Seagrass ecosystem biodiversity mapping in part of Rote Island using multi-generation PlanetScope imagery
Abstract
Remote sensing offers an effective and efficient solution to provide information on the biodiversity of seagrass ecosystems, which is currently lacking in most parts of the world. Therefore, this study aimed to map the biodiversity of seagrass ecosystems in parts of Rote Island, which is one of the seagrass biodiversity hotspots, using multi-generation PlanetScope magery to see how they compare. The most frequently used biodiversity indicators were identified, including the major benthic habitat (coral, seagrass, macroalgae, bare substrate) and the composition of seagrass species based on life forms. We also aim to understand the actual biodiversity indicators of seagrass ecosystems captured by PlanetScope imagery. To achieve this, field data was integrated with the resulting ISODATA classification results to assess what ISODATA class clusters represent in the field, and new classification schemes are developed accordingly. The random forest algorithm was used to carry out the classification, with seagrass field data serving as training data. Independent field data was subsequently used to assess the accuracy. The results showed that the accuracy of benthic habitat and seagrass mapping ranged from 60%-70%. However, through the use of a classification scheme built on ISODATA clustering, the spatial distribution of classes and accuracy of all PlanetScope images was significantly improved to > 90%. This highlighted the importance of understanding which indicators of seagrass biodiversity were effectively captured by PlanetScope images to achieve higher mapping accuracy. Overall, this approach optimized the ability of PlanetScope images to map seagrass biodiversity while obtaining a higher number of biodiversity indicator classes and mapping accuracy than the commonly used biodiversity indicator classification scheme.
Keywords
INTRODUCTION
PlanetScope imagery is a high spatial resolution multispectral satellite image, which possesses the highest temporal resolution currently available, being recorded daily[1]. This attribute arises from the multitude of satellites within the Planet constellation[2,3]. The elevated temporal resolution of PlanetScope imagery offers notable advantages, enabling users to acquire images that correspond to the dates of field surveys, select images with minimal cloud cover during the study period, conduct regular monitoring at the necessary frequency, and opt for images with the most optimal recording conditions based on the requirements of the mapping application.
The PlanetScope image maintains a 3-meter ground sample distance (GSD), situating it at the same level of precision as the IKONOS (4 m GSD) and Quickbird (2.4 m GSD) images. One potential application of time-series PlanetScope images is seagrass mapping[4-6]. Given that environmental conditions within seagrass beds are influenced by sunglint, waves, and tides, the selection of an ideal image takes precedence over applying extensive corrections, such as those for sunglint and water column[7]. The abundance of PlanetScope image archives empowers users to choose images with the most favorable conditions for seagrass mapping. Moreover, the PlanetScope image archives have facilitated the creation of a Global Coral Atlas, offering an overview of the distribution of benthic habitats, including seagrass beds, across the Earth’s surface[8-10].
Most previous studies used PlanetScope imagery and employed machine learning to map biophysical information of seagrass[5,11-14]. The high resolution in the PlanetScope imagery also optimizes object-based classification approach for the mapping of benthic habitat which includes seagrass[15]. In addition, in the classification process, the PlanetScope imagery is combined with other supporting spatial data such as bathymetry[6,15] and reef zonation[8] to maximize object-based and machine learning classification results. However, the use of PlanetScope imagery for mapping seagrass beds has several challenges, which are mainly caused by the high level of noise on water pixels and also the inconsistency of image acquired between sensors in the Planet constellation[4-6]. Several previous studies have shown varying degrees of accuracy in using PlanetScope imagery for this purpose[4-6,11-13]. Moreover, issues related to radiometric inconsistency of PlanetScope images, which make the direct comparison between them difficult, have been previously documented[5,6,16-18].
Following the results of benthic habitat and seagrass mapping from PlanetScope imagery since its release, it appears that the mapping accuracy could vary significantly between studies. Meanwhile, some have managed to achieve respectable accuracy[4,15], while others have raised concerns about the accuracy and consistency of the results[5]. Although the Global Coral Atlas[8] uses PlanetScope images to map global coral reefs, its results are inconsistent due to image radiometric disparities, hindering the model’s robustness and causing several areas of seagrass to be classified as rock, rubble, or coral. Furthermore, the use of inexpensive sensors has also been identified as a contributing factor to these inconsistencies, making comparisons challenging even when the images have undergone atmospheric correction[6,16-18]. A similar inconsistency was reported by[6] when mapping seagrass in Seychelles. For example, images captured on adjacent dates may exhibit significant differences in reflectance when taken using different satellite IDs, unlike the more consistent Sentinel-2 L2A product[19].
The Planet company has recently launched the SuperDove, a third-generation sensor that is technologically more advanced than the first generation (Dove Classic) and second generation (Dove-R) in terms of spectral resolution and radiometric quality[20,21]. Compared to the earlier sensors, the SuperDove has eight bands with additional coastal blue, second green, yellow, and red-edge bands, which were previously unavailable. These bands have the ability to penetrate water bodies, making them useful for mapping seagrass beds[22,23]. The availability of seven effective bands, in contrast to the previous three, provides significant benefits for seagrass mapping. The near-infrared band is also useful for seagrass mapping, but only during low tide and for sunglint correction when required. Furthermore, the company has made various improvements in terms of radiometric quality, thereby reducing inconsistencies, artifacts/noise, and making the image more suitable for multitemporal analysis[21,24].
This paper presents the mapping of seagrass ecosystem biodiversity using PlanetScope Surface Reflectance Product (SR) obtained from Dove Classic, Dove-R, and SuperDove sensors. We limit our research to the commonly available PlanetScope product, excluding the more advanced Planet Fusion (PF) product[24], which is unavailable to us. The targeted seagrass ecosystem biodiversity indicators are benthic habitat and species composition distribution. Additionally, this study aims to understand the biodiversity indicators of seagrass ecosystems optimally captured by PlanetScope images, and construct a classification scheme for mapping seagrass biodiversity, with the goal of comprehending the optimal seagrass biodiversity classification scheme for PlanetScope imagery. Seagrass biodiversity information is important as it can be related to the ability to store carbon, which differs among each species. Larger species with high complexity can store more carbon stock than small species with low complexity[25]. Therefore, understanding seagrass biodiversity becomes essential, despite the existing challenges, which include different species composition within a pixel, varying water depth, and differing water turbidity affecting the pixel’s reflectance. According to[26], calculating seagrass biodiversity based on an ecological approach needs to consider both biotic and abiotic variables, and remote sensing can provide spatial and temporal information related to these aspects. The study was conducted in Rote Island, Rote Ndao Regency, East Nusa Tenggara Province, Indonesia [Figure 1], with the main location being Nemberala Village, in the Southwest Rote Sub-district.
METHODS
Study area
The seagrass beds on Rote Island are primarily distributed across the reef flats and lagoons with tides reaching ± 2 m and are exposed to the water surface during low tides. Moreover, they have a very high biodiversity, with eight different species identified, namely Enhalus acoroides, Thalassia hemprichii, Cymodocea rotundata, Thalassodendron ciliatum, Cymodocea serrulata, Halodule uninervis, Syringodium isoetifolium, and Halophila ovalis. The study area is characterized by dense seagrass meadows that extend up to 1 km from the shoreline. The presence of various macroalgae species that grow between seagrasses, such as Padina spp., Halimeda spp., Sargassum spp., Caulerpa spp., Udotea spp., Eucheuma spp., Dictyota spp., and Ulva spp. also add the biodiversity of seagrass meadow in the study area.
PlanetScope image
The details of the three PlanetScope SR Product imagery used in this study can be seen in Table 1. All imagery was acquired between the dates of 23rd and 27th August 2021. As a result, each image captures comparable seagrass conditions within the study area. These represent the most suitable images obtainable nearest to the date of the seagrass field survey (9-16 September 2021) and are expected to correspond accurately to the seagrass’s actual condition in the field. Moreover, because they were captured within a similar timeframe, these images are directly comparable to one another. The main difference between them is their radiometric quality and the tides during image acquisition. The Dove Classic and SuperDove were acquired during low tide, while Dove-R was captured during high tide. Unfortunately, no Dove-R image was captured during low tide close to the survey date. Figure 2 clearly shows that the reflectance variation of Dove-R is the lowest due to the addition of the water column effect during high tide. Sunglint also affected the Dove-R image, making it the lowest-quality image. Nevertheless, the variation in image quality is beneficial in assessing the impact of sunglint and the water column on the quality of PlanetScope images to deliver meaningful underwater object information variation.
Comparison of specifications between PlanetScope sensors for this study
Sensor | Dove Classic | Dove-R | SuperDove |
Date | 26 August 2021 | 23 August 2021 | 27 August 2021 |
Spatial ground sample distance (GSD) | 3 m | 3 m | 3 m |
Radiometric resolution (bits) | 12 (scaled to 16) | 12 (scaled to 16) | 12 (scaled to 16) |
Spectral bands | Blue (0.455-0.515 µm) | Blue (0.464-0.517 µm) | Coastal blue (0.431-0.452 µm) |
Green (0.500-0.590 µm) | Green (0.547-0.585 µm) | Blue (0.465-0.515 µm) | |
Red (0.590-0.670 µm) | Red (0.650-0.682 µm) | Green 1 (0.513-0.549 µm) | |
NIR (0.780-0.860 µm) | NIR (0.846-0.888 µm) | Green 2 (0.547-0.583 µm) | |
Yellow (0.600-0.620 µm) | |||
Red (0.650-0.682 µm) | |||
Red edge (0.697-0.713 µm) | |||
NIR (0.845-0.885 µm) | |||
Tides (m) | -1.078 | 1.297 | -0.759 |
Field data collection
The field survey was conducted between September 9th and September 16th, 2021, using the photo-transect method[27]. The transect line was determined based on the visual appearance of the spatial distribution of benthic habitats in the PlanetScope Image. The variation in pixel color and texture was assumed to represent the variation in benthic habitat classes. The field data were divided into samples to train the classification algorithm and validation samples to assess the mapping accuracy. While determining the transect locations, we ensured that both the training and validation transects covered the variations in seagrass across the study area. We also made sure that both the training and validation samples had comparable seagrass variations.
During the survey, the surveyor walked through the planned transect line while taking photos of the seagrass meadows, as the tides were extremely low. An underwater camera was used to capture the seagrass field photos, while a handheld GNSS (Garmin 78s) placed in a dry bag was set in tracking mode. It recorded location coordinates every two seconds to georeference the field photos. The photos were taken every two footsteps or within a distance of 1-1.5 m, covering an approximate area of 1 square meter plot. The field photos were automatically linked to GNSS coordinates by using time synchronization between the handheld GNSS and underwater camera through the DNRGarmin software. As a result, all photos were georeferenced, enabling the viewing of their positions in the PlanetScope image, along with the information on the composition of the benthic habitat biodiversity.
Field data interpretation
The resulting seagrass field photographs were interpreted and analyzed using CPCe software[28] to obtain the percentage of benthic habitat cover and species composition in each photo. Each of them had 24 randomly distributed points overlaid, and every point was manually assigned to a specific benthic cover subclass. A total of 16 benthic cover subclasses were used to label each point in the photo [Table 2]. The seagrass class was divided into eight subclasses of seagrass species, while the coral reef class was divided into two subclasses of coral reef condition. The bare substrate class and macroalgae were both divided into three subclasses each, based on the type of substrate and the color of algae pigment, respectively.
The benthic class used for the interpretation of field benthic photos using CPCE
Major class | Subclass (detailed class) |
Cymodocea rotundata (Cr) | |
Cymodocea serrulata (Cs) | |
Enhalus acoroides (Ea) | |
Seagrass (Sg) | Halophila ovalis (Ho) |
Halodule uninervis (Hu) | |
Thalassodendron ciliatum (Tc) | |
Thalassia hemprichii (Th), | |
Syringodium isoetifolium (Si) | |
Coral reef (C) | Live coral (Co) |
Dead coral with algae (DCA) | |
Brown algae (Ba) | |
Macroalgae (M) | Green algae (Ga) |
Red algae (Ra) | |
Carbonate sand (Csd) | |
Bare substrate (BS) | Rubble (Rb) |
Sand with microbenthos (SMb) |
Further processing of the field data is necessary to make it suitable for mapping purposes using remote sensing data. In a classification context, one pixel is considered to represent one object, specifically when selecting pixels for training areas and validation samples[29-31]. Since there are more than one set of field data within PlanetScope image pixels, the average percentage of each benthic cover from the field data located within PlanetScope pixels was calculated using fishnet grids with the size of 3 m × 3 m GSD. Figure 3 provides a systematic illustration of these steps. The modeling and validation samples were rasterized following the GSD of the PlanetScope images before running random forest (RF) classification.
Classification scheme
One of the main issues of mapping seagrass ecosystem biodiversity lies in the development of a suitable classification scheme that can be mapped from remote sensing data. To achieve this, it is essential to assess the existing schemes and analyze what can be mapped using PlanetScope images. An existing scheme for biodiversity indicators is the major benthic habitat distribution classes, consisting of seagrass (Sg), coral reef (C) - including live coral and dead coral with algae - macroalgae (M), and bare substrate (BS)[32]. Therefore, the field data was labeled into one of these major classes based on the dominant percentage of the benthic class present in each photo.
The second biodiversity indicator is the seagrass species composition. Mapping seagrass species composition can be challenging, as evidenced by the varying degree of accuracy obtained by previous works[4,10,33-35]. To address this challenge, a classification scheme developed by[34] based on the variations in seagrass life-forms was used. This classification scheme consists of Ea (long leaf species), EaTh (a combination of long and short leaf species), and ThCr (a combination of short leaf species). This classification scheme is relatively suitable for the study area, as mapping at a more individual species composition level, which includes up to six species grouped in one patch on Rote Island, is impractical. A detailed explanation of this scheme is presented in[34] and the qualitative descriptor of each class can be seen in Table 3.
Class descriptor of seagrass species composition based on their life form[35]
Class name | Species composition | Additional information |
Ea/long leaves | Enhalus acoroides | Species grow and extend vertically within the water column, sometimes reaching the surface. Other seagrass life forms may be present within this class with insignificant coverage |
ThCr/short leaves | Thalassia hemprichii, Cymodocea rotundata, Halodule uninervis, Syringodium isoetifolium, Halophila ovalis | Species grow covering the substrate and do not extend vertically within the water column. Other seagrass life forms may be present within this class with insignificant coverage |
EaTh/long- short leaves | Enhalus acoroides | A mix between Ea-type and ThCr-type species at comparable and proportional coverage |
Thalassia hemprichii, Cymodocea rotundata, Halodule uninervis, Syringodium isoetifolium, Halophila ovalis |
Although the first and second indicators are considered supervised classification schemes based on the main benthic cover and species composition variations found in the field, they do not account for the actual biodiversity indicators captured by PlanetScope imagery. To fully optimize PlanetScope images for seagrass ecosystems biodiversity mapping, a biodiversity indicator classification scheme was developed by identifying truly mappable benthic composition classes from PlanetScope data using the following approach[36].
ISODATA (iterative Self-Organizing Data Analysis Technique) unsupervised classification was used to determine how sensors perceive objects (spectrally, radiometrically, and spatially), what information they can discriminate, and how far apart they can distinguish that information (how much detailed information they can provide)[36]. The ISODATA algorithm was used to generate ten spectral classes from 100 iterations. In the classification process, the parameter of the percentage change of pixels in each spectral class during iteration to 5% was used. Moreover, a maximum limit of only two spectral classes that could be combined during each iteration was used when the cluster distances were closer than the specified range.
The classification results were subsequently converted into vector polygons. Each spectral class resulting from ISODATA classification has several overlapped polygons with the field data. The field data within each spectral class were separated accordingly. Subsequently, these polygons were assigned attributes based on the average percentage value of the main benthic cover class (Sg, C, M, BS) calculated from field data. Therefore, the attribute for each spectral class is the average of the attributes of all polygons in the corresponding class that overlap with the field data.
The following rules were applied to use the spectral class attribute for constructing the classification scheme:
1. When a class has a percentage cover of > 80% or < 80%, and that of other classes is < 20%, it is categorized as dominant,
2. When a class has a percentage cover of < 20%, it is considered irrelevant and its name will not appear in the classification scheme,
3. When the difference between classes is < 20%, it is categorized as mix class,
4. When the difference between classes is > 20%, It is categorized as subclass (the subclass name is added after +)
The steps are systematically shown in Figure 4. This approach was applied for each sensor type of the PlanetScope image; hence each image has a different seagrass classification scheme. The field data were respectively labeled based on these schemes.
Noise reduction
The noise reduction techniques used in this study are Minimum Noise Fraction (MNF)[37] and Principal Component Analysis (PCA)[38,39]. Noise reduction is applied to reduce the noise present in PlanetScope images, specifically in water pixels. MNF and PCA techniques are effective for different types of noise[40]. However, since the characteristics of noise in PlanetScope images are not certainly known, both methods are compared to determine the most optimal. The MNF or PC Bands that were considered noise were eliminated based on the visual inspection and their eigenvalues, while the remaining were inverted to produce noise-reduced reflectance bands.
Sunglint correction
Sunglint correction is an important process of eliminating specular reflections that can affect the seagrass mapping process. This study used a sunglint correction technique developed by[41], which was only applied to images captured by the Dove-R sensor. Conversely, images captured by Dove Classic and SuperDove sensors are not affected by sunglint.
Image masking
Land pixels were masked out using the Normalized Difference Vegetation Index (NDVI) threshold
Seagrass ecosystem biodiversity mapping
Seagrass mapping was carried out using supervised machine learning classification, namely Random Forest (RF)[42]. The RF algorithm is effective in producing accurate results and reducing noise and outliers when splitting random samples[43-45]. This classification method has been successfully used to map benthic habitats[6,35,36,46-48] and various characteristics of seagrass beds[11-13]. The parameter for running the RF classification was set based on the experimental results on the number of trees, as other parameters have no significant effect on accuracy when applied to images with a low number of highly correlated bands. The experiment indicated that the optimum number of trees for benthic habitat and ISODATA-based scheme was 300, while the number of trees for seagrass life-form based classification was 500.
The input bands for the classification include Dove Classic 4-bands, sunglint-corrected Dove-R 4-bands, SuperDove 4-bands, and SuperDove 8-bands, which were either noise-reduced or not. The classification scheme input includes the major benthic habitat scheme, life-form-based scheme, and scheme constructed from ISODATA classification analysis. The training area and accuracy assessment samples were taken from the results of the rasterized analyzed field data. Due to the limitation of field data for the coral and rock platform classes, more samples were added from the visual interpretation of images based on the local knowledge from field survey experience. The classification result from each scheme was tested for accuracy using the confusion matrix method, which reported overall accuracy (OA), user’s accuracy (UA), and producer’s accuracy (PA)[49]. The comparison of the accuracy from the three schemes was used as the basis to determine the most effective classification scheme development approach to be used with PlanetScope image.
The flowchart of this research is provided in Figure 5.
RESULTS
Field seagrass data collection
The field survey was conducted to obtain the benthic habitat and seagrass species composition data on Rote Island. Figure 1 shows the spatial distribution of the collected field, which was divided into samples. One set was used to train the RF algorithm and, the other was used to assess the accuracy of the resulting classification. Table 4 presents the number of samples for the benthic habitat and seagrass life-form classification scheme after being integrated into PlanetScope GSD. Although the life-form scheme used was classified into three, there was no mono-specific meadow of Enhalus acoroides in the study area. Hence, the seagrass class was only divided into two, namely EaTh and ThCr. For the scheme developed based on ISODATA classification, the number of samples can be found in the “classification scheme” section.
The number of samples for benthic habitat and seagrass life-form classification scheme
Number of samples | Number of samples | ||||
Benthic habitat class | Training area | Accuracy assessment | Seagrass life-form class | Training area | Accuracy assessment |
Ea | 0 | 0 | |||
Seagrass (Sg) | 1,122 | 631 | EaTh | 107 | 53 |
ThCr | 1,024 | 587 | |||
Coral reef (C) | 147 | 139 | Coral reef (C) | 147 | 139 |
Macroalgae (M) | 212 | 109 | Macroalgae (M) | 217 | 115 |
Bare substrate (BS) | 96 | 113 | Bare substrate (BS) | 82 | 98 |
First biodiversity indicator: benthic habitat mapping
The OA difference of benthic habitat maps produced from the three sensors was within 7% [Table 5]. The highest OA obtained from Dove Classic, Dove-R, SuperDove 4 band and SuperDove 8 band were 68.3%, 61.0%, 66.5%, and 67.4%, respectively. The OA from Dove-R was the lowest due to the high tide, the presence and the difficulties of removing the sunglint from the image, as well as the noise that emerged as a result of the process. Despite the application of MNF and PCA, there was no improvement in the OA, and the accuracy of the SR bands was found to be more consistent than the denoised bands. The reduction of the noise by excluding the low MNF and PC band eigenvalue bands did not aid the mapping effort. It is highly possible that the information in the later MNF and PCA bands still had useful information, although mixed with noise. As a consequence, eliminating the noise band also removed a portion of information useful for mapping.
Overall accuracy summary (in %) of benthic habitat mapping from PlanetScope images. SR – surface reflectance, Deglint – sunglint-corrected, Denoise – noise reduction applied
Image | SR | SR deglint | Denoise MNF | Denoise PCA | Avera ge | Stdev |
Dove Classic | 68.3 | - | 65.6 | 65.0 | 66.3 | 1.8 |
Dove-R | - | 61.0 | 54.0 | 51.3 | 55.4 | 5.0 |
SuperDove 4-bands | 66.4 | - | 66.5 | 65.8 | 66.2 | 0.4 |
SuperDove 8-bands | 67.4 | - | 67.0 | 63.9 | 66.1 | 1.9 |
Average | 67.4 | 61.0 | 63.3 | 61.5 | ||
Stdev | 1.0 | - | 6.2 | 6.9 |
The spatial distribution of benthic habitat between sensor generations is relatively similar, with the majority of difference being the extent of coral class in the reef crest area. The Dove-R failed to map the coral class in the reef crest area and instead classified it as seagrass [Figure 6]. The distribution of seagrass, macroalgae, and bare substratum is relatively comparable between sensors. The pattern of PA and UA between sensors is similar, with the seagrass class having the highest, followed by bare substrate and coral class, while the macroalgae class had the lowest. The noise reduction effort managed to improve the UA and PA of macroalgae (5% on average) but at the expense of other classes (decreased up to 2.5% on average). The misclassification mainly involved the seagrass class since it has the highest number of samples, hence it was considered overestimated. The gaps in seagrass meadows covered by dead coral, macroalgae, and bare substrate were also classified as seagrass. Macroalgae are frequently misclassified as coral and bare substrate, which is not surprising since the reflectance of live and dead corals covered by particular algae (DCA) is similar to those of brown macroalgae[50,51]. The misclassification between macroalgae and seagrass can be attributed to the green pigment in green macroalgae, which is similar to healthy green seagrass[35,52], brown macroalgae resembling damaged seagrass leaves or seagrass covered by epiphytes[52,53], and also the location of macroalgae in between seagrass canopy. Macroalgae can also be misclassified as substrate due to its low cover and vice versa when the layer of microbenthos is present on top of the substrate. It appears that these misclassifications can be attributed to the nature of the seagrass ecosystems and may not be solely linked to the PlanetScope sensors quality.
Second biodiversity indicator: seagrass life-form mapping
The classification was conducted on SR and SR deglint, and not on the MNF and PCA results, based on the outcomes of the benthic habitat mapping. The results were similar to those of benthic habitat mapping as there was no obvious accuracy [Table 6] or spatial distribution differences [Figure 7] from all sensors, except for Dove-R. The results from all images showed that the EaTh class was underestimated, while ThCr class was overestimated.
Figure 7. Seagrass life-form maps from multi-generation PlanetScope imagery (left to right: Dove Classic, Dove-R, SuperDove 4-bands, SuperDove 8-band).
Summary of accuracy assessment of benthic habitat mapping with detailed seagrass classes from PlanetScope images. SR - surface reflectance, Deglint - sunglint-corrected
Image | SR | SR deglint |
Dove classic | 62.5 | - |
Dove-R | - | 55.4 |
SuperDove 4-bands | 62.2 | - |
SuperDove 8-bands | 62.9 | - |
Mean | 62.5 | 55.4 |
Third biodiversity indicator: seagrass ecosystem biodiversity mapping based on PlanetScope images
The results of the ISODATA classification showed that the spectral classes created were highly inconsistent. The percentage of pixel changing in a class on each iteration was more than 5% of the initial cluster. Consequently, the maximum number of iterations (100) became the stopping criteria for completing the ISODATA classification. This indicates that the spectral class is not solid enough to be considered as a proper class since its pixels member keeps changing on different iterations. This should be carefully considered when mapping benthic habitats with PlanetScope images.
Following the approach explained in the section “classification scheme”, the benthic composition represented by each spectral class was determined and the uniqueness of each class was concluded to be assessed by the composition of each major benthic habitat class (seagrass, bare substrate, coral, macroalgae). Based on the rules previously explained in the “classification scheme” section, a classification scheme was constructed for each PlanetScope sensor [Tables 7-10].
The classification scheme for seagrass biodiversity indicator using ISODATA clustering based on PlanetScope Dove Classic sensor. There are six unique classes. The value in the bracket is the number of samples
Class No. | Scheme Dove Classic | Quantitative class descriptor |
Class 1 | Sg Dominated | Seagrass ≥ 62%, other cover < 20% (T = 674, A = 275) |
Class 2 | Sg Dominated | Seagrass ≥ 62%, other cover < 20% (T = 674, A = 275) |
Class 3 | Sg + BS | Seagrass 54%, Seagrass 30%, other cover < 20% (T = 216, A = 110) |
Class 4 | Mix Sg BS | Seagrass 30%-51%, sand 37%-49%, other cover < 20% (T = 410, A = 488) |
Class 5 | Mix Sg BS | Seagrass 30%-51%, sand 37%-49%, other cover < 20% (T = 410, A = 488) |
Class 6 | Mix Sg BS C | Seagrass 34%, sand 28%, coral/DCA 26%, other cover < 20% (T = 141, A = 61) |
Class 7 | Mix Sg BS | Seagrass 30%-51%, sand 37%-49%, other cover < 20% (T = 410, A = 488) |
Class 8 | BS dominated | ≥ 83%, other cover < 20% (T = 26, A = 12) |
Class 9 | BS+ Sg | Sand 70%, seagrass 26%, other cover < 20% (T = 52, A = 36) |
Class 10 | Mix Sg BS | Seagrass 30%-51%, sand 37%-49%, other cover <20% (T = 410, A = 488) |
The classification scheme for seagrass biodiversity using ISODATA clustering based on PlanetScope Dove-R sensor. There are four unique classes. The value in the bracket is the number of samples
Class No. | Scheme Dove-R | Quantitative class descriptor |
Class 1 | Sg dominated | Seagrass ≥ 64%, other cover < 20% (T = 748, A = 359) |
Class 2 | Sg dominated | Seagrass ≥ 64%, other cover < 20% (T = 748, A = 359) |
Class 3 | Sg dominated | Seagrass ≥ 64%, other cover < 20% (T = 748, A = 359) |
Class 4 | Mix Sg Bs | Seagrass 38%-47%, sand 32%-51%, other cover < 20% (T = 659, A = 495) |
Class 5 | Mix Sg Bs | Seagrass 38%-47%, sand 32%-51%, other cover < 20% (T = 659, A = 495) |
Class 6 | Mix Sg Bs | Seagrass 38%-47%, sand 32%-51%, other cover < 20% (T = 659, A = 495) |
Class 7 | Mix Sg Bs | Seagrass 38%-47%, sand 32%-51%, other cover < 20% (T = 659, A = 495) |
Class 8 | Bs dominated | Sand ≥ 73%, other cover < 20% (T = 44, A = 62) |
Class 9 | Bs dominated | Sand ≥73%, other cover < 20% (T = 44, A = 62) |
Class 10 | Bs + Sg | Sand 54%, seagrass 32%, other cover < 20% (T = 68, A = 66) |
The classification scheme for seagrass biodiversity using ISODATA clustering based on PlanetScope SuperDove 4-bands sensor. There are five unique classes. The value in the bracket is the number of samples
Class No. | Scheme SuperDove 4- bands | Quantitative class descriptor |
Class 1 | Sg dominated | Seagrass ≥ 56%, other cover < 20% (T = 776, A = 381) |
Class 2 | Mix Sg BS | Seagrass 42%-51%, sand 31%-43%, other cover < 20% (T = 435, A = 396) |
Class 3 | Sg dominated | Seagrass ≥ 56%, other cover <20% (T = 776, A = 381) |
Class 4 | Mix Sg BS | Seagrass 42%-51%, sand 31%-43%, other cover < 20% (T = 435, A = 396) |
Class 5 | Sg dominated | Seagrass ≥ 56%, other cover < 20% (T = 776, A = 381) |
Class 6 | Mix Sg BS C | Seagrass 27%-42%, sand 31%-37%, coral/DCA 20%-31% (T = 218, A = 118) |
Class 7 | Mix Sg BS C | Seagrass 27%-42%, sand 31%-37%, coral/DCA 20%-31% (T = 218, A = 118) |
Class 8 | BS dominated | Sand ≥ 71%, other cover < 20% (T = 8, A = 6) |
Class 9 | Mix Sg BS | Seagrass 42%-51%, sand 31%-43%, other cover < 20% (T = 435, A = 396) |
Class 10 | BS + Sg | Sand 65%, seagrass 28%, other cover < 20% (T = 82, A = 81) |
The classification scheme for seagrass biodiversity using ISODATA clustering based on PlanetScope SuperDove 8-bands sensor. There are six unique classes. The value in the bracket is the number of samples
Class No. | Scheme SuperDove 8- bands | Quantitative class descriptor |
Class 1 | Sg dominated | Seagrass ≥ 67%, other cover < 20% (T = 617, A = 269) |
Class 2 | Sg dominated | Seagrass ≥ 67%, other cover < 20% (T = 617, A = 269) |
Class 3 | Mix Sg BS | Seagrass 42%-46%, sand 36%-47%, other cover < 20% (T = 430, A = 347) |
Class 4 | Sg + BS | Seagrass 58%, sand 22%, other cover < 20% (T = 173, A = 159) |
Class 5 | Mix Sg BS C | Seagrass 28%-43%, sand 28%-44%, coral/DCA 21%-22% (T = 217, A = 120) |
Class 6 | BS + Sg | Sand 66%, seagrass 28%, other cover < 20% (T = 73, A = 82) |
Class 7 | Mix Sg BS | Seagrass 42%-46%, sand 36%-47%, other cover < 20% (T = 430, A = 347) |
Class 8 | Mix Sg BS | Seagrass 42%-46%, sand 36%-47%, other cover < 20% (T = 430, A = 347) |
Class 9 | BS dominated | Sand ≥ 70%, other cover < 20% (T = 8, A = 5) |
Class 10 | Mix Sg BS C | Seagrass 28%-43%, sand 28%-44%, coral/DCA 21%-22% (T = 217, A = 120) |
Based on these schemes, the field data on each ISODATA spectral class were labeled and used to train the supervised RF classification algorithm as well as to assess the classification results. However, there were issues using the Dove-R scheme as the ISODATA classification failed to properly cluster pixels with
The overall accuracy summary (in %) of seagrass biodiversity mapping based on the schemes in Tables 7-10. SR - surface reflectance, Deglint - sunglint-corrected
Image | Number of classes | SR | SR deglint |
Dove Classic | 6 | 94.3 | - |
Dove-R | 4 | - | 98.5 |
SuperDove 4-bands | 5 | 95.7 | - |
SuperDove 8-bands | 6 | 96.1 | - |
Mean | 95.6 | 98.5 |
Figure 8 shows the spatial distribution of seagrass biodiversity classes. Although Dove Classic had more class variation in the reef flat area, it could not completely map the Mix Sg Bs C class in the reef crest area. On the other hand, the SuperDove 4- and 8-bands results had similar patterns and provided an accurate representation of seagrass biodiversity classes across the study area, particularly on Mix Sg Bs C class where Dove Classic and Dove-R failed to accurately map. The main difference observed is the absence of Sg + BS class in the perimeter of Sg dominated class in the SuperDove 4-band result. These results highlight the improved performance of the SuperDove sensor in detecting variations in benthic habitats.
DISCUSSION
Mapping benthic habitats such as seagrass meadows, coral reefs, and macroalgae using remote sensing requires a different approach compared to mapping terrestrial objects. This is because it involves more complex electromagnetic energy interactions. Apart from path radiance, electromagnetic energy also interacts with the water surface, the water column, and the bottom object itself[29]. These interactions have effects, with water column attenuation impacting bottom reflectance and causing sunglint to appear under specific image acquisition conditions[41,54,55].
Furthermore, environmental conditions contribute to the complexity of these energy interactions, including factors such as water transparency, bathymetry, and substrate type[56]. In their research[56], demonstrated that benthic objects are more readily identifiable in waters with lower attenuation coefficients, characterized by high transparency or shallow water. They also confirmed the existence of a mapping depth limit due to these energy interactions, although it is still possible to recognize bottom objects up to a depth of 20 meters using visible wavelengths in highly transparent water[51,55].
In our study, the complexity of mapping seagrass biodiversity is low due to the minimal impact of water column energy attenuation and sunglint. This is primarily because the majority of the area mapped consists of very shallow reef flats with minimal underwater topographic variation, and the Dove Classic and SuperDove images were recorded during low tide. Therefore, the variations in reflectance, which serve as the basis for the seagrass biodiversity classes in the produced classification scheme, are primarily due to the benthic cover composition. Meanwhile, the Dove-R images, which were recorded during high tide, yielded the lowest accuracy and exhibited minimal class variation. This indicates that the capability to detect variations in benthic objects is hampered by the overlying water column and the sunglint effect.
Theoretically, it is possible to map the seagrass ecosystem biodiversity at species level, which is the aim of this study. However, there are challenges as reported in previous works[57,58]. Mapping the seagrass biodiversity of monospecific meadows with a large area, such as subtropical regions with low diversity of seagrass species, is less difficult. This has been demonstrated by[59] in New Zealand with Zostera muelleri[60], in the Mediterranean Coast of Turkey with Posidonia oceanica, and[60] in Malaysia with Enhalus acoroides. However, seagrass meadows do not always consist of a single species, specifically in Indo-pacific and Australian regions where seagrass species diversity is very high[10,33,35]. In the location of the current study, the seagrass meadows grow with a mixture of species, and a total of six species were found in a 1 m2 plot of the high seagrass density area during the field activity. These conditions are challenging to map using remote sensing data since a pixel contains more than one species with similar reflectance spectra. It is difficult to differentiate seagrass species by their reflectance spectra due to the limitation in detecting detailed adsorption features and the similarity of spectra between healthy species[35,53]. Therefore, one of the most likely approaches to map the seagrass ecosystem biodiversity using satellite remote sensing data is by relying on their ecological composition[32,61,62].
Generally, mapping seagrass biodiversity using classification scheme provided by the user may or may not work for any satellite image. This is related to the effective descriptive resolution of the satellite image and the mapping method used. This is also one of the reasons why accuracy for a particular satellite image can vary greatly between works, where the given classification scheme is not mappable from the satellite image used. Therefore, understanding what can be effectively mapped will ensure higher map accuracy, not only in terms of statistics but also in the spatial distribution of the object.
In this research, we are trying to bridge the gap in seagrass mapping: between what the user wants to map (which is usually based on an ecological perspective and user-driven) and what can be mapped by the PlanetScope image. What can optimally be mapped from a particular satellite image may not always be less complex than what the user wants to map. In the case of our research, the number of classes that can be mapped from multi-generation PlanetScope images is higher than the frequently used major benthic habitat classification scheme (consisting of coral reefs, seagrass, bare substratum, and macroalgae). Moreover, with the higher number of classes, the accuracy also increases. Typically, a higher number of classes leads to lower accuracy, but since the scheme was created based on the composition of benthic habitat captured by PlanetScope pixels, the resulting mapping accuracy is higher. This is not to conclude that the major benthic habitat classification scheme is not suitable for the PlanetScope image; it is just that the information captured by PlanetScope pixels is not similar to the class descriptor within the major benthic habitat classification scheme, which is based on the dominant benthic cover. This also applies to the life-form class, where the main descriptor is the species composition. After analyzing the accuracy and spatial distribution of benthic classes in the PlanetScope imagery for mapping seagrass life-form and benthic habitat, it became apparent that effectively capturing the biodiversity indicator in PlanetScope images is crucial for achieving better mapping results. The resulting ISODATA classification provided spectral classes and indicated the type of biodiversity information that can be spectrally clustered by PlanetScope image. Based on
By using the aforementioned approach to develop a working classification scheme for PlanetScope imagery, a huge accuracy improvement was achieved, both in terms of overall accuracy and class spatial distribution. However, it is worth noting that despite similar accuracy, the quality of the map delivered from each sensor differs.
Dove Classic - Dove Classic appeared to have better precision and contrast in the reef flat but lacks color variations for the coral/DCA part in the reef crest compared to the SuperDove image [Figure 2]. The color of coral/DCA even appeared similar to that of the green vegetation in the land area. This is evident in the lower accuracy of the coral/DCA class composition in the reef crest area, despite delivering excellent seagrass biodiversity results in the reef flat area.
Dove-R - Dove-R was only capable of mapping four biodiversity classes and the result did not represent the seagrass biodiversity condition in the study area. This is mainly due to the absence of Sg + B and coral/DCA composition classes. Moreover, the image was acquired during high tide, which led to additional noise due to sunglint and water column attenuation energy, greatly reducing the seagrass biodiversity detection capabilities. Figure 2 shows that sunglint is visible on top of the optically shallow water and the water column strongly reduces the reflectance variation in the reef crest, making the coral/DCA composition class indistinguishable from other classes. Sunglint correction is a challenging process when applied to PlanetScope images because the noise in the water pixels makes it difficult to obtain a strong empirical model between the visible and near-infrared bands for proper correction. Consequently, the sunglint correction frequently adds noise to the image and decreases its radiometric quality. Water column correction was not conducted in the study area due to the dominance of reef flats with small depth variation, making it difficult to find a representative training area and develop the water column correction model. In addition, water column correction may lower the image quality when applied on shallow areas with small depth variation[5,48]. It is believed that Dove-R, being an upgrade to the Dove Classic sensor, may deliver similar results with other generation sensors when captured during low tide.
SuperDove - SuperDove image appears visually softer and somewhat has a smoother texture compared to Dove Classic, but exhibits better color variations [Figure 2]. The smoother texture is observed in the area between Sg dominated class, while reef crest is dominated by Mix Sg BS C, unlike the Dove Classic result where Mix Sg BS dominated the reef crest area. In area near the shoreline, the Dove Classic had a better variation with Sg + BS class in between Sg dominated class. Due to its smoother and more generalized image quality, the SuperDove image is incapable of mapping Sg + BS class like Dove Classic when only four bands are used. Nevertheless, it is capable of mapping coral/DCA composition class in the reef crest much more accurately with and without using the additional four bands. Therefore, it is believed that SuperDove delivers improved performance for seagrass mapping as it is capable of distinguishing benthic habitat class composition better. However, the loss of details in the smoother SuperDove image will need to be investigated further.
The new SuperDove sensor has been found to provide better mapping results than the Dove Classic and Dove-R. This study has also shown how the sunglint and the water column can significantly affect the ability of PlanetScope images to detect underwater objects. However, the greatest benefit of the PlanetScope constellation is that it offers daily acquisitions of rich images, even though the usable acquisition frequency is much lower than specified due to inconsistent recording quality and persistent cloud cover in the tropics. This is very useful for objects such as corals that do not have seasonal variations, as images can be selected or composed from a wider range of time to obtain the most ideal[7]. In contrast, for seagrasses and macroalgae, which have monthly and seasonal variations, the selection of suitable monthly images will be more challenging[7,63].
It is also important to mention that Planet has released the Planet Fusion (PF) Product, which utilizes an advanced method called CESTEM (CubeSat-Enabled Spatio-Temporal Enhancement Method)[64,65]. This product has been developed to address issues related to sensor interoperability, cross-calibration challenges, and atmospheric contamination in time-series imagery. The product is expected to be beneficial for tasks such as inter-day change detection, time-series analysis, phenological monitoring, vegetation growth and disturbance monitoring, physically-based model retrieval, machine learning, and other applications relying on radiometric, geometric, and temporal consistency[24]. A future assessment of the PF Product is also required to understand its capabilities for time-series seagrass mapping, where consistent image quality is a significant factor contributing to reliable results. Furthermore, the understanding of seagrass biodiversity can be enhanced with hyperspectral sensors currently available, such as PRISMA, and future hyperspectral constellations like Tanager from Planet. These constellations are also planned to deliver hyperspectral data on a daily to weekly basis in some areas. While satellite hyperspectral sensors usually capture images at medium spatial resolutions, they provide the opportunity for subpixel mapping through unmixing analyses, given the availability of targeted endmembers.
CONCLUSIONS
The key information obtained from the mapping of seagrass biodiversity using multi-generation PlanetScope imagery in parts of Rote Island is as follows. The biodiversity information mapped from images captured from the three sensors is relatively comparable for classes containing variations of seagrass and bare substrates, with the main difference being the superiority of the SuperDove sensor to map coral/DCA composition classes more accurately. Moreover, Dove-R yielded the lowest accuracy and variation of biodiversity information, mainly due to sunglint and water column disturbance. This highlights the importance of selecting images with ideal conditions for seagrass mapping, as image correction may not always work favorably. Finally, it is crucial to understand which indicators of seagrass biodiversity are effectively captured by PlanetScope images in achieving higher mapping accuracy. This approach enables the optimization of the capabilities of PlanetScope images to map seagrass biodiversity while obtaining a wider range of biodiversity indicator classes and higher accuracy (> 90%) than the commonly used user and ecologically driven classification of biodiversity indicators such as dominant benthic habitat and composition of species life forms (< 70%).
DECLARATIONS
AcknowledgmentsThe authors are grateful to Huwaida Nur Salsabila (Remote Sensing Master Program, Universitas Gadjah Mada, Indonesia) and Filman Firdausman (Cartography and Remote Sensing, Universitas Gadjah Mada, Indonesia) for assisting in interpreting the seagrass photos collected in the field.
Authors’ contributionsContribution to the conception and the development of the manuscript: Wicaksono P, Harahap SD, Hafizt M, Maishella A, Yuwono DM
Availability of data and materialsNot applicable.
Financial support and sponsorshipThis study was funded by “Hibah Penelitian Mandiri Dosen Fakultas Geografi Universitas Gadjah Mada 2022” Grant No. 54/UN1/FGE/KPT/SETD/2022. The authors are also grateful to the Planet via Planet Research and Education Program for providing access to the PlanetScope images used in this study.
Conflicts of interestAll authors declared that there are no conflicts of interest.
Ethical approval and consent to participateNot applicable.
Consent for publicationNot applicable.
Copyright© The Author(s) 2023.
REFERENCES
1. Planet Labs. PlanetScope. 2023. Available from: https://developers.planet.com/docs/data/planetscope/ [Last accessed on 22 Sep 2023].
2. Planet Labs Inc. PLANET IMAGERY PRODUCT SPECIFICATIONS. 2019. Available from: https://assets.planet.com/docs/combined-imagery-product-spec-final-may-2019.pdf [Last accessed on 18 Sep 2023].
3. Maroneze MM, Zepka LQ, Vieira JG, Queiroz MI, Jacob-Lopes E. A tecnologia de remoção de fósforo: gerenciamento do elemento em resíduos industriais. Rev Ambiente e Agua 2014;9:445-58.
4. Wicaksono P, Lazuardi W. Assessment of PlanetScope images for benthic habitat and seagrass species mapping in a complex optically shallow water environment. Int J Remote Sens 2018;39:5739-65.
5. Wicaksono P, Maishella A, Lazuardi W, Muhammad FH. Consistency assessment of multi-date PlanetScope imagery for seagrass percent cover mapping in different seagrass meadows. Geocarto Int 2022;37:15161-86.
6. Lee CB, Traganos D, Martin L, Rowlands G, Reinartz P. Nationwide seagrass mapping using analysis-ready Sentinel-2 and PlanetScope data to support the nationally determined contributions of seychelles. 2022. Available from: https://elib.dlr.de/188097/1/ISBW14_seychelles_seagrass.pdf [Last accessed on 18 Sep 2023].
7. Hedley JD, Roelfsema C, Brando V, et al. Coral reef applications of Sentinel-2: coverage, characteristics, bathymetry and benthic mapping with comparison to Landsat 8. Remote Sens Environ 2018;216:598-614.
8. Lyons MB, Roelfsema CM, Kennedy EV, et al. Mapping the world's coral reefs using a global multiscale earth observation framework. Remote Sens Ecol Conserv 2020;6:557-68.
9. Kennedy EV, Roelfsema CM, Lyons MB, et al. Reef cover, a coral reef classification for global habitat mapping from remote sensing. Sci Data 2021;8:196.
10. Roelfsema CM, Lyons M, Kovacs EM, et al. Multi-temporal mapping of seagrass cover, species and biomass: a semi-automated object based image analysis approach. Remote Sens Environ 2014;150:172-87.
11. Astuty IS, Wicaksono P. Seagrass species composition and above-ground carbon stock mapping in Parang Island using Planetscope image. Proceedings of the 6th Geoinformation Science Symposium; 2019 Aug 26-27; Yogyakarta, Indonesia.
12. Ariasari A, Wicaksono P. Random forest classification and regression for seagrass mapping using PlanetScope image in Labuan Bajo, East Nusa Tenggara. Proceedings of the 6th International Symposium on LAPAN-IPB Satellite; 2019 Sep 17-18; Bogor, Indonesia.
13. Munir M, Wicaksono P. Support vector machine for seagrass percent cover mapping using PlanetScope image in Labuan Bajo, East Nusa Tenggara. Proceedings of the 6th International Symposium on LAPAN-IPB Satellite; 2019 Sep 17-18; Bogor, Indonesia.
14. Isnaen Z, Pratama AP, Utami AA, et al. Carbon stock estimation of seagrass species thalassia hempricii using planet imagery with band ratio transformation in nirwana beach, padang city. Proceedings of the 4th International Conference of Indonesian Society for Remote Sensing; 2018 Oct 30; Makassar, Indonesia.
15. Li J, Schill SR, Knapp DE, Asner GP. Object-based mapping of coral reef habitats using planet dove satellites. Remote Sens 2019;11:1445.
16. Houborg R, Mccabe M. High-resolution NDVI from planet’s constellation of earth observing nano-satellites: a new data source for precision agriculture. Remote Sens 2016;8:768.
17. Chénier R, Faucher M, Ahola R. Satellite-derived bathymetry for improving canadian hydrographic service charts. IJGI 2018;7:306.
18. Poursanidis D, Traganos D, Reinartz P, Chrysoulakis N. On the use of Sentinel-2 for coastal habitat mapping and satellite-derived bathymetry estimation using downscaled coastal aerosol band. Int J Appl Earth Obs Geoinf 2019;80:58-70.
19. Wicaksono P, Wulandari SA, Lazuardi W, Munir M. Sentinel-2 images deliver possibilities for accurate and consistent multi-temporal benthic habitat maps in optically shallow water. Remote Sens Appl Soc Environ 2021;23:100572.
20. Planet Labs. PlanetScope. 2023. Available from: https://developers.planet.com/docs/data/planetscope/ [Last accessed on 18 Sep 2023].
21. Planet Labs. Planet imagery product specifications. 2022. Available from: https://assets.planet.com/docs/Planet_Combined_Imagery_Product_Specs_letter_screen.pdf [Last accessed on 22 Sep 2023].
22. Green E, Mumby P, Edwards A, Clark C. Remote sensing handbook for tropical coastal management. Paris: The United Nations Educational, Scientific and Cultural Organization; 2000.
23. Goodman JA, Purkis S, Phinn S. Coral reef remote sensing: a guide for mapping, monitoring and management. London: Springer; 2013.
24. Planet Labs Inc. Planet fusion monitoring technical specification calibration, Analysis Ready Data, and InterOperability (CARDIO) operations. 2021. Available from: https://assets.planet.com/docs/Planet_fusion_specification_March_2021.pdf [Last accessed on 22 Sep 2023].
25. Simpson J, Bruce E, Davies KP, Barber P. A blueprint for the estimation of seagrass carbon stock using remote sensing-enabled proxies. Remote Sens 2022;14:3572.
26. Supriyadi IH, Cappenberg HAW, Wouthuyzen S, et al. Seagrass condition at some small islands in the taka bonerate national marine park, south sulawesi indonesia. J Segara 2021;17:83-96.
27. Roelfsema C, Phinn S. A manual for conducting georeferenced photo transects surveys to assess the benthos of coral reef and seagrass habitats. 2009. Available from: https://sees-rsrc.science.uq.edu.au/Publications/GPS_Photo_Transects_for_Benthic_Cover_Manual.pdf [Last accessed on 18 Sep 2023].
28. Kohler KE, Gill SM. Coral point count with excel extensions (CPCe): a visual basic program for the determination of coral and substrate coverage using random point count methodology. Comput Geosci 2006;32:1259-69.
29. Jensen JR. Remote sensing of the environment: an earth resource perspective. England: Pearson Education Limited; 2014. p. 614.
30. Jensen JR. Introductory digital image processing. Hoboken: Pearson Education; 2015.
31. Lilesand TM, Kiefer RW, Chipman JW. Remote sensing and image interperation. 2015. Available from: https://www.wiley.com/en-us/Remote+Sensing+and+Image+Interpretation%2C+7th+Edition-p-9781118343289 [Last accessed on 18 Sep 2023].
32. Mumby PJ, Harborne AR. Development of a systematic classification scheme of marine habitats to facilitate regional management and mapping of Caribbean coral reefs. Biol Conserv 1999;88:155-63.
33. Phinn S, Roelfsema C, Dekker A, Brando V, Anstee J. Mapping seagrass species, cover and biomass in shallow waters: an assessment of satellite multi-spectral and airborne hyper-spectral imaging systems in Moreton Bay (Australia). Remote Sens Environ 2008;112:3413-25.
34. Wicaksono P, Hafizt M. Mapping seagrass from space: addressing the complexity of seagrass LAI mapping. Eur J Remote Sens 2013;46:18-39.
35. Wicaksono P, Aryaguna PA, Lazuardi W. Benthic habitat mapping model and cross validation using machine-learning classification algorithms. Remote Sens 2019;11:1279.
36. Yuwono DM. Development of a systematic reef habitat classification scheme using multi-scale remotely sensed data for Indonesia’s coastal management. The University of Queensland. 2022. Available from: https://espace.library.uq.edu.au/view/UQ:4d3b2e7 [Last accessed on 18 Sep 2023].
37. Green A, Berman M, Switzer P, Craig M. A transformation for ordering multispectral data in terms of image quality with implications for noise removal. IEEE Trans Geosci Remote Sens 1988;26:65-74.
38. Hotelling H. Analysis of a complex of statistical variables into principal components. J Educ Psychol 1933;24:417-41.
39. Pearson K. LIII. On lines and planes of closest fit to systems of points in space. Lond Edinb Dublin Philos Mag J Sci 1901;2:559-72.
40. Luo G, Chen G, Tian L, Qin K, Qian S. Minimum noise fraction versus principal component analysis as a preprocessing step for hyperspectral imagery denoising. Can J Remote Sens 2016;42:106-16.
41. Hedley JD, Harborne AR, Mumby PJ. Technical note: simple and robust removal of sun glint for mapping shallow-water benthos. Int J Remote Sens 2005;26:2107-12.
43. Pal M. Random forests for land cover classification. IEEE Int Geosci Remote Sens Symp 2003;6:3510-2.
44. Mutanga O, Adam E, Cho MA. High density biomass estimation for wetland vegetation using WorldView-2 imagery and random forest regression algorithm. Int J Appl Earth Obs Geoinf 2012;18:399-406.
45. Senf C, Leitão PJ, Pflugmacher D, van der Linden S, Hostert P. Mapping land cover in complex Mediterranean landscapes using Landsat: Improved classification accuracies from integrating multi-seasonal and synthetic imagery. Remote Sens Environ 2015;156:527-36.
46. Traganos D, Pertiwi AP, Lee CB, et al. Earth observation for ecosystem accounting: spatially explicit national seagrass extent and carbon stock in Kenya, Tanzania, Mozambique and Madagascar. Remote Sens Ecol Conserv 2022;8:778-92.
47. Blume A, Pertiwi AP, Lee CB, Traganos D. Bahamian seagrass extent and blue carbon accounting using Earth Observation. Front Mar Sci 2023;10:1058460.
48. Zhang C, Selch D, Xie Z, Roberts C, Cooper H, Chen G. Object-based benthic habitat mapping in the florida keys from hyperspectral imagery. Estuar Coast Shelf Sci 2013;134:88-97.
49. Congalton RG, Kass. Green, assessing the accuracy of remotely sensed data: principles and practices. CRC Press/Taylor & Francis, 2009. Available: https://books.google.com/books/about/Assessing_the_Accuracy_of_Remotely_Sense.html?id=T4zj2bnGldEC.
50. Kutser T, Dekker AG, Skirving W. Modeling spectral discrimination of great barrier reef benthic communities by remote sensing instruments. Limnol Oceanogr 2003;48:497-510.
51. Hedley J, Roelfsema C, Chollett I, et al. Remote sensing of coral reefs for monitoring and management: a review. Remote Sens 2016;8:118.
52. Thorhaug A, Richardson AD, Berlyn GP. Spectral reflectance of the seagrasses: thalassia testudinum, halodule wrightii, syringodium filiforme and five marine algae. Int J Remote Sens 2007;28:1487-501.
53. Wicaksono P, Kamal M. Spectral response of healthy and damaged leaves of tropical seagrass Enhalus acoroides, Thalassia hemprichii, and Cymodocea rotundata. Proceedings of SPIE Remote Sensing; 2017 Sep 11-14 . Warsaw, Poland.
54. Manessa M, Kanno A, Sekine M, Ampou E, Widagti N, As-syakur A. Shallow-water benthic identification using multispectral satellite imagery: investigation on the effects of improving noise correction method and spectral cover. Remote Sens 2014;6:4454-72.
55. Zoffoli ML, Frouin R, Kampel M. Water column correction for coral reef studies by remote sensing. Sensors 2014;14:16881-931.
56. Sagawa T, Komatsu T. Simulation of seagrass bed mapping by satellite images based on the radiative transfer model. Ocean Sci J 2015;50:335-42.
57. Pittman SJ, Roelfsema C, Thapa B, Otaño Cruz A. Outlining a methodological pathway to improve the global seagrass map. The Pew Charitable Trusts. 2021. Available from: https://www.researchgate.net/publication/359513011 [Last accessed on 18 Sep 2023].
58. Hossain M, Bujang J, Zakaria M, Hashim M. The application of remote sensing to seagrass ecosystems: an overview and future research prospects. Int J Remote Sens 2015;36:61-114.
59. Ha N, Pham T, Pham H, Tran D, Hawes I. Total organic carbon estimation in seagrass beds in Tauranga Harbour, New Zealand using multi-sensors imagery and grey wolf optimization. Geocarto Int 2023;38:2160832.
60. Bakirman T, Gumusay MU, Tuney I. Mapping of the seagrass cover along the mediterranean coast of turkey using landsat 8 OLI images. Int Arch Photogramm Remote Sens Spatial Inf Sci 2016;41:1103-5.
61. Phinn SR, Roelfsema CM, Mumby PJ. Multi-scale, object-based image analysis for mapping geomorphic and ecological zones on coral reefs. Int J Remote Sens 2012;33:3768-97.
62. Wicaksono P. Improving the accuracy of multispectral-based benthic habitats mapping using image rotations: the application of principle component analysis and independent component analysis. Eur J Remote Sens 2016;49:433-63.
63. Wicaksono P, Maishella A, Wahyudi AJ, Hafizt M. Multitemporal seagrass carbon assimilation and aboveground carbon stock mapping using Sentinel-2 in Labuan Bajo 2019-2020. Remote Sens Appl Soc Environ 2022;27:100803.
64. Houborg R, Mccabe MF. A cubesat enabled spatio-temporal enhancement method (CESTEM) utilizing planet, Landsat and MODIS data. Remote Sens Environ 2018;209:211-26.
Cite This Article
How to Cite
Wicaksono, P.; Harahap, S. D.; Hafizt, M.; Maishella, A.; Yuwono, D. M. Seagrass ecosystem biodiversity mapping in part of Rote Island using multi-generation PlanetScope imagery. Carbon Footprints. 2023, 2, 19. http://dx.doi.org/10.20517/cf.2023.09
Download Citation
Export Citation File:
Type of Import
Tips on Downloading Citation
Citation Manager File Format
Type of Import
Direct Import: When the Direct Import option is selected (the default state), a dialogue box will give you the option to Save or Open the downloaded citation data. Choosing Open will either launch your citation manager or give you a choice of applications with which to use the metadata. The Save option saves the file locally for later use.
Indirect Import: When the Indirect Import option is selected, the metadata is displayed and may be copied and pasted as needed.
About This Article
Copyright
Data & Comments
Data
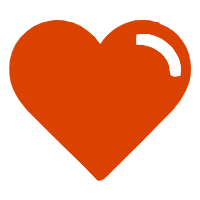
Comments
Comments must be written in English. Spam, offensive content, impersonation, and private information will not be permitted. If any comment is reported and identified as inappropriate content by OAE staff, the comment will be removed without notice. If you have any queries or need any help, please contact us at support@oaepublish.com.