. |
Hui-Ming Cheng, Gang Liu | Institute of Metal Research, CAS | China P.R. |
Jinlan Wang | Southeast University | China P.R. |
David A. Winkler, Rachel A. Caruso | Royal Melbourne Institute of Technology | Australia |
Geoffrey A. Ozin | University of Toronto | Canada |
Xiaoying Zhuang, Alexander Shapeev | Leibniz University Hannover | Germany |
Andrew I. Cooper | University of Liverpool | Great Britain |
Zhenhua Pan, Kenji Katayama | Chuo University | Japan |
Alicja Mikolajczyk | Fahrenheit Universities | Poland |
Ramazan Yildirim | Bogazici University | Türkiye |
Xiong Yu | University System of Ohio | United States |
In Figure 2B, the keyword clustering analysis highlights three primary thematic areas in deep-learning-assisted photocatalyst design: methodology, materials, and applications. Prominent keywords such as “Learning”, “Machine”, and “Network” emphasize the critical role of machine learning techniques, particularly neural networks, in optimizing photocatalytic processes. The focus on “band gap” further suggests the integration of deep learning for theoretical predictions and mechanistic insights, supported by computational methods such as “DFT”. In terms of materials, clusters around “TiO2” and “metal oxide” indicate widely studied photocatalysts. Keywords such as “rate” and “density” reflect efforts to optimize material properties, including electronic structure and surface functionalities. Meanwhile, keywords such as “degradation”, “water splitting” and “hydrogen” underscore the key applications of photocatalysis, such as environmental remediation through pollutant degradation and energy conversion via water splitting. The presence of terms such as “hydrogen” and “oxidation” highlights the critical role of photocatalysis in renewable energy generation and environmental sustainability. This categorization illustrates how deep learning fosters innovation across fundamental research and practical applications in photocatalysis.
High-impact journals have been instrumental in shaping the field, with several leading publications contributing significantly to the dissemination of cutting-edge research. As depicted in Figure 2C, Applied Catalysis B-Environment and Energy has emerged as a key platform. Chemical Reviews and ACS Applied Materials and Interfaces have also made substantial contributions, reinforcing the importance of this interdisciplinary approach. Additionally, journals such as Advanced Energy Materials and Journal of the American Chemical Society have been central to promoting advances in deep-learning-enhanced photocatalyst design, further solidifying the role of this technology in the future of materials discovery and development.
The photocatalyst design has seen substantial progress in materials, applications, mechanistic understanding, algorithms, and computility, as shown in Figure 3. Early photocatalysts, such as TiO2 and ZnO, have evolved into more advanced systems, including nanomaterials, heterojunctions, quantum dots, and 1D/2D structures, greatly expanding their functionality. Initial applications focused on environmental remediation, specifically organic pollutant degradation and heavy metal reduction, but have since diversified to include CO2 reduction, hydrogen production, sterilization, and nitrogen fixation, reflecting the growing versatility of photocatalysis in addressing global issues. Mechanistic insights have deepened, allowing precise control over electron-hole pair dynamics, band structures, and surface reactions, which has led to significant improvements in stability and efficiency. Concurrently, advancements in deep learning algorithms have enabled more accurate predictions of photocatalytic behavior, accelerating the discovery and optimization of photocatalytic systems. Additionally, enhanced computility, driven by breakthroughs in GPUs and parallel computing, has allowed researchers to simulate complex processes and manage large datasets, pushing the boundaries of what can be achieved in photocatalyst design. The interplay between deep learning and photocatalyst development is driving rapid innovation, where new machine learning models refine material discovery and mechanistic insights, while advances in photocatalysis provide rich data that fuel further algorithmic evolution. This dynamic feedback is accelerating progress toward the next generation of high-performance photocatalysts, with far-reaching implications for environmental and energy applications.
Figure 3. Progress of deep learning algorithm in photocatalyst design, application and mechanism together with computility development. DNN: Deep Neural Network; CNN: Convolutional Neural Network; GNN: Graph Neural Networks; SVR: support vector regression; RNN: Recurrent Neural Network; LSTM: Long Short-Term Memory; GRU: Gated recurrent unit; GAN: Generative Adversarial Networks; KAN: Kolmogorov-Arnold Network; KNN: K-nearest neighbors; GPR: Gaussian process regression; RFR: random forest regression; FNN: feed-forward neural network; ENIAC: electronic numerical integrator and computer; UNIVAC I: universal automatic computer I; ARM: advanced risc machines; GTX: Giga texel shader eXtreme; RTX: ray tracing texel eXtreme.
COMPUTATIONAL DESIGN OF PHOTOCATALYSTS
First-principles calculation
First-principles calculations are a cornerstone of modern computational materials design, offering insights into the fundamental properties of photocatalysts without relying on empirical parameters[71,72]. Based on quantum mechanical principles, particularly DFT, first-principles methods allow for the accurate prediction of electronic structure, band gaps, and surface reactions, which are critical for understanding photocatalytic activity. By solving the Schrödinger equation for electrons, first-principles calculations describe the behavior of charge carriers, providing detailed information about electron-hole pair dynamics, defect states, and adsorption properties at the atomic level. DFT plays a pivotal role in these first-principles approaches, providing a practical and efficient method to approximate the many-body electron problem. Using DFT, properties such as charge density distribution, adsorption energies, and band structure can be determined with a level of detail that aids in deciphering the reactivity and efficiency of photocatalysts[73]. The use of generalized gradient approximation (GGA)[74,75] or more sophisticated hybrid functionals[76] allows for refined predictions that align well with experimental observations, offering valuable insights that drive the rational design of advanced photocatalysts. Computational approaches to property optimization, such as cocatalyst addition and elemental doping, can be utilized to modify the electronic properties of photocatalysts, including increasing the band gap and enhancing charge separation. These calculated modifications are essential for reducing electron-hole recombination and ultimately improving photocatalytic efficiency, addressing complex design challenges effectively[77].
As listed in Table 3, several widely used software packages are central to these calculations. VASP (Vienna Ab initio Simulation Package), one of the most commonly used tools, integrates projector augmented wave (PAW)/Perdew-Burke-Ernzerh (PBE) modules and hybrid functionals such as HSE06 for more accurate electronic structure calculations. Quantum ESPRESSO, known for its use of the PBE functional and DFT-D2 for dispersion corrections, is frequently employed for band gap and optical absorption predictions. Additionally, Materials Studio, with modules such as CASTEP, offers robust capabilities for calculating electronic properties, including band structure and light absorption coefficients. These software tools, combined with the growing computational power, enable researchers to predict the performance of potential photocatalysts with high precision, guiding experimental validation and accelerating the discovery process. These computational parameters, such as band gap, adsorption energy, and Gibbs free energy, are not only applicable to specific photocatalysts but also have broad applicability across various semiconductors for predicting photocatalytic activity. The universality of these first-principles-derived parameters across different systems provides a solid foundation for the theoretical design of photocatalysts. Through accurate calculations, these parameters help identify candidates with optimal electronic structures, thereby accelerating the development of efficient photocatalysts.
Photocatalyst design based on first-principles calculation
Photocatalyst | Software/Package | Property | Ref. |
Ag- and Cu-based delafossite ABO2 | VASP: GGA+U, PAW | Band structure, DOS, work functions, absorption coefficient | [74] |
SWCNTs/g-C3N4 nanocomposites | VASP: PAW/PBE, DFT-D2, HSE06, TDHF | Band gap, charge transfer, light absorption, Gibbs free energy | [76] |
LDHs/Ti3C2O2 | VASP: PAW, GGA, PBE | Adsorption activation, Gibbs free energy | [78] |
g-C3N4 | Gaussian 16 package | Band structure, HOMO, LUMO, electrostatic potential, DOS | [79] |
GeC monolayer | Quantum ESPRESSO: PBE, DFT-D2 | Band gap, optical absorption | [80] |
Non-metal doped MoSe2 | VASP: PAW, PBE | Band structure, formation energy, redox potential | [81] |
N-doped ZnO | DFT+Dmol3 | Band gap, local density state | [82] |
Nb-doped LiSbO3 | VASP: SCAN, PAW, MBJ | Bandgap, elastic modulus, ferroelectric properties | [83] |
Pt-C/N/P/S-ZrO2 | Materials Studio: CASTEP, GGA, PBE, OTFG, Tkatchenchen-Scheffler, HSE06 | Band structure, HER, free energy, work function, DOS, light absorption | [84] |
M@2DPI | VASP: PAW, PBE, DFT-D3, HSE06 | Band structure, CO2RR, HER | [85] |
Pt-non-metal-doped WS2 | VASP: PAW, PBE, DFT-D3, HSE06 | Band structure, Gibbs free energy, CO2RR | [86] |
Mo-SnO2 | Materials Studio: CASTEP, GGA, PBE | Band structure, DOS, absorption coefficient | [87] |
Co-doped g-C3N4 | VASP: PAW, PBE, GGA | Band structure, charge density difference, optical absorption | [88] |
SiC/WS2 | Materials Studio: meta-GGA SCAN, CASTEP, HSE06 | Band structure, effective mass, optical property | [89] |
C4N/MoS2 | VASP: PAW, PBE, GGA, DFT-D2 | Band structure, charge density difference, optical absorption, electrostatic potential | [90] |
Table 3 also lists some classical works of photocatalyst designs based on first-principles calculation. One notable example exhibited in Figure 4A is the work by Shen et al., where DFT calculations played a pivotal role in designing a defective ZnTi-LDHs/Ti3C2O2 photocatalyst aimed at improving the selectivity for C2 organics in the photocatalytic reduction of CO2[78]. The DFT simulations revealed that the formation of a Schottky junction significantly enhanced charge separation and increased the concentration of photogenerated electrons on the photocatalyst surface, while oxygen vacancies facilitated CO2 adsorption and activation by lowering the Gibbs free energy barrier for CO intermediate formation. This DFT-guided strategy not only optimized C2 selectivity but also provided detailed insights into the reaction mechanisms, underscoring the crucial role of first-principles calculations in advancing the design of photocatalysts for high-value product formation. In another example shown in Figure 4B, Wang et al. utilized DFT to guide the molecular engineering of g-C3N4 through the integration of donor-acceptor units to address inherent structural defects[79]. DFT calculations were employed to predict the optimal electron-donating unit, with benzaldehyde identified as the ideal candidate to enhance intramolecular charge transfer and narrow the band gap. This theoretical prediction was validated experimentally, where the modified g-C3N4 demonstrated a 3.73-fold improvement in photocatalytic tetracycline degradation compared to the unmodified version, indicating the critical role of DFT in optimizing photocatalysts for environmental applications. In summary, first-principles calculations, particularly those grounded in DFT, have proven indispensable in predicting and fine-tuning the electronic and structural properties of photocatalysts, thereby facilitating the rational design of highly efficient systems across a wide range of applications.
Figure 4. Typical works of photocatalyst design by first-principle calculations. (A) LDHs/Ti3C2O2 designed by DFT calculation for photocatalytic reduction of CO2 to C2 organics[78]. Copyright 2022, Elsevier; (B) DFT predirected design of donor-acceptor structured g-C3N4 for efficient photocatalytic tetracycline abatement[79]. Copyright 2023, Wiley. DFT: Density functional theory; TDOS: total density of states; HOMO: highest occupied molecular orbital; LUMO: lowest unoccupied molecular orbital; PDOS: partial density of states; LDHs: layered double hydroxide.
HTS
HTS[91,92] is a technique that rapidly evaluates large sets of materials through the integration of computational and experimental methods, facilitating the efficient identification of candidates with optimal properties. It is instrumental in materials science, allowing for the automation of the evaluation process, which significantly accelerates the discovery and optimization of novel materials. HTS allows for the swift screening of various compositions, surface modifications, and structural configurations, identifying those with enhanced photocatalytic performance. This method reduces the time and resources typically required for experimental validation and expands the search space for high-performance photocatalysts. The HTS process in photocatalyst design typically starts with the creation of a comprehensive library of candidate materials, generated through either computational predictions or experimental synthesis. These candidates are then evaluated in a systematic manner, employing automated computational simulations or high-throughput experimental platforms to assess critical photocatalytic properties, such as band gap, charge carrier dynamics, and surface reactivity. Based on the screening results, the most promising candidates are identified for in-depth experimental validation and further optimization, streamlining the discovery of high-performance photocatalysts.
HTS has been successfully applied in several key studies to accelerate the discovery and optimization of photocatalysts, demonstrating its effectiveness in identifying high-performance candidates [Figure 5]. In a study by Yang et al., HTS combined with machine learning was employed to explore ternary organic heterojunction photocatalysts for sacrificial hydrogen evolution[93]. By conducting 736 experiments out of a possible 4,320 combinations, the most active systems achieved photocatalytic hydrogen production rates exceeding 500 mmol·g-1·h-1, showcasing the power of HTS in rapidly identifying high-performance photocatalysts. Sa et al. employed HTS to identify promising 2D Janus III-VI van der Waals heterostructures for solar energy applications[94]. From a total of 19,926 heterostructures, 1,035 were selected based on stability, and further application-driven screening revealed 66 photocatalysts and 71 solar cell candidates, highlighting the efficiency of HTS in pinpointing top-performing systems for energy conversion. Singh et al. conducted a large-scale HTS of 68,860 potential photocathodes for CO2 reduction, utilizing first-principles computations to assess synthesizability, corrosion resistance, visible-light absorption, and electronic structure compatibility with fuel synthesis[95]. Out of this vast pool, only 52 systems met all criteria, with 43 newly discovered candidates emerging as promising for further exploration, underscoring the capacity of high-throughput methods to efficiently filter through vast datasets and identify high-performing photocatalysts. Thermodynamic stability is initially prioritized to eliminate impractical candidates and minimize computational burden, while band edge alignment is assessed last due to its computational intensity, applied only to candidates that meet all preceding criteria. Wang et al. employed a data-driven, large-scale screening approach to explore intrinsic direct-gap 2D systems for photocatalytic water splitting[96]. Through a three-stage process of high-throughput DFT calculations, 16 candidates were identified as highly promising for efficient solar-to-hydrogen conversion, demonstrating the power of HTS in uncovering potential photocatalysts from vast, uncharted chemical spaces. HTS has emerged as a powerful tool for the rapid identification of high-performance photocatalysts by systematically exploring vast chemical spaces through a combination of computational modeling and experimental techniques. This approach not only expedites the discovery of photocatalysts with enhanced properties but also provides an efficient pathway for optimizing systems prior to in-depth experimental validation.
Figure 5. Photocatalyst designs by high-throughput screening. (A) Machine learning accelerated exploration of ternary organic heterojunction photocatalysts for sacrificial hydrogen evolution[93]. Copyright 2023, American Chemical Society; (B) High-throughput computational screening of Janus 2D III-VI van der Waals heterostructures for solar energy applications[94]. Copyright 2022, American Chemical Society; (C) Data-driven materials discovery of robust and synthesizable photocatalysts for CO2 reduction[95]. Copyright 2019, Springer; (D) Data-driven discovery of intrinsic direct-gap 2D materials as potential photocatalysts for efficient water splitting[96]. Copyright 2024, American Chemical Society. PBE: Perdew-Burke-Ernzerh; ML: machine learning; HSE: Heyd-Scuseria-Ernzerhof.
WORKFLOW FOR DEEP-LEARNING-ASSISTED PHOTOCATALYST DESIGN
Figure 6 presents the workflow for deep-learning-assisted photocatalyst design, which integrates several critical stages. The process begins with data collection, followed by featurization, where raw data are transformed into meaningful descriptors. Model training is conducted to optimize deep learning models based on these features, and model evaluation ensures the accuracy and reliability of predictions. The trained model is then applied to explore the unknown materials space, identifying promising photocatalysts. Based on the evaluation outcomes, model improvement is implemented, leading to the discovery of materials with superior properties that advance to practical applications, forming a continuous cycle of innovation.
Data collection and preprocess
Data forms the essential backbone of any deep learning model. In the photocatalyst design, data is typically derived from experimental measurements, published literature, and high-throughput computational simulations. These sources provide extensive information on material properties, performance metrics, and structural features, offering valuable insights into photocatalytic behavior. The process of data collection and preprocessing is critical for ensuring dataset quality and consistency. Data cleaning is conducted to address issues such as duplicate entries, missing values, and errors. To handle missing data, various imputation strategies were employed, tailored to the characteristics of the dataset and the specific attributes[97]. A hybrid framework leveraging Markov Logic Networks (MLNs) was implemented to infer probable values by supplementing insufficient integrity constraints with learned instantiated rules, thus establishing a robust data cleaning mechanism[98]. Additionally, an "active label cleaning" strategy[99] was utilized to improve data quality, particularly in datasets with label noise. This method prioritized samples for re-annotation based on estimated label correctness and labeling difficulty, enabling a more targeted and efficient correction process compared to random selection, thereby optimizing data preparation and significantly enhancing the overall quality of the training dataset.
Standardization techniques such as min-max scaling and z-score normalization[100] are employed to ensure uniformity across different data sources, particularly when integrating datasets with varying formats. For feature extraction, methods such as principal component analysis (PCA) and autoencoders[101,102] are commonly used to reduce dimensionality and isolate the most relevant characteristics, transforming raw data into a structured format suitable for deep learning model inputs. Table 4 lists the popular databases available for photocatalyst design. These databases offer a wide array of data, ranging from crystal structures (ICSD, CSD, COD) to computational predictions and material properties (Materials Project, AFLOW, NREL). Some databases, including MatWeb and MatNavi, focus on experimental data, while others, such as the Open Quantum Materials Database (OQMD), provide computationally derived properties and high-throughput calculations, enabling researchers to explore new material candidates and optimize photocatalytic performance.
List of popular databases available for photocatalyst design
Name | Database details | Data source |
ICSD | Inorganic Crystal Structure Database | Published structures |
CSD | Crystal structure data of small molecule organic compounds and metal-organic compounds | Experiments |
COD | Organic, inorganic, metal-organic, and mineral structures | Published and unpublished structures |
Materials Project | Provide material crystal structure and physicochemical properties, as well as other calculation tools such as structures and phase diagrams | Calculation using standard calculation scheme |
Chem Spider | The structure of chemical organic materials | Calculation and experiments |
AFLOW | Structure and properties | High-throughput calculation |
Harvard Clean Energy Project | Properties of organic solar compounds | High-throughput calculation |
Springer Materials | High quality numerical database on material properties | Published structures |
MatWeb | Material physical and chemical properties, grades | Experiments |
MatNavi | Phase diagrams and properties of metals, inorganic non-metals, polymers, superconductors, and other materials | Calculation and experiments |
Materials Cloud | Open and seamless sharing of computing science resources | Computational science |
Khazana | Structure and property, tools to design materials by learning from the data | Calculation |
NREL Materials Database | Properties of materials for renewable energy applications | Calculation |
Open Materials Database | Electronic structure database for 3D organic crystals | Calculation |
Open Quantum Materials Database | Thermodynamic and structural properties | DFT calculation |
Topological Materials Database | Topology Material Database | High-throughput computation |
Feature engineering and descriptors
Feature engineering[103] is a fundamental step in constructing deep learning models for the design of photocatalysts, where raw data must be transformed into structured and informative features that reflect the photocatalyst properties. This process directly affects the model capability to capture complex relationships within the data, ultimately determining its accuracy and predictive power. A crucial aspect of feature engineering is the use of descriptors, which quantitatively represent the physical and chemical characteristics of photocatalysts. Selecting the appropriate descriptors is essential for ensuring the model focuses on the most relevant features, significantly enhancing its ability to predict photocatalytic performance and optimize photocatalyst design[104].
Common descriptors in photocatalyst design include electronic structure, geometric structure, and surface properties, as listed in Table 5. Electronic structure descriptors, such as band gap, valence band maximum (VBM), conduction band minimum (CBM), and DOS, are crucial for understanding light absorption and charge carrier dynamics. Geometric descriptors, including size, morphology, crystal planes, and lattice symmetry, provide insights into the spatial arrangement of atoms and its effect on photocatalytic activity. Surface property descriptors, including specific surface area, pore structure, and surface functional groups, influence reactivity and adsorption behavior. These descriptors are essential for building deep learning models that can accurately predict and optimize photocatalytic performance across various applications, such as water splitting, pollutant degradation, and hydrogen production. Furthermore, the descriptors encompass not only electronic structure parameters but also geometrical and surface characteristics, such as pore structure and surface functional groups. These features help establish nonlinear correlations between semiconductor properties and photocatalytic performance, facilitating a generalized performance prediction across various photocatalysts. Deep learning approaches leverage these diverse features to extract patterns from large datasets, aiding in identifying and optimizing the most promising candidate and enhancing the efficiency of photocatalyst design and application.
Typical descriptors for photocatalysts in deep-learning approach
Material | Application | Descriptors | Ref. |
MOFs | Water splitting | Morphology, pore structure, band structure, bandgap, VBM, CBM, carrier mobility | [105] |
Conjugated Polyelectrolytes | Hydrogen production | Morphology, surface functional groups, band structure, bandgap, VBM, CBM | [106] |
Bi12TiO20 | Cefixime removal | Morphology, size, specific surface area, space group, stoichiometry, band structure, bandgap | [107] |
Bi2O3/MnO2 | Acetaminophen degradation | Morphology, size, specific surface area, porous structure, surface functional groups, crystal planes, band structure, light absorption coefficient, carrier mobility, carrier lifetime | [108] |
g-C3N4 | NO removal | Specific surface area, pore structure, doping atoms, bandgap, absorption wavelength | [109] |
TiO2 | Phenazopyridine ozonation | Morphology, size, specific surface area, porous structure, surface functional groups, crystal face, band structure, light absorption coefficient, carrier mobility, carrier lifetime, photocurrent density, absorption wavelength | [110] |
ZnO | Dye degradation | Morphology, size, specific surface area, surface functional groups, crystal plane, lattice symmetry, space group, band structure, carrier mobility, carrier lifetime, light absorption coefficient, absorption wavelength | [111] |
TiO2-biochar | BB41 degradation | Morphology, size, specific surface area, pore structure, surface functional groups, crystal plane, lattice symmetry, band structure, carrier mobility, light absorption coefficient, absorption wavelength | [112] |
2D materials | Water splitting | Size, band structure, bandgap, VBM, CBM, carrier mobility, carrier lifetime | [113] |
Co3O4/TiO2 | MB degradation | Morphology, size, surface functional groups | [114] |
Model construction and training
The construction and training of deep learning models for photocatalyst design involve defining the model architecture, selecting relevant descriptors as input features, and optimizing the model parameters. Typical deep learning models with their features and applications are listed in Table 6. Traditional deep learning models, including deep neural networks (DNNs)[115], are widely used for classification and regression in predicting photocatalytic properties. Convolutional neural networks (CNNs)[116] analyze spatial data, aiding in surface and structural characterization. Recurrent neural networks (RNNs)[117] and Long Short-Term Memory (LSTM)[118] handle time-series data, crucial for studying reaction kinetics. State-of-the-art models such as transformers[119] capture complex dependencies using self-attention mechanisms[120]. Shapley additive explanations (SHAP)[121] and Gradient-weighted class activation mapping (Grad-CAM)[122] improve model interpretability by highlighting key features. Kolmogorov-Arnold networks (KANs)[123] are adept at modeling nonlinear systems, revealing insights into complex photocatalytic processes.
Typical deep learning models with their features and applications
Deep learning model | Features | Applications |
DNN | Multi-layer architecture | Classification, regression |
CNN | Convolutional layers for spatial data | Image classification, object detection |
RNN | Sequence modeling | Time-series, speech recognition |
LSTM | Long-term dependency handling | Time-series forecasting |
GAN | Adversarial training | Image generation, data augmentation |
GNN | Graph structure processing | Social networks, drug discovery |
Transformer | Self-attention mechanism | NLP, machine translation |
SHAP | Feature importance attribution | Model interpretability, finance |
Grad-CAM | Visual attention mapping | Model transparency, medical imaging |
KAN | Universal function approximation | Nonlinear system modeling |
Model validation and evaluation
Model validation and evaluation are essential steps in ensuring the robustness and effectiveness of deep learning models in photocatalyst design. These processes verify predictive accuracy and generalization ability, ensuring alignment between model predictions and experimental data, while mitigating issues such as overfitting and underfitting[124]. Key evaluation metrics include mean squared error (MSE) and root MSE (RMSE), which quantify prediction errors, and R-squared (R²), which measures the proportion of variance explained by the model. Cross-validation and hold-out methods[125] are commonly used to partition datasets into training and testing subsets, providing unbiased estimates of model performance.
DEEP LEARNING APPROACHES IN PHOTOCATALYST DESIGN
Building on the deep-learning-assisted workflow outlined in the previous section, deep learning techniques have profoundly transformed multiple facets of photocatalyst design. These approaches encompass six critical areas: novel photocatalyst discovery, microstructure design, property optimization, innovative methodologies, application exploration, and mechanistic insights into photocatalytic processes. The following sections provide a detailed examination of each area, illustrating how deep learning drives advancements in the design and development of high-performance photocatalysts.
Discovery of novel photocatalysts
Deep learning has revolutionized the discovery of novel photocatalysts by enabling the rapid screening of vast chemical spaces and predicting materials with superior photocatalytic properties. By leveraging data-driven models, deep learning accelerates the identification of candidates optimized for light absorption, charge separation, and photocatalytic efficiency, significantly reducing the time and resources required compared to traditional experimental approaches.
A recent study[126] utilized machine learning to design perovskite oxide materials for photocatalytic water splitting, addressing the inefficiency of traditional trial-and-error methods in discovering new visible-light photocatalysts, as shown in Figure 7A. The study constructed structural-property models to predict hydrogen production rates and optimal bandgaps using algorithms such as gradient boosting regression (GBR), support vector regression (SVR), and backpropagation artificial neural networks (BPANN). The feature selection process began with an initial set of 24 features, comprising 18 atomic parameters and six experimental conditions. These features were then reduced to 20 through an initial filtering step, focusing on eliminating redundancies. Subsequently, advanced selection techniques such as Max Relevance Min Redundancy (mRMR) and embedded feature selection were applied, ultimately narrowing the set to 7-9 key features that significantly enhanced model accuracy while minimizing overfitting. The BPANN model achieved the highest prediction accuracy for hydrogen production, while GBR showed the best performance for bandgap prediction. Ultimately, 14 promising perovskite photocatalysts were identified from 30,000 candidates, and two online web servers were developed to share the prediction models, allowing public access for future research. Huang et al. applied machine learning techniques to address the complex task of selecting optimal cocatalysts for BiVO4 photoanodes in photoelectrochemical systems, as exhibited in Figure 7B[127]. The approach involved training multi-layer perceptron neural networks and tree-based ensemble models to predict high-performance cocatalyst-photoanode combinations. The random forest model achieved a classification accuracy of 96.30%, with cocatalyst type and preparation method identified as the most influential factors affecting photocatalytic performance. Additionally, the study utilized SHAP to derive heuristic rules for guiding the selection of promising cocatalyst/photoanode systems.
Figure 7. Machine learning aided discovery of novel photocatalysts. (A) Machine learning aided design of perovskite oxide materials for photocatalytic water splitting[126]. Copyright 2021, Elsevier; (B) Machine learning strategy for designing high-performance photoanode catalysts[127]. Copyright 2023, Royal Society of Chemistry.
There are also some successful cases in novel photocatalyst design accelerated by deep learning. Machine learning has been applied to the design of TiO2-coated glass for improved NOx removal efficiency[128]. Additionally, core-shell Au-silica nanoparticles[129], lead-free Bi-based perovskites[130], and g-C3N4-based single-atom photocatalysts[131] have been identified through deep-learning approaches. Choudhary et al. developed the InterMat framework, which combines DFT and GNN to predict band offsets in semiconductor interfaces, enabling the rapid screening of over 1.4 trillion potential interfaces[132]. This large-scale model, which is publicly available, offers an efficient tool for discovering novel photocatalysts. The application of deep learning in the discovery of novel photocatalysts has significantly accelerated the identification and design of high-performance photocatalytic systems, demonstrating its transformative impact across various applications.
Microstructure design of photocatalysts
The microstructure of photocatalysts is critical in determining their efficiency, as it directly affects light absorption, charge separation, and surface activity. Microstructures such as heterojunctions provide significant advantages by promoting more efficient charge separation and transfer at interfaces, which in turn enhances overall photocatalytic performance. Guevarra et al. employed a deep learning-based materials structure-property factorization (MSPF) to accelerate the design of photocatalyst structures[133] [Figure 8]. This approach utilized Deep Reasoning Networks (DRNets) for phase mapping and matrix factorization for modeling key properties, revealing synergistic interactions between BiVO4-like and Cu-based phases that significantly enhanced photocatalytic performance. The study highlights the role of deep learning in advancing structural innovation and optimizing complex photocatalytic systems.
Figure 8. Phase mapping in the Bi-Cu-V oxide system. (A) Photographs of 4 sputter-deposited composition libraries in Bi–Cu–V oxide system; (B) Representative XRD patterns collected on the composition libraries, where each XRD pattern is plotted at the location of the composition in the Bi–Cu–V composition graph; (C) The DRNets solution for one composition, showing that the XRD pattern of the Bi0.11Cu0.39V0.50 oxide sample is composed of 42% BiVO4 and 58% Cu2V2O7; (D) The resulting phase map of the primary 14 of the 21 phases identified by DRNets, where each phase has a unique symbol whose point size indicates phase concentration in the composition graph[133]. Copyright 2022, Springer Nature. XRD: X-ray diffraction; DRNets: deep reasoning networks.
Building on recent advances, further efforts have utilized deep learning models to explore complex structural modifications. Jiang et al. developed an interpretable CNN to predict active sites involved in
Property optimization of photocatalysts
Predicting key properties of photocatalysts by deep learning models has emerged as a critical research area, which offers high-precision predictions, greatly enhancing the optimization of photocatalytic performance. Bonke[136] employed machine learning algorithms to optimize the multivariable performance of a self-assembled photocatalytic system for CO2 reduction [Figure 9]. By defining a holistic performance metric that integrates multiple figures of merit, the model guided experimental optimization of yield, quantum yield, turnover number, frequency, and selectivity simultaneously. Additionally, Wang et al. demonstrated the efficacy of intelligent algorithms, particularly artificial neural networks (ANNs), in optimizing photocatalyst properties[137]. These models enable accurate predictions of key photocatalytic performance metrics, significantly reducing the time and resources required for experimental iterations, thereby accelerating the design of efficient photocatalysts. This methodology provided a standardized approach for optimizing multimetric photocatalytic systems, offering deeper insights into performance bottlenecks and accelerating advancements in photocatalysis.
Figure 9. Multivariable multimetric optimization of self-assembled photocatalytic CO2 reduction performance using machine learning algorithms. (A) Overview of the workflow; (B) Holistic improvement measured by objective function alongside improvement of both YieldCO and TOFCO; (C) Ascending YieldCO and corresponding TOFCO[136]. Copyright 2024, American Chemical Society. TON: Turnovers for CO formation; TOF: turnover frequency; QY: quantum yield.
Beyond multivariable optimization, machine learning has been extensively applied to enhance the performance of diverse photocatalytic systems. For instance, random forest models have been used to assess the contribution of pretreatment methods on TiO2 surfaces for CO2 reduction[138], while CatBoost models have optimized malachite green degradation efficiency using noble metal-doped BiFeO3 (NM-BiFeO3) composites[139]. Additionally, decision tree models have predicted gas and liquid product types in photocatalytic CO2 reduction over metal-organic frameworks[140]. Gated recurrent unit (GRU) neural networks have forecasted hydrogen production in continuous water splitting systems[141]. Moreover, GBR methods have modeled the degradation of persistent pollutants such as perfluorooctanoic acid (PFOA)[142]. Through the integration of experimental data and theoretical models, deep learning has significantly enhanced the ability to predict and optimize key photocatalytic properties, such as light absorption, photocatalytic efficiency, and degradation performance, driving improvements across diverse applications in photocatalyst design.
Novel deep learning approaches of photocatalyst design
Beyond the common deep learning models, novel approaches continue to emerge, offering enhanced capabilities for photocatalyst design. These advanced methods improve adaptability and the ability to handle complex relationships in large datasets, driving significant progress in the field. Figure 10 reveals a hybrid method combining computational fluid dynamics (CFD), ANNs, and genetic algorithms (GA) for the analysis and optimization of micro-photocatalytic reactors aimed at NOx abatement[143]. CFD simulations were used to investigate the effects of key variables, while ANN models were developed to predict NO conversion with high accuracy (R2 = 0.9997). GA was then employed to optimize operating conditions, achieving full NO conversion (100%) under specific parameters, with residence time identified as the most influential factor. A multi-objective optimization further applied GA to maximize NO consumption while minimizing pressure drop, offering valuable insights into balancing performance metrics.
Figure 10. CFD, ANN and GA as a hybrid method for the analysis and optimization of micro-photocatalytic reactors for NOx abatement[143]. Copyright 2021, Elsevier. CFD: Computational fluid dynamics; ANN: artificial neural network; GA: genetic algorithm; MSE: mean squared error.
In addition to hybrid CFD-ANN-GA approaches, recent advancements have introduced innovative methods to enhance the design and optimization of photocatalysts. Li et al. combined machine learning with high-throughput experimentation to identify organic molecules with high photocatalytic activity, successfully predicting molecular performance and significantly reducing experimental costs[144]. Similarly, Parmar et al. applied a comparative approach using ANN and response surface methodology to optimize ciprofloxacin degradation, achieving high predictive accuracy and offering a novel strategy for pharmaceutical waste removal through computational modeling[145]. The emergence of novel deep learning approaches has significantly enhanced photocatalyst discovery and optimization, providing innovative solutions that improve predictive accuracy and reduce experimental costs across various applications.
Application exploration for photocatalysts
Deep learning has significantly broadened the scope of photocatalyst applications by enabling precise predictions of system performance in complex environments. This advancement accelerates the discovery of new uses in areas such as environmental remediation, energy conversion, and medical applications. Malayeri et al. leveraged ANN and GA to optimize photocatalytic oxidation (PCO) reactors for air purification, thereby expanding the applicability of PCO technology in indoor environments[146]. By accurately predicting volatile organic compound (VOC) and by-product concentrations, the ANN model facilitated the identification of optimal operating conditions, significantly enhancing the efficiency of harmful compound removal. This approach also minimized the formation of toxic by-products, addressing a critical challenge in the widespread adoption of PCO technology. The study illustrated how advanced machine learning techniques can broaden PCO application in the development of safer and more effective air purification systems [Figure 11].
Figure 11. Application of artificial neural network and genetic algorithm in optimization of photocatalytic oxidation reactor for air purifier design[146]. Copyright 2023, Elsevier. ANN: Artificial neural network; GA: genetic algorithm; VOC: volatile organic compound.
Beyond air purification, deep learning has significantly expanded the applications of photocatalysts in fields such as water treatment[147], energy conversion[148], and medical applications[149]. In water treatment, machine learning has optimized the photocatalytic degradation of pollutants such as dyes and pharmaceuticals[150], enhancing process efficiency and introducing innovative treatment approaches. In the energy and medical sectors, deep learning has driven advancements in solar-to-hydrogen conversion[151] and the design of antimicrobial surfaces[152]. Therefore, it has greatly expanded the scope of photocatalyst applications, facilitating advancements in cutting-edge areas, driving innovative solutions to complex global challenges.
Mechanism insights for photocatalysis
Deep learning has become an essential tool in unraveling photocatalytic reaction mechanisms by analyzing reaction pathways and intermediates. These models offer profound insights into the fundamental processes of photocatalysis, enabling more precise experimental design and the optimization of photocatalytic systems. Kim et al. utilized a machine learning approach to unravel the complex mechanisms influencing the photocatalytic reaction rate constant (k) in semiconductor-based photocatalysts [Figure 12], specifically for dye removal applications[153]. By employing a decision tree model and SHAP feature selection, the analysis identified 11 key input features that significantly impacted the reaction rate. Experimental conditions emerged as the most influential factor (59%), followed by atomic composition (39%), offering valuable insights into how process parameters and co-catalysts interact to affect photocatalytic performance. This study demonstrated the ability of machine learning models to enhance our understanding of reaction mechanisms by elucidating the interactions between multiple process variables.
Figure 12. Machine learning analysis to interpret the effect of the photocatalytic reaction rate constant (k) of semiconductor-based photocatalysts on dye removal[153]. Copyright 2024, Elsevier. SVR: Support vector regression; SHAP: shapley additive explanations; LGBM: light gradient boosting machine; XGB: eXtreme gradient boosting.
Beyond reaction rate analysis, another work[154] explored the excitonic effects in nearly 50 photocatalysts for CO2 reduction, an often overlooked but crucial aspect of photocatalytic performance. By applying the Bethe-Salpeter formalism, this study identified six promising materials through optical property screening, offering new insights into the role of exciton binding energies in enhancing solar-energy harvesting applications. The works highlighted how deep-learning approaches were advancing the understanding of photocatalytic mechanisms, from reaction kinetics to excitonic effects, offering valuable insights for optimizing photocatalyst performance. Therefore, deep learning approaches in photocatalyst design have significantly advanced the field by enabling the discovery of novel photocatalysts, optimizing microstructures, and refining material properties for enhanced performance. These approaches have also expanded the range of photocatalyst applications, from environmental remediation to energy conversion and medical uses, while providing critical insights into the underlying mechanisms driving photocatalytic reactions. As deep learning models evolve, their integration with experimental data and computational methods continues to push the boundaries of photocatalyst design, paving the way for future breakthroughs in cutting-edge research areas.
SYNERGY OF MULTIDIMENSIONAL COMPUTATION AND DEEP LEARNING IN PHOTOCATALYST DESIGN
The discovery of novel photocatalysts has significantly benefited from both computational and deep learning methodologies, each contributing distinct advantages that, when integrated, provide a comprehensive framework for materials design. Initially, computational methods, particularly those based on DFT, enable accurate assessment of key properties such as electronic structure, thermodynamic stability, and adsorption energetics. These insights are essential for identifying promising candidates grounded in fundamental physical and chemical principles. In contrast, deep learning complements computational approaches by capturing complex, nonlinear relationships within existing data, thus enabling rapid predictions of photocatalytic performance across a vast range of materials. When combined, the integrated approach leverages the precision of computational methods to generate high-quality training datasets for deep learning models, while deep learning expedites the screening process, efficiently identifying potential high-performance materials. This synergistic use of computational and deep learning techniques not only reduces the discovery timeline but also enhances the accuracy and scalability of photocatalyst development, thereby providing a robust framework for the advancement of next-generation photocatalytic systems. This integration of deep learning and computational methodologies lays the foundation for more sophisticated multidimensional approaches, designed to further expand capabilities by synthesizing data from multiple sources and scales.
Multidimensional computational models encompass a range of techniques designed to handle complex systems by integrating data from various sources and scales. These models include hybrid approaches that combine different computational methods, multiscale simulations that link phenomena across different levels of resolution, and multimodal analyses that incorporate diverse data types to provide a more holistic understanding of intricate processes. Multimodal and multiscale computational models have been successfully applied in areas such as estimating permeate flux in dynamic membranes[155] and predicting wettability evolution on laser-textured surfaces[156]. These methods have also advanced the understanding of surface-enhanced spectroscopy[157] and photocatalysis[158,159], providing detailed insights into reaction mechanisms and kinetics. In photocatalyst design, such approaches hold the potential to optimize structures and performance by integrating atomic-scale simulations with larger environmental models, enabling more efficient and scalable solutions for real-world applications.
Loh et al. explored the integration of multiscale physics and machine learning to tackle the challenges of photocatalyst-photoreactor synergy in solar fuel production[160] [Figure 13]. By leveraging physics-informed neural networks (PINN), this approach captured complex, multiscale phenomena such as charge-carrier dynamics and solar energy collection, offering a deeper understanding of material-photoreactor interactions. The study exemplified how combining multidimensional computation with deep learning could optimize reactor performance and drive advancements in scalable solar fuel technology.
Figure 13. Deep learning in multiscale physics of photocatalyst and photoreactor design[160]. Copyright 2024, Wiley. DFT: Density functional theory; TD-DFT: time-dependent density functional theory.
CHALLENGES AND FUTURE PROSPECTS
The integration of deep learning with photocatalyst design has driven innovations across multiple dimensions, including the discovery of novel photocatalysts, the optimization of microstructures and properties, and the exploration of advanced approaches, applications, and mechanistic insights. The synergy between deep learning and multidimensional computational models has facilitated more precise predictions, cross-scale analyses, and enhanced reactor designs. These combined methodologies have broadened the applications of photocatalysis, accelerating breakthroughs in environmental remediation, energy conversion, and performance optimization. Despite these advancements, several critical challenges remain to be addressed to fully unlock the potential of deep-learning-assisted photocatalyst design.
Dataset availability and quality
One of the fundamental challenges in deep-learning-assisted photocatalyst design is the acquisition of high-quality, comprehensive datasets. Photocatalytic reactions involve complex interactions between factors such as composition, structure, environmental conditions, and experimental setups, making it difficult to compile standardized data across different studies. Existing datasets are often fragmented, with inconsistencies in reporting, missing information, and limited experimental reproducibility. The scarcity of large, labeled datasets also limits the training of robust deep learning models, reducing their ability to generalize across diverse photocatalytic systems. Addressing these challenges requires the aggregation of data from multiple sources and the development of uniform data collection protocols to ensure data quality and consistency.
To manage and organize the growing volume of photocatalytic data, effective data management strategies are essential. Implementing standardized data formats and ontologies can streamline the integration of diverse datasets from experimental and computational studies. Advanced data cleaning techniques, such as automated outlier detection and missing value imputation, are necessary to ensure the reliability of the data used for model training. The development of centralized databases or repositories for photocatalytic research will facilitate data sharing and collaboration within the scientific community. Cloud-based platforms and machine learning pipelines can further enhance data processing efficiency and scalability, ensuring continuous model improvement as more data becomes available.
Interpretable deep learning model
The “black box” nature of deep learning models poses a significant challenge in photocatalyst design, limiting the understanding of how model predictions are made. Improving the transparency of these models is essential to close the gap between predictions and physical experiments, enabling a clearer interpretation of how specific features influence photocatalytic performance. Approaches such as SHAP and local interpretable model-agnostic explanations (LIME) are proving effective in identifying the most critical input variables. The KAN model further enhances interpretability by providing a more quantifiable explanation of the relationships between inputs and outputs. Additionally, integrating physics-based principles through models such as PINN aligns the deep learning process more closely with known physical laws. These advances will facilitate a deeper understanding of photocatalytic mechanisms and promote the broader application of deep learning in photocatalyst design.
Scalability and computing resources
The increasing complexity and size of deep learning models, especially large-scale models, used in photocatalyst design require significant computational resources, presenting a challenge in managing vast datasets and running high-dimensional simulations. As large models such as transformers and foundation models become more prevalent, the demand for scalable computing infrastructures, such as high-performance computing clusters and cloud-based systems, has become essential to handle the intense computational workloads. Hardware advancements, particularly in the development of GPUs and TPUs, are driving improvements in training speed and model efficiency. Moreover, the development of more efficient architectures, along with advancements in large model fine-tuning, is reducing computational cost while maintaining high predictive accuracy. Techniques such as model pruning, quantization, and efficient distributed computing frameworks enable parallelization, allowing the processing of massive datasets across multiple machines. These large models open the door for unprecedented accuracy in predictions, but the hardware and algorithmic advancements will be critical to overcoming the computational bottlenecks associated with their scale. The integration of these advancements is key for making large-scale photocatalyst design feasible and scalable.
Reversal design of photocatalysts
Reversal design is a methodology that works backward from a desired property or performance target to identify the optimal structures or configurations, offering a powerful approach in materials science. In photocatalyst design, reversal design enables the identification of systems with optimized performance by working backward from specific targets, such as light absorption or photocatalytic efficiency, to tailor structural or compositional features. This methodology accelerates the discovery process by narrowing the search space to systems that meet predefined performance metrics. Deep learning enhances reversal design by using predictive models that efficiently map the relationship between structures and properties, allowing rapid iteration toward optimal solutions. It can generate new candidates through generative models and apply reinforcement learning to refine designs based on experimental feedback. With its ability to explore vast design spaces, deep learning-driven reversal design is transforming the way photocatalysts are discovered and developed.
Multiscale modeling and computation
Photocatalytic reactions inherently span multiple scales, from electron-level interactions to macroscopic phenomena, posing significant challenges for comprehensive modeling. The integration of models that describe different scales - such as atomic-scale electron dynamics, charge carrier transport, and bulk-phase mass transfer - into a unified multiscale framework remains a critical hurdle in photocatalyst design. Multiscale deep learning models provide an opportunity to bridge these gaps, enabling more accurate performance predictions by incorporating insights from various scales. This requires combining quantum simulations, molecular dynamics, and continuum models, which presents not only computational demands but also algorithmic complexity. Successful advances in multiscale modeling could yield deeper insights into how atomic and molecular processes influence macroscopic behavior, driving the development of more efficient photocatalysts. Additionally, ensuring the interpretability and practical applicability of these models is essential for their experimental validation. Addressing these challenges is a pivotal goal in advancing both photocatalyst design and materials informatics.
Future directions amidst challenges
Despite their substantial advantages in reducing experimental costs and time, deep learning and other computational methodologies present several inherent challenges. A primary challenge is the need for further enhancement of computationally designed photocatalysts. While integrated computational approaches can identify promising candidates, these photocatalysts frequently require additional modifications - such as surface engineering, doping, or structural optimization - to achieve optimal performance[162]. Addressing these limitations often involves iterative cycles of computational predictions followed by experimental validation to refine photocatalyst properties[163]. Another critical challenge involves scaling these technologies from laboratory settings to industrial applications. Photocatalysts that demonstrate exceptional performance on a small scale often encounter significant obstacles in maintaining efficiency and stability during scale-up. Overcoming these scale-up challenges requires advancements in reactor design, synthesis methodologies, and engineering practices to ensure economically feasible production processes. Moreover, the successful commercialization of photocatalyst technologies necessitates a multidisciplinary approach. Issues such as stability, selectivity, and overall efficiency cannot be fully addressed within a single discipline. Effective commercialization requires collaboration among chemists, materials scientists, chemical engineers, and industry professionals to bridge the gap between laboratory research and market-ready technologies, ultimately facilitating the transition to commercially viable photocatalytic solutions. In conclusion, although challenges remain, rapid progress in computational tools, coupled with targeted experimental efforts and interdisciplinary collaboration, suggests a promising future for photocatalyst design and application. With ongoing innovation, these technologies hold substantial potential for contributing to sustainable energy and environmental applications.
CONCLUSIONS
In conclusion, the integration of deep learning with photocatalyst design has transformed the field, unlocking new strategies for optimizing photocatalytic systems. This review highlights how computational methods combined with deep learning have significantly advanced the discovery of novel photocatalysts, refined microstructure design, optimized functional properties, and provided deeper mechanistic insights, driving innovations in environmental remediation, energy conversion, and chemical processes. Furthermore, the synergy between multidimensional computational models and deep learning has enabled more precise predictions, efficient experimental validation, and greater scalability in photocatalytic applications. Despite these advancements, challenges remain in areas such as dataset quality, model interpretability, and the seamless integration of multiscale modeling. Addressing these challenges will be crucial for further breakthroughs, guiding the future design of high-performance photocatalysts and fostering the development of sustainable technologies.
DECLARATIONS
Authors’ contributions
Conceptualization: Liu J, Su B, Liang L
Methodology: Liu J, Su B, Liang L
Software: Wu D
Writing - original draft: Liu J, Su B, Liang L
Writing - review and editing: Zhang Y, Wu J, Fu C
Supervision and funding acquisition: Fu C
Availability of data and materials
Not applicable.
Financial support and sponsorship
This work is supported by the Liaoning Applied Fundamental Research Project (Grant Nos. 2022JH2/101300158 and 2023JH2/101300014) and the Fundamental Research Funds for the Central Universities (Grant Nos. 3132024243 and 3132023506).
Conflicts of interest
All authors declared that there are no conflicts of interest.
Ethical approval and consent to participate
Not applicable.
Consent for publication
Not applicable.
Copyright
© The Author(s) 2024.
REFERENCES
1. Lee WH, Lee CW, Cha GD, et al. Floatable photocatalytic hydrogel nanocomposites for large-scale solar hydrogen production. Nat Nanotechnol 2023;18:754-62.
2. Zhang Y, Huang X, Yeom J. A floatable piezo-photocatalytic platform based on semi-embedded ZnO nanowire array for high-performance water decontamination. Nanomicro Lett 2019;11:11.
3. Sayed M, Ren B, Ali AM, et al. Solar light induced photocatalytic activation of peroxymonosulfate by ultra-thin Ti3+ self-doped
4. Zhao D, Wu X, Gu X, Liu J. Investigation into the degradation of air and runoff pollutants using nano g-C3N4 photocatalytic road surfaces. Constr Build Mater 2024;411:134553.
5. Liu J, Zhang Q, Tian X, et al. Highly efficient photocatalytic degradation of oil pollutants by oxygen deficient SnO2 quantum dots for water remediation. Chem Eng J 2021;404:127146.
6. Barakat M. New trends in removing heavy metals from industrial wastewater. Arab J Chem 2011;4:361-77.
7. Zhang X, Wu F, Li G, et al. Modulating electronic structure and sulfur p-band center by anchoring amorphous Ni@NiSx on crystalline CdS for expediting photocatalytic H2 evolution. Appl Catal B Environ 2024;342:123398.
8. Zhang L, Jiang Z, Guo J, et al. Deep insight into regulation mechanism of band distribution in phase junction CdS for enhanced photocatalytic H2 production. J Colloid Interface Sci 2024;669:146-56.
9. Gong E, Ali S, Hiragond CB, et al. Solar fuels: research and development strategies to accelerate photocatalytic CO2 conversion into hydrocarbon fuels. Energy Environ Sci 2022;15:880-937.
10. Liang C, Niu H, Guo H, et al. Efficient photocatalytic nitrogen fixation to ammonia over bismuth monoxide quantum dots-modified defective ultrathin graphitic carbon nitride. Chem Eng J 2021;406:126868.
11. Shi D, Zhang L, Cao Y. Band structure engineering of Pd, Rh, Cu-modified SrMoO4 for enhanced activity and selectivity in photocatalytic CO2 reduction to CH4. Appl Surf Sci 2024;648:158979.
12. Mai H, Le TC, Chen D, Winkler DA, Caruso RA. Machine learning for electrocatalyst and photocatalyst design and discovery. Chem Rev 2022;122:13478-515.
13. Dong W, Zhou S, Ma Y, et al. N-doped C-coated MoO2/ZnIn2S4 heterojunction for efficient photocatalytic hydrogen production. Rare Met 2023;42:1195-204.
14. Li R, Bian Y, Yang C, et al. Electronic structure regulation and built-in electric field synergistically strengthen photocatalytic nitrogen fixation performance on Ti-BiOBr/TiO2 heterostructure. Rare Met 2024;43:1125-38.
15. Ma Y, Fang H, Chen R, et al. 2D-MOF/2D-MOF heterojunctions with strong hetero-interface interaction for enhanced photocatalytic hydrogen evolution. Rare Met 2023;42:3993-4004.
16. Xiong J, Li X, Huang J, et al. CN/rGO@BPQDs high-low junctions with stretching spatial charge separation ability for photocatalytic degradation and H2O2 production. Appl Catal B Environ 2020;266:118602.
17. Wu X, Yao X, Xie B, et al. Unraveling the atmospheric oxidation mechanism and kinetics of naphthalene: insights from theoretical exploration. Chemosphere 2024;352:141356.
18. Arce-Saldaña L, Soto G, Herrera JR, Simakov A, Flores UC. Compact device for in situ ultraviolet-visible spectrophotometric measurement of photocatalytic kinetics. Rev Sci Instrum 2023;94:085106.
19. Schnabel T, Dutschke M, Schuetz F, Hauser F, Springer C. Photocatalytic air purification of polycyclic aromatic hydrocarbons: application of a flow-through reactor, kinetic studies and degradation pathways. J Photochem Photobiol A Chem 2022;430:113993.
20. Guo Y, Dai Y, Wang Y, et al. Boosted visible-light-driven degradation over stable ternary heterojunction as a plasmonic photocatalyst: mechanism exploration, pathway and toxicity evaluation. J Colloid Interface Sci 2023;641:758-81.
21. Dhawan A, Sudhaik A, Raizada P, et al. BiFeO3-based Z scheme photocatalytic systems: advances, mechanism, and applications. J Ind Eng Chem 2023;117:1-20.
22. Hu Y, Chen J, Wei Z, He Q, Zhao Y. Recent advances and applications of machine learning in electrocatalysis. J Mater Inf 2023;3:18.
23. Humayun M, Ullah H, Cao J, et al. Experimental and DFT studies of Au deposition over WO3/g-C3N4 Z-scheme heterojunction. Nanomicro Lett 2019;12:7.
24. Liu J, Gao F, Wu L, et al. Size effect on oxygen vacancy formation and gaseous adsorption in ZnO nanocrystallites for gas sensors: a first principle calculation study. Appl Phys A 2020;126:3643.
25. Yang Y, Zeng G, Huang D, et al. Molecular engineering of polymeric carbon nitride for highly efficient photocatalytic oxytetracycline degradation and H2O2 production. Appl Catal B Environ 2020;272:118970.
26. Wang V, Tang G, Liu YC, et al. High-throughput computational screening of two-dimensional semiconductors. J Phys Chem Lett 2022;13:11581-94.
27. Fu C, Zhang K, Guan H, et al. Progressive prediction algorithm by multi-interval data sampling in multi-task learning for real-time gas identification. Sens Actuators B Chem 2024;418:136271.
28. Liu Y, Yang B, He H, Yang S, Duan X, Wang S. Bismuth-based complex oxides for photocatalytic applications in environmental remediation and water splitting: a review. Sci Total Environ 2022;804:150215.
29. Lim L, Lynch R. A proposed photocatalytic reactor design for in situ groundwater applications. Appl Catal A Gen 2010;378:202-10.
30. Lam S, Sin J, Zeng H, et al. Green synthesis of Fe-ZnO nanoparticles with improved sunlight photocatalytic performance for polyethylene film deterioration and bacterial inactivation. Mater Sci Semicond Process 2021;123:105574.
31. zhang T, Maihemllti M, Okitsu K, Talifur D, Tursun Y, Abulizi A. In situ self-assembled S-scheme BiOBr/pCN hybrid with enhanced photocatalytic activity for organic pollutant degradation and CO2 reduction. Appl Surf Sci 2021;556:149828.
32. Li X, Xiong J, Gao X, et al. Recent advances in 3D g-C3N4 composite photocatalysts for photocatalytic water splitting, degradation of pollutants and CO2 reduction. J Alloys Compd 2019;802:196-209.
33. Guo Q, Zhou C, Ma Z, Yang X. Fundamentals of TiO2 photocatalysis: concepts, mechanisms, and challenges. Adv Mater 2019;31:e1901997.
34. Xu Q, Jiang J, Wang X, Duan L, Guo H. Understanding oxygen vacant hollow structure CeO2@In2O3 heterojunction to promote CO2 reduction. Rare Met 2023;42:1888-98.
35. Li W, Li J, Liu Z, et al. Fast charge transfer kinetics in Sv-ZnIn2S4/Sb2S3 S-scheme heterojunction photocatalyst for enhanced photocatalytic hydrogen evolution. Rare Met 2024;43:533-42.
36. Ali S, Ismail PM, Khan M, et al. Charge transfer in TiO2-based photocatalysis: fundamental mechanisms to material strategies. Nanoscale 2024;16:4352-77.
37. Yu W, Hu C, Bai L, Tian N, Zhang Y, Huang H. Photocatalytic hydrogen peroxide evolution: what is the most effective strategy? Nano Energy 2022;104:107906.
38. Hashimoto K, Irie H, Fujishima A. TiO2 photocatalysis: a historical overview and future prospects. Jpn J Appl Phys 2005;44:8269.
39. Nasr M, Eid C, Habchi R, Miele P, Bechelany M. Recent progress on titanium dioxide nanomaterials for photocatalytic applications. ChemSusChem 2018;11:3023-47.
40. Wu D, Liu X, Liu J, Akhtar A, Fu C. Hydrothermal synthesis of Z-scheme photocatalyst Zn2SnO4-g-C3N4 for efficient tetracycline antibiotic removal. Diam Relat Mater 2024;141:110572.
41. Li J, Li Y. Recent advances in the interface structure prediction for heteromaterial systems. J Mater Inf 2023;3:22.
42. Cai M, Tong X, Zhao H, et al. Regulating intragap states in colloidal quantum dots for universal photocatalytic hydrogen evolution. Appl Catal B Environ 2024;343:123572.
43. Mao L, Cai X, Zhu M. Hierarchically 1D CdS decorated on 2D perovskite-type La2Ti2O7 nanosheet hybrids with enhanced photocatalytic performance. Rare Met 2021;40:1067-76.
44. Yang J, Liang Y, Li K, Yang G, Yin S. One-step low-temperature synthesis of 0D CeO2 quantum dots/2D BiOX (X = Cl, Br) nanoplates heterojunctions for highly boosting photo-oxidation and reduction ability. Appl Catal B Environ 2019;250:17-30.
45. Liu J, Qu X, Zhang C, et al. High-yield aqueous synthesis of partial-oxidized black phosphorus as layered nanodot photocatalysts for efficient visible-light driven degradation of emerging organic contaminants. J Clean Prod 2022;377:134228.
46. Guo S, Ji Y, Li Y, et al. Amorphous quantum dots co-catalyst: defect level induced solar-to-hydrogen production. Appl Catal B Environ 2023;330:122583.
47. Ye S, Zhou X, Xu Y, et al. Photocatalytic performance of multi-walled carbon nanotube/BiVO4synthesized by electro-spinning process and its degradation mechanisms on oxytetracycline. Chem Eng J 2019;373:880-90.
48. Karpuraranjith M, Chen Y, Rajaboopathi S, et al. Three-dimensional porous MoS2 nanobox embedded g-C3N4@TiO2 architecture for highly efficient photocatalytic degradation of organic pollutant. J Colloid Interface Sci 2022;605:613-23.
49. Hao X, Hu Z, Xiang D, Jin Z. Ultra-thin carbon coated amorphous N-doped CoP enhancing electron transfer for wide spectrum photocatalytic hydrogen evolution. Int J Hydrog Energy 2023;48:600-15.
50. Yang C, Li X, Li M, Liang G, Jin Z. Anchoring oxidation co-catalyst over CuMn2O4/graphdiyne S-scheme heterojunction to promote eosin-sensitized photocatalytic hydrogen evolution. Chin J Catal 2024;56:88-103.
51. Lee CW, Lee BH, Park S, et al. Photochemical tuning of dynamic defects for high-performance atomically dispersed catalysts. Nat Mater 2024;23:552-9.
52. Chen R, Chen S, Wang L, Wang D. Nanoscale metal particle modified single-atom catalyst: synthesis, characterization, and application. Adv Mater 2024;36:e2304713.
53. Li S, You C, Xue Q, et al. Carbon quantum dots and interfacial chemical bond synergistically modulated S-scheme Mn0.5Cd0.5S/BiOBr photocatalyst for efficient water purification. J Mater Sci Technol 2025;214:255-65.
54. Yang M, Zhu X, Zhu Z, et al. Atomic activation triggering selective photoreduction of CO2 to CH4 over NiAl-LDH/CeO2 heterojunction. Chem Eng J 2023;472:145071.
55. Wu Y, Zhu P, Li Y, Zhang L, Jin Z. In Situ derivatization of NiAl-LDH/NiS a p-n heterojunction for efficient photocatalytic hydrogen evolution. ACS Appl Energy Mater 2022;5:8157-68.
56. Yang M, Wang P, Li Y, et al. Graphene aerogel-based NiAl-LDH/g-C3N4 with ultratight sheet-sheet heterojunction for excellent visible-light photocatalytic activity of CO2 reduction. Appl Catal B Environ 2022;306:121065.
57. Wang C, You C, Rong K, Shen C, Yang F, Li S. An S-scheme MIL-101(Fe)-on-BiOCl heterostructure with oxygen vacancies for boosting photocatalytic removal of Cr(VI). Acta Phys Chim Sin 2024;40:2307045.
58. Liu Z, Li Y, Jin Z. Mechanochemical preparation of graphdiyne (CnH2n-2) based Ni-doped MoS2 S-scheme heterojunctions with in situ XPS characterization for efficient hydrogen production†. J Mater Chem C 2023;11:9327-40.
59. Dong K, Shen C, Yan R, Liu Y, Zhuang C, Li S. Integration of plasmonic effect and S-scheme heterojunction into Ag/Ag3PO4/C3N5 photocatalyst for boosted photocatalytic levofloxacin degradation. Acta Phys Chim Sin 2024;40:2310013.
60. Laxmi V, Agarwal S, Khan S. Advanced nanoribbons in water purification: a comprehensive review. J Environ Manage 2024;370:122645.
61. Jo W, Kumar S, Isaacs MA, Lee AF, Karthikeyan S. Cobalt promoted TiO2/GO for the photocatalytic degradation of oxytetracycline and Congo Red. Appl Catal B Environ 2017;201:159-68.
62. Wang Y, Wu X, Liu J, et al. Mo-modified band structure and enhanced photocatalytic properties of tin oxide quantum dots for visible-light driven degradation of antibiotic contaminants. J Environ Chem Eng 2022;10:107091.
63. Mouloua D, Lejeune M, Rajput NS, et al. One-step chemically vapor deposited hybrid 1T-MoS2/2H-MoS2 heterostructures towards methylene blue photodegradation. Ultrason Sonochem 2023;95:106381.
64. Yang K, Zhong S, Zhou X, et al. Controllable Al2O3 coating makes TiO2 photocatalysts active under visible light by pulsed chemical vapor deposition. Chem Eng Sci 2023;277:118792.
65. Ge L, Ke Y, Li X. Machine learning integrated photocatalysis: progress and challenges. Chem Commun 2023;59:5795-806.
66. Merchant A, Batzner S, Schoenholz SS, Aykol M, Cheon G, Cubuk ED. Scaling deep learning for materials discovery. Nature 2023;624:80-5.
67. Steinmann SN, Wang Q, Seh ZW. How machine learning can accelerate electrocatalysis discovery and optimization. Mater Horiz 2023;10:393-406.
68. Javed MF, Shahab MZ, Asif U, et al. Evaluation of machine learning models for predicting TiO2 photocatalytic degradation of air contaminants. Sci Rep 2024;14:13688.
69. Subramanian Y, Gajendiran J, Veena R, et al. Structural, photoabsorption and photocatalytic characteristics of BiFeO3-WO3 nanocomposites: an attempt to validate the experimental data through SVM-based artificial intelligence (AI). J Electron Mater 2023;52:2421-31.
70. Ahmed F, Kang IS, Kim KH, et al. Drug repurposing for viral cancers: a paradigm of machine learning, deep learning, and virtual screening-based approaches. J Med Virol 2023;95:e28693.
71. Yadav A, Acosta CM, Dalpian GM, Malyi OI. First-principles investigations of 2D materials: challenges and best practices. Matter 2023;6:2711-34.
72. Liu J, Wu L, Gao F, Hong W, Jin G, Zhai Z. Size effects of vacancy formation and oxygen adsorption on gas- sensitive tin oxide semiconductor: a first principle study. CNANO 2021;17:327-37.
73. Gu Y, Gu Y, Tao Q, Wang X, Zhu Q, Ma J. Machine learning for prediction of CO2/N2/H2O selective adsorption and separation in metal-zeolites. J Mater Inf 2023;3:19.
74. Pu Y, Liu Y, Liu D, et al. First-principles screening visible-light active delafossite ABO2 structures for photocatalytic application. Int J Hydrog Energy 2018;43:17271-82.
75. Liu Y, Lu Y, Wang WY, et al. Effects of solutes on thermodynamic properties of (TMZrU)C (TM = Ta, Y) medium-entropy carbides: a first-principles study. J Mater Inf 2023;3:17.
76. Xu L, Zeng J, Li Q, et al. Insight into enhanced visible-light photocatalytic activity of SWCNTs/g-C3N4 nanocomposites from first principles. Appl Surf Sci 2020;530:147181.
77. Liu J, Li W, Li H, et al. A novel detection method for sulfur content in ship fuel based on metal-doped tin oxide quantum dots as fluorescent sensor. Fuel 2024;357:129739.
78. Shen H, Ouyang T, Guo J, Mu M, Yin X. A perspective LDHs/Ti3C2O2 design by DFT calculation for photocatalytic reduction of CO2 to C2 organics. Appl Surf Sci 2023;609:155445.
79. Wang G, Dong X, Cheng M, et al. DFT predirected molecular engineering design of donor-acceptor structured g-C3N4 for efficient photocatalytic tetracycline abatement. Small 2024;20:e2311798.
80. Vu TV, Anh NTT, Hoat D, et al. Electronic, optical and photocatalytic properties of fully hydrogenated GeC monolayer. Physica E 2020;117:113857.
81. Zhao Y, Wang W, Li C, et al. Enhanced photocatalytic activity of nonmetal doped monolayer MoSe2 by hydrogen passivation: first-principles study. Appl Surf Sci 2018;456:133-9.
82. Tang C, Chen C, Zhang H, Zhang J, Li Z. Enhancement of degradation for nitrogen doped zinc oxide to degrade methylene blue. Physica B 2020;583:412029.
83. Su K, Xu X, Lai G, et al. First-principles investigation of the elastic, photocatalytic and ferroelectric properties of LiNbO3-type LiSbO3 under high pressure. Mater Today Commun 2021;27:102406.
84. Dong S, Li Y, Zhang X, et al. Pt single-atom loaded on nonmetallic elements (C, N, P, S) doped ZrO2 in photocatalytic hydrogen evolution: first principles. Mater Des 2023;231:112068.
85. Gong M, Yin H, Lyu P, Sun L. Single transition metal atoms anchored on a two-dimensional polyimide covalent-organic framework as single-atom catalysts for photocatalytic CO2 reduction: a first-principles study. Catal Commun 2023;175:106604.
86. Zhu L, Qin C, Wang Y, Cao J. Single-atom Pt supported on non-metal doped WS2 for photocatalytic CO2 reduction: a first-principles study. Appl Surf Sci 2023;626:157252.
87. Liu J, Zhang H, Li Y, et al. Enhanced Vis-NIR light absorption and thickness effect of Mo-modified SnO2 thin films: a first principle calculation study. Results Phys 2021;23:103997.
88. Zhu Z, Tang X, Wang T, et al. Insight into the effect of co-doped to the photocatalytic performance and electronic structure of g-C3N4 by first principle. Appl Catal B Environ 2019;241:319-28.
89. Ren J, Zhang J, Tian B, et al. First-principles study of the electronic, optical adsorption, and photocatalytic water-splitting properties of a strain-tuned SiC/WS2 heterojunction. Int J Hydrog Energy 2024;87:554-65.
90. Zhang R, Jian W, Yang Z, Bai F. Insights into the photocatalytic mechanism of the C4N/MoS2 heterostructure: a first-principle study. Chin Chem Lett 2020;31:2319-24.
91. Ga S, An N, Lee GY, Joo C, Kim J. Multidisciplinary high-throughput screening of metal-organic framework for ammonia-based green hydrogen production. Renew Sustain Energy Rev 2024;192:114275.
92. Mooraj S, Chen W. A review on high-throughput development of high-entropy alloys by combinatorial methods. J Mater Inf 2023;3:4.
93. Yang H, Che Y, Cooper AI, Chen L, Li X. Machine learning accelerated exploration of ternary organic heterojunction photocatalysts for sacrificial hydrogen evolution. J Am Chem Soc 2023;145:27038-44.
94. Sa B, Hu R, Zheng Z, et al. High-throughput computational screening and machine learning modeling of janus 2D III-VI van der Waals heterostructures for solar energy applications. Chem Mater 2022;34:6687-701.
95. Singh AK, Montoya JH, Gregoire JM, Persson KA. Robust and synthesizable photocatalysts for CO2 reduction: a data-driven materials discovery. Nat Commun 2019;10:443.
96. Wang Y, Brocks G, Er S. Data-driven discovery of intrinsic direct-gap 2D materials as potential photocatalysts for efficient water splitting. ACS Catal 2024;14:1336-50.
97. Côté P, Nikanjam A, Ahmed N, Humeniuk D, Khomh F. Data cleaning and machine learning: a systematic literature review. Autom Softw Eng 2024;31:453.
98. Ge C, Gao Y, Miao X, Yao B, Wang H. A hybrid data cleaning framework using markov logic networks. IEEE Trans Knowl Data Eng 2022;34:2048-62.
99. Bernhardt M, Castro DC, Tanno R, et al. Active label cleaning for improved dataset quality under resource constraints. Nat Commun 2022;13:1161.
100. Lima FT, Souza VM. A large comparison of normalization methods on time series. Big Data Res 2023;34:100407.
101. Chen S, Guo W. Auto-encoders in deep learning-a review with new perspectives. Math 2023;11:1777.
102. Li P, Pei Y, Li J. A comprehensive survey on design and application of autoencoder in deep learning. Appl Soft Comput 2023;138:110176.
103. Xie J, Sage M, Zhao YF. Feature selection and feature learning in machine learning applications for gas turbines: a review. Eng Appl Artif Intell 2023;117:105591.
104. Wang J, Xu P, Ji X, Li M, Lu W. Feature selection in machine learning for perovskite materials design and discovery. Materials 2023;16:3134.
105. Wang C, Wan Y, Yang S, et al. Revealing the untapped potential of photocatalytic overall water splitting in metal organic frameworks. Adv Funct Mater 2024;34:2313596.
106. Wan Y, Ramirez F, Zhang X, Nguyen T, Bazan GC, Lu G. Data driven discovery of conjugated polyelectrolytes for optoelectronic and photocatalytic applications. npj Comput Mater 2021;7:541.
107. Baaloudj O, Nasrallah N, Bouallouche R, Kenfoud H, Khezami L, Assadi AA. High efficient Cefixime removal from water by the sillenite Bi12TiO20: photocatalytic mechanism and degradation pathway. J Clean Prod 2022;330:129934.
108. Parida VK, Srivastava SK, Chowdhury S, Gupta AK. Facile synthesis of 2D/0D Bi2O3/MnO2 Z-scheme heterojunction for enhanced visible light-assisted photocatalytic degradation of acetaminophen. Chem Eng J 2023;472:144969.
109. Li J, Liu X, Wang H, Sun Y, Dong F. Prediction and interpretation of photocatalytic NO removal on g-C3N4-based catalysts using machine learning. Chin Chem Lett 2024;35:108596.
110. Fathinia M, Khataee A, Aber S, Naseri A. Development of kinetic models for photocatalytic ozonation of phenazopyridine on TiO2 nanoparticles thin film in a mixed semi-batch photoreactor. Appl Catal B Environ 2016;184:270-84.
111. Amani-ghadim A, Dorraji MS. Modeling of photocatalyatic process on synthesized ZnO nanoparticles: Kinetic model development and artificial neural networks. Appl Catal B Environ 2015;163:539-46.
112. K C A, Rao CS, Nair V. Combination of ensemble machine learning models in photocatalytic studies using nano TiO2 - Lignin based biochar. Chemosphere 2024;352:141326.
113. Wang Y, Sorkun MC, Brocks G, Er S. ML-aided computational screening of 2D materials for photocatalytic water splitting. J Phys Chem Lett 2024;15:4983-91.
114. Rashtbari S, Dehghan G, Marefat A, Khataee S, Khataee A. Proficient sonophotocatalytic degradation of organic pollutants using
115. Li Z, Li H, Meng L. Model compression for deep neural networks: a survey. Computers 2023;12:60.
116. Fu C, Li H, Li W, et al. Rapid detection of trace sulfur content in ship fuel oil based on tin oxide quantum dot fluorescent sensors assisted by multi-column convolutional neural network. Microchem J 2024;205:111396.
117. Sethi S, Dhir A, Arora V. Photocatalysis based hydrogen production and antibiotic degradation prediction using neural networks. Reac Kinet Mech Cat 2023;136:3283-97.
118. Kakhki R, Zirjanizadeh S, Mohammadpoor M. A review of clinoptilolite, its photocatalytic, chemical activity, structure and properties: in time of artificial intelligence. J Mater Sci 2023;58:10555-75.
119. Isazawa T, Cole JM. How beneficial is pretraining on a narrow domain-specific corpus for information extraction about photocatalytic water splitting? J Chem Inf Model 2024;64:3205-12.
120. Aid L, Abbou MS, Gafour AR, et al. Data-augmenting self-attention network for predicting photocatalytic degradation efficiency: a study on TiO2/curcumin nanocomposites. Reac Kinet Mech Cat 2024;137:3499-516.
121. Li J, Shi H, Li Z, et al. Interaction of metal ions in high efficiency seawater hydrogen peroxide production by a carbon-based photocatalyst. Appl Catal B Environ 2024;343:123541.
122. Hayashi Y, Nagai Y, Pan Z, Katayama K. Convolutional neural network prediction of the photocurrent-voltage curve directly from scanning electron microscopy images†. J Mater Chem A 2023;11:22522-32.
123. Schmidt-Hieber J. The Kolmogorov-Arnold representation theorem revisited. Neural Netw 2021;137:119-26.
124. Wang C, Tan X, Zhu B, et al. Deep learning-assisted non-invasive pediatric tic disorder diagnosis using EEG features extracted by residual neural networks. J Radiat Res Appl Sci 2024;17:101151.
125. He H, Wang Y, Qi Y, Xu Z, Li Y, Wang Y. From prediction to design: recent advances in machine learning for the study of 2D materials. Nano Energy 2023;118:108965.
126. Tao Q, Lu T, Sheng Y, Li L, Lu W, Li M. Machine learning aided design of perovskite oxide materials for photocatalytic water splitting. J Energy Chem 2021;60:351-9.
127. Huang M, Wang S, Zhu H. A comprehensive machine learning strategy for designing high-performance photoanode catalysts. J Mater Chem A 2023;11:21619-27.
128. Lin Z, Li Y, Haque SA, Ganose AM, Kafizas A. Insights from experiment and machine learning for enhanced TiO2 coated glazing for photocatalytic NOx remediation†. J Mater Chem A 2024;12:13281-98.
129. Tamtaji M, Guo X, Tyagi A, et al. Machine learning-aided design of gold core-shell nanocatalysts toward enhanced and selective photooxygenation. ACS Appl Mater Interfaces 2022;14:46471-80.
130. Miodyńska M, Mikolajczyk A, Mazierski P, et al. Lead-free bismuth-based perovskites coupled with g-C3N4: a machine learning based novel approach for visible light induced degradation of pollutants. Appl Surf Sci 2022;588:152921.
131. Chen F, Yang Y, Chen X. A first-principles and machine learning study on design of graphitic carbon nitride-based single-atom photocatalysts. ACS Appl Nano Mater 2024;7:11862-70.
132. Choudhary K, Garrity KF. InterMat: accelerating band offset prediction in semiconductor interfaces with DFT and deep learning. Digital Discov 2024;3:1365-77.
133. Guevarra D, Zhou L, Richter MH, et al. Materials structure-property factorization for identification of synergistic phase interactions in complex solar fuels photoanodes. npj Comput Mater 2022;8:747.
134. Jiang Z, Hu J, Samia A, Yu X. Predicting active sites in photocatalytic degradation process using an interpretable molecular-image combined convolutional neural network. Catalysts 2022;12:746.
135. Zhou Y, Wang X, Huang X, Deng H, Hu Y, Lu L. Predicting the photosynthetic ammonia on nanoporous cobalt zirconate via graph convolutional neural networks. Mol Catal 2022;529:112565.
136. Bonke SA, Trezza G, Bergamasco L, et al. Multi-variable multi-metric optimization of self-assembled photocatalytic CO2 reduction performance using machine learning algorithms. J Am Chem Soc 2024;146:15648-58.
137. Wang S, Mo P, Li D, Syed A. Intelligent algorithms enable photocatalyst design and performance prediction. Catalysts 2024;14:217.
138. Liu Y, Ge Q, Wang T, et al. Investigating the impact of pretreatment strategies on photocatalyst for accurate CO2RR productivity quantification: a machine learning approach. Chem Eng J 2023;473:145255.
139. Jaffari ZH, Abbas A, Lam SM, et al. Machine learning approaches to predict the photocatalytic performance of bismuth ferrite-based materials in the removal of malachite green. J Hazard Mater 2023;442:130031.
140. Özsoysal S, Oral B, Yıldırım R. Analysis of photocatalytic CO2 reduction over MOFs using machine learning. J Mater Chem A 2024;12:5748-59.
141. Yang Y, Zheng Y, Liu S, et al. Deep learning prediction of photocatalytic water splitting for hydrogen production under natural light based on experiments. Energy Convers Manage 2024;301:118007.
142. Navidpour AH, Hosseinzadeh A, Huang Z, Li D, Zhou JL. Application of machine learning algorithms in predicting the photocatalytic degradation of perfluorooctanoic acid. Catal Rev 2024;66:687-712.
143. Lira JO, Riella HG, Padoin N, Soares C. Computational fluid dynamics (CFD), artificial neural network (ANN) and genetic algorithm (GA) as a hybrid method for the analysis and optimization of micro-photocatalytic reactors: NOx abatement as a case study. Chem Eng J 2022;431:133771.
144. Li X, Maffettone PM, Che Y, Liu T, Chen L, Cooper AI. Combining machine learning and high-throughput experimentation to discover photocatalytically active organic molecules. Chem Sci 2021;12:10742-54.
145. Parmar N, Srivastava JK. Process optimization and kinetics study for photocatalytic ciprofloxacin degradation using TiO2 nanoparticle: a comparative study of artificial neural network and surface response methodology. J Indian Chem Soc 2022;99:100584.
146. Malayeri M, Nasiri F, Haghighat F, Lee C. Optimization of photocatalytic oxidation reactor for air purifier design: application of artificial neural network and genetic algorithm. Chem Eng J 2023;462:142186.
147. Truong H, Cuong Nguyen X, Hur J. Recent advances in g-C3N4-based photocatalysis for water treatment: magnetic and floating photocatalysts, and applications of machine-learning techniques. J Environ Manage 2023;345:118895.
148. Saadetnejad D, Oral B, Can E, Yıldırım R. Machine learning analysis of gas phase photocatalytic CO2 reduction for hydrogen production. Int J Hydrog Energy 2022;47:19655-68.
149. Gordanshekan A, Arabian S, Solaimany Nazar AR, Farhadian M, Tangestaninejad S. A comprehensive comparison of green Bi2WO6/g-C3N4 and Bi2WO6/TiO2 S-scheme heterojunctions for photocatalytic adsorption/degradation of Cefixime: artificial neural network, degradation pathway, and toxicity estimation. Chem Eng J 2023;451:139067.
150. Anandhi G, Iyapparaja M. Photocatalytic degradation of drugs and dyes using a maching learning approach. RSC Adv 2024;14:9003-19.
151. Liu Q, Pan K, Zhu L, et al. Ensemble learning to predict solar-to-hydrogen energy conversion based on photocatalytic water splitting over doped TiO2†. Green Chem 2023;25:8778-90.
152. Park H, Bentria ET, Rtimi S, Arredouani A, Bensmail H, El-mellouhi F. Accelerating the design of photocatalytic surfaces for antimicrobial application: machine learning based on a sparse dataset. Catalysts 2021;11:1001.
153. Kim CM, Jaffari ZH, Abbas A, Chowdhury MF, Cho KH. Machine learning analysis to interpret the effect of the photocatalytic reaction rate constant (k) of semiconductor-based photocatalysts on dye removal. J Hazard Mater 2024;465:132995.
154. Biswas T, Singh AK. Excitonic effects in absorption spectra of carbon dioxide reduction photocatalysts. npj Comput Mater 2021;7:640.
155. Jeong H, Yun B, Na S, et al. Multimodal deep learning models incorporating the adsorption characteristics of the adsorbent for estimating the permeate flux in dynamic membranes. J Membr Sci 2024;709:123105.
156. Zhang Z, Yang Z, Zhao Z, Liu Y, Wang C, Xu W. Multimodal deep-learning framework for accurate prediction of wettability evolution of laser-textured surfaces. ACS Appl Mater Interfaces 2023;Online ahead of print.
157. Liang W, Huang J, Sun J, Zhang P, Li A. Multiscale modeling and simulation of surface-enhanced spectroscopy and plasmonic photocatalysis. WIREs Comput Mol Sci 2023;13:e1665.
158. Kovačič Ž, Likozar B, Huš M. Photocatalytic CO2 reduction: a review of Ab initio mechanism, kinetics, and multiscale modeling simulations. ACS Catal 2020;10:14984-5007.
159. Gusarov S. Advances in computational methods for modeling photocatalytic reactions: a review of recent developments. Materials 2024;17:2119.
160. Loh JYY, Wang A, Mohan A, et al. Leave no photon behind: artificial intelligence in multiscale physics of photocatalyst and photoreactor design. Adv Sci 2024;11:e2306604.
161. Oliveira GX, Kuhn S, Riella HG, Soares C, Padoin N. Combining computational fluid dynamics, photon fate simulation and machine learning to optimize continuous-flow photocatalytic systems. React Chem Eng 2023;8:2119-33.
162. Huang G, Guo Y, Chen Y, Nie Z. Application of machine learning in material synthesis and property prediction. Materials 2023;16:5977.
Cite This Article
How to Cite
Liu, J.; Liang, L.; Su, B.; Wu, D.; Zhang, Y.; Wu, J.; Fu, C. Transformative strategies in photocatalyst design: merging computational methods and deep learning. J. Mater. Inf. 2024, 4, 33. http://dx.doi.org/10.20517/jmi.2024.48
Download Citation
Export Citation File:
Type of Import
Tips on Downloading Citation
Citation Manager File Format
Type of Import
Direct Import: When the Direct Import option is selected (the default state), a dialogue box will give you the option to Save or Open the downloaded citation data. Choosing Open will either launch your citation manager or give you a choice of applications with which to use the metadata. The Save option saves the file locally for later use.
Indirect Import: When the Indirect Import option is selected, the metadata is displayed and may be copied and pasted as needed.
About This Article
Special Issue
Copyright
Data & Comments
Data
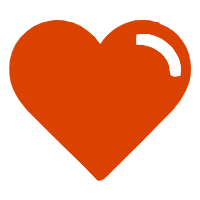
Comments
Comments must be written in English. Spam, offensive content, impersonation, and private information will not be permitted. If any comment is reported and identified as inappropriate content by OAE staff, the comment will be removed without notice. If you have any queries or need any help, please contact us at support@oaepublish.com.