Challenges and strategies in estimating soil organic carbon for multi-cropping systems: a review
Abstract
Multi-cropping systems play a crucial role in global agricultural production. Accurately estimating the soil carbon sequestration capacity of multi-cropping systems is of significant importance for enhancing agricultural productivity, mitigating greenhouse gas emissions, and reducing carbon footprint. However, soil carbon cycling is more complex in multi-cropping systems compared with single-cropping systems, and existing assessment methods cannot accurately estimate soil carbon sequestration in multi-cropping systems with high operability. Here, we reviewed the accuracy and efficiency of the three primary global soil carbon assessment methods, including statistical models, process-based models, and the Intergovernmental Panel on Climate Change (IPCC) steady-state method. Our study concludes that it is difficult to simulate the dynamic evolution of soil organic carbon (SOC) using the statistical models, while the well simulation through process-based models demands a large amount of data. Additionally, the IPCC Tier 2 method cannot be directly applied to estimate SOC in multi-cropping systems due to mismatches in parameters and time steps. We suggest modifying the structures and parameters of the IPCC Tier 2 method by revising the inventory unit and redetermining the parameter values, which should efficiently address its bottleneck in estimating SOC for multi-cropping systems. Moreover, long-term experimental observations and multi-model ensemble simulations are beneficial for determining the parameter values to address the data deficiencies in IPCC Tier 2. This study aims to explore pathways for improving the accuracy of SOC estimation in multi-cropping systems and, thus, carbon footprint calculation worldwide.
Keywords
INTRODUCTION
Soil is the largest natural carbon sink, and ranks as the second-largest carbon emission source following fossil fuel combustion. Enhancing soil carbon sequestration is crucial for mitigating global warming[1,2]. The initiative in the Paris Agreement indicated that an annual increase of 0.4% in global soil carbon storage can offset anthropogenic greenhouse gas emissions, which highlighted the vital role of soil carbon sequestration in mitigating global warming[3]. In general, cropland covers 11.2% of the global land area, serving as a significant carbon sink, while its role has been undermined because of original cultivation and tillage activities. Optimizing management practices plays a substantial role in enhancing the carbon sequestration potential of cropland[4,5]. Global cropland could sequester up to 1.85 Pg C annually, which accounts for 53% of the carbon sequestration needed for the “4 per 1,000 program”[6]. However, global cropland exhibits distinct differences in climate, crops, soil and management practices. Accurately assessing soil organic carbon (SOC) stocks and their trends in cropland remains a focal point of international attention and has not been fully addressed[7,8]. Changes in soil carbon storage are crucial in carbon footprint assessments, and accurate estimation of SOC is essential for refining agricultural carbon footprint calculations[9].
Variations of SOC in cropland represent a dynamic equilibrium between carbon input and carbon output, with its underlying mechanisms being complex[10,11]. Generally, primary productivity is a core factor limiting the maximum carbon input into soil. Agricultural practices, including straw management, green manure incorporation, grazing intensity, and organic fertilizer application, determine the actual amount of carbon entering the soil[12-14]. After crop residues and organic amendments enter the soil, the transformation and sequestration processes of soil carbon are motivated by the collaborative effects of climate and soil biology[15]. Previous studies showed that soil tillage, fertilization, and irrigation significantly influence SOC turnover and mineralization rates[16-18], as well as the interaction between C cycling and other nutrients, such as nitrogen and phosphorus[19,20]. Generally, global cropland includes single-cropping and multi-cropping based on the number of crop types planted in the same field within one year, and multi-cropping represents the planting method that two or more crops are grown in the same field within a year[21]. Single-cropping systems are mainly distributed in the mid-to-high latitudes of the Northern Hemisphere and the high latitudes of the Southern Hemisphere, accounting for about 88% of global cropland. In contrast, multi-cropping systems are widely distributed in tropical and subtropical humid climates, including regions in central and southern China, Southeast Asia, Central America, and Africa, covering another 12% of global cropland[22]. Among them, paddy-upland rotation is a widely adopted agricultural planting pattern in Asia, covering an area of 23.5 million hectares, accounting for 14.4% of Asia’s multi-cropping area, and making a significant contribution to global food security[23]. Previous studies have thoroughly explained the drivers of SOC sequestration in single-cropping systems, and identified practical methods for estimating SOC sequestration with ease of operation[24,25]. Compared with single-cropping, multi-cropping alters soil microorganism dynamics, increases the diversity of organic inputs and accelerates carbon cycling, introducing significant uncertainties in tracing SOC turnover[26,27]. Previous studies emphasized the need for detailed consideration of management intensity and the differences in varying residue properties when modeling SOC in multi-cropping systems, while the overall lack of parameter values and low operability hampers the further development of current SOC estimation method toward integrating multi-cropping[28,29].
Given the substantial differences in global agriculture among different regions, and the lack of in-depth understanding and precise assessment of SOC sequestration in multi-cropping systems, it is crucial to establish a scientific and universally standardized method for assessing SOC in multi-cropping systems. Here, we review the fundamental principles of estimating SOC sequestration in cropland, summarize the challenges for the major methods in estimating SOC in cropland, and explore the strategy of raising an accurate SOC estimation method by improving the Intergovernmental Panel on Climate Change (IPCC) Tier 2 method. This study aims to provide a scalable method for estimating SOC sequestration in multi-cropping systems, which should be beneficial for improving the carbon footprint calculation in agricultural production systems.
CHALLENGES IN ESTIMATING SOC SEQUESTRATION IN MULTI-CROPPING SYSTEMS
Generally, SOC sequestration is mainly regulated by the processes of decomposition, mineralization and stabilization, representing a current international research hotspot[30,31]. Previous studies indicated that the mechanisms of SOC turnover and sequestration in cropland mainly fall into the following three categories, namely chemical stabilization mechanism, physical protection mechanism, and microbiological mechanism. Generally, the chemical stabilization mechanism hypothesized that simple compounds produced during the decomposition of plant residues form humus during the microbial aggregation process. Humus constitutes 60%-80% of soil organic matter, possessing a complex chemical structure and high stability, making it resistant to microbial decomposition[32]. The physical protection mechanism hypothesized that root exudates or decomposition products of organic residues, acting as mucilage, interact with soil clay particles to form aggregates. These aggregates physically isolate soil organic matter from decomposers[33]. Organic matter can also enter the micro-pores of clay minerals for isolation[34]. The microbiological mechanism considers that microbes are the first responders to environmental changes and play a major regulatory role in the dynamics of soil organic matter[35]. Moreover, microbial residues contribute greatly to the long-term accumulation of SOC, and the growth dynamics and activity of microbes in the SOC sequestration process should be well captured[36,37]. SOC sequestration has been relatively well-studied and understood in single-cropping systems, while SOC sequestration mechanisms in most multi-cropping systems remain unclear[31,38,39].
Previous studies demonstrated that the diversified crop types and frequent agricultural practices (i.e., tillage, fertilizer management and irrigation) resulted in the complex SOC sequestration process in multi-cropping systems[40,41]. Different crop sequences in rotations significantly influence the structure and dynamics of the soil microbial community through changes in root exudates. Additionally, the lignin content, C:N ratio, and the decomposition rates of crop residues vary among different crops[42-44]. This indicates that multi-cropping systems cannot be formulated as a singular input-output balance process, but as interactive and synergistic progression of two or even multiple systems. For instance, the residues that return to the soil undergo rapid decomposition and enter a slow decomposition stage. The addition of fresh residues peaks microbial activity, and promotes the decomposition of pre-existing organic matter[45,46]. Frequent incorporation of fresh residues enhances the priming effect in multi-cropping systems, and significantly increases the rate and complexity of soil organic matter turnover mechanisms [Figure 1A][47,48]. Moreover, the complexity is heightened in systems with alternating wet and dry cycles, such as rice-wheat rotations. Generally, the slow organic matter decomposition under flooded conditions is well-understood, but the impact of wet-dry alternation on organic matter decomposition remains unclear[49,50], as the rewetting soil after prolonged drought stimulates microbial activity, and accelerates organic matter decomposition[32,51]. Furthermore, frequent wet-dry alternation favors the formation of composite structures, such as Fe-organic matter associations, promoting carbon fixation through adsorption and co-precipitation processes [Figure 1B][52,53].
SOC STORAGE CALCULATION METHODS
Statistical models
Statistical models are built on large datasets using regression analysis to elucidate the functional relationships between variables[54]. They predict SOC based on the principles of soil carbon sequestration and organic matter decomposition, using meteorological factors, soil physicochemical properties, and anthropogenic disturbance factors as independent variables dominating SOC reserves[55-57]. For instance, Burke et al. constructed a SOC estimation model employing annual temperature, annual precipitation, and soil clay fraction with data from the United States. Great Plain, and silt fraction was set as the input parameters through an all-possible-subsets regression analysis[55]. The model could explain over 50% of the variation in SOC for croplands and grasslands in the U.S. Great Plain. However, it exhibited an overall underestimation of 30% when assessing the SOC in the Inner Mongolia region of China[56]. The limited applicability of statistical models across different regions is a crucial issue attributed to the lack of consideration for regional differences in carbon inputs, especially the net primary production. Previous study utilized multiple regression analysis to enhance the applicability of statistical models in estimating SOC stocks across diverse regions, which considered soil texture, pH, and groundwater level as primary driving factors, and also introduced specific parameters for different land-use types, leading to more precise predictions[58]. However, this model exhibits lower accuracy in grid-based simulations due to the lack of consideration of climate effects on SOC changes and relevant parameters for management and soil erosion, which are known determinants of SOC accrual and loss[59,60].
Incorporating more predictive parameters is a crucial approach to enhance the accuracy of statistical models. Increasing parameters places higher demands on the statistical methods, and conventional regression analysis methods often struggle to meet these modeling requirements. In recent years, machine learning methods have been widely employed in the construction of SOC prediction models to improve modeling efficiency and simulation accuracy[61-63]. Were et al. utilized 19 predictors, including climate data, land cover data, and digital elevation model (DEM) for machine learning modeling of SOC reserves in agricultural ecosystems[57]. They analyzed the performance of random forests, support vector machines, and artificial neural networks in simulating SOC reserves, and found that soil total nitrogen is the most crucial factor explaining SOC changes, with the support vector machine algorithm performing best, explaining 64% of SOC variation. Another machine learning study showed that the random forest algorithm generally outperformed other algorithms in simulating SOC reserves, while hybrid models within the regression kriging framework showed better simulation results than any single model[64]. Generally, the selection of parameters in the model is related to the simulation scale. At the regional scale, climate is the predominant factor influencing SOC changes, whereas at the farm or plot scale, the impacts generated by land use and vegetation characteristics are more significant.
Due to the high operability and scalability, statistical models have been widely applied in SOC spatiotemporal mapping at either regional, national, or global scale, often integrated with geographical data interpolation or remote sensing methods to address data gaps[57,58]. Furthermore, statistical models are helpful in predicting the comprehensive effect of various environmental factors and management practices on SOC storage. However, there are significant discrepancies among different statistical models regarding the dominant factors influencing SOC changes and their respective impact magnitudes. This divergence may be attributed to the statistical models overlooking the mechanistic processes of SOC changes. The weakness of statistical models in mechanistic understanding prevents them from accurately reflecting the response characteristics of SOC dynamics to complex management practices, rendering them insufficient to support simulations of SOC changes in multi-cropping systems, farms, and smaller scales[64].
Process-based models
Carbon pools and carbon fluxes
Crop models typically comprise an ensemble of several sub-models simulating the influence of management on crop growth, soil water, senescence, nutrient turnover, and organic matter dynamics[65]. As most widely used SOC models assumed, crop residues or other organic amendments are first applied to soil; afterwards, organic carbon decomposes at a specific rate, only parts of which go to the SOC pools after the death of microbes. Decomposition rates and flow directions varied largely in different SOC pools; carbon typically turns out from one pool to another or is mineralized[28,66]. Most widely used process-based SOC models, including Century-DayCent, CERES, DNDC, STICS, APSIM, and DAISY, exhibit significant differences in SOC pool divisions, carbon fluxes, and factors influencing carbon turnover rates, as shown in Figure 2[67]. Models generally divide crop residues into a series of litter organic carbon pools (LOC), considering the discrepancies in decomposition rates and flow direction between different types of carbon. Specifically, LOC is divided into structural carbon and metabolic carbon in DayCent, while a recalcitrant pool is added in DAISY[66,68]. STICS applies a simpler method, dividing LOC into a “labile pool” and a “recalcitrant pool”, while DNDC has an additional “very labile pool”[69,70]. CERES and APSIM divide LOC pools into carbohydrate, cellulose, and lignin based on plant physiology[67,71]. On the other hand, the “active, slow and passive SOC pool” was incorporated in DayCent[66,72]. Additionally, microbial biomass is divided into different fractions according to the resistance and turnover rate in DNDC and DAISY, which have more than one microbial pool[68,73]. However, some models simplify the SOC pools; for example, STICS does not have a slow pool, and CERES has the simplest model structure, considering all SOC as a single pool[74,75].
Determinants of SOC decomposition
Soil temperature and soil moisture are considered the most important environmental factors for simulating SOC decomposition in all process-based models. Different models use multiplication factors to reflect the influences of environmental factors on SOC decomposition [Table 1]. In general, soil microorganisms incorporate carbon based on a specific C:N ratio, and thus, the C:N ratio of LOC or SOC, as well as the soil mineral N availability, are important factors affecting the carbon turnover rate[66,67]. However, CERES ignores the effect of C:N ratio, and the N availability is not considered a factor in CERES and DayCent[71]. Furthermore, soil texture and soil anaerobic condition are considered factors in DayCent, DAISY, and DNDC, to simulate the oxygen demand of microbes when decomposing SOC[68,69,74].
Comparisons of factors driving SOC in widely used process-based models
Influence factors of decomposition rate | Reference | ||||||
Soil temperature | Soil moisture | C: N | Soil texture | Soil N availability | Anaerobic condition | ||
APSIM | √ | √ | √ | √ | Probert et al. (1998)[75] Probert et al. (2005)[67] Baum et al.(2023)[76] | ||
CERES | √ | √ | Gijsman et al. (2002)[71] Luo et al.(2021)[77] | ||||
DAISY | √ | √ | √ | √ | √ | Hansen et al. (2012)[68] Laub et al.(2021)[78] | |
DayCent | √ | √ | √ | √ | √ | Parton et al. (1987)[66] Necpálová et al. (2015)[74] | |
DNDC | √ | √ | √ | √ | √ | Li (2000)[73] Gilhespy et al.(2014)[79] | |
STICS | √ | √ | √ | √ | Brisson et al. (2003)[80] Levavasseur et al. (2021)[70] |
Uncertainties in simulating SOC
Process-based models have been widely used in simulating SOC in diverse areas across the world, mainly in single-cropping systems. However, challenges still exist when models are applied in multiple-cropping systems due to the diversified carbon inputs and more intensive agricultural management[81-84]. The crop growing processes, such as dry matter accumulation and nitrogen uptake of different crops, need to be more accurately simulated in multi-cropping systems[85]. Surface soil is more frequently influenced by tillage and compaction in multi-cropping systems, which requires more sensitivity in modeling the agronomic management effects[86,87]. Additionally, the conversion between aerobic and anaerobic conditions in paddy-upland rotation systems plays an important role in SOC dynamic, which is not commonly well simulated in most plant-soil models[84].
The uneven distribution of microorganisms and plant residues in the soil brings great uncertainty in simulating carbon mineralization and sequestration. Microorganisms play a leading role in SOC transformations, and their community structure and spatial distribution are influenced by numerous factors, such as soil mineral and organic matter characteristics[88]. Previous studies showed that process-based models have overlooked the evolutionary dynamics of soil microorganisms, as shown in Table 1. However, recent studies indicated that the SOC sequestration rate will rapidly decrease as the SOC stock increases, achieving carbon saturation earlier than previously expected[89]. The high stability of inert organic matter in classical models has also been questioned; the resistance based on biochemical properties is now believed to withstand decomposition for only several decades, rather than the previously thought thousands of years[90]. These new perspectives were derived from studies on the dynamics of microbial communities related to soil organic matter, indicating that crop-soil models should incorporate more microbial mechanisms. Previous studies indicated that the CENTURY model was efficiently improved by employing microbial enzymatic litter transformation methods[91], resulting in simulation results close to field measurements and satellite estimates[92]. Researchers have integrated the DAMM (Dual Arrhenius Michaelis-Menten) soil flux model into an ecosystem model, which better simulated the inter-annual dynamics of soil respiration compared to the original model[93,94]. These studies showed that incorporating microbial enzymatic reactions into process-based models is possible, while the characterization of microbially-mediated litter decomposition schemes is not yet widespread and further research is needed.
Model calibration is vital to reduce the uncertainties in simulating SOC using large amounts of field observations. However, the observed data are not always complete enough to optimize all parameters, and SOC storage usually changes too slowly to show significant differences in a relatively short period, which aggravates the challenge[95,96]. In general, an incubation experiment with lower errors is a potentially effective method to purposefully determine some of the parameter values, such as the potential decomposition rate of residue and the corresponding optimal soil temperature and moisture. However, the ideal values obtained from incubation experiments under specific environments often cannot directly support model calibrations. In addition, the SOC pools in the models are hypothetical and cannot be directly quantified through experimental methods[97,98]. Therefore, it is necessary to design incubation experiments reasonably considering a wide range of environmental conditions, which should be helpful for understanding the mechanisms of SOC transformation and provide more effective guidance for modeling work[99].
The steady-state method of IPCC
The IPCC updated the SOC estimation methods for cropland in 2019[100]. Generally, there are three tiers in estimating SOC generated by IPCC, where higher tiers provide more accurate results but also demand stricter requirements for input data details and software technology [Figure 3]. IPCC Tier 1 and Tier 2 are established based on the SOC balance hypothesis, while Tier 3 involves the process-based modeling methods as described in section 3.2. The SOC balance hypothesis posits that, under long-term unchanged land use, SOC reserves will reach and maintain a balanced state determined by factors such as climate, soil, and management measures. IPCC Tier 1 provides the reference soil organic carbon (SOCref) considering climate zones and soil classifications, along with impact coefficients corresponding to different land uses and management measures. For SOC assessments in specific regions, the users only need to look for SOCref and its corresponding impact coefficients and easily obtain the SOC reserves. If there is a change in land use, SOC reserves will transition to a new equilibrium. IPCC Tier 1 roughly considers this process as a linear change with a standard 20-year time span for the transition. Consequently, utilizing IPCC Tier 1 may introduce a considerable degree of variability in assessing SOC changes.
Figure 3. Comparative analysis of IPCC SOC tiered approaches. SOC: soil organic carbon; IPCC: Intergovernmental Panel on Climate Change.
IPCC Tier 2 categorizes SOC into active, slow, and passive carbon pools referencing the Century model framework, which assumes the steady state and turnover rates of each pool and calculates the annual variation in organic carbon reserves[100]. Initially, IPCC Tier 2 requires the provision of initial values for the capacities of the three carbon pools. Typically, carbon inputs over the recent 20 years or a longer period (average annual carbon input, lignin content of materials, carbon-nitrogen ratio), climate data (monthly average temperature and precipitation), and soil information (percentage of sand) are used for carbon pool initialization. The detailed calculation process of IPCC Tier 2 is illustrated in Figure 4. Specifically, for each year during the inventory period, the first calculations involve water (wfac) and temperature (tfac) impact coefficients. These coefficients, combined with the potential decomposition rates of the carbon pools (kfac-p, kfac-s, and kfac-a for passive, slow, and active pools, respectively), tillage coefficient (tillfac), and sand percentage, yield the actual decomposition rates (kp, ks, ka). Subsequently, the theoretical steady states for each carbon pool (PASSIVEy*, SLOWy*, ACTIVEy*) are calculated based on the decomposition rates and carbon inputs, including input amount (Cinput), lignin proportion of materials (LC), and nitrogen fraction of the carbon input (NC). Finally, each carbon pool approaches its theoretical steady state based on the actual decomposition rates, resulting in the annual change. The calculation process of IPCC Tier 2 indicated that SOC reserves will approach theoretical steady states in a nonlinear manner, which better aligns with actual patterns compared to the linear change assumed by IPCC Tier 1. Nevertheless, both IPCC Tier 1 and Tier 2 can only estimate SOC at the 0-30 cm soil layer.
Figure 4. Process for quantifying annual change in soil organic carbon using the Tier 2 method. Transfer coefficients (f1-f8) for determining the flow direction of C, and the calculation formula are detailed in the IPCC 2019 report[100]. IPCC: Intergovernmental Panel on Climate Change.
IPCC Tier 2 is most suitable for widespread application among the three methods, as it is more operationally feasible and allows optimization of key parameters based on regional characteristics to enhance simulation accuracy compared to IPCC Tier 3 (process-based model). Previous studies showed that IPCC Tier 2 performed much better than other assessment methods in evaluating the long-term response of SOC reserves to crop types and straw management[101]. The limitations of the IPCC Tier 2 method mainly lie in its applicability to specific cropping patterns. Currently, it is primarily suitable for assessing SOC dynamics in rainfed single-cropping systems and cannot be directly applied to paddy fields and multi-cropping systems[100]. Accurately evaluating the impacts of residue diversity in multi-cropping systems and the effects of wet-dry alternation processes on SOC using the IPCC Tier 2 still presents significant challenges.
PRACTICAL STRATEGY TO ESTIMATE SOC STORAGE IN MULTI-CROPPING SYSTEMS
An accurate and easily conducted SOC calculation method in multi-cropping systems is beneficial for precisely estimating SOC storage in cropland. Though both statistical models and process-based models can provide relatively robust simulations when abundant observations are available, they need very strong academic skills and cannot be easily operated. This seriously influences their adaptations in SOC estimation. Our review indicates that IPCC Tier 2 is a potentially widely used SOC estimation method for multi-cropping systems if we solve the challenges concluded above. Therefore, practical strategies to improve the IPCC Tier 2 are essential for realizing the easy estimation of SOC in multi-cropping systems with high accuracy.
Revising the parameters of IPCC Tier 2
Generally, IPCC Tier 2 cannot be directly applied to estimate soil carbon sequestration in multi-cropping systems, mainly because carbon input, environmental impact factors, and carbon pool decomposition rates are calculated at the annual scale in the standard algorithm. It is available to designate each growing season as the inventory unit in IPCC Tier 2 to enable its application in multi-cropping systems. Firstly, the carbon input from each crop growing season can be determined, incorporating lignin proportions and carbon-to-nitrogen ratios specific to the crop type. The maximum decomposition amount for each carbon pool per season can be initially calculated by converting the maximum decomposition amount from the entire year to the growing season. The temperature and moisture impact coefficients for carbon pool decomposition rates, initially calculated monthly and averaged over 12 months, can be adjusted to reflect the monthly averages within a season in multi-cropping systems. However, the subdivision of the computational unit will result in changes to many parameter values, and comprehensive parameter databases are needed for extensive calibration and validation. Enhancing the IPCC Tier 2 method for multi-cropping systems will promote consistency in SOC assessment methodologies, though validating its applicability will entail a considerable duration.
Optimizing the region-specific parameters of IPCC Tier 2
The lack of regional agricultural parameter databases hampers the widespread application of the IPCC Tier 2 method in simulating soil organic carbon in multi-cropping systems. The Bayesian calibration was utilized in the IPCC report (2019) to provide default parameter values globally in ensuring the accessibility of the IPCC Tier 2 method. Simultaneously, countries are advised to build region-specific parameter databases based on field sampling data to enhance the accuracy of SOC estimates. However, it is very difficult to measure/determine the carbon partitioning pathway and carbon pools in soils due to the variations in soil, vegetation and climate. This is a limitation in resolving soil spatiotemporal heterogeneity at a large scale. Therefore, the widespread issue of missing sampling data makes it challenging to construct specific parameter databases for many regions[102]. Process-based models have been demonstrated to be very efficient in addressing this data scarcity[65,82]. Sufficiently high-frequency field trial data, leveraging high-resolution climate and soil data, are beneficial for calibrating process-based models. The well-calibrated process models can be applied to diverse regions and scenarios. This can replace actual sampling and provide data support for the regional calibration of IPCC Tier 2. Ensuring the accuracy of process model simulations is fundamental to implementing this strategy, and multi-model integration has also been proven to be an effective approach to reducing simulation uncertainties[103-105]. Multi-model ensembles were identified to significantly improve the accuracy of SOC simulations in cropping systems[106,107]. Furthermore, algorithms like genetic optimization, Bayesian calibration, and maximum likelihood estimation can be utilized to ascertain the optimal values for region-specific parameters in IPCC Tier 2, thereby achieving accuracy comparable to multi-model integration. The strategy of multi-model integration and multi-algorithm fusion calibration aims to establish relevant parameter thresholds for IPCC Tier 2 in different regions, and build a parameter database for SOC in multi-cropping systems in order to ensure the robustness and accuracy of SOC estimation.
Improving the structure of IPCC Tier 2
The overview of the IPCC methodology indicated that introducing the priming effect and wet-dry alternation to the IPCC Tier 2 method should improve the model algorithm. The IPCC 2019 report explicitly mentioned the inapplicability of the current Tier 2 method to rice cultivation, and further developments in IPCC Tier 2 may be applicable to other land uses[100]. Therefore, it is essential to clarify whether there is a mechanistic difference in SOC steady-state between paddy fields and uplands. Generally, applications of meta-analysis and random forest algorithms should be helpful for determining the key driving factors for the priming effect and physical protection effect. Subsequently, the application of machine learning techniques can help construct the wet-dry alternation sub-modules align with IPCC Tier 2 guidelines[108,109]. Moreover, soil microorganisms are crucial driving factors for the priming effect[110], and incorporating the corresponding mechanism into the model should be necessary[111]. Although the efficiency of carbon assimilation by microorganisms and its substrate use efficiency have been considered by IPCC Tier 2 in the decay rates for carbon pools (kp, ka, and ks), they are still determined solely by weather conditions[112]. However, the increase in microbial biomass due to the priming effect is ignored by IPCC Tier 2 when changing crops. The potential decomposition rate of SOC (kfac-p, kfac-s, and kfac-a) may need to increase in months when wet-dry alternation occurs, with new coefficients being added to define the relevant function [Figure 4]. Further work is essential to determine the values of new parameters, including the effects of weather conditions and residue characteristics on the magnitude of the priming effect, by synthesizing various research findings from diverse regions.
If the above-mentioned improvements and revisions were successfully finalized for the IPCC Tier 2, a more accurate depiction of SOC turnover in multi-cropping systems can be achieved. Consequently, CO2 emissions and carbon footprints from relevant food production processes can be more precisely predicted using a more unified framework. These enhancements will address the gaps in previous agricultural carbon footprint calculations, and have the potential to contribute to updates in global ecosystem models for designing low-carbon development strategies in the future.
CONCLUSIONS AND PERSPECTIVES
This study comprehensively analyzed the advantages and deficiencies of the major soil carbon sequestration assessment methods, namely statistical models, process-based models, and the IPCC Tier 2, in estimating SOC storage for multi-cropping systems. It is difficult to simulate the dynamic evolution of SOC at the field scale using the statistical models based on regression, while the well simulation through process-based models demands data richness. The IPCC Tier 2 stands out for its clear structure and operational simplicity. Developed for global applications, it has the potential for widespread use. However, IPCC Tier 2 cannot be directly applied to assess SOC in multi-cropping systems due to parameter mismatches. To address this bottleneck, a shorter time scale is essential to account for discrepancies in input residues and the associated seasonal climatic conditions. Furthermore, the determination of certain constant parameters and multi-model ensembles could help meet the data requirements. Additionally, the effects of flooding and wet-dry alternation, which are lacking in the current method, should be incorporated into new equations in the advanced version. These revisions would address the current shortcomings and inaccuracies in assessing organic carbon stocks in multi-cropping systems, providing methodological support to enhance the SOC assessments in global cropland.
DECLARATIONS
Authors’ contributions
Designed the study: Yin X, Chen F, Bai Z, Zhang D
Contributed to materials collection and literature analysis: Wang Z, Harrison MT, Liu K
Proofread the manuscript and provided directional guidance: Song Z, Chen F
Supervised the study and provided the funding: Yin X
All authors wrote, reviewed, and edited the manuscript.
Availability of data and materials
Not applicable.
Financial support and sponsorship
This study was financially supported by the National Key Research & Development Program of China (2023YFD2302300).
Conflicts of interest
Yin X is an Editorial Board member of Carbon Footprints, while the other authors declared that there are no conflicts of interest.
Ethical approval and consent to participate
Not applicable.
Consent for publication
Not applicable.
Copyright
© The Author(s) 2024.
REFERENCES
1. Scharlemann JP, Tanner EV, Hiederer R, Kapos V. Global soil carbon: understanding and managing the largest terrestrial carbon pool. Carbon Manag 2014;5:81-91.
2. Zou J, Yang Y, Shi S, et al. Farm-scale practical strategies to reduce carbon footprint and emergy while increasing economic benefits in crop production in the North China plain. J J Clean Prod 2022;359:131996.
3. 4 per 1000 research program, 2017. Available from: https://4p1000.org/?lang=en. [Last accessed on 25 Nov 2024]
5. Borrelli P, Robinson DA, Fleischer LR, et al. An assessment of the global impact of 21st century land use change on soil erosion. Nat Commun 2017;8:2013.
6. Zomer RJ, Bossio DA, Sommer R, Verchot LV. Global sequestration potential of increased organic carbon in cropland soils. Sci Rep 2017;7:15554.
7. Henry B, Dalal R, Harrison MT, Keating B. Creating frameworks to foster soil carbon sequestration. In: Rumpel C, editor. Understanding and fostering soil carbon sequestration. Burleigh Dodds Science Publishing Limited. 2023; pp.767-808. Available from: https://library.oapen.org/handle/20.500.12657/61538. [Last accessed on 22 Nov 2024].
8. Sándor R, Ehrhardt F, Grace P, et al. Ensemble modelling of carbon fluxes in grasslands and croplands. Field Crop Res 2020;252:107791.
9. Ren W, Banger K, Tao B, Yang J, Huang Y, Tian H. Global pattern and change of cropland soil organic carbon during 1901-2010: Roles of climate, atmospheric chemistry, land use and management. Geogr Sustain 2020;1:59-69.
10. Lal R. Soil carbon sequestration impacts on global climate change and food security. Science 2004;304:1623-7.
11. Muleke A, Harrison MT, Eisner R, et al. Clarifying confusions over carbon conclusions: antecedent soil carbon drives gains realised following intervention. Glob. Environ Change Adv 2023;1:100001.
12. Poeplau C, Don A. Carbon sequestration in agricultural soils via cultivation of cover crops - a meta-analysis. Agric Ecosyst Environ 2015;200:33-41.
13. Brewer KM, Gaudin AC. Potential of crop-livestock integration to enhance carbon sequestration and agroecosystem functioning in semi-arid croplands. Soil Biol Biochem 2020;149:107936.
14. Gaudaré U, Kuhnert M, Smith P, et al. Soil organic carbon stocks potentially at risk of decline with organic farming expansion. Nat Clim Change 2023;13:719-25.
15. Gregorich EG, Janzen H, Ellert BH, et al. Litter decay controlled by temperature, not soil properties, affecting future soil carbon. Glob Chang Biol 2017;23:1725-34.
16. Chenu C, Angers DA, Barré P, Derrien D, Arrouays D, Balesdent J. Increasing organic stocks in agricultural soils: knowledge gaps and potential innovations. Soil Till Res 2019;188:41-52.
17. Amelung W, Bossio D, de Vries W, et al. Towards a global-scale soil climate mitigation strategy. Nat Commun 2020;11:5427.
18. Kan ZR, Liu WX, Liu WS, et al. Mechanisms of soil organic carbon stability and its response to no-till: a global synthesis and perspective. Glob Chang Biol 2022;28:693-710.
19. Bilotto F, Harrison MT, Migliorati MA, et al. Can seasonal soil N mineralisation trends be leveraged to enhance pasture growth? Sci Total Environ 2021;772:145031.
20. Bilotto F, Vibart R, Mackay A, Costall D, Harrison MT. Towards an integrated phosphorus, carbon and nitrogen cycling model for topographically diverse grasslands. Nutr Cycl Agroecosyst 2022;124:153-72.
21. Yin X, Song Z, Shi S, et al. Developments and prospects of multiple cropping in China. Farm Syst 2024;2:100083.
22. Waha K, Dietrich JP, Portmann FT, et al. Multiple cropping systems of the world and the potential for increasing cropping intensity. Glob Environ Chang 2020;64:102131.
23. Zhao X, Wang Y, Cai S, et al. Legacy nitrogen fertilizer in a rice-wheat cropping system flows to crops more than the environment. Sci Bull 2024;69:1212-6.
24. Wang C, Wang X, Zhang Y, et al. Integrating microbial community properties, biomass and necromass to predict cropland soil organic carbon. ISME Commun 2023;3:86.
25. Garsia A, Moinet A, Vazquez C, Creamer RE, Moinet GYK. The challenge of selecting an appropriate soil organic carbon simulation model: a comprehensive global review and validation assessment. Glob Chang Biol 2023;29:5760-74.
26. Chen T, Hu R, Zheng Z, et al. Soil bacterial community in the multiple cropping system increased grain yield within 40 cultivation years. Front Plant Sci 2021;12:804527.
27. Kroschewski B, Richter C, Baumecker M, Kautz T. Effect of crop rotation and straw application in combination with mineral nitrogen fertilization on soil carbon sequestration in the thyrow long-term experiment thy_D5. Plant Soil 2023;488:121-36.
28. Parton WJ, Scurlock J, Ojima DS et al. Observations and modeling of biomass and soil organic matter dynamics for the grassland biome worldwide. Glob Biogeochem Cycles 1993;7:785-809.
29. Li S, Li J, Li C, et al. Testing the RothC and DNDC models against long-term dynamics of soil organic carbon stock observed at cropping field soils in North China. Soil Till Res 2016;163:290-7.
30. Tao F, Huang Y, Hungate BA, et al. Microbial carbon use efficiency promotes global soil carbon storage. Nature 2023;618:981-5.
31. Cotrufo MF, Ranalli MG, Haddix ML, Six J, Lugato E. Soil carbon storage informed by particulate and mineral-associated organic matter. Nat Geosci 2019;12:989-94.
32. Weil R, Brady N. The nature and properties of soils. 15th ed. Pearson International; 2016. p.1104. Available from: https://elibrary.pearson.de/book/99.150005/9781292162249. [Last accessed on 25 Nov 2024]
34. Drewnik M, Skiba M, Szymański W, Żyła M. Mineral composition vs. soil forming processes in loess soils - a case study from Kraków (Southern Poland). CATENA 2014;119:166-73.
35. Muhammad H, Fahad S, Saud S, et al. A Paradigm shift towards beneficial microbes enhancing the efficiency of organic and inorganic nitrogen sources for a sustainable environment. Land 2023;12:680.
36. Kögel-knabner I. The macromolecular organic composition of plant and microbial residues as inputs to soil organic matter. Soil Biol Biochem 2002;34:139-62.
37. Kögel-knabner I. The macromolecular organic composition of plant and microbial residues as inputs to soil organic matter: fourteen years on. Soil Biol Biochem 2017;105:A3-8.
38. Angst G, Mueller KE, Castellano MJ, Vogel C, Wiesmeier M, Mueller CW. Unlocking complex soil systems as carbon sinks: multi-pool management as the key. Nat Commun 2023;14:2967.
39. Huang Q, Huang Y, Wang B, et al. Metabolic pathways of CO2 fixing microorganisms determined C-fixation rates in grassland soils along the precipitation gradient. Soil Biol Biochem 2022;172:108764.
40. Wu J, Bao X, Zhang J, et al. Temporal and spatial effects of crop diversity on soil carbon and nitrogen storage and vertical distribution. Soil Tillage Res 2024;235:105913.
41. Lin BJ, Li RC, Liu KC, et al. Management-induced changes in soil organic carbon and related crop yield dynamics in China's cropland. Glob Chang Biol 2023;29:3575-90.
42. Bonkowski M. Protozoa and plant growth: the microbial loop in soil revisited. New Phytol 2004;162:617-31.
43. Singh S, Ghoshal N, Singh K. Variations in soil microbial biomass and crop roots due to differing resource quality inputs in a tropical dryland agroecosystem. Soil Biol Biochem 2007;39:76-86.
44. Baumann K, Marschner P, Smernik RJ, Baldock JA. Residue chemistry and microbial community structure during decomposition of eucalypt, wheat and vetch residues. Soil Biol Biochem 2009;41:1966-75.
45. Kuzyakov Y. Priming effects: Interactions between living and dead organic matter. Soil Biol Biochem 2010;42:1363-71.
46. Fontaine S, Henault C, Aamor A, et al. Fungi mediate long term sequestration of carbon and nitrogen in soil through their priming effect. Soil Biol Biochem 2011;43:86-96.
47. Hall SJ, Russell AE, Moore AR. Do corn-soybean rotations enhance decomposition of soil organic matter? Plant Soil 2019;444:427-42.
48. Panettieri M, Guigue J, Chemidlin Prevost-bouré N, et al. Grassland-cropland rotation cycles in crop-livestock farming systems regulate priming effect potential in soils through modulation of microbial communities, composition of soil organic matter and abiotic soil properties. Agr Ecosyst Environ 2020;299:106973.
49. Timsina J, Connor D. Productivity and management of rice-wheat cropping systems: issues and challenges. Field Crop Res 2001;69:93-132.
50. Chen X, Hu Y, Feng S, et al. Lignin and cellulose dynamics with straw incorporation in two contrasting cropping soils. Sci Rep 2018;8:1633.
51. Yang C, Yang L, Ouyang Z. Organic carbon and its fractions in paddy soil as affected by different nutrient and water regimes. Geoderma 2005;124:133-42.
52. Wang Y, Wang H, He JS, Feng X. Iron-mediated soil carbon response to water-table decline in an alpine wetland. Nat Commun 2017;8:15972.
53. Chen X, Hu Y, Xia Y, et al. Contrasting pathways of carbon sequestration in paddy and upland soils. Glob Chang Biol 2021;27:2478-90.
54. Viaud V, Angers DA, Walter C. Toward landscape-scale modeling of soil organic matter dynamics in agroecosystems. Soil Sci Soc Am J 2010;74:1847-60.
55. Burke IC, Yonker CM, Parton WJ, Cole CV, Flach K, Schimel DS. Texture, climate, and cultivation effects on soil organic matter content in U.S. grassland soils. Soil Sci Soc Am J 1989;53:800-5.
56. Evans SE, Burke IC, Lauenroth WK. Controls on soil organic carbon and nitrogen in Inner Mongolia, China: a cross-continental comparison of temperate grasslands. Global Biogeochem Cycles 2011;25:B3006.
57. Were K, Bui DT, Dick ØB, Singh BR. A comparative assessment of support vector regression, artificial neural networks, and random forests for predicting and mapping soil organic carbon stocks across an afromontane landscape. Ecol Indic 2015;52:394-403.
58. Meersmans J, De Ridder F, Canters F, De Baets S, Van Molle M. A multiple regression approach to assess the spatial distribution of soil organic carbon (SOC) at the regional scale (Flanders, Belgium). Geoderma 2008;143:1-13.
59. Yang Y, Ti J, Zou J, et al. Optimizing crop rotation increases soil carbon and reduces GHG emissions without sacrificing yields. Agr Ecosyst Environ 2023;342:108220.
60. Sándor R, Ehrhardt F, Basso B, et al. C and N models intercomparison - benchmark and ensemble model estimates for grassland production. Adv Anim Biosci 2016;7:245-7.
61. Malone B, Mcbratney A, Minasny B, Laslett G. Mapping continuous depth functions of soil carbon storage and available water capacity. Geoderma 2009;154:138-52.
62. Rossel RV, Behrens T. Using data mining to model and interpret soil diffuse reflectance spectra. Geoderma 2010;158:46-54.
63. Grimm R, Behrens T, Märker M, Elsenbeer H. Soil organic carbon concentrations and stocks on barro colorado island - digital soil mapping using random forests analysis. Geoderma 2008;146:102-13.
64. Lamichhane S, Kumar L, Wilson B. Digital soil mapping algorithms and covariates for soil organic carbon mapping and their implications: a review. Geoderma 2019;352:395-413.
65. Harrison MT, Roggero PP, Zavattaro L. Simple, efficient and robust techniques for automatic multi-objective function parameterisation: case studies of local and global optimisation using APSIM. Environ Modell Softw 2019;117:109-33.
66. Parton WJ, Schimel DS, Cole CV, Ojima DS. Analysis of factors controlling soil organic matter levels in great plains grasslands. Soil Sci Soc Am J 1987;51:1173-9.
67. Probert M, Delve R, Kimani S, Dimes J. Modelling nitrogen mineralization from manures: representing quality aspects by varying C:N ratio of sub-pools. Soil Biol Biochem 2005;37:279-87.
68. Hansen, P. Abrahamsen, C. T. Petersen, M. Styczen. Daisy: model use, calibration, and validation. Tran ASABE 2012;55:1317-35.
69. Qiu J, Li C, Wang L, Tang H, Li H, Van Ranst E. Modeling impacts of carbon sequestration on net greenhouse gas emissions from agricultural soils in China. Global Biogeochem Cy 2009;23:2008GB003180.
70. Levavasseur F, Mary B, Houot S. C and N dynamics with repeated organic amendments can be simulated with the STICS model. Nutr Cycl Agroecosyst 2021;119:103-21.
71. Gijsman AJ, Hoogenboom G, Parton WJ, Kerridge PC. Modifying DSSAT crop models for low-input agricultural systems using a soil organic matter-residue module from CENTURY. Agrono J 2002;94:462-74.
72. Parton WJ, Hartman M, Ojima D, Schimel D. DAYCENT and its land surface submodel: description and testing. Global Planet Change 1998;19:35-48.
73. Li C. Modeling trace gas emissions from agricultural ecosystems. Nutr Cycl Agroecosys 2000;58:259-76.
74. Necpálová M, Anex RP, Fienen MN, et al. Understanding the DayCent model: calibration, sensitivity, and identifiability through inverse modeling. Environ Modell Softw 2015;66:110-30.
75. Probert ME, Dimes JP, Keating BA, Dalal RC, Strong WM. APSIM's water and nitrogen modules and simulation of the dynamics of water and nitrogen in fallow systems. Agr syst 1998; 56:1-28.
76. Baum ME, Sawyer JE, Nafziger ED, et al. Evaluating and improving APSIM's capacity in simulating long-term corn yield response to nitrogen in continuous - and rotated-corn systems. Agric Syst 2023;207:103629.
77. Luo Y, Zhang Z, Zhang L, Cao J. Spatiotemporal patterns of winter wheat phenology and its climatic drivers based on an improved pDSSAT model. Sci China Earth Sci 2021;64:2144-60.
78. Laub M, Ali RS, Demyan MS, et al. Modeling temperature sensitivity of soil organic matter decomposition: splitting the pools. Soil Biol. Biochem 2021;153:108108.
79. Gilhespy SL, Anthony S, Cardenas L, et al. First 20 years of DNDC (DeNitrification DeComposition): model evolution. Ecol Modell 2014;292:51-62.
80. Brisson N, Gary C, Justes E, Roche R, Mary B, et al. An overview of the crop model STICS. Eur J Agron 2003;18:309-32.
81. Luo Z, Wang E, Sun OJ, Smith CJ, Probert ME. Modeling long-term soil carbon dynamics and sequestration potential in semi-arid agro-ecosystems. Agr Forest Meteorol 2011;151:1529-44.
82. Luo Z, Eady S, Sharma B, et al. Mapping future soil carbon change and its uncertainty in croplands using simple surrogates of a complex farming system model. Geoderma 2019;337:311-21.
83. Caride C, Piñeiro G, Paruelo JM. How does agricultural management modify ecosystem services in the argentine pampas? Agr Ecosyst Environ 2012;154:23-33.
84. Kraus D, Weller S, Klatt S, et al. How well can we assess impacts of agricultural land management changes on the total greenhouse gas balance (CO2, CH4 and N2O) of tropical rice-cropping systems with a biogeochemical model? Agr Ecosyst Environ 2016;224:104-15.
85. Wang G, Luo Z, Han P, Chen H, Xu J. Critical carbon input to maintain current soil organic carbon stocks in global wheat systems. Sci Rep 2016;6:19327.
86. Kucharik CJ, Brye KR, Norman JM, Foley JA, Gower ST, Bundy LG. Measurements and modeling of carbon and nitrogen cycling in agroecosystems of southern wisconsin: potential for SOC Sequestration during the next 50 years. Ecosystems 2001;4:237-58.
87. Liu DL, Chan KY, Conyers MK. Simulation of soil organic carbon under different tillage and stubble management practices using the Rothamsted carbon model. Soil Till Res 2009;104:65-73.
88. Lehmann J, Hansel CM, Kaiser C, et al. Persistence of soil organic carbon caused by functional complexity. Nat Geosci 2020;13:529-34.
89. Moinet GYK, Hijbeek R, van Vuuren DP, Giller KE. Carbon for soils, not soils for carbon. Glob Chang Biol 2023;29:2384-98.
90. Dynarski KA, Bossio DA, Scow KM. Dynamic stability of soil carbon: reassessing the “permanence” of soil carbon sequestration. Front Environ Sci 2020;8:514701.
91. Fujita Y, Witte JM, van Bodegom PM. Incorporating microbial ecology concepts into global soil mineralization models to improve predictions of carbon and nitrogen fluxes. Glob Biogeochem Cycles 2014;28:223-38.
92. Bhanja SN, Wang J, Bol R. Soil CO2 emission largely dominates the total ecosystem CO2 emission at canadian boreal forest. Front Environ Sci 2022;10:898199.
93. Davidson EA, Samanta S, Caramori SS, Savage K. The dual arrhenius and michaelis-menten kinetics model for decomposition of soil organic matter at hourly to seasonal time scales. Global Change Biol 2012;18:371-84.
94. Sihi D, Davidson EA, Chen M, et al. Merging a mechanistic enzymatic model of soil heterotrophic respiration into an ecosystem model in two AmeriFlux sites of northeastern USA. Agric For Meteorol 2018;252:155-66.
96. Zimmermann M, Leifeld J, Schmidt MWI, Smith P, Fuhrer J. Measured soil organic matter fractions can be related to pools in the RothC model. Eu J Soil Sci 2007;58:658-67.
97. Bruun S, Jensen LS. Initialisation of the soil organic matter pools of the daisy model. Ecol Mode 2002;153:291-5.
98. Dimassi B, Guenet B, Saby NP, et al. The impacts of CENTURY model initialization scenarios on soil organic carbon dynamics simulation in French long-term experiments. Geoderma 2018;311:25-36.
99. Kwon H, Grunwald S. Inverse modeling of CO2 evolved during laboratory soil incubation to link modeled pools in CENTURY with measured soil properties. Soil Sci 2015;180:28-32.
100. Buendia E, Tanabe K, Kranjc A, Jamsranjav B, Fukuda M, et al. Intergovernmental Panel on Climate Change. 2019 Refinement to the 2006 IPCC guidelines for national greenhouse gas inventories. 2019. Available from: https://www.ipcc-nggip.iges.or.jp/public/2019rf/vol4.html. [Last accessed on 22 Nov 2024].
101. Thiagarajan A, Liang C, Macdonald JD, et al. Prospects and challenges in the use of models to estimate the influence of crop residue input on soil organic carbon in long-term experiments in Canada. Geoderma Reg 2022;30:e00534.
102. Villarino SH, Studdert GA, Laterra P, Cendoya MG. Agricultural impact on soil organic carbon content: testing the IPCC carbon accounting method for evaluations at county scale. Agr Ecosyst Environ 2014;185:118-32.
103. Yin X, Kersebaum K, Beaudoin N, et al. Uncertainties in simulating N uptake, net N mineralization, soil mineral N and N leaching in European crop rotations using process-based models. Field Crops Res 2020;255:107863.
104. Asseng S, Ewert F, Rosenzweig C, et al. Uncertainty in simulating wheat yields under climate change. Nat Clim Change 2013;3:827-32.
105. Yin X, Kersebaum KC, Kollas C, et al. Multi-model uncertainty analysis in predicting grain N for crop rotations in Europe. Eur J Agron 2017;84:152-65.
106. Riggers C, Poeplau C, Don A, Bamminger C, Höper H, Dechow R. Multi-model ensemble improved the prediction of trends in soil organic carbon stocks in German croplands. Geoderma 2019;345:17-30.
107. Bruni E, Chenu C, Abramoff RZ, et al. Multi-modelling predictions show high uncertainty of required carbon input changes to reach a 4‰ target. Eur J Soil Sci 2022;73:e13330.
108. Xiao Y, Xue J, Zhang X, et al. Improving pedotransfer functions for predicting soil mineral associated organic carbon by ensemble machine learning. Geoderma 2022;428:116208.
109. Yang Y, Chen X, Liu L, et al. Nitrogen fertilization weakens the linkage between soil carbon and microbial diversity: a global meta-analysis. Glob Chang Biol 2022;28:6446-61.
110. Liu Y, Chen Y, Wang Y, Lu H, He L, Yang S. Negative priming effect of three kinds of biochar on the mineralization of native soil organic carbon. Land Degrad Dev 2018;29:3985-94.
111. Wieder WR, Bonan GB, Allison SD. Global soil carbon projections are improved by modelling microbial processes. Nat Clim Change 2013;3:909-12.
Cite This Article
How to Cite
Bai, Z.; Zhang, D.; Wang, Z.; Harrison, M. T.; Liu, K.; Song, Z.; Chen, F.; Yin, X. Challenges and strategies in estimating soil organic carbon for multi-cropping systems: a review. Carbon Footprints 2024, 3, 19. http://dx.doi.org/10.20517/cf.2024.15
Download Citation
Export Citation File:
Type of Import
Tips on Downloading Citation
Citation Manager File Format
Type of Import
Direct Import: When the Direct Import option is selected (the default state), a dialogue box will give you the option to Save or Open the downloaded citation data. Choosing Open will either launch your citation manager or give you a choice of applications with which to use the metadata. The Save option saves the file locally for later use.
Indirect Import: When the Indirect Import option is selected, the metadata is displayed and may be copied and pasted as needed.
About This Article
Copyright
Data & Comments
Data
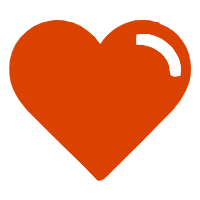
Comments
Comments must be written in English. Spam, offensive content, impersonation, and private information will not be permitted. If any comment is reported and identified as inappropriate content by OAE staff, the comment will be removed without notice. If you have any queries or need any help, please contact us at [email protected].