An optimized strategy for density prediction of intermetallics across varied crystal structures via graph neural network
Abstract
Intermetallic compounds are crucial in modern industry due to their exceptional properties, where density is identified as a critical parameter determining their potentiality for lightweight applications. In this study, over 7,000 density data points are collected for binary intermetallic compounds from different crystal structures. A new intermetallics graph neural network (IGNN) model is developed to perform regression and classification tasks for density prediction. Compared to traditional machine learning models, the IGNN model demonstrated superior capability in capturing crystal structure and effectively addressing challenges posed by polymorphism. The interpretability of the IGNN model classification process is enhanced through the t-distributed stochastic neighbor embedding (t-SNE) visualization method. Additionally, the IGNN model exhibited excellent performance in predicting the density of multicomponent complex intermetallic compounds, indicating its robustness and generalizability. This study presents a graph neural network (GNN) method suitable for multi-crystal structure data modeling, providing a novel computational framework for density prediction in intermetallic compounds. This advancement represents a significant contribution to this field, paving the way for more targeted material selection and application in lightweight technologies.
Keywords
INTRODUCTION
Owing to their unique physical and chemical properties, intermetallic compounds are recognized for considerable application potential in modern industry, particularly in aerospace, energy, electronics, and automotive industries[1-3]. These compounds are usually composed of two or more metallic elements in defined ratios, demonstrating excellent mechanical properties, corrosion resistance, thermal stability, and magnetic properties through their specific crystal structures. Such attributes render intermetallic compounds a vital option for creating high-performance and durable materials, meeting the demands of modern technology for materials withstanding extreme conditions[4-6]. Density, a critical parameter of intermetallic compounds, is essential for their material properties and applications, and it directly influences the lightweight design capabilities required in many industrial applications[7-9]. For example, lightweight materials are required in the aerospace and automotive fields to maintain low density without sacrificing strength under extreme conditions, such as high temperatures and pressures, to reduce structural load and improve fuel efficiency while reducing greenhouse gas emissions[10,11].
Although density is crucial for the applications of intermetallic compounds, their experimental measurement is challenging, particularly when involving multiple metallic elements and complex crystal structures. Certain intermetallic compounds may not be reliably synthesized in the laboratory due to preparation difficulties or compound instability, increasing the difficulty and cost of experimental density measurement[12,13]. The efficiency of direct experimental density measurement is considered low, especially when large-scale compound data needs to be studied. Consequently, developing effective methods for density prediction holds practical significance. Density prediction via theoretical models or data-driven methods can reduce experimental costs and time, while also offering a reliable foundation for high-throughput material screening and early design. However, accurate prediction of intermetallic compound density faces some challenges. Conventional physical models such as density functional theory (DFT) are known to yield accurate results on smaller datasets but are inefficient for large-scale density predictions[14,15]. Additionally, physical models struggle to accurately address isomers, where compounds of the same chemical composition exhibit significant density differences due to varying crystal structures. Such factors, relatively common in intermetallic compounds, further complicate and increase the difficulty of prediction.
To address these challenges, data-driven machine learning methods have been gradually introduced into the field of intermetallic compounds in recent years[16-18]. Researchers have employed machine learning algorithms to predict the physical properties of intermetallic compounds. Although these traditional machine learning methods have demonstrated some effectiveness in improving prediction efficiency, they also encounter limitations in handling complex crystal structures, particularly in capturing structural differences between isomers. Traditional machine learning models are generally reliant on Vegard’s law, which calculates the average feature representations by summing the elemental features to the properties of the entire compounds. However, these methods are limited, as they fail to sufficiently incorporate the complex interactions between atoms.
Graph neural networks (GNNs) are recognized as powerful and flexible deep learning tools that represent complex information through patterns and their relationships[19]. GNNs have demonstrated excellent performance in material property prediction. For instance, to overcome the limitations associated with manual feature construction and complex transformations in crystal material design, Xie et al. developed the crystal graph convolutional neural networks (CGCNN) framework to learn material properties directly from crystal structures[20]. It demonstrated DFT-level accuracy for predicting multiple material properties, along with interpretable chemical insights. To enhance the accuracy and efficiency of the CGCNN in predicting material properties, Park et al. developed an improved version (iCGCNN) by incorporating Voronoi tessellation, three-body interactions, and optimized bond representations[21]. The improved model achieved a 20% increase in accuracy and a 2.4-fold rise in the success rate for high-throughput material discovery. To address challenges in predicting material properties and designing new materials in materials science and chemistry, Reiser et al. reviewed the fundamentals of GNNs, current applications, and potential in accelerating simulations, material screening, and inverse design[22].
GNNs offer a novel approach for predicting the density of intermetallic compounds. This is achieved by encoding the crystal structure of compounds as graph-structured data, which facilitates the learning of information atoms and their bonding interactions[23]. The GNN method avoids the complex feature engineering required by traditional methods by capturing directly the information of atoms and bonding relationships with explicit physical and chemical properties[24,25]. Furthermore, the GNN-based method extracts structural differences between crystals using input graph structure data, which consists of topological structures made up of atoms and bonds[26,27]. This methodology uncovers underlying relationships between structural characteristics and material properties, highlighting the potential of GNNs to enhance the accuracy and efficiency of material property predictions. However, existing GNN models may encounter difficulties when directly applied to intermetallic compounds with isomeric structures. These models are not sufficient for direct application to the density prediction of intermetallic compounds across different crystal structures.
In this study, a new intermetallics GNN (IGNN) is constructed to perform regression and classification predictions on the density of over 7,000 binary intermetallic compounds, and to compare its performance with traditional machine learning models. The t-distributed stochastic neighbor embedding (t-SNE) method is employed to visualize the IGNN’s performance in capturing differences across various crystal structures. Furthermore, ternary and quaternary intermetallic compound density datasets are constructed as test sets to validate the effectiveness of the proposed IGNN model. This work presents a novel and efficient approach for predicting the densities of intermetallic compounds, offering valuable insights into materials design and advancing the development of data-driven methods in materials science.
METHODOLOGY
Data sources and preprocessing
Density data for binary intermetallic compounds are collected using the API provided by the materials project website[28]. Only compounds composed of metallic elements are included, while those containing non-metallic elements or noble gases are excluded to ensure data quality and consistency. To guarantee thermodynamic stability, only compounds with an “energy above hull” value of 0 are retained. This selection ensures high stability and reliability for practical use, minimizing prediction errors caused by unstable compounds. Each downloaded data entry includes the following: Material ID, chemical formula, crystal system, density, and crystallographic information file (CIF). CIF is a standardized format used to record the space group, unit cell parameters, atomic coordinates, and atom types of crystalline materials. After data collection, the raw data is cleaned and preprocessed to ensure data quality and enhance the model’s predictive performance. Incomplete or outlier data points are excluded, such as those without density or crystal structure values. A total of 7,253 binary intermetallic compound density data are gathered. These compounds cover seven major crystal structure types: cubic, hexagonal, monoclinic, orthorhombic, trigonal, tetragonal, and triclinic. The diversity of these structural types better represents the complexity of intermetallic compounds in practical applications, providing comprehensive training data for subsequent machine learning modeling.
The pie chart in Figure 1A shows the proportion of data for each crystal structure. Cubic and hexagonal structures are the most common, comprising 29% and 28.3% of the data, respectively. The triclinic structure has the fewest data points, representing only 0.5%. In addition, the periodic table in Figure 1A shows the frequency of elements in the intermetallic compound density dataset. The higher the frequency, the darker the corresponding color. The violin plot illustrates the density distributions of compounds across six crystal structures, as shown in Figure 1B. Each structure is represented by a distinct violin plot. The shape and width of each plot reflect the density distribution, and the white dots within the plots indicate the median density for each crystal structure.
Dataset splitting and feature construction
The dataset in this study is split using the 80/20 method, with 80% of the data used for the training set and the remaining 20% for the test set. Moreover, to further assess the model’s generalization capability and prediction accuracy on complex compounds, density data from 237 ternary and 33 quaternary intermetallic compounds are collected as an independent test set. These multicomponent compound samples cover various element combinations and complex crystal structures, enabling a more comprehensive evaluation of the model’s performance. This study employs several common evaluation metrics to comprehensively evaluate the model’s performance. These include regression metrics such as root mean square error (RMSE)[29], mean absolute error (MAE)[30], and the coefficient of determination (R2)[31], as well as classification metrics such as precision, recall, F1-score, and receiver operating characteristic (ROC) curves[32].
The Python library XenonPy is leveraged to introduce 58 properties of 94 elements (H to Pu), covering multiple aspects such as electronic structures, physicochemical properties, and microscopic geometric structures[33]. An additional nine features related to bond energy are included, resulting in a total of 67 elemental features for constructing machine learning features and serving as input parameters for IGNN model nodes. Detailed information on the 67 element features is given in Table 1. The min-max scaling method is used to normalize the feature set, mapping all values between 0 and 1 to eliminate the influence of scale differences on model performance[34]. This process reduces the model’s sensitivity to specific numerical ranges, preventing adverse effects on model training caused by large data ranges. Furthermore, it preserves the relative distribution of the data while ensuring consistent learning efficiency across different features during model training.
List of features used
Number | Element properties | Number | Element properties |
1 | Electron binding energies | 35 | Gs Est Bcc latcnt |
2 | Nearest neighbor distance | 36 | Gs Est Fcc latcnt |
3 | Atomic concentration | 37 | Gs mag moment |
4 | Configuration energy | 38 | Gs volume Per |
5 | Cohesive energy | 39 | Hhi_p |
6 | Gram atomic volume | 40 | Hhi_r |
7 | Molar mass | 41 | Heat capacity mass |
8 | Screening percentage | 42 | Heat capacity molar |
9 | Enthalpy of vaporization | 43 | Icsd volume |
10 | Enthalpy of fusion | 44 | Heat of formation |
11 | First ionization energy | 45 | Lattice constant |
12 | Atomic radius | 46 | Mendeleev number |
13 | Atomic radius rahm | 47 | Melting point |
14 | Atomic volume | 48 | Molar volume |
15 | Atomic weight | 49 | Num unfilled |
16 | Boiling point | 50 | Num valance |
17 | Bulk modulus | 51 | Num d unfilled |
18 | C6 Gb | 52 | Num d valence |
19 | Covalent radius cordero | 53 | Num f unfilled |
20 | Covalent radius pyykko | 54 | Num f valence |
21 | Covalent radius pyykko double | 55 | Num p unfilled |
22 | Covalent radius pyykko triple | 56 | Num p valence |
23 | Covalent radius slater | 57 | Num s unfilled |
24 | Density | 58 | Num s valence |
25 | Dipole polarizability | 59 | Period |
26 | Electron negativity | 60 | Specific heat |
27 | Electron affinity | 61 | Thermal conductivity |
28 | En allen | 62 | Vdw radius |
29 | En ghosh | 63 | Vdw radius alvarez |
30 | En pauling | 64 | Vdw radius Mm3 |
31 | First ion En | 65 | vdw_radius_uff |
32 | Fusion enthalpy | 66 | Sound velocity |
33 | Gs bandgap | 67 | Polarizability |
34 | Gs energy |
Machine learning algorithms and GNN model
In this study, various commonly used traditional machine learning models, along with the constructed GNN model, are used to predict the density of binary intermetallic compounds. The traditional machine learning models included linear regression (LR), support vector machine (SVM), K-nearest neighbors (KNN), random forest (RF), and EXtreme gradient boosting (XGBoost). LR is a fundamental regression model that assumes a linear relationship between independent and dependent variables. Although its structure is simple, it can still perform effectively when the data exhibits linear characteristics. SVM conducts classification and regression by identifying a hyperplane in the feature space that maximizes class separation. It is particularly effective for small datasets and capable of addressing nonlinear issues. KNN is an instance-based learning method that makes predictions by calculating the distance between test samples and training samples. RF consists of multiple decision trees, each trained on different subsets of data and features. The final prediction is obtained by averaging the outputs or majority voting of all the trees, offering high robustness and noise resistance. XGBoost is an optimized implementation of gradient boosting decision tree, known for its precision and efficiency. It minimizes prediction errors by combining multiple weak learners with weighted contributions, and is capable of handling high-dimensional data and missing values.
The main idea in this study is to represent the crystal structure as graph structure data with nodes corresponding to atoms and edges corresponding to chemical bonds. The entire graph and the corresponding adjacency matrix are constructed based on the locations and connectivity of atoms in the crystal. The properties of the nodes in the graph are represented by physical and chemical properties inherent to the atoms, such as atomic concentration, atomic radius, and boiling point. Mathematically, let
To completely take advantage of the valuable information in crystal graph-structured data, a novel IGNN model is proposed for crystal property prediction based on the topological information and atomic properties in the crystal graph. The model can efficiently capture complex associated relationships between atoms based on the topological characteristics of the given crystal graph-structured data, combining with the atomic features from the prior knowledge and employing a message-passing mechanism for information aggregation, which enables entire graph representation learning to obtain the specific properties of the crystal graph. The method enables the automatic learning of effective representations from the basic compositions and topological information of crystal graph-structured data without the requirement of extensive human-designed feature engineering, allowing the reliable and accurate prediction of material properties. The architecture includes the following critical components: (1) crystal structure transformation; (2) node features construction; and (3) GNN-based graph representation learning.
(1) Crystal structure transformation. Let Atoms = {a1, a2, …, an} denote the set of atoms in the unit cell, where ai indicates the i-th atom. The distance r is assigned to identify the neighbors of each atom in the unit cell, determining the connectivity between the atoms. This enables the transformation of the 3D spatial information of the crystal into graph-structured data. The connectivity between atoms is recorded by constructing an adjacency matrix, ensuring that their interactions are accurately reflected in the graph. The relationship between atoms and their neighbors can be expressed as
where N (ai) denotes the neighbors of atom ai, Atoms is the set of all atoms in the crystal. d (ai, aj) is the distance between atoms ai and aj. Specifically, for each atom, neighbors are identified within 6 Å radius, where these atoms share a Voronoi face and the interatomic distance is shorter than the sum of the Cordero covalent radii to within a 0.25 Å tolerance[20,35].
(2) Node features construction. To obtain prior knowledge about the atoms, feature representations are constructed for each node in the crystal graph. Specifically, atomic properties are extracted as attributes of the nodes in the corresponding crystal graph, derived from the physical and chemical properties of the atoms, informed by prior knowledge. An eigenvector xi for atom ai is defined, containing multiple related physical and chemical properties such as atomic concentration, atomic radius, boiling point, etc. The feature vector can be given as follows:
where the fj (ai) denotes the j-th feature associated with atom ai and m is the total number of features. The feature vectors of all atoms are combined into a feature matrix X, which is defined as follows:
The introduction of prior knowledge allows the model to effectively utilize these physical and chemical properties in the proposed method, thus improving the ability of the model to understand and predict crystal properties.
(3) GNN-based graph representation learning. GNNs are employed as a learning strategy for crystal graph-structured data, using a multi-layer architecture designed to capture complex interactions and global properties between atoms in the given crystal. Specifically, Batch Normalization is utilized as a regularizer to alleviate the internal covariate shift problem in deep neural networks. Normalization and linear transformation enable the mean and variance of the input data in each layer of the network to be within a certain range[36]. The method of data normalization in Batch Normalization is given in
where μ and σ are statistics for the current row and are unlearnable. γ and β are the scale and shift parameters to be learned to control the variance and mean of yi.
After executing Batch Normalization, a linear layer is introduced to further transform the feature representations of the nodes, which enables the mapping of the batch-normalized feature matrix to a new feature space. This linear layer adjustment enhances the model’s capacity to learn higher-order feature representations by altering the dimensions of the features, thereby boosting the model’s expressive power. After obtaining the preprocessed node feature matrix, a l-layer GNN architecture is designed to discern complex patterns and relationships in the crystal graph-structured data. The GNN model is more expressive and generalizable when dealing with crystal structure data by stacking l-layer GNNs. The GNN primarily focuses on identifying a node aggregation function to effectively aggregate node features X, resulting in updated node embedding. For instance, the GCN model with the following layer-wise propagation rule:
where H(l) is the matrix of activations in the l-layer, σ is the activation function, and W is a layer-specific trainable weight matrix.
In the spectrum domain theory, existing methods commonly utilize the convolution theorem and filtering operations in the spectral domain to define convolution operations, such as computing the eigen-decomposition of the normalized Laplace matrix of a graph[19]. The graph convolution in GCN is defined as a first-order Chebyshev polynomial of the graph Laplace matrix, while the graph convolution adopted by the topology adaptive graph convolutional network (TAGCN) method is defined as a multiplication of the polynomials of the graph adjacency matrix[37]. In contrast to the simplified formal spectral method that is equivalent to propagating vertex features in a graph employed in the GCN method, the K-localized filter is employed in TAGCN to explore crystal graph-structured data. Specifically, a set of receptive fields of size ranging from 1 to K is defined to learn localized features on graph-structured data. This approach is given in
Finally, after the k-layer feature aggregation and transformation, the features learned by the GNN are effectively transformed into the final density prediction results. This transformation is accomplished through two linear layers, interspersed with a nonlinear activation function between them, enhancing the model’s ability to predict complex outcomes from the graph-structured data. The learning process for density of crystal structure can be expressed as follows:
where y denotes the predicted density of crystal structure, and H(k) is the output feature matrix after obtaining k-layers of aggregation. W(1) and W(2) are the learnable weight matrices for two linear layers, and
For our method, hyperparameter optimization is conducted over 500 trials using Hyperopt optimizer, with 80% for training and 20% for validation. Final classification accuracies are reported on the test set. All experiments are conducted on a Linux server with Nvidia RTX 4090 GPU (24GB memory). All methods and GNN basic architectures are implemented according to PyTorch 2.1.2 and basic modules of PyTorch Geometric 2.5.3. Key training parameters are fixed through hyperparameter optimization, including a learning rate of lr ≈ 0.002, weight decay w ≈ 0.003, a batch size of 512, and 512 hidden channels. AdamW is used as the optimizer, PowerMeanAggregation is selected for global pooling, and the model includes three graph convolutional layers as learners. The detailed flow chart is shown in Figure 2.
RESULTS AND DISCUSSION
Machine learning modeling for specific crystal structures
To conduct an in-depth analysis and improve the accuracy of density prediction, this study first establishes machine learning models based on the seven different crystal structures. This process of modeling utilizes the five common machine learning models mentioned above. Each crystal structure’s intermetallic compounds are extracted into individual data subsets. The input features selected are variables closely related to density, such as atomic mass, atomic radius, electronegativity difference, and lattice parameters. Six statistical treatments are applied to the elemental property data, such as calculating weighted averages, weighted variances, geometric means, harmonic means, maximum values, minimum values, and weighted sums. An initial feature set comprising 290 representative density features is generated, providing robust data support for comprehensively exploring the multifactorial influences on intermetallic compound density.
Figure 3 presents the performance of machine learning models in predicting density across different crystal structures. A bar chart in the bottom right compares the accuracy of various machine learning models within a given crystal structure. Adjacent to this, a scatter plot shows the relationship between the experimental density values and those predicted by the most effective machine learning model for intermetallic compounds. Figure 3A shows the machine learning model predictions for the Hexagonal system. The bar chart indicates that among the five machine learning models, XGBoost achieves the highest accuracy with R2 = 0.9798, while the SVM model has the lowest accuracy with R2 = 0.8538. The scatter plot in Figure 3A compares XGBoost predictions with calculated values by DFT, where the points cluster closely around the diagonal line (y = x), demonstrating a strong agreement between the predicted and experimental densities. XGBoost still shows the best prediction accuracy for the cubic system, with R2 close to 0.98, as shown in Figure 3B. However, for the monoclinic system, R2 = 0.9825 is achieved by the RF model, which outperforms XGBoost, as depicted in Figure 3C. Figure 3D-F shows that XGBoost achieves the highest accuracy for the remaining systems including orthorhombic, trigonal, and tetragonal systems with R2 values of 0.9692, 0.9825, and 0.9826, respectively. From Figure 3, it can be seen that the data points for each model (especially XGBoost) are mostly aligned along the diagonal line, indicating that the predictions are generally accurate and demonstrating the potential of machine learning models for predicting the density of intermetallic compounds.
Figure 3. Performance comparison of machine learning models for density prediction across crystal structures. (A) Hexagonal; (B) Cubic; (C) Monoclinic; (D) Orthorhombic; (E) Trigonal; (F) Tetragonal. Bar charts compare the predictive accuracy of different machine learning models for each crystal structure, while the scatter plots show the relationship between calculated values by DFT and predictions from the best-performing model. DFT: Density functional theory.
Machine learning modeling on aggregated crystal structures
In the above section, machine learning models are built based on data subsets for specific crystal structures, achieving high prediction accuracy. However, this single crystal structure-based modeling approach has limitations in practical applications, especially when studies require density prediction on mixed data of multiple crystal structures. In this section, an approach without distinguishing crystal structures is adopted, integrating the data from all seven crystal structures for unified modeling to explore the performance of traditional machine learning models on this complex dataset. The model results are shown in Figure 4A and B. Among the traditional machine learning models, the accuracy of simple models such as LR or KNN remained almost the same or slightly increased, while the prediction accuracy of complex models such as RF or XGBoost decreased to varying degrees. For example, the accuracy of the XGBoost model that shows optimal performance in the above section decreased from 0.98 to 0.9468. Figure 4C-E shows scatter plots of the predictions from the LR, SVM, and XGBoost models. From these images, it can be seen that the prediction results of the LR and SVM models are poor, with some points deviating far from the diagonal line. The XGBoost model has higher accuracy than the other two, but a few data points deviate significantly from the diagonal, reducing prediction accuracy. A review of these compounds revealed that they all have polymorphic forms in the dataset. For instance, the test set includes the compound In3Hg with an “Orthorhombic” structure and an experimental density of 1.4236 g/cm3. However, in the training set, In3Hg is classified under a “Hexagonal” structure with a significantly higher experimental density of 8.6732 g/cm3. This discrepancy likely contributes to the XGBoost model inaccurately predicting the density of In3Hg as 7.5879 g/cm3 in the test set. The compound PuPt3, which exhibits a “Tetragonal” structure and a density of 18.6427 g/cm3 in the training set, appears with a “Cubic” structure and a density of 19.9649 g/cm3 in the test set. The XGBoost prediction is 17.1343 g/cm3, aligning more closely with the training set density.
Figure 4. Performance comparison of different machine learning models and the IGNN model on the test dataset. (A and B) Comparison of model performance, illustrating the R2 and RMSE values of different models; (C-F) Scatter plots of predicted values vs. calculated values by DFT. Dots of different colors represent various crystal structure types, with the gray diagonal line indicating perfect agreement between predicted values and calculated values by DFT. IGNN: Intermetallics graph neural network; R2: the coefficient of determination; RMSE: root mean square error; DFT: density functional theory.
The above analysis shows that intermetallic density data for different crystal structures vary significantly. Directly combining all structures’ data for modeling causes traditional models to struggle in recognizing differences between crystal structures, which in turn affects prediction performance. In this context, The study introduces an IGNN model that is better suited for multi-crystal structure data, addressing the limitations of traditional models when applied to mixed crystal structure datasets. As illustrated in Figure 4A and B, the constructed IGNN model clearly outperforms traditional machine learning models, achieving the highest R2 value of 0.9884 and the lowest RMSE of 0.4291 g/cm3. Additionally, the scatter plot in Figure 4F shows that the IGNN model can utilize node information in the graph structure to automatically recognize differences between crystal structures and incorporate this information as input for modeling, enhancing its adaptability to multi-structure datasets. The prediction error for the compound
To further assess the generalization capability of the IGNN model in handling the density of complex intermetallic compounds, an independent density test set for ternary and quaternary compounds is applied. The extended test set is more complex because the structures of ternary and quaternary compounds involve more types of atoms and more complex crystal structures, providing a more comprehensive examination of the model’s robustness across different compound types and structures. Figure 5A shows the performance of IGNN on the ternary compound test set, with an R2 value of 0.9852 and an RMSE of 0.3313 g/cm3, indicating a high prediction accuracy for the ternary system. The IGNN prediction results on the quaternary compound test set are shown in Figure 5B, with an R2 of 0.9694 and an RMSE of 0.3340 g/cm3. Compared to ternary compounds, the prediction accuracy for quaternary compounds is slightly lower, likely due to the higher structural complexity of quaternary compounds. From the scatter plot distribution, the IGNN model’s prediction results on the ternary and quaternary compound test sets are highly consistent with calculated values by DFT, with most data points concentrated near the ideal diagonal, indicating minimal deviation between predicted values and calculated values by DFT. The data points for each crystal structure are distributed relatively evenly, without significant deviation for any particular crystal structure. This suggests that the IGNN model demonstrates strong stability and generalization ability when handling diverse crystal structures.
Figure 5. IGNN model performance on density prediction for ternary and quaternary intermetallic compounds. (A) Prediction results on ternary compounds; (B) Prediction results on quaternary compounds (Dots of different colors represent various crystal structure types). IGNN: Intermetallics graph neural network.
Classification prediction and visualization
In studying the crystal structure and density prediction of intermetallic compounds, it is necessary not only to perform accurate regression predictions but also to conduct density classification analysis to explore the influence of different crystal structures on model classification. To gain a better understanding of the IGNN model’s performance in classification tasks, t-SNE is employed to visualize classification results across different layers of the IGNN model[38]. Additionally, a comparative analysis of the classification performance of IGNN and various traditional machine learning models is conducted.
t-SNE is a widely used dimensionality reduction technique that projects high-dimensional data into a two-dimensional space, facilitating visual assessments of a model’s ability to differentiate between categories. In this study, t-SNE is employed to visualize the feature representations at each layer of the IGNN model [Figure 6]. Figure 6A presents the t-SNE visualization of the input layer. In the input layer, data points from various crystal systems are not yet clearly separated, suggesting that the model has not deeply separated data features at this initial stage. The t-SNE visualization of the normalization layer is shown in Figure 6B. Following normalization, the data distribution becomes more concentrated, with emerging boundaries between different crystal systems, suggesting initial feature refinement. The t-SNE visualization of the global pooling layer is shown in Figure 6C. The data points from different crystal systems demonstrate a clear clustering tendency, reflecting the model’s capacity to aggregate node features and capture significant structural differences among the crystal systems. Figure 6D displays the t-SNE visualization of the output layer, where data points from different crystal systems are distinctly separated, indicating a strong classification outcome. The features extracted at the output layer of the IGNN model possess high discriminative power, creating clear boundaries between data from different crystal structures in two-dimensional space. Through the t-SNE visualization analysis of each layer in the IGNN model, a progressive enhancement in the model’s ability to distinguish among different crystal structures is observed as the layers deepen. This systematic feature extraction and aggregation approach provides IGNN with a distinct advantage in complex density classification tasks.
Figure 6. t-SNE visualization of density classification predictions at different IGNN model layers. (A) Input layer; (B) Normalization layer; (C) Global pooling layer; (D) Output layer. Each subplot shows the output from a different layer, with data points in two-dimensional space colored by a crystal system. t-SNE: t-Distributed stochastic neighbor embedding; IGNN: intermetallics graph neural network.
To further verify the performance of the IGNN model in classification tasks, this study compares it with several traditional machine learning models, including LR, RF, KNN, XGBoost, and SVM. Figure 7A presents the performance comparison of these models, covering evaluation metrics such as Precision, Recall, F1 score, and micro-average area under the curve (AUC_micro). Compared to these traditional models, IGNN shows superior performance in all evaluation metrics, reaching high levels in area under the curve (AUC), Precision, and F1 scores. To more intuitively display the performance of each model in classification tasks, Figure 7B-E shows the multi-class ROC curves for the SVM, KNN, XGBoost, and IGNN models. The ROC curve reflects the model’s classification ability, with a larger AUC indicating stronger discrimination power. In Figure 7B, SVM performs decently in some categories. Due to its rigid classification boundaries, it lacks flexibility in multi-crystal structure classification, leading to a lower average AUC value and difficulty in competing with IGNN. The KNN model shows a relatively low average AUC score in multi-class classification, with a flatter curve, as seen in Figure 7C. Particularly in different crystal structure categories with close densities, KNN struggles to make accurate distinctions, demonstrating certain limitations. As depicted in Figure 7D, the XGBoost model shows higher AUC values in some categories, though its overall average AUC score remains below that of IGNN. As shown in Figure 7E, the ROC curves for each category in IGNN exhibit high AUC values, demonstrating its excellent classification ability. IGNN captures subtle differences among crystal structures using its graph structure, achieving superior performance in multi-class classification tasks.
Figure 7. Performance comparison of machine learning models and IGNN model in classification tasks. (A) Comparison of performance metrics for different models in classification tasks, including Precision, Recall, F1 Score, and micro-average AUC; (B-E) Multi-class ROC curves for different models, with larger AUC values indicating stronger classification ability. (B) SVM; (C) KNN; (D) XGBoost; (E) IGNN. IGNN: Intermetallics graph neural network; AUC: Area under the curve; ROC: receiver operating characteristic; SVM: support vector machine; KNN: K-nearest neighbors; XGBoost: EXtreme gradient boosting.
From the above results, it can be concluded that the IGNN model captures complex relationships between atoms in crystal structures via graph-level representations, inherently understanding spatial and bonding configurations. Especially, for polymorphic intermetallic compounds, IGNN incorporates relevant features from the graph structure, and thus reduces the need for extensive feature engineering, minimizes potential biases, thereby enhancing the model generalization capabilities. Compared to traditional models (e.g., SVM, KNN, XGBoost, etc.) that rely on fixed feature vectors, IGNN demonstrates significant advantages in structural awareness, feature interactions, generalization ability, and flexibility. It captures complex dependencies and nonlinear relationships, and exhibits superior performance across different crystal structures and compositions.
CONCLUSIONS
For the density regression and classification tasks of binary intermetallic compounds, several machine learning models are constructed to evaluate their performance on datasets with various crystal structures. The results indicate that traditional machine learning models perform well on datasets with single crystal structures, but exhibit decreased effectiveness on mixed datasets containing multiple crystal structures, failing to effectively capture the complex relationships among these structures. To address this limitation, the IGNN model, based on GNNs, is introduced and constructed. Through graph structure learning, the IGNN achieves higher prediction accuracy on mixed datasets with multiple crystal structures, demonstrating adaptability and superiority in modeling the complex density of intermetallic compounds. Overall, this study contributes by proposing a GNN method that is suitable for multi-crystal structure data modeling, providing a novel computational framework for density prediction in intermetallic compounds. This research offers significant advancements in the predictive modeling of intermetallic compounds, facilitating more efficient material design and contributing to the development of high-performance materials in various industrial applications.
DECLARATIONS
Authors’ contributions
Writing - original draft, software, methodology, formal analysis, data curation: Zhu, D.; Nie, M.; Shang, C.
Writing - review and editing, supervision, project administration, investigation: Wu, H. H.; Gao, J.;
Validation, investigation, data collection: Zhu, J.; Zhu, Y.; Wang, S.
Visualization, resources, formal analysis, conceptualization: Zhou, X.; Wang, F.; Wang, B.
Supervision, conceptualization, revision, and finalization of the manuscript: Zhang, C.; Mao, X.
Availability of data and materials
The data that support the findings of this study are available from the corresponding author upon reasonable request.
Financial support and sponsorship
The present work is supported by the National Natural Science Foundation of China (Nos. 52122408 and 52071023). Wu, H. H. also thanks the financial support from the Fundamental Research Funds for the Central Universities (University of Science and Technology Beijing, Nos. FRF-TP-2021-04C1 and 06500135). The computing work is supported by USTB MatCom of Beijing Advanced Innovation Center for Materials Genome Engineering.
Conflicts of interest
Wu, H. H. serves as a Junior Editorial Board Member of Journal of Materials Informatics. However, Wu, H. H. was not involved in any aspect of the editorial process for this manuscript, including reviewer selection, manuscript handling, or decision-making. The remaining authors declare that they have no conflicts of interest.
Ethical approval and consent to participate
Not applicable.
Consent for publication
Not applicable.
Copyright
© The Author(s) 2025.
REFERENCES
2. Taub, A. I.; Fleischer, R. L. Intermetallic compounds for high-temperature structural use. Science 1989, 243, 616-21.
3. Dshemuchadse, J.; Steurer, W. Some statistics on intermetallic compounds. Inorg. Chem. 2015, 54, 1120-8.
4. Yamaguchi, M.; Inui, H.; Ito, K. High-temperature structural intermetallics. Acta. Mater. 2000, 48, 307-22.
5. Kimura, Y.; Pope, D. P. Ductility and toughness in intermetallics. Intermetallics 1998, 6, 567-71.
6. Zhu, D.; Pan, K.; Wu, H.; et al. Identifying intrinsic factors for ductile-to-brittle transition temperatures in Fe–Al intermetallics via machine learning. J. Mater. Res. Technol. 2023, 26, 8836-45.
7. Ravindran, P.; Asokamani, R. Correlation between electronic structure, mechanical properties and phase stability in intermetallic compounds. Bull. Mater. Sci. 1997, 20, 613-22.
8. Kim, S. H.; Kim, H.; Kim, N. J. Brittle intermetallic compound makes ultrastrong low-density steel with large ductility. Nature 2015, 518, 77-9.
10. Fleischer, R. L. High-strength, high-temperature intermetallic compounds. J. Mater. Sci. 1987, 22, 2281-8.
11. Stoloff, N.; Liu, C.; Deevi, S. Emerging applications of intermetallics. Intermetallics 2000, 8, 1313-20.
12. Uenishi, K.; Kobayashi, K. Processing of intermetallic compounds for structural applications at high temperature. Intermetallics 1996, 4, S95-101.
13. Paul, A. R.; Mukherjee, M.; Singh, D. A critical review on the properties of intermetallic compounds and their application in the modern manufacturing. Cryst. Res. Technol. 2022, 57, 2100159.
14. Fatima, B.; Chouhan, S. S.; Acharya, N.; Sanyal, S. P. Density functional study of XRh (X=Sc, Y, Ti and Zr) intermetallic compounds. Comput. Mater. Sci. 2014, 89, 205-15.
15. Lu, Z. W.; Wei, S.; Zunger, A.; Frota-Pessoa, S.; Ferreira, L. G. First-principles statistical mechanics of structural stability of intermetallic compounds. Phys. Rev. B. Condens. Matter. 1991, 44, 512-44.
16. Zhu, D.; Pan, K.; Wu, Y.; et al. Improved material descriptors for bulk modulus in intermetallic compounds via machine learning. Rare. Met. 2023, 42, 2396-405.
17. Oliynyk, A. O.; Mar, A. Discovery of intermetallic compounds from traditional to machine-learning approaches. Acc. Chem. Res. 2018, 51, 59-68.
18. Medasani, B.; Gamst, A.; Ding, H.; et al. Predicting defect behavior in B2 intermetallics by merging ab initio modeling and machine learning. npj. Comput. Mater. 2016, 2, 1.
19. Nie, M.; Chen, D.; Wang, D. Reinforcement learning on graphs: a survey. IEEE. Trans. Emerg. Top. Comput. Intell. 2023, 7, 1065-82.
20. Xie, T.; Grossman, J. C. Crystal graph convolutional neural networks for an accurate and interpretable prediction of material properties. Phys. Rev. Lett. 2018, 120, 145301.
21. Park, C. W.; Wolverton, C. Developing an improved crystal graph convolutional neural network framework for accelerated materials discovery. Phys. Rev. Mater. 2020, 4, 063801.
22. Reiser, P.; Neubert, M.; Eberhard, A.; et al. Graph neural networks for materials science and chemistry. Commun. Mater. 2022, 3, 93.
23. Dreger, M.; Eslamibidgoli, M. J.; Eikerling, M. H.; Malek, K. Synergizing ontologies and graph databases for highly flexible materials-to-device workflow representations. J. Mater. Inf. 2023, 3, 2.
24. Choudhary, K.; Decost, B. Atomistic line graph neural network for improved materials property predictions. npj. Comput. Mater. 2021, 7, 650.
25. Wu, X.; Wang, H.; Gong, Y.; et al. Graph neural networks for molecular and materials representation. J. Mater. Inf. 2023, 3, 12.
26. Fung, V.; Zhang, J.; Juarez, E.; Sumpter, B. G. Benchmarking graph neural networks for materials chemistry. npj. Comput. Mater. 2021, 7, 554.
27. Dai, M.; Demirel, M. F.; Liang, Y.; Hu, J. Graph neural networks for an accurate and interpretable prediction of the properties of polycrystalline materials. npj. Comput. Mater. 2021, 7, 574.
28. Jain, A.; Ong, S. P.; Hautier, G.; et al. Commentary: the materials project: a materials genome approach to accelerating materials innovation. APL. Materials. 2013, 1, 011002.
29. Chicco, D.; Warrens, M. J.; Jurman, G. The coefficient of determination R-squared is more informative than SMAPE, MAE, MAPE, MSE and RMSE in regression analysis evaluation. PeerJ. Comput. Sci. 2021, 7, e623.
30. Chai, T.; Draxler, R. R. Root mean square error (RMSE) or mean absolute error (MAE)? - Arguments against avoiding RMSE in the literature. Geosci. Model. Dev. 2014, 7, 1247-50.
31. Zhu, D.; Wu, H.; Hou, F.; et al. A transfer learning strategy for tensile strength prediction in austenitic stainless steel across temperatures. Scr. Mater. 2024, 251, 116210.
32. Bradley, A. P. The use of the area under the ROC curve in the evaluation of machine learning algorithms. Pattern. Recognit. 1997, 30, 1145-59.
33. Yoshida Laboratory. XenonPy. 2020. https://github.com/yoshida-lab/XenonPy. (accessed 2025-02-05).
34. Fessler, J.; Sutton, B. Nonuniform fast fourier transforms using min-max interpolation. IEEE. Trans. Signal. Process. 2003, 51, 560-74.
35. Isayev, O.; Oses, C.; Toher, C.; Gossett, E.; Curtarolo, S.; Tropsha, A. Universal fragment descriptors for predicting properties of inorganic crystals. Nat. Commun. 2017, 8, 15679.
36. Ioffe, S.; Szegedy, C. Batch normalization: accelerating deep network training by reducing internal covariate shift. arXiv2015, arXiv:1502.03167. Available online: https://doi.org/10.48550/arXiv.1502.03167. (accessed 5 Feb 2025)
37. Du, J.; Zhang, S.; Wu, G.; Moura, J. M. F.; Kar, S. Topology adaptive graph convolutional networks. arXiv2017, arXiv:1710.10370. Available online: https://doi.org/10.48550/arXiv.1710.10370. (accessed 5 Feb 2025)
Cite This Article
How to Cite
Zhu, D.; Nie, M.; Wu, H. H.; Shang, C.; Zhu, J.; Zhou, X.; Zhu, Y.; Wang, F.; Wang, B.; Wang, S.; Gao, J.; Zhao, H.; Zhang, C.; Mao, X. An optimized strategy for density prediction of intermetallics across varied crystal structures via graph neural network. J. Mater. Inf. 2025, 5, 8. http://dx.doi.org/10.20517/jmi.2024.76
Download Citation
Export Citation File:
Type of Import
Tips on Downloading Citation
Citation Manager File Format
Type of Import
Direct Import: When the Direct Import option is selected (the default state), a dialogue box will give you the option to Save or Open the downloaded citation data. Choosing Open will either launch your citation manager or give you a choice of applications with which to use the metadata. The Save option saves the file locally for later use.
Indirect Import: When the Indirect Import option is selected, the metadata is displayed and may be copied and pasted as needed.
About This Article
Special Issue
Copyright
Data & Comments
Data
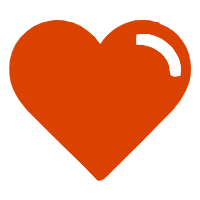
Comments
Comments must be written in English. Spam, offensive content, impersonation, and private information will not be permitted. If any comment is reported and identified as inappropriate content by OAE staff, the comment will be removed without notice. If you have any queries or need any help, please contact us at [email protected].