AI-driven sustainability assessment for greener lithium-ion batteries
Abstract
Lithium-ion batteries (LIBs) are pivotal for electric vehicles and energy storage, yet their sustainability assessment is hindered by methodological limitations. Artificial intelligence (AI) is poised to transform lifecycle assessment (LCA) paradigms for LIBs. This study employs strengths, weaknesses, opportunities and threats analysis to comprehensively examine the role and prospects of AI for LCA in LIBs. The objective is to capitalize on the technology's strengths, mitigate its weaknesses, and identify potential opportunities and threats. Furthermore, this research proposes future studies to enhance the application of AI in the LCA of LIBs, including the establishment of unified standards for data collection, processing, and sharing, improving data transparency, promoting interdisciplinary collaboration, and developing more robust AI models. This study will provide a scientific reference for the research on efficient, scalable, and automated sustainability assessment methods in LIBs, driving the battery and transportation toward more sustainable development.
Keywords
INTRODUCTION
Global warming brings a series of severe issues such as glacial melting, frequent extreme weather events, and changes in precipitation distribution. Mitigating greenhouse gas emissions has emerged as a universal agreement among nations[1]. The transportation field contributes roughly a quarter of all energy-linked greenhouse gas emissions, of which three-quarters stem from vehicular traffic on roads[2]. With continuous breakthroughs and innovations in lithium-ion batteries (LIBs) technology, countries worldwide are vigorously advancing the shift from conventional internal combustion engine vehicles to electric vehicles (EVs), aiming for carbon neutrality in the transportation sector[3]. It is projected that EVs will grow from 30 million in 2022 to 380 million in 2030[4]. With the explosive growth of EVs, the demand for power batteries is also rapidly increasing. Global demand for LIBs in EVs is expected to reach 1,525 GWh by 2030[5]. The expanding production and use of LIBs have also given rise to a series of complex environmental and social issues, necessitating a comprehensive sustainability assessment to determine their impact on the ecological environment and human health.
Life cycle assessment (LCA) is a method for evaluating the environmental impact of a product from raw material extraction, production, and use to disposal. The LCA of different types of batteries reveals that LIBs have significant environmental advantages compared to nickel metal hydride batteries and solar cells[6]. Conducting LCA on LIBs helps to uncover environmental issues throughout their entire life cycle, providing a basis for the optimized design and sustainable development of LIBs. Environmental impacts of battery production vary with different chemical systems, with an average cumulative energy demand and greenhouse gas emissions of 328 kWh and 110 kg CO2eq per kWh of storage capacity, respectively[7]. Carbon footprint and other environmental impacts of the use and recycling phases of LIBs also vary depending on the calculation methods and process routes[8]. The use of multiple evaluation methods contributes to enhancing the comprehensiveness and objectivity of product system assessment results[9]. However, traditional LCA methods have limitations in the depth and breadth of data processing and analysis throughout the entire life cycle of LIBs, lacking dynamic analysis, which makes it difficult to adapt to the rapid development of the LIBs industry and the high demands of environmental protection and low carbon emission.
In this context, the innovative development of artificial intelligence (AI) presents revolutionary opportunities for the LCA of LIBs[10,11]. AI technology has significant advantages in data processing, model construction, and result analysis, and has been widely applied in environmental science and engineering fields, such as pollution detection and the development and protection of marine resources[12,13]. It is expected to address the challenges of data scarcity, complexity, and inefficiency in traditional LCA methods for LIBs assessment. AI can empower the LCA (goal and scope definition, life cycle inventory, life cycle impact analysis, and life cycle interpretation) of the entire life cycle of LIBs. It plays a key role across various stages of the battery life cycle, from lifetime prediction to end-of-life recycling and safety management[14]. For example, AI can facilitate the recognition of the type and state of the components to be disassembled, ensuring that the disassembly operations are conducted safely and efficiently[15]. Furthermore, the recovery rate of metal elements in spent batteries can be improved by optimizing the recycling process through AI[16], thereby reducing carbon emissions and other environmental impacts during the recycling process. The application of AI in battery recycling has emerged as a promising avenue to reduce costs and improve the environmental sustainability of battery waste management, with significant market potential[17]. The integration of AI with LCA in the environmental impact assessment of LIBs can be beneficial but may also pose issues. In this study, the strengths, weaknesses, opportunities and threats (SWOT) analysis method is employed to delve into the application of AI for LCA in LIBs, conducting a forward-looking analysis of the strengths and weaknesses of the technology. The aim is to more actively leverage strengths and avoid weaknesses, while also identifying potential opportunities and threats, in order to formulate more scientific development strategies and flexible response measures.
METHODOLOGY
The SWOT analysis is a widely applied and crucial tool in strategic planning that aids businesses and organizations in gaining profound insights into the current status of the research subject, thereby planning future strategies to confront challenges[18]. Through the SWOT analysis, we can systematically assess the project’s strengths (aspects that can be further utilized and reinforced), weaknesses (areas that require attention and improvement), opportunities (potential development points that can be seized by leveraging existing strengths), and threats (external factors that may impact the project’s progress)[19]. Strengths and weaknesses are predominantly influenced by internal factors, while opportunities and threats are more significantly shaped by the external environment. As shown in Figure 1, in AI-driven sustainability assessment of LIBs, the SWOT analysis offers a clear and structured framework that enables us to comprehensively identify and analyze the internal strengths and weaknesses, as well as external opportunities and threats.
RESULTS AND DISCUSSION
Strengths
The application of AI for LCA in LIBs demonstrates significant advantages. Firstly, large amounts of data covering the entire life cycle of batteries can be processed and analyzed by AI, thereby uncovering deep-seated relationships within the data and providing more profound insights into environmental impact assessments[20]. This aids decision-makers in forming accurate and effective solutions. Secondly, the performance and service life of LIBs can be predicted and optimized using the predictive analytics capability of AI. For example, the relative error in the predicted lifetime of LIBs using semi-supervised self-learning methods is less than 4.1%[21]. The carbon emissions and other environmental impacts during the use phase can be reduced by optimizing battery system energy management strategies through AI[22]. At the same time, the missing values in life cycle inventories or impacts can also be filled in via AI predictions[23], ensuring the effective progression and accuracy of the battery life cycle evaluation process. Additionally, AI can collect data from various sources during the production, use, and recycling of LIBs through text mining, enhancing the computational speed and resource efficiency of complex battery assessment models. This allows for real-time and efficient calculations, reducing the time and labor required for the LCA in LIBs. For instance, automation data management and multimodal analysis from different sources can be achieved by integrating large language models (LLMs) into battery life cycle inventory (LCI) modeling. This not only improves the scalability and generalizability of battery LCI but also significantly increases the efficiency and speed of assessments.
Weaknesses
Although AI holds great potential in LCA for LIBs, there are some undeniable drawbacks. Firstly, the performance of AI models is heavily dependent on the quality and availability of data[24]. Training models often requires a large volume of high-quality data covering the entire life cycle of LIBs, which can be difficult or costly to obtain, especially for battery technologies with proprietary secrets. How to accurately conduct environmental impact assessments with a limited amount of battery life cycle data is a significant challenge. Secondly, AI models may suffer from issues of interpretability[25]. The “black box” nature of AI models has long been a challenge to understanding their decision-making processes, which will reduce the credibility and traceability of AI in the comprehensive sustainable assessment of batteries. Additionally, there is a risk of overfitting historical data with AI models, which can decrease the accuracy of predictions for future scenarios and hinder the effective formulation of policies. The specific application of AI models in the field of sustainability assessment for LIBs is still in the exploratory stage, lacking unified industry standards for guidance, and the results are difficult to quantify and compare.
Threats
The threats associated with the application of AI in the sustainable assessment of LIBs involve data security and privacy risks, algorithmic bias, and ethical responsibilities[26]. Firstly, AI-based processing of LCA requires a large amount of data covering stages such as the production, use, and recycling of LIBs, which may involve corporate trade secrets and personal information of users. A data breach could severely harm the interests of businesses and the privacy of consumers. Secondly, if the data used to train AI models for the entire life cycle of LIBs is incomplete or biased, it could lead to misleading results, affecting the accuracy and reliability of the sustainability assessment for LIBs. Additionally, AI models may not be capable of making decisions that align with human values when dealing with issues involving ethics and environmental responsibility. Unclear responsibility for resource allocation and environmental impact assessment could potentially lead to legal and moral controversies.
Opportunities
The innovative development of AI offers new opportunities for the sustainability assessment of LIBs. Emerging AI technologies and algorithms, particularly the advancement of complex algorithms such as natural language processing and deep learning, enable the automatic extraction and analysis of text data from research articles, patents, and industry reports[26]. This provides more powerful methods and analytical tools for a more accurate and efficient comprehensive sustainable assessment of LIBs. Concurrently, AI can facilitate the rapid updating and iteration of LCA databases, meeting the evaluation needs of different technologies across various periods[23]. Policies and market mechanisms formulated by governments and enterprises incentivize the application of AI for LCA in LIBs. For instance, China has included the "Artificial Intelligence +" initiative in its 2024 government report. Moreover, the application of AI in the LCA of LIBs fosters interdisciplinary collaboration, aiding in the integration of expertise from diverse fields and driving innovation in LCA methodologies. This collaboration is instrumental in more effectively addressing complex issues during the assessment process. At the same time, the application of AI in the LCA of LIBs presents an opportunity to establish global unified evaluation standards, thereby reducing barriers to transnational trade and technological cooperation.
CONCLUSIONS AND RECOMMENDATIONS
Integrating AI into the sustainability assessment of LIBs holds tremendous potential to revolutionize the way of assessing and managing the environmental impact of batteries. Although there are challenges and threats to be overcome, the advantages and opportunities brought about by AI-driven LCA for LIBs are substantial. Future research should focus on establishing unified standards for data collection, processing, and sharing across the entire life cycle of LIBs, enhancing data quality, and promoting interdisciplinary intersections among environmental science, data science, and battery engineering. The development of transparent, adaptable, and robust AI models is essential to ensure that AI-driven LCA promotes the sustainable development of LIBs.
DECLARATIONS
Authors’ contributions
Methodology, investigation, formal analysis, writing - original draft: Chen, Q.
Conceptualization, methodology, writing - review & editing, supervision, funding acquisition: Lai, X.
Investigation: Zhang, Y.
Methodology, investigation: Chen, J.
Conceptualization, funding acquisition: Zheng, Y.
Methodology, funding acquisition: Song, X.
Conceptualization: Han, X.; Ouyang, M.
Availability of data and materials
Not applicable.
Financial support and sponsorship
This research was supported by the National Natural Science Foundation of China (NSFC) (52277223, 52277222, and 42471331), Scientific Research Foundation of Shanghai Polytechnic University (EGD25QD03), Shanghai Pujiang Programme (23PJD062), and Natural Science Foundation of Shanghai (22ZR1444500).
Conflicts of interest
Zheng, Y. and Lai, X. are Guest Editors of the journal Carbon Footprints. Zheng, Y. and Lai, X. were not involved in any steps of editorial processing, notably including reviewer selection, manuscript handling, and decision making, while the other authors have declared that they have no conflicts of interest.
Ethical approval and consent to participate
Not applicable.
Consent for publication
Not applicable.
Copyright
© The Author(s) 2025.
REFERENCES
1. Dong, Q.; Zhong, C.; Geng, Y.; Dong, F.; Chen, W.; Zhang, Y. A bibliometric review of carbon footprint research. Carbon. Footprints. 2024, 3, 3.
2. Zhang, C.; Zhao, X.; Sacchi, R.; You, F. Trade-off between critical metal requirement and transportation decarbonization in automotive electrification. Nat. Commun. 2023, 14, 1616.
3. Dou, H.; Hao, H. The greenhouse gas emissions reduction co-benefit of end-of-life electric vehicle battery treatment strategies. Carbon. Footprints. 2024, 3, 2.
4. Istrate, R.; Mas-Fons, A.; Beylot, A.; et al. Decarbonizing lithium-ion battery primary raw materials supply chain. Joule 2024, 8, 2992-3016.
5. Chen, Q.; Lai, X.; Chen, J.; et al. A critical comparison of LCA calculation models for the power lithium-ion battery in electric vehicles during use-phase. Energy 2024, 296, 131175.
6. Liang, Y.; Su, J.; Xi, B.; et al. Life cycle assessment of lithium-ion batteries for greenhouse gas emissions. Resour. Conserv. Recy. 2017, 117, 285-93.
7. Peters, J. F.; Baumann, M.; Zimmermann, B.; Braun, J.; Weil, M. The environmental impact of Li-ion batteries and the role of key parameters - A review. Renew. Sustain. Energy. Rev. 2017, 67, 491-506.
8. Yu, W.; Zhou, J.; Hu, J.; Shang, Z.; Zhou, X.; Xu, S. Exploring the energy and environmental sustainability of advanced lithium-ion battery technologies. Resour. Conserv. Recy. 2025, 212, 107963.
9. Stavropoulos, P.; Giannoulis, C.; Papacharalampopoulos, A.; Foteinopoulos, P.; Chryssolouris, G. Life cycle analysis: comparison between different methods and optimization challenges. Procedia. CIRP. 2016, 41, 626-31.
10. Xu, Y.; Wang, F.; Zhang, T. Artificial intelligence is restructuring a new world. Innovation 2024, 5, 100725.
11. Buster, G.; Benton, B. N.; Glaws, A.; King, R. N. High-resolution meteorology with climate change impacts from global climate model data using generative machine learning. Nat. Energy. 2024, 9, 894-906.
12. Wu, Y.; Xu, M.; Liu, S. Generative artificial intelligence: a new engine for advancing environmental science and engineering. Environ. Sci. Technol. 2024, 58, 17524-8.
13. Wang, P.; Zhang, L. Y.; Tzachor, A.; Chen, W. Q. E-waste challenges of generative artificial intelligence. Nat. Comput. Sci. 2024, 4, 818-23.
14. Li, S.; Gao, H. O.; You, F. AI for science in electrochemical energy storage: A multiscale systems perspective on transportation electrification. Nexus 2024, 1, 100026.
15. Meng, K.; Xu, G.; Peng, X.; Youcef-toumi, K.; Li, J. Intelligent disassembly of electric-vehicle batteries: a forward-looking overview. Resour. Conserv. Recy. 2022, 182, 106207.
16. Pregowska, A.; Osial, M.; Urbańska, W. The application of artificial intelligence in the effective battery life cycle in the closed circular economy model - a perspective. Recycling 2022, 7, 81.
17. Ren, T.; Wu, X.; Wang, D.; et al. Sustainable recovery progress of ternary cathodes in lithium-ion batteries in the artificial intelligence era. Mater. Today. Energy. 2025, 49, 101844.
18. Dhumal, P.; Chakraborty, S.; Ibrahim, B.; Kaur, M.; Valsami-jones, E. Green-synthesised carbon nanodots: A SWOT analysis for their safe and sustainable innovation. J. Clean. Prod. 2024, 480, 144115.
19. Olabi, A.; Wilberforce, T.; Sayed, E. T.; et al. Battery energy storage systems and SWOT (strengths, weakness, opportunities, and threats) analysis of batteries in power transmission. Energy 2022, 254, 123987.
20. Romeiko, X. X.; Zhang, X.; Pang, Y.; et al. A review of machine learning applications in life cycle assessment studies. Sci. Total. Environ. 2024, 912, 168969.
21. Che, Y.; Stroe, D.; Hu, X.; Teodorescu, R. Semi-supervised self-learning-based lifetime prediction for batteries. IEEE. Trans. Ind. Inf. 2023, 19, 6471-81.
22. Jondhle, H.; Nandgaonkar, A. B.; Nalbalwar, S.; Jondhle, S. An artificial intelligence and improved optimization-based energy management system of battery-fuel cell-ultracapacitor in hybrid electric vehicles. J. Energy. Storage. 2023, 74, 109079.
23. Tu, Q.; Guo, J.; Li, N.; Qi, J.; Xu, M. Mitigating grand challenges in life cycle inventory modeling through the applications of large language models. Environ. Sci. Technol. 2024, 58, 19595-603.
24. Xu, Y.; Lin, T.; Du, P.; Wang, J. The research on a novel multivariate grey model and its application in carbon dioxide emissions prediction. Environ. Sci. Pollut. Res. Int. 2024, 31, 21986-2011.
25. Zhang, Y.; Teoh, B. K.; Wu, M.; Chen, J.; Zhang, L. Data-driven estimation of building energy consumption and GHG emissions using explainable artificial intelligence. Energy 2023, 262, 125468.
Cite This Article

How to Cite
Download Citation
Export Citation File:
Type of Import
Tips on Downloading Citation
Citation Manager File Format
Type of Import
Direct Import: When the Direct Import option is selected (the default state), a dialogue box will give you the option to Save or Open the downloaded citation data. Choosing Open will either launch your citation manager or give you a choice of applications with which to use the metadata. The Save option saves the file locally for later use.
Indirect Import: When the Indirect Import option is selected, the metadata is displayed and may be copied and pasted as needed.
About This Article
Special Issue
Copyright
Data & Comments
Data
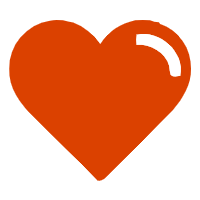
Comments
Comments must be written in English. Spam, offensive content, impersonation, and private information will not be permitted. If any comment is reported and identified as inappropriate content by OAE staff, the comment will be removed without notice. If you have any queries or need any help, please contact us at [email protected].