Establishing a biomonitoring baseline by characterizing the hair metabolome across age and sex using high-resolution mass spectrometry
Abstract
Hair provides an excellent matrix for long-term biomonitoring due to its chemical accumulation during growth. Despite recent uses of hair for biomonitoring to characterize the chemical exposome across demographics, no established baseline exists for the hair metabolome based on age and sex. This study aimed to establish a baseline for the hair metabolome influenced by age and sex, utilizing an Orbitrap mass spectrometer, a high-resolution mass spectrometry (HRMS) technique. We collected hair samples from 48 participants divided by age and sex into four groups: elderly males, elderly females, young males, and young females. Metabolic profiling was conducted using ultrahigh-performance liquid chromatography coupled with Q ExactiveTM Plus Orbitrap mass spectrometer. Our analysis revealed significant age- and sex-dependent variations in metabolite profiles. Volcano plots highlighted the differential metabolic features between groups, with age showing a stronger influence on metabolic variations in females and sex in younger individuals. We identified 205 chemical compounds affected by age and/or sex, with a significant portion showing overlap in their influence. Pathway enrichment analysis pinpointed perturbations in 41 metabolic pathways, including those involved in lipid metabolism, amino acid turnover, and hormone-associated pathways. Notably, the pathways of arachidonic acid metabolism and fatty acid biosynthesis were consistent with known age and sex influences. Our findings underscore the potential of using hair metabolomics for comprehensive environmental exposure assessment and health research, offering insights into the biological impact of age and sex on the human metabolome.
Keywords
INTRODUCTION
Biomonitoring is an approach used to assess the doses of environmental pollutants to which humans are exposed by measuring either the parent compounds or their corresponding biotransformation products in biospecimens following the absorption of these chemicals into the body[1,2]. This approach is integral for public health investigations as it provides direct evidence of the actual exposure of individuals to environmental pollutants, rather than estimates based on environmental samples[2]. A recent study investigated the levels of 59 persistent organic pollutants (POPs) in plasma, finding that p,p’-DDE, HCB, and β-HCH were detected and quantified in more than 95% of the samples[3]. This underscores the importance of continuous biomonitoring investigations to track these substances within human populations and evaluate the effectiveness of environmental policies. Biomonitoring has been widely applied in evaluating the contamination of ecosystems and assists in the regulatory assessment of chemicals. It is particularly useful in occupational health for monitoring workers’ exposure to hazardous substances[4-6]. In one study, 14 structure-related metabolite signals were quantified in urine samples from five rubber workers using ultra-high-performance liquid chromatography coupled with high-resolution mass spectrometry/mass spectrometry (UHPLC-HRMS/MS)[6]. Additionally, another recent report revealed the relationship between the levels of naphthalene in the air and those of its exposure markers in urine samples, showing that 1-NMA, 1,2-DHN, 1-and 2-naphthol are suitable exposure for assessing occupational exposure to naphthalene[7]. Recently, biomonitoring has been extended to include large-scale population assessments, such as the National Report on Human Exposure to Environmental Chemicals in the United States. This survey evaluates chemicals known to have adverse health effects across different demographic groups[8].
Blood and urine are commonly used as biological samples in biomonitoring studies. According to the National Report on Human Exposure to Environmental Chemicals, hydrophobic parent compounds such as per- and polyfluoroalkyl substances are primarily detected in serum due to their protein-binding properties and poor water solubility[9]. Although these compounds are less frequently detected in urine, trace amounts can still be found depending on the sensitivity of the analytical methods used. Additionally, the biotransformation products formed when environmental pollutants undergo biotransformation reactions in the human body are more soluble in urine. For instance, phthalate metabolites are commonly used as exposure markers to evaluate phthalate ester exposure in biomonitoring investigations, as the half-life of phthalate esters is approximately 12 h in urine[10]. Consequently, both blood and urine samples are valuable for biomonitoring investigations. However, the variability in exposure times and the fluctuations in chemical compound levels in these matrices can complicate the profiling of environmental chemical alterations over extended periods[11-13]. In contrast, hair serves as an excellent matrix for long-term biomonitoring due to the accumulation of chemicals during its growth[14-16]. A study indicated that hair allows the detection of both parent pesticides and hydrophilic metabolites, while urine can only be applied for hydrophilic chemicals[17]. Additionally, the exposure markers of phthalate esters could be detected and quantified in rat and human hair samples[18,19]. Moreover, 28 exposure markers of DPHP were identified in the hair matrix, whereas only 7 exposure markers of DPHP were detected in the urine 30 days post-exposure in rats[20]. This disparity underscores the utility of hair for long-term determination of exposure markers in biomonitoring. A 1-cm hair segment typically represents approximately one month of exposure markers and endogenous metabolite accumulation, providing a stable record of exposure[21,22]. Segmented hair analysis, based on the average growth rate of hair, can offer insights into metabolic changes over time[21]. Thus, hair provides a broader detection window and a more enduring record of metabolite accumulation compared to blood and urine in biomonitoring investigations.
Hair has recently been utilized for biomonitoring investigations to characterize the chemical exposome across various demographic populations[23-27]. Variations in the human exposome might be influenced by numerous factors, such as genetics, ethnicity, age, and sex[28-30]. Among these, age and sex significantly affect hormone levels, enzyme activities, and metabolic rates, which can introduce potential confounding factors when characterizing long-term chemical exposome and conducting biomonitoring investigations. Establishing a baseline associated with age and sex by characterizing hair metabolome is essential for using hair in environmental exposure assessment across different demographic groups. This approach might eliminate the confounding factors related to age and sex in biomonitoring investigations. However, a baseline for the hair metabolome based on age and sex has not yet been established in humans. Metabolomics, an omics approach that focuses on identifying small molecules (< 1,500 Da) in biological systems, has become a suitable strategy for exploring hair metabolome baseline for biomonitoring investigations[31]. In this study, we aimed to establish a baseline of the metabolic profile in human hair across age and sex using high-resolution mass spectrometry (HRMS).
EXPERIMENTAL
Study design
We aimed to explore the differences of metabolites in human hair based on age and sex using HRMS-based metabolomics approach for developing a baseline for biomonitoring investigation. This study design is shown in Figure 1. This study was divided into three parts: hair collection and preparation, data acquisition and processing, and statistical analysis, identification, distribution, and metabolic pathway search.
Figure 1. Study design for determining a biomonitoring baseline by characterizing the hair metabolome across age and sex. A study design for exploring the variations of metabolomic profiles with age and sex in human hair by UHPLC-HRMS. Hair samples from the elder group (12 males and 12 females) and the younger group (12 males and 12 females) were collected and subjected to untargeted metabolomics analysis. After hair sample decontamination, homogenization, and extraction, the hair extracts were analyzed with UHPLC-HRMS. The raw MS data were converted to peak lists using MS-DIAL for feature detection, alignment, and compound identification to explore chemical structure, and then, the metabolic pathways were analyzed. UHPLC-HRMS: Ultra-high-performance liquid chromatography coupled with high-resolution mass spectrometry; MS: mass spectrometry.
In the Hair collection and preparation section, hair samples were collected from a diverse group of 48 volunteers, categorized into four equal groups: old males (OM), old females (OF), young males (YM), and young females (YF). The participants were enlisted from the National Cheng Kung University and its corresponding hospital located in Tainan, Taiwan. Hair metabolites were extracted using procedures established and optimized previously[32].
In the data acquisition and processing section, the samples including hair extract samples, an instrumental analysis QC sample, sample preparation QC samples, and solvent blank were subjected to UHPLC-HRMS analysis. These were then analyzed using UHPLC-HRMS analysis in full scan mode to obtain the mass spectrometry signals. The reverse phase column was used for hair metabolome profiling. The data were imported into the MS-DIAL for data processing[33]. The data processing procedure included peak detection and peak alignment.
In statistical analysis, identification, distribution, and metabolic pathway search section, the discriminatory features associated with age and sex were filtered by univariate analysis using Student’s t test. The discriminatory features were determined by |log 2 (Fold change)| ≥ 1 with P values calculated by t test below 0.05. The discriminatory features underwent extra fragmentation analysis to obtain the MS/MS spectrum for chemical identification. For chemical identification, the MS/MS spectral database search is performed for identifying chemical compounds according to a previous study[34]. The MS/MS spectral database was constructed by experimental MS/MS spectrum of reference authentic standards (Massbank of North America) and in silico predicted MS/MS spectrum (MS-FINDER)[35]. The chemical compounds showing significant alternation across age and sex were subjected to pathway enrichment analysis using MetaboAnalyst 5.0 to discover the metabolic pathways associated with age or sex.
Hair sampling and metabolite extraction
Hairs were cut 0.1 cm away from the scalp with scissors, firmed in aluminum foil, and stored at 4 °C until further analysis. Throughout the collection phase, each participant signed a written informed consent form, complying with the protocols established by the Institutional Review Board of National Cheng Kung University Hospital (IRB approval numbers A-ER-107-373 and B-ER-108-188). The washing procedure is a mandatory step to remove contaminants deposited on the hair shaft according to the Hair Testing Association guidelines. Twenty milligrams of hair samples were weighed in a glass tube, washed with acetone (3 mL), and then with deionized water (DIW, 3 mL) in an ultrasonic bath for 2 min each. The wash solution was then discarded, and the samples were dried under nitrogen. Afterward, the dried hair samples were cut into small pieces with a pair of scissors and subjected to the extraction procedure. Six milligrams of washed hair samples were mixed with 300 μL of methanol: phosphate buffer saline (PBS) 50:50 (v/v) solvent and extracted for 240 min at 55 °C with 37k Hz for ultrasonic-associated extraction. After that, the extracts were centrifuged at 15,000 × g for 15 min and the supernatants were collected. The hair extract was then evaporated to dryness by SpeedVac (EYELA, cue-2200) and the residue was reconstituted with 50% MeOH (30 µL). An instrumental quality control (QC) sample for monitoring the robustness of instrumental analysis was prepared by pooling 5 μL of each of 48 hair extracts, resulting in a total pooled hair extract of approximately 240 μL. This sample was analyzed after every 4 hair extract injections, with a total of 12 instrumental QC samples analyzed within the analytical sequence [Supplementary Table 1]. Regarding the QC procedure for sample preparation, a pooled QC sample was prepared by combining approximately 10 mg of each of 48 hair samples, resulting in a total pooled sample weighing approximately 480 mg. From this sample, five replicates of 6 mg each were subjected to the sample preparation procedure described previously. Moreover, to evaluate the levels of noise signals from the extraction solvent, methanol: phosphate buffer saline (PBS) 50:50 (v/v) solvent underwent the same extraction procedure as the hair sample with triplicate.
UHPLC-HRMS analysis
A UHPLC system coupled with a Q Exactive orbitrap mass spectrometer system (Thermo Fisher Scientific) was used for sample analysis. Chromatographic separation was performed on a Waters Acquity UPLC BEH C18 column (2.1 mm × 100 mm, 1.7 μm). The mobile phase consisted of (A) 2% acetonitrile (ACN) in DIW with 0.1% formic acid and (B) 100% ACN with 0.1% formic acid. The gradient conditions were as follows:
Data processing, statistical analysis, and metabolic pathway enrichment analysis
The raw data collected by HRMS were converted to the “.mzXML” format by MSconverter, and the converted files were further converted into “.abf” format. The converted files were imported into MS-DIAL 4.48[33] and were processed for feature detection, alignment, and identification. During alignment, the tolerance for retention time was set at 0.2 min and m/z value was set at 0.0015 Da. Features with S/N < 3 were considered as the absence of peaks and hence filtered. Each raw abundance value was normalized by dividing it based on the sum of the raw abundance values of all peaks in the corresponding sample before performing a univariate analysis to discover the differential features. Discriminatory features were filtered by |log2 (fold-change)| ≥ 1 and P ≤ 0.05. The discriminatory features were subjected to extra fragmentation analysis for chemical identification. Chemical identification was performed based on the accuracy of precursor mass and tandem mass spectrometry (MS/MS) fragmentation spectral matching using a combination of different tools, including MS-DIAL with MassBank of North America (MoNA) database which was downloaded on 1 June 2023 and MS-FINDER 3.52 with PubChem, Human Metabolome Database (HMDB), and LIPID MAPS as the backend knowledge base. For performing the MS-DIAL with MoNA database, a cutoff value for MS/MS spectral match score was set at 70 (Maximum = 100). For performing MS-FINDER 3.52, a cutoff value of match score was set at 7 (maximum = 10).
The confidence level of metabolite identification was defined using the criteria reported by Schymanski et al.[36]. The chemical structures of the discriminatory features were determined using the MS/MS analysis, but it provided insufficient information for one single exact structure, assigned as a level-3 annotation. Only one chemical structure of the discriminatory features was determined using the MS/MS analysis, assigned as a level-2 annotation. The Classyfire was used to classify the categories of the identified discriminatory chemical compounds based on their chemical structures[37]. The InChI strings of the chemical compounds were retrieved by the package “webchem” using R 4.4.3. Subsequently, the InChI was imported into Classyfire to obtain the chemical categories. To determine the alterations in metabolic pathways related to age and sex, we used the online software MetaboAnalyst 5.0[38] to perform pathway enrichment analysis. The metabolites collect the detectable specimen sources using the online database HMDB[39].
RESULTS AND DISCUSSION
Volcano plots of age- and sex-dependent differential features and identification of differential features
To explore a biomonitoring baseline in human hair across age and sex, we enlisted 48 volunteers divided into two distinct age groups: 61~70 years old (Old group, N = 24) and 18~27 years old (Young group,
Characteristics of study participants
Characteristic | 61~70 years old | P (OM vs. OF) | 18~27 years old | P (YM vs. YF) | |||||
Total | OM | OF | Total | YM | YF | ||||
Age (years, mean ± SD) | 65.2 ± 2.8 | 65.2 ± 2.2 | 65.3 ± 2.7 | 0.94 | 23.5 ± 2.4 | 23.5 ± 1.9 | 23.4 ± 2.9 | 0.92 | |
BMI (kg/m2, mean ± SD) | 25.9 ± 4.1 | 26.6 ± 4 | 25.2 ± 4.4 | 0.44 | 21.7 ± 3.4 | 20.4 ± 2.8 | 23.4 ± 3.6 | 0.06 | |
Cosmetic hair treatment | Never | 11 | 11 | 0 | < 0.05 | 22 | 11 | 7 | 0.09 |
Dyeing | 11 | 1 | 10 | 0 | 0 | 4 | |||
Perming | 0 | 0 | 0 | 2 | 1 | 1 | |||
Both | 2 | 0 | 2 | 0 | 0 | 0 | |||
Smoking status | Never | 21 | 9 | 12 | 0.06 | 24 | 12 | 12 | 1.00 |
Current | 0 | 0 | 0 | 0 | 0 | 0 | |||
Past | 3 | 3 | 0 | 0 | 0 | 0 | |||
Alcohol consumption | Never | 20 | 9 | 11 | 0.46 | 20 | 9 | 11 | 0.27 |
Current | 3 | 2 | 1 | 4 | 3 | 1 | |||
Past | 1 | 1 | 0 | 0 | 0 | 0 |
The samples, including 48 hair extracts, 1 instrumental QC sample, 5 sample preparation QC sample, and 3 blank samples, were analyzed using UHPLC-HRMS in both positive and negative ion modes. For evaluating the analytical reproducibility by instrumental analysis and sample preparation, the principal component analysis (PCA) was performed [Supplementary Figure 1]. The blue and pink dots in Supplementary Figure 1 represent the 6 injections of instrumental QC sample and 5 replicates of sample preparation QC sample, respectively. It was shown that not only the 6 injections of instrumental QC samples but also 5 sample preparation QC samples were clustered tightly in the plot, indicating that minimal technical errors were presented in instrumental analysis and sample preparation procedure. Significant changes in peak abundance due to age and sex (|log2 (fold-change)| ≥ 1 and P-value ≤ 0.05) are depicted in volcano plots
Figure 2. Volcano plots for filtering age-dependent features. Volcano plots illustrated the perturbed features between elders and youngers in (A) positive mode and (B) negative ion mode; In addition, the volcano plots show the comparison of OM and YM in (C) positive and (D) negative ion modes, and the comparison of OF and YF in (E) positive and (F) negative ion modes. The dark blue spot represents the features displayed with larger magnitude fold changes (x-axis, |log2(elder/younger)| ≥ 1.0) and statistical significance difference
Figure 3. Volcano plots for screening sex-dependent features. Volcano plots illustrated the perturbed features between females and males in (A) positive mode and (B) negative ion mode; In addition, the volcano plots show the comparison of OF and OM in (C) positive and (D) negative ion modes, and the comparison of YF and YM in (E) positive and (F) negative ion modes. The dark blue spot represents the features displayed with larger magnitude fold changes (x-axis, |log2(elder/younger)| ≥ 1.0) and statistical significance difference (y-axis, -log P-value ≥ 1.33). OF: Old females; OM: old males; YF: young females; YM: young males.
The peaks with significant abundance changes were subjected to tandem mass spectrometry (MS/MS) for chemical structure identification. Two hundred and five chemical compounds were identified, with 71 chemical compounds being identified through MS-DIAL with MoNA database and 134 chemical compounds being identified by MS-FINDER with the HMDB, PubChem, and LIPID MAPS databases. These chemical compounds are listed in Supplementary Table 2. Among them, 96 chemical compounds were associated with age, 45 chemical compounds were related to sex, and 64 chemical compounds were linked to both age and sex. This comprehensive set of metabolites defines the baseline metabolic profile for environmental exposure assessment across different demographic groups. The established baseline highlights significant age- and sex-related variations in metabolic profiles, emphasizing the need to consider these demographic factors in biomonitoring studies. This comprehensive baseline serves as a valuable tool for further research, facilitating more accurate assessments of environmental exposure and its potential health impacts.
In recent years, hair has been increasingly used for biomonitoring investigations. Therefore, the 205 baseline compounds identified in hair were compared with those reported in human urine or blood. Using the Human Metabolome Database (HMDB) for comparison, 140 of the 205 baseline compounds are recorded in HMDB[39]. Among the 140 hair baseline metabolites, 70 of these compounds have been identified in human blood, and 66 of these chemicals have been identified in human urine. A total of 77 of these compounds have previously been detected in urine or blood. This comparison highlighted that the chemical composition in human hair might differ from that in urine or blood. The chemicals were incorporated into human hair matrix and accumulated over extended periods, reflecting long-term chemical exposure and metabolic changes, whereas urine and blood typically represent more immediate metabolic states. These differences underscore the importance of understanding the unique metabolic profiles captured by different biological matrices and the potential implications for biomonitoring and environmental exposure assessments.
Taxonomic distribution of chemical compounds
To elucidate the relationship between sex-dependent and age-dependent compounds among the 205 identified chemicals, a Venn diagram is presented in Figure 4A. Of these, 96 compounds (46.8%) are significantly influenced by age, 45 (22%) by sex, and 64 (31.2%) by both age and sex. The taxonomic distribution of the 205 chemical compounds is categorized into 12 classes based on chemical entities [Figure 4B]. These classes include lipid and lipid-like molecules (26.3%, 54 compounds), organoheterocyclic compounds (22.9%, 47 compounds), organic acids and derivatives (16.6%, 34 compounds), benzenoids (10.7%, 22 compounds), phenylpropanoids and polyketides (6.8%, 14 compounds), organic nitrogen compounds (5.9%, 12 compounds), organic oxygen compounds (2%, 4 compounds), nucleosides, nucleotides, and analogs (2%, 4 compounds), alkaloids and derivatives (1.6%, 4 compounds), hydrocarbons (1.5%, 3 compounds), lignans, neolignans, and related compounds (0.5%, 1 compound), and organosulfur compounds (0.5%, 1 compound). This distribution indicates that both non-polar compounds, such as lipids and lipid-like molecules, as well as polar compounds, like organoheterocyclic compounds and organic acids and derivatives, are detectable in hair metabolism.
Figure 4. Venn diagram of age- and sex-dependent metabolites and pie chart of compounds classification. (A) The numbers of age-dependent, sex-dependent, and both age- and sex-dependent metabolites are shown in Venn diagram; (B) Taxonomic distribution of chemical compounds was classified and illustrated by pie chart. The numbers of features are indicated in brackets.
To investigate the influence of environmental exposure or diets on metabolites related to age/sex, a total of 9 major metabolites, such as amino acids and lipids and 8 environmental exposure or diet-related chemicals were selected for the correlation analysis [Supplementary Figure 2]. The diethyl phthalate, monobutyl phthalate, and deisopropylatrazine were selected to represent environmental exposure, and alpha-linolenic acid, arachidonic acid, cinnamic acid, equol, and piperettine represented dietary chemicals. The heatmap demonstrated that most environmental pollutants/ dietary chemicals and the metabolites associated with age/sex do not exhibit a high correlation. Additionally, most correlations are not statistically significant, as indicated by the lack of pronounced color changes. This suggests that the metabolites linked to age and sex may not be directly related to environmental pollution or diet. However, it is important to note that these results are based on partial data. A more comprehensive questionnaire and biomonitoring approach will be implemented in the future to collect detailed exposure data, allowing for a more thorough and comprehensive analysis.
The emerging organic contaminants (EOC) were identified in the human hair, and with significant differences observed based on age or sex. They included the plasticizers (monobutyl phthalate and diethyl phthalate), surfactants (dodecyl hydrogen sulfate and 2-dodecoxyethyl hydrogen sulfate), personal care products (diethylamino hydroxybenzoyl hexyl benzoate), and pesticide (deisopropylatrazine). However, the POPs were not discovered in the hair samples. The potential reason was that our sample preparation procedure might not be suitable for the POPs which were lower hydrophobic chemicals. Our sample preparation procedure involved using methanol and PBS to extract as many chemicals as possible from human hair. POPs such as PFAS, dioxin, and PCB were considered as more hydrophobic chemicals that are hard to solve in the mixture of methanol and PBS. For the analysis of POPs, optimizing a specific sample preparation procedure will be required.
Age- and sex-dependent differential metabolites and perturbed metabolic pathways
To investigate the effects of age- and sex-dependent metabolites in metabolic pathways, pathway enrichment analysis was conducted on 205 compounds using the online tool MetaboAnalyst 5.0[38] [Figure 5]. This analysis identified 41 perturbed metabolic pathways, detailed in Table 2. These pathways are categorized into eight types: lipid metabolism, amino acid metabolism, carbohydrate metabolism, cofactor and vitamin metabolism, hormone-associated pathways, nucleotide metabolism, biosynthesis of other secondary metabolites, and other metabolic processes [Table 2]. Of the 41 pathways, 13 are age-dependent, 11 are sex-dependent, and 17 are influenced by both age and sex. To determine if the age- and/or sex-related metabolic pathways identified from hair metabolism align with previous findings, a PubMed search was conducted. Out of the 41 pathways, 30 have been reported in prior studies as being age- and/or sex-related, while 11 have not. Fourteen of these 30 pathways align with the age and/or sex associations observed in previous studies, including arachidonic acid metabolism and fatty acid biosynthesis [Table 2].
Figure 5. Pathway enrichment analysis for 41 metabolic pathways affected by age and sex. The enriched metabolite sets of (A) age-dependent metabolites, (B) age-dependent lipids, (C) sex-dependent metabolites, and (D) sex-dependent lipids are presented. The enriched metabolite sets in metabolites and lipids are analyzed using the online software MetaboAnalyst 5.0. The x-axis displayed the
Age- and sex-dependent metabolic pathways
Pathway classification | Pathway name | Detected METABOLITES IN THIS STUDY | Related to AGE OR SEX IN THIS STUDY | Ref. |
Lipid metabolism | Arachidonic acid metabolism | L-glutamic acid, arachidonic acid, leukotriene a4, 8,9-epoxyeicosatrienoic acid, 15-deoxy-d-12,14-PGJ2 | Age, sex | [40,41] |
Fatty acid biosynthesis | Biotin, palmitic acid, 3-hydroxybutyric acid, dodecanoic acid, trans-Dodec-2-enoic acid, trans-Tetra-dec-2-enoic acid | Age, sex | [42,43] | |
Mitochondrial beta-oxidation of medium chain saturated fatty acids | Dodecanoic acid | Age, sex | - | |
Beta oxidation of very long chain fatty acids | Dodecanoic acid | Age, sex | [44] | |
Sphingolipid metabolism | Sphinganine | Age | [45] | |
Alpha linolenic acid and linoleic acid metabolism | Linoleic acid, arachidonic acid, alpha-linolenic acid, stearidonic acid | Sex | [46,47] | |
Carbohydrate metabolism | Propanoate metabolism | Biotin, L-glutamic acid | Age, sex | [48] |
Citric acid cycle | Biotin | Sex | [49] | |
Gluconeogenesis | Biotin | Sex | [50] | |
Pyruvate metabolism | Biotin | Sex | [51] | |
Amino ACID METABOlism | Alanine metabolism | Biotin, L-glutamic acid | Age, sex | [50] |
Arginine and proline metabolism | L-glutamic acid, L-proline, ornithine | Age, sex | [52] | |
Tryptophan metabolism | L-glutamic acid, kynurenic acid, L-tryptophan, 2-aminobenzoic acid, 3-hydroxyanthranilic acid | Age, sex | [53,54] | |
Carnitine synthesis | N6,N6,N6-trimethyl-L-lysine | Age, sex | - | |
Tyrosine metabolism | L-glutamic acid | Age | [55] | |
Histidine metabolism | L-glutamic acid | Age | - | |
Lysine degradation | L-glutamic acid | Age | - | |
Methionine metabolism | Dimethylglycine, 2-oxo-4-methylthiobutanoic acid | Age | [56] | |
Aspartate metabolism | L-glutamic acid | Age | [57] | |
Cysteine metabolism | L-glutamic acid | Age | - | |
Malate-aspartate shuttle | L-glutamic acid | Age | [58] | |
Beta-alanine metabolism | L-glutamic acid | Age | [59] | |
Glutamate metabolism | Biotin, L-glutamic acid | Sex | [60] | |
Glutathione metabolism | L-glutamic acid, pyroglutamic acid | Sex | [61] | |
Valine, leucine and isoleucine degradation | Biotin, L-glutamic acid, L-isoleucine | Sex | - | |
Hormone ASSOCIATED METABOlism | Estrone metabolism | Estradiol | Age, sex | [62] |
Androgen and estrogen metabolism | Estradiol, testosterone glucuronide | Age, sex | [63] | |
Biosynthesis OF OTHER SECONDARY Metabolism | Caffeine metabolism | 5-acetylamino-6-formylamino-3-methyluracil | Age, sex | [64] |
Nucleotide metabolism | Purine metabolism | Adenine, L-glutamic acid, hypoxanthine | Age, sex | [62] |
Metabolism of COFACTORS AND VITamins | Retinol metabolism | All-trans-13,14-dihydroretinol | Age, sex | [65,66] |
Folate metabolism | L-glutamic acid | Age | [67] | |
Nicotinate and nicotinamide metabolism | L-glutamic acid, N1-methyl-2-pyridone-5-carboxamide | Age | [68] | |
Biotin metabolism | Biotin | Sex | [69] | |
Others | Ammonia recycling | Biotin, L-glutamic acid | Age, sex | - |
Phenylacetate metabolism | Alpha-N-phenylacetyl-L-glutamine | Age, sex | [70] | |
Urea cycle | L-glutamic acid, ornithine | Age, sex | [71,72] | |
Threonine and 2-oxobutanoate degradation | Biotin | Sex | - | |
Glucose-alanine cycle | L-glutamic acid | Age | - | |
Amino sugar metabolism | L-glutamic acid | Age | - | |
Transfer of acetyl groups into mitochondria | Biotin | Sex | - | |
Warburg effect | Biotin, L-glutamic acid | Sex | - |
However, 16 pathways exhibit differences from prior research. For example, propanoate metabolism, which involves the conversion of propionate to propanol adenylate by propionyl-CoA synthase and acetyl-CoA synthase, is linked to both age and sex based on hair metabolism[73]. Previous studies have identified this pathway as age-related but not sex-related [Table 2]. Biotin metabolism is associated with sex in this study, whereas it has been shown to be age-related in earlier research[69]. Phenylacetate metabolism is identified as age- and sex-related based on hair metabolism, yet no prior studies have documented this association.
Among the 11 metabolic pathways not reported to be age- and/or sex-related, one involves lipid metabolism, four pertain to amino acid metabolism, and six relate to other metabolic processes. For instance, mitochondrial beta-oxidation of medium-chain saturated fatty acids, categorized under lipid metabolism, is associated with both age and sex in this study, although no prior research has confirmed this. The four amino acid metabolisms - valine, leucine and isoleucine degradation, carnitine synthesis, lysine degradation, and cysteine metabolism - show distinct age or sex associations based on hair metabolism, yet no studies have established these connections. Additionally, other metabolisms like ammonia recycling, glucose-alanine cycle, and amino sugar metabolism show age-related trends, while threonine and
Limitations
It is significant to discuss some inherent limitations that may affect the interpretation of our findings in this study. First, some of the hair samples collected for this study had undergone cosmetic treatment, which might be a confounding factor for evaluating the altered metabolome across age and sex. For example, Eisenbeiss et al. reported that treatment of hair with 9% hydrogen peroxide for 30 min resulted in statistically significant changes in a total of 69 metabolites[74]. Significant alternations in amino acids, cysteic acid, and lipids in hair were observed during the cosmetic treatments, including perming, dyeing and bleaching[75]. Although the hair after cosmetic treatments should be ideally excluded from this study, doing so would make it challenging to recruit enough participants. Secondly, 205 chemical compounds included in 41 metabolic pathways were discovered to be associated with age or sex. This set of chemical compounds and metabolic pathways can serve as a baseline for future biomonitoring investigations. However, the precise measurements of the concentrations of these chemicals were not available in this study. Once the precise levels of these chemicals in human hair are determined, it will greatly enhance the use of hair for biomonitoring investigations, providing more valuable information and facilitating its application in environmental exposure assessments. Thirdly, underlying health conditions can significantly influence the metabolome and exposome, potentially confounding the results and interpretations of our study. The absence of this critical information might affect the accuracy and reliability of our findings. Including comprehensive health profiles of participants would help account for the impact of underlying diseases.
CONCLUSION
In this study, we investigate the impact of age and sex on metabolism using hair samples analyzed through UHPLC-HRMS. A total of 41 significant metabolic distinctions across different age and sex groups were identified, thereby contributing valuable insights into the biological impact of these factors on the human metabolome. These results indicated that age has a pronounced influence on metabolic profiles, especially among females, while sex-related metabolic differences are more prominent in younger cohorts. These findings underscore the complexity of metabolic interactions and the importance of considering both age and sex in biomonitoring and health research. A total of 205 distinct chemical compounds were identified, demonstrating the intricate nature of how these factors interplay to affect metabolic pathways. Importantly, our research highlights several key metabolic pathways affected by age and sex, including lipid metabolism and amino acid turnover. Noteworthy are the pathways such as arachidonic acid metabolism and fatty acid biosynthesis, which consistently exhibit dependencies on both age and sex, reflecting their critical roles in maintaining physiological homeostasis and response to environmental exposures. This study represents a significant step toward establishing a reliable hair metabolomic baseline, which can serve as a robust tool for environmental exposure assessment. This advantage is critical for studies requiring a longitudinal perspective on exposure and metabolic dynamics. As we advance our understanding of the hair metabolome’s complexity, we continue to advocate for the integration of metabolomic data with clinical and environmental data to better understand the full scope of exposure and metabolic response. The implications of our findings are manifold, providing a foundation for future investigations and applications in the field of biomonitoring and beyond.
DECLARATIONS
Acknowledgments
The authors gratefully acknowledge the mass spectrometry analysis supported by the National Taiwan University Consortia of Key Technologies and National Taiwan University Instrumentation Center, Taiwan, and ICP00401 and MS004000 equipment belonging to the Core Facility Center of National Cheng Kung University, Taiwan.
Authors’ contributions
Conducted the original draft writing and revision, the experiment, data curation and analysis: Chang CW
Responsible for revising the manuscript and recruiting subjects: Wu CH, Wang RH, Lo YT
Designed the study, oversaw its conceptualization and supervision, administered the project, and revised the manuscript: Liao PC
Availability of data and materials
Not applicable.
Financial support and sponsorship
This work was supported by the National Science and Technology Council, Taiwan (grant number MOST109-2113-M-006-015, MOST110-2113-M-006-014, MOST111-2113-M-006-011, and NSTC 112-2113-M-006-002).
Conflicts of interest
Liao PC is an Editorial Board member of Journal of Environmental Exposure Assessment, while other authors declared that there are no conflicts of interest.
Ethical approval and consent to participate
Throughout the collection phase, each participant signed a written informed consent form, complying with the protocols established by the Institutional Review Board of National Cheng Kung University Hospital (IRB approval numbers A-ER-107-373 and B-ER-108-188).
Consent for publication
Not applicable.
Copyright
© The Author(s) 2024.
Supplementary Materials
REFERENCES
2. Needham LL, Calafat AM, Barr DB. Uses and issues of biomonitoring. Int J Hyg Environ Health 2007;210:229-38.
3. Henríquez-Hernández LA, Ortiz-Andrelluchi A, Álvarez-Pérez J, et al. Human biomonitoring of persistent organic pollutants in elderly people from the Canary Islands (Spain): a temporal trend analysis from the PREDIMED and PREDIMED-Plus cohorts. Sci Total Environ 2021;758:143637.
4. Albertini R, Bird M, Doerrer N, et al. The use of biomonitoring data in exposure and human health risk assessments. Environ Health Perspect 2006;114:1755-62.
5. Zhou Q, Zhang J, Fu J, Shi J, Jiang G. Biomonitoring: an appealing tool for assessment of metal pollution in the aquatic ecosystem. Anal Chim Acta 2008;606:135-50.
6. Wang Y, Hsu J, Hsu Y, Liao P. Identification of potential urinary exposure markers for the toxicant diisononyl phthalate in rubber worker urine specimens by high-resolution mass spectrometry-based metabolomics. URINE 2019;1:8-16.
7. Klotz K, Weiß T, Zobel M, et al. Validity of different biomonitoring parameters in human urine for the assessment of occupational exposure to naphthalene. Arch Toxicol 2019;93:2185-95.
8. Crinnion WJ. The CDC fourth national report on human exposure to environmental chemicals: what it tells us about our toxic burden and how it assists environmental medicine physicians. Altern Med Rev 2010;15:101-9.
9. USCDC. Fourth national report on human exposure to environmental chemicals. Updated tables, March 2021 : volume three: analysis of pooled serum samples for select chemicals, NHANES 2005-2016. Available from: https://stacks.cdc.gov/view/cdc/105344. [Last accessed on 15 Aug 2024].
10. Hoppin JA, Brock JW, Davis BJ, Baird DD. Reproducibility of urinary phthalate metabolites in first morning urine samples. Environ Health Perspect 2002;110:515-8.
11. Slupsky CM, Rankin KN, Wagner J, et al. Investigations of the effects of gender, diurnal variation, and age in human urinary metabolomic profiles. Anal Chem 2007;79:6995-7004.
12. Saude EJ, Adamko D, Rowe BH, Marrie T, Sykes BD. Variation of metabolites in normal human urine. Metabolomics 2007;3:439-51.
13. Giskeødegård GF, Davies SK, Revell VL, Keun H, Skene DJ. Diurnal rhythms in the human urine metabolome during sleep and total sleep deprivation. Sci Rep 2015;5:14843.
14. Caplan YH, Goldberger BA. Alternative specimens for workplace drug testing. J Anal Toxicol 2001;25:396-9.
15. Jang WJ, Choi JY, Park B, et al. Hair metabolomics in animal studies and clinical settings. Molecules 2019;24:2195.
16. Alves A, Kucharska A, Erratico C, et al. Human biomonitoring of emerging pollutants through non-invasive matrices: state of the art and future potential. Anal Bioanal Chem 2014;406:4063-88.
17. Hardy EM, Dereumeaux C, Guldner L, et al. Hair versus urine for the biomonitoring of pesticide exposure: Results from a pilot cohort study on pregnant women. Environ Int 2021;152:106481.
18. Hsu JY, Ho HH, Liao PC. The potential use of diisononyl phthalate metabolites hair as biomarkers to assess long-term exposure demonstrated by a rat model. Chemosphere 2015;118:219-28.
19. Hsu JF, Chang WC, Ho WY, Liao PC. Exploration of long-term exposure markers for phthalate esters in human hair using liquid chromatography-tandem mass spectrometry. Anal Chim Acta 2022;1200:339610.
20. Shih CL, Wu HY, Liao PM, et al. Profiling and comparison of toxicant metabolites in hair and urine using a mass spectrometry-based metabolomic data processing method. Anal Chim Acta 2019;1052:84-95.
21. Delplancke TDJ, de Seymour JV, Tong C, et al. Analysis of sequential hair segments reflects changes in the metabolome across the trimesters of pregnancy. Sci Rep 2018;8:36.
23. Iglesias-González A, Schweitzer M, Palazzi P, et al. Investigating children’s chemical exposome - Description and possible determinants of exposure in the region of Luxembourg based on hair analysis. Environ Int 2022;165:107342.
24. Chang CW, Hsu JY, Su YH, Chen YC, Hsiao PZ, Liao PC. Monitoring long-term chemical exposome by characterizing the hair metabolome using a high-resolution mass spectrometry-based suspect screening approach. Chemosphere 2023;332:138864.
25. Ruiz-Castell M, Le Coroller G, Pexaras A, et al. Characterizing the adult exposome in men and women from the general population: Results from the EHES-LUX study. Environ Int 2023;173:107780.
26. Gilles L, Govarts E, Rodriguez Martin L, et al. Harmonization of human biomonitoring studies in Europe: characteristics of the HBM4EU-aligned studies participants. Int J Environ Res Public Health 2022;19:6787.
27. Polledri E, Mercadante R, Consonni D, Fustinoni S. Cumulative pesticides exposure of children and their parents living near vineyards by hair analysis. Int J Environ Res Public Health 2021;18:3723.
28. Bucher ML, Anderson FL, Lai Y, Dicent J, Miller GW, Zota AR. Exposomics as a tool to investigate differences in health and disease by sex and gender. Exposome 2023;3:osad003.
29. Ding E, Wang Y, Liu J, Tang S, Shi X. A review on the application of the exposome paradigm to unveil the environmental determinants of age-related diseases. Hum Genomics 2022;16:54.
30. Costanzo M, Caterino M, Sotgiu G, Ruoppolo M, Franconi F, Campesi I. Sex differences in the human metabolome. Biol Sex Differ 2022;13:30.
31. Muthubharathi BC, Gowripriya T, Balamurugan K. Metabolomics: small molecules that matter more. Mol Omics 2021;17:210-29.
32. Chang WC, Wang PH, Chang CW, Chen YC, Liao PC. Extraction strategies for tackling complete hair metabolome using LC-HRMS-based analysis. Talanta 2021;223:121708.
33. Tsugawa H, Cajka T, Kind T, et al. MS-DIAL: data-independent MS/MS deconvolution for comprehensive metabolome analysis. Nat Methods 2015;12:523-6.
34. Kind T, Tsugawa H, Cajka T, et al. Identification of small molecules using accurate mass MS/MS search. Mass Spectrom Rev 2018;37:513-32.
35. Tsugawa H, Kind T, Nakabayashi R, et al. Hydrogen rearrangement rules: computational MS/MS fragmentation and structure elucidation using MS-FINDER software. Anal Chem 2016;88:7946-58.
36. Schymanski EL, Jeon J, Gulde R, et al. Identifying small molecules via high resolution mass spectrometry: communicating confidence. Environ Sci Technol 2014;48:2097-8.
37. Djoumbou Feunang Y, Eisner R, Knox C, et al. ClassyFire: automated chemical classification with a comprehensive, computable taxonomy. J Cheminform 2016;8:61.
38. Pang Z, Chong J, Zhou G, et al. MetaboAnalyst 5.0: narrowing the gap between raw spectra and functional insights. Nucleic Acids Res 2021;49:W388-96.
39. Wishart DS, Guo A, Oler E, et al. HMDB 5.0: the human metabolome database for 2022. Nucleic Acids Res 2022;50:D622-31.
40. Thomas MH, Pelleieux S, Vitale N, Olivier JL. Dietary arachidonic acid as a risk factor for age-associated neurodegenerative diseases: potential mechanisms. Biochimie 2016;130:168-77.
41. Forner F, Kumar C, Luber CA, Fromme T, Klingenspor M, Mann M. Proteome differences between brown and white fat mitochondria reveal specialized metabolic functions. Cell Metab 2009;10:324-35.
42. Corona-Meraz FI, Vázquez-Del Mercado M, Ortega FJ, Ruiz-Quezada SL, Guzmán-Ornelas MO, Navarro-Hernández RE. Ageing influences the relationship of circulating miR-33a and miR- 33b levels with insulin resistance and adiposity. Diab Vasc Dis Res 2019;16:244-53.
43. Sibbons CM, Brenna JT, Lawrence P, et al. Effect of sex hormones on n-3 polyunsaturated fatty acid biosynthesis in HepG2 cells and in human primary hepatocytes. Prostaglandins Leukot Essent Fatty Acids 2014;90:47-54.
44. Yang L, Zhang Y, Wang S, Zhang W, Shi R. Decreased liver peroxisomal β-oxidation accompanied by changes in brain fatty acid composition in aged rats. Neurol Sci 2014;35:289-93.
45. Muilwijk M, Callender N, Goorden S, Vaz FM, van Valkengoed IGM. Sex differences in the association of sphingolipids with age in Dutch and South-Asian Surinamese living in Amsterdam, the Netherlands. Biol Sex Differ 2021;12:13.
46. Hrelia S, Bordoni A, Celadon M, Turchetto E, Biagi PL, Rossi CA. Age-related changes in linoleate and alpha-linolenate desaturation by rat liver microsomes. Biochem Biophys Res Commun 1989;163:348-55.
47. Burdge GC, Calder PC. Dietary alpha-linolenic acid and health-related outcomes: a metabolic perspective. Nutr Res Rev 2006;19:26-52.
48. Vignoli A, Tenori L, Luchinat C, Saccenti E. Age and sex effects on plasma metabolite association networks in healthy subjects. J Proteome Res 2018;17:97-107.
49. Yarian CS, Toroser D, Sohal RS. Aconitase is the main functional target of aging in the citric acid cycle of kidney mitochondria from mice. Mech Ageing Dev 2006;127:79-84.
50. Snell K, Walker DG. Age-related changes in gluconeogenesis and the metabolism of alanine in the perfused liver of neonatal rats. Biochem Soc Trans 1975;3:128-9.
51. Agostini A, Yuchun D, Li B, Kendall DA, Pardon MC. Sex-specific hippocampal metabolic signatures at the onset of systemic inflammation with lipopolysaccharide in the APPswe/PS1dE9 mouse model of Alzheimer's disease. Brain Behav Immun 2020;83:87-111.
52. Xie K, Qin Q, Long Z, et al. High-throughput metabolomics for discovering potential biomarkers and identifying metabolic mechanisms in aging and Alzheimer’s disease. Front Cell Dev Biol 2021;9:602887.
53. van der Goot AT, Nollen EA. Tryptophan metabolism: entering the field of aging and age-related pathologies. Trends Mol Med 2013;19:336-44.
54. Songtachalert T, Roomruangwong C, Carvalho AF, Bourin M, Maes M. Anxiety disorders: sex differences in serotonin and tryptophan metabolism. Curr Top Med Chem 2018;18:1704-15.
55. Durani LW, Hamezah HS, Ibrahim NF, et al. Age-related changes in the metabolic profiles of rat hippocampus, medial prefrontal cortex and striatum. Biochem Biophys Res Commun 2017;493:1356-63.
56. Stadtman ER, Van Remmen H, Richardson A, Wehr NB, Levine RL. Methionine oxidation and aging. Biochim Biophys Acta 2005;1703:135-40.
57. Luo HH, Feng XF, Yang XL, Hou RQ, Fang ZZ. Interactive effects of asparagine and aspartate homeostasis with sex and age for the risk of type 2 diabetes risk. Biol Sex Differ 2020;11:58.
58. Goyary D, Sharma R. Late onset of dietary restriction reverses age-related decline of malate-aspartate shuttle enzymes in the liver and kidney of mice. Biogerontology 2008;9:11-8.
59. Saransaari P, Oja SS. Characterization of sodium-independent beta-alanine binding to cerebral cortical membranes from 7-day-old and adult mice. Int J Dev Neurosci 1994;12:491-7.
60. Taskiran D, Sagduyu A, Yüceyar N, Kutay FZ, Pögün S. Increased cerebrospinal fluid and serum nitrite and nitrate levels in amyotrophic lateral sclerosis. Int J Neurosci 2000;101:65-72.
61. Zhu H, Wang N, Yao L, et al. Moderate UV exposure enhances learning and memory by promoting a novel glutamate biosynthetic pathway in the brain. Cell 2018;173:1716-27.e17.
62. Wehner G, Schweikert HU. Estrone sulfate source of estrone and estradiol formation in isolated human hair roots: identification of a pathway linked to hair growth phase and subject to site-, gender-, and age-related modulations. J Clin Endocrinol Metab 2014;99:1393-9.
63. Stanhewicz AE, Wenner MM, Stachenfeld NS. Sex differences in endothelial function important to vascular health and overall cardiovascular disease risk across the lifespan. Am J Physiol Heart Circ Physiol 2018;315:H1569-88.
64. Murray A, Tharmalingam S, Nguyen P, Tai TC. Untargeted metabolomics reveals sex-specific differences in lipid metabolism of adult rats exposed to dexamethasone in utero. Sci Rep 2021;11:20342.
65. Zhang PP, Ma KX, Ke XF, et al. Development and validation of a five-RNA-based signature and identification of candidate drugs for neuroblastoma. Front Genet 2021;12:685646.
66. Li H, Rong Z, Wang H, et al. Proteomic analysis revealed common, unique and systemic signatures in gender-dependent hepatocarcinogenesis. Biol Sex Differ 2020;11:46.
67. Auley MT, Mooney KM, Salcedo-Sora JE. Computational modelling folate metabolism and DNA methylation: implications for understanding health and ageing. Brief Bioinform 2018;19:303-17.
68. Liu X, Zhang M, Liu X, et al. Investigation of plasma metabolic and lipidomic characteristics of a chinese cohort and a pilot study of renal cell carcinoma biomarker. Front Oncol 2020;10:1507.
69. Fukuwatari T, Wada H, Shibata K. Age-related alterations of B-group vitamin contents in urine, blood and liver from rats. J Nutr Sci Vitaminol 2008;54:357-62.
70. Caterino M, Ruoppolo M, Villani GRD, et al. Influence of sex on urinary organic acids: a cross-sectional study in children. Int J Mol Sci 2020;21:582.
71. Plubell DL, Wilmarth PA, Zhao Y, et al. Extended multiplexing of tandem mass tags (TMT) labeling reveals age and high fat diet specific proteome changes in mouse epididymal adipose tissue. Mol Cell Proteomics 2017;16:873-90.
72. Leskanicova A, Chovancova O, Babincak M, et al. Sexual dimorphism in energy metabolism of wistar rats using data analysis. Molecules 2020;25:2353.
73. Pronk JT, van der Linden-Beuman A, Verduyn C, Scheffers WA, van Dijken JP. Propionate metabolism in Saccharomyces cerevisiae: implications for the metabolon hypothesis. Microbiology 1994;140:717-22.
74. Eisenbeiss L, Binz TM, Baumgartner MR, Kraemer T, Steuer AE. Cheating on forensic hair testing? Detection of potential biomarkers for cosmetically altered hair samples using untargeted hair metabolomics. Analyst 2020;145:6586-99.
Cite This Article
How to Cite
Chang, C. W.; Wu C. H.; Wang R. H.; Lo Y. T.; Liao P. C. Establishing a biomonitoring baseline by characterizing the hair metabolome across age and sex using high-resolution mass spectrometry. J. Environ. Expo. Assess. 2024, 3, 19. http://dx.doi.org/10.20517/jeea.2024.17
Download Citation
Export Citation File:
Type of Import
Tips on Downloading Citation
Citation Manager File Format
Type of Import
Direct Import: When the Direct Import option is selected (the default state), a dialogue box will give you the option to Save or Open the downloaded citation data. Choosing Open will either launch your citation manager or give you a choice of applications with which to use the metadata. The Save option saves the file locally for later use.
Indirect Import: When the Indirect Import option is selected, the metadata is displayed and may be copied and pasted as needed.
About This Article
Special Issue
Copyright
Data & Comments
Data
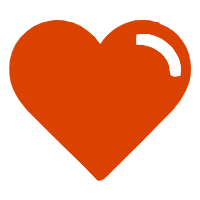
Comments
Comments must be written in English. Spam, offensive content, impersonation, and private information will not be permitted. If any comment is reported and identified as inappropriate content by OAE staff, the comment will be removed without notice. If you have any queries or need any help, please contact us at support@oaepublish.com.