Improved hardness prediction for reduced-activation high-entropy alloys by incorporating symbolic regression and domain adaptation on small datasets
Abstract
The reduced-activation high-entropy alloys (RAHEAs) have promising applications in advanced nuclear systems due to their low activation, excellent mechanical properties and radiation resistance. However, compared to the conventional high-entropy alloys (HEAs), the relatively small datasets of RAHEAs pose challenges for alloy design by using conventional machine learning (ML) methods. In this work, we proposed a framework by incorporating symbolic regression (SR) and domain adaptation to improve the accuracy of property prediction based on the small datasets of RAHEAs. The conventional HEA datasets and RAHEA datasets were classified as source and target domains, respectively. SR was used to generate features from element-based features in the source domains. The domain-invariant features related to hardness were captured and used to construct the ML model, which significantly improved the prediction accuracy for both HEAs and RAHEAs. The normalized root mean square error decreases by 24% for HEAs and 30% for RAHEAs compared to that of the models trained with element-based features. The proposed framework can achieve accurate and robust prediction on small datasets with interpretable domain-invariant features. This research paves the way for efficient material design under small dataset scenarios.
Keywords
INTRODUCTION
High-entropy alloys (HEAs)[1] are a new series of metallic materials of excellent mechanical[2,3], high temperature[4], and irradiation properties[5], making them promising candidate structural materials for nuclear applications. Unlike conventional alloys, HEAs consist of multi-principal components, with compositions ranging from 5% to 35% by atomic fraction, which introduced their unique core effects[6]. HEAs offer a broad compositional space for design, which makes the trial-and-error method almost impossible. Recently, machine learning (ML) has been proven to be an effective approach for structure and property prediction[7-12], thereby accelerating HEA design. The current HEA datasets usually contain hundreds of samples[13,14], with high-dimension features[8,9,15]. These characteristics lead to sparse and imbalanced data distribution, ultimately affecting the model performance. Besides, typical HEAs contain neutron activation elements, such as Co and Nb, which must be strictly controlled for nuclear fusion applications[16]. Reduced-activation HEAs (RAHEAs)[17-19] were developed with these element constraints. Furthermore, compositional differences and limited research have resulted in small datasets, posing a challenge on RAHEA design.
The challenge can be addressed from data, model and algorithmic perspectives[20], which requires prior knowledge for learning or searching the best model. Several strategies have been employed to tackle the problem in materials science, such as transfer learning (TL), which is one of the mainstream methods[21]. TL acquires prior knowledge from a source domain and transfers the knowledge into the target domain (i.e., the small dataset) for the improvement of ML model performance[22]. A TL problem can be solved from instance-, feature-, parameter-, and relation-based methods[23]. Recent applications of TL in materials science[24-26] have shown the potential of TL to achieve good accuracy under small data schemes. When employing TL on small datasets, the dataset shift[27] between the source and the target domain needs to be carefully considered. Li et al. reported that models trained on Materials Project 2018 would fail to predict novel materials on Materials Projects 2021 due to the dataset shift[28]. In the field of computer science, a series of works were conducted to solve the dataset shifts through domain generalization[29] or adaptation[30-32]. However, there are only few applications of domain adaptation in materials science. Goetz et al. utilized unsupervised domain adaptation with an adversarial network for microstructure image classification[33]. Hu et al. conducted a comprehensive benchmark of the performance enhancement of ML models augmented by various domain adaptation methods, illustrating the potential of domain adaptation, as well as the demand of new methods to capture the invariant relationships avoiding dataset shift across domains[34].
A critical issue in feature-based domain adaptation is identifying domain-invariant features, ensuring good generalization ability of the model[35,36]. Features adopted for material property prediction in previous studies are usually simple statistical combinations of composition and corresponding element properties, capturing part of the feature-property relationship. Therefore, several typical feature-based domain methods[37-39] may be inappropriate, as they require finding a shared feature subspace among existing features. In this context, exploring complex nonlinear relationships through feature augmentation and transformation becomes crucial for capturing the stochastic influencing factors of material properties. Genetic programming (GP)[40], as a powerful tool for feature extraction, has gained attention in recent years for its ability to generate nonlinear feature combinations with numerical operators, enabling the discovery of interpretable and highly correlated features from limited data, enhancing predictive accuracy and intensifying the understanding of material behavior[41-43]. In the field of materials science, Lee et al. proposed a synergistic combination between conventional ML model and GP-based symbolic regression (SR) model with physical-based insights, successfully establishing a robust and reliable framework for out-of-distribution prediction of yield strength[44]. Feng et al. employed SR to discover new features for the fatigue life of T91 and 316SS steel, demonstrating a strong correlation with fatigue life and improved performance of ML algorithms[45].
Motivated by these considerations, we propose a framework that integrates SR and domain adaptation to improve the accuracy of material property predictions on small datasets, specifically targeting RAHEAs. Experimental data on conventional HEAs and RAHEAs were collected into two distinct datasets. The HEA dataset served as a source domain, while the RAHEA dataset was treated as a target domain. Element-based features were generated and selected by the framework to create the initial feature set for SR exploration. These generated features were evaluated to identify the domain-invariant features for adaptation. Finally, ML models were constructed based on various feature sets to assess and compare the performance enhancement of the models, illustrating the effectiveness of domain adaptation. The proposed framework can provide a consistent and effective strategy for improving the accuracy of property predictions on small datasets.
MATERIALS AND METHODS
Introduction to the framework
Figure 1 illustrates the workflow of the proposed framework. In the first step, we labeled the HEA and RAHEA datasets as source domain S and target domain T, respectively. Due to the insufficient number of samples in the target domain, virtual samples were generated near the compositional space for each RAHEA, as shown in Table 1, to augment the dataset for the discriminator. In the second step, element-based features were generated and selected, to establish an initial feature set for SR exploration and selection. In the third step, the feature generators based on SR were employed to explore feature combinations within the initial feature set to generate new features. In the fourth step, the generated features were screened by the discriminator to obtain domain-invariant features for adaptation. Finally, the enhancement of prediction accuracy from the invariant features was examined using a ML prediction model trained on the HEA dataset.
Compositions (in atom fraction) and hardness of 9 RAHEAs
Experimental data collection and preprocessing
Data collection and augmentation
The raw data for this study, including the hardness values and corresponding compositions of HEAs and RAHEAs, were collected from published literature[9,12,14,18,19,46-50]. The dataset encompasses eight transition metal elements (Co, Cr, Cu, Fe, Ni, Mn, Ti, and V), six refractory metal elements (Hf, Mo, Nb, Ta, W, and Zr), and two additional elements (Al and Sn). Data cleaning was applied to remove any abnormal values and redundant samples. Considering the impact of the preparation process, only the hardness data for as-cast alloys were adopted. The final dataset comprised 460 as-cast HEAs and nine RAHEAs, with the details of the nine RAHEAs listed in Table 1.
To mitigate the impact of the imbalanced data size between the source and target domains for the discriminator, virtual samples were generated near the composition of each RAHEA with an atom fraction interval of 0.05. For each real RAHEA, 50 virtual RAHEAs were generated, resulting in an augmented target dataset that contains 459 samples (nine real samples and 450 virtual samples without hardness).
Generation of element-based features
The generation of features derived from elemental properties is emphasized as a crucial step to facilitate subsequent feature selection and exploration. As listed in Supplementary Table 1, 22 types of elemental properties that may affect the hardness were extracted from the relevant database[51]. These include the atomic properties (Z, Ra, Rc, VEC, χp, χar, Fw, I1), the thermodynamic properties (Tm, Hfus, Hvap, Hatm, S0, Cp, K, Lt) and elastic properties (Cij, B, E, G, v). There are 16 elements considered for the compositions of HEAs and RAHEAs in both datasets.
Features were generated based on the elemental properties and corresponding concentrations, using four expressions outlined below: the mean value (MX), the variance value (VX), the deviation value (FX) and the local mismatch value (DX) of related elemental properties. Besides, Three empirical descriptors were also collected in this study, which may relate to the hardness of HEAs according to the literature, including the enthalpy of mixing (Hmix)[52], the entropy of mixing (Smix)[52], and six powers of the work function (w6)[53]. The equations for numerical expression and empirical descriptors are provided in the Supplementary Materials.
Feature preprocessing and selection
A comprehensive approach for generating and selecting these features was proposed in this section, aimed at developing an effective initial feature set for subsequent SR exploration. Firstly, all features were normalized using a Z-score scaler, which is robust against outliers. Secondly, the Pearson correlation coefficient (PCC) was implemented to measure the correlation between a feature and hardness or another feature, as given in
A higher absolute value of the PCC usually indicates a stronger linear correlation, which can help select hardness-related features and drop redundant ones.
The original features generated in Section “Generation of element-based features” were selected using a three-step approach that included correlation and variance threshold selection. In the first step, correlation between features and hardness was evaluated by PCC. Those features with low correlation to the target performance (|PCC| < 0.4) were removed, as they are unlikely to provide valuable information to the model. In the second step, the variance of the remaining features from the first step was calculated and sorted in descending order. The bottom 15% of the features based on variance were eliminated, while the most informative features with sufficient variability were selected. In the third step, the correlation among selected features was checked. Features with high correlation coefficients (|PCC| > 0.85) to other features were removed to improve the model performance.
Feature-based domain adaptation using SR
Feature generation
To reveal the explicit mathematical relationship between element properties and hardness, and discover the domain-invariant features between the source and target domain, SR was employed with iterative search manner to reduce the randomness from the algorithm[41,54]. Figure 2A illustrates the flowchart of the SR, where the genetic algorithm controls the combination of features through algebraic operators to form the initial population. Each individual in the population corresponds to an expression. During the genetic algorithm search, the performance of each expression is evaluated based on a fitness function, allowing for the selection of the best expressions from each generation. These expressions undergo crossover and mutation to enhance diversity, generating new individuals (expressions) for the next generation, as shown in Figure 2B. The purpose of crossover and mutation is to prevent optimized expressions from becoming trapped in local optima. When the optimization meets the termination criteria, SR stops and outputs the optimal expression.
Figure 2. Schematic illustration of SR-based domain adaptation. (A) SR algorithm; (B) Feature selection and adaptation. SR: Symbolic regression.
The types of operators used in SR, along with parameters such as mutation rate, crossover rate, and population size, are crucial for its effectiveness. As shown in Supplementary Table 2, the population size is set to 500 to ensure enough features for evaluation, while generations were limited to 50 to control runtime. A Hall of Fame retained the 50 best features, preserving excellent solutions, and ten features were selected from the generator to focus on the most promising options. The function set employed various mathematical operators (add, substrate, multiply, divide, inverse, logarithmic, exponential, maximum, minimum, square root) to construct expressions. The crossover probability determined the frequency of feature combinations, while the subtree mutation probability and hoist mutation probability ensured population diversity. The point mutation probability guarantees that the total mutation probability sums to 1. Additionally, the depth of expression trees was limited to 2 to 5, balancing complexity and interpretability. The parsimony coefficient was set to 0.001, promoting simpler, more generalizable models. This configuration optimizes the efficiency and effectiveness of the SR process.
Domain-invariant feature screening
A two-step method was developed to screen for the domain-invariant features. In the first step, the optimal SR feature generator was selected based on loss minimization across iteratively running generators. Each generator produced ten new feature expressions for further selection and exploration. The procedure involves building a regression model using the features provided by each generator to predict the target property (hardness) and calculate the loss for generator selection. In the second step, we utilized the feature expressions from the best generator to create new features in both the source and target domains. Each new feature was labeled “S” or “T”, indicating whether the sample originates from the source or target domain. These features are then evaluated using a classifier that serves as a discriminator[30]. The features with the lowest classification accuracy are identified as domain-invariant features.
ML based-hardness prediction models
ML algorithms
Several ML algorithms were employed to evaluate the performance based on the selected features. These algorithms included decision tree regression (DTR), extremely randomized tree regression (ETR), gradient boosting regression (GBR), K-nearest neighbor regression (KNR), random forest regression (RFR), ridge regression (Ridge), and support vector regression (SVR). This comprehensive evaluation allowed us to identify the most effective algorithm for the HEA dataset. To construct the ML prediction model, GridSearchCV[55] was utilized to streamline the hyperparameter tuning process with ten-fold cross-validation, which can explore all possible hyperparameter combinations and help reduce the risk of overfitting.
Evaluation of ML models
The performance of each algorithm was assessed using multiple metrics, specifically the coefficient of determination (R2), normalized root mean squared error (NRMSE), and mean relative error (MRE). The best model for each algorithm was determined based on a comprehensive assessment of the R2, NRMSE, and MRE metrics, using
where n,
Shapley additive explanations
Shapley additive explanations (SHAP)[56] were employed to interpret ML models and evaluate the contributions of features for each sample, as expressed by
where F refers to the set of all features adopted in the model, G indicates a feature subset of F, and nF and nG are the numbers of features in the two sets, respectively. The SHAP value can be either negative or positive, indicating different contributions to the predicted property. The mean absolute SHAP values and the corresponding sampled SHAP values for each feature were employed to assess the mean contribution of each specified feature.
RESULTS AND DISCUSSION
Feature-based domain adaptation for small dataset
Discovery of domain-invariant features with the framework
Both source and target datasets consisted of 88 original features based on the combinations of elemental properties and compositions, and three additional empirical descriptors. The source dataset was split into training and testing sets using the holdout method, while with the testing set comprising 10% of the source dataset. Correlation and variance threshold methods were applied on the training set to eliminate redundant features, as described in Section “Feature preprocessing and selection”. After applying these methods, 13 features remained in the training set were used to search for SR-generated domain-invariant features (Noted as GPFs). Figure 3 shows the correlation heatmap among initial features and GPFs. The map shows that most of the initial features are not or only slightly correlated with each other, while three GPFs are highly correlated.
Figure 3. Correlation heatmap among features from initial feature sets and GPF1-3, with blue indicating negative correlations, red indicating positive correlations, and deeper colors representing stronger correlations.
As shown in Supplementary Table 2, three operator sets were used to explore domain-invariant features using the proposed framework. The three domain-invariant features generated and screened by these operator sets were designated as GPF1, GPF2, and GPF3, respectively. A Ridge was chosen to evaluate the NRMSE loss for the optimal selection of SR generators. A 10-fold cross-validation method was applied on the training set for SR feature generation. For each fold in training, ten SR generators were initialized to mitigate the impact of randomness. NRMSE metrics were calculated on both training and testing sets to assess the overfitting trend of the model. The final generator was chosen based on error minimization without overfitting, which ensures the ten features created by the generator have good generalization ability on unseen data. As shown in Supplementary Figure 1, three SR feature generators were selected with test NRMSE metrics of 0.197, 0.205 and 0.160, respectively. GPF1, GPF2 and GPF3 were extracted separately from these best SR feature generators. A logistic regression classifier was implemented as the discriminator for feature extraction, and the accuracy was calculated as the ratio of correct classifications to total classifications:
Figure 4 illustrates the correlation between the initial features, GPFs, and target performance, as well as the similarity in feature distribution between the source and target domains. The maximum mean discrepancy (MMD)[61] was employed to measure the similarity between the two distributions. Figure 4C analyzes the distribution similarity across domains based on MMD for the initial features. A low MMD value usually indicates good similarity in feature distribution across domains. Features with smaller MMD values, such as Dc12, Mc12 and VE, exhibit smaller changes in PCC according to Figure 4A and B. This suggests that these features are more effective in capturing the underlying feature-hardness relationship, rather than being affected by specific data distributions. In contrast, features with large MMD values, such as FI1 and Dρ, exhibit significant changes in PCC regarding hardness between Figure 4A and B, shifting from positive to negative correlations. This indicates that these features may have been selected using conventional feature screening methods based on specific data distributions. Figure 4D-F displays the generated GPFs. We observed that the MMD values of the three GPFs are significantly lower than those of the initial features, indicating good distribution consistency across domains. The PCC change of three GPFs from the source domain to the target domain are 0.02, 0.28, and 0.10, respectively. Considering both the PCC change and MMD, GPF1 seems to be closer to the “ideal” domain-invariant feature, that is, maintaining a high target performance correlation under different data distributions.
Figure 4. Analysis of the relationship between features and target performance in the source and target domains. (A) and (B) display the PCC between features and target performance across the source and target domains; (C) illustrates the MMD of features in both domains; (D-F) present scatter plots of the generated GPF1, GPF2, and GPF3 with standardization against target performance, along with their respective PCC and MMD values. PCC: Pearson correlation coefficient; MMD: maximum mean discrepancy.
Explanation of the domain-invariant features
Based on the mathematical expressions of the three GPFs listed in Table 2, we can identify their similarities and differences, which aids in understanding the physical insights of the domain-invariant features. The same components utilized in three GPFs include DRa and Mv, which reflect the local mismatch of atomic radius, mean Poisson’s ratio, reflecting the contribution from lattice distortion and shear response. The local mismatch of atomic radius DRa highlights the lattice distortion effect in HEAs, recognized as a core factor and a significant contributor to hardness due to solid solution strengthening[6,10]. HEAs and RAHEAs that exhibit a higher shear response during Vickers hardness testing tend to demonstrate greater hardness, as indicated by Mc12 in both GPF1 and GPF2.
Mathematical expressions of screened domain-invariant features
Feature | Expression |
GPF1 | 2DRa + Mv + Mc12 + DI1 + VHfus + max[(DRa + DI1), Dχar, Fρ] |
GPF2 | 2DRa + Mv + Mc12 + DI1 + VHfus + Fρ |
GPF3 |
The primary distinction between GPF1 and GPF2 lies in the incorporation of a maximum operator, which results in significant deviations in the cross-domain PCC between the two GPFs. The local mismatch of electronegativity Dχar was proposed by Wen et al., as a hardness descriptor from the perspective of the solid solution strengthening[10]. However, Dχar does not effectively describe the solid solution strengthening in FCC HEAs due to its low sensitivity. By employing a maximum operator, GPF1 captures the contributions of Dχar while mitigates its limitations in FCC HEAs. Additionally, a high local mismatch in density may indicate the incorporation of lightweight elements such as Al and Ti, which are associated with phase transformations in HEAs and contribute to hardness through the solid solution strengthening[62,63]. The high ionization energy mismatch DI1 shows the mixing between elements with high ionization energy (Al, V, Zr, Nb, Cr) and low ionization energy (Fe, Co, W, Cu), reflecting the alloying effect. The fusion enthalpy Hfus is related to the crystal structure and bond energy, the high variance of Hfus reflects the alloying of refractory elements and transition metal elements.
In summary, several influencing factors can be identified from the screened domain-invariant features: (1) Differences in crystal structure, reflected by Fρ, VHfus and DI1; (2) Bond strength, indicated by VHfus and DI1; (3) Solid solution strengthening due to DRa; (4) Additional solid solution strengthening factors Dχar along with features indicating strong alloying effects. The domain-invariant features screened in this study exhibit a comprehensive view of hardness contributors by nonlinear mathematical combinations. The influence of these domain-invariant features on performance of ML models is also a key issue for further discussion.
Construction of hardness prediction models
Based on generated domain-invariant features, we investigated three distinct feature sets: models that rely solely on initial features (NoGPF), models that utilize only GPFs (referred to as GPFOnly), and models that incorporate both feature sets (GPFCombined). This comparison aimed to evaluate the impact of GPFs on the performance of ML models across both the source and target domains.
All three sets of feature sets in the source domain were used for data partitioning using the holdout method, reserving 10% of the samples for model testing, and the remaining 90% of the samples were used using ten-fold cross-validation combined with hyperparameter optimization to evaluate the performance of all seven ML algorithms presented in Section “ML based-hardness prediction models” for constructing hardness prediction models. The hyperparameters used by each algorithm to build the model were searched using GridSearchCV[55], and the range of each parameter search is listed in Supplementary Table 3. During the algorithm evaluation process, we cycled through ten holdouts and recorded the performance of each algorithm in building the model to calculate the average performance for robust evaluation.
The box plots were used to clearly illustrate the central tendencies and variabilities of the performance of each ML algorithm. Each box in the plot represents the interquartile range, encompassing the data from the 25th to the 75th percentile, while the additional dots indicate the outliers. According to Figure 5 and Table 3, the GPFOnly-trained models demonstrate the best test performance, highlighting the contribution of GPFs to improvements in prediction accuracy. A detailed analysis of the NRMSE metrics for all ML models utilizing three feature sets is provided, along with associated P-values from Wilcoxon tests[64], to indicate the statistical significance (P-value < 0.05) of the improvements. GPFonly-trained models show a significant average performance improvement of 24% compared to NoGPF-trained models. Specifically, the DTR and RFR algorithms achieved a notable 39% improvement (P-value = 0.002), while KNR reached a 32% improvement (P-value = 0.002). In contrast, the GPFCombined-trained models result in only a 9% average improvement without statistical significance, indicating that this combination of features does not enhance performance as effectively as the GPFonly set. The performance of GPFCombined-trained models is not as strong as GPFonly-trained models, which may be attributed to the excessive redundancy introduced by combining GPFs with the initial feature set.
Figure 5. Comparison of testing R2, NRMSE and MRE among different ML algorithms from varying feature sets: (A-C) NoGPF; (D-F) GPFOnly; and (G-I) GPFCombined. The results came from ten times of holdouts. R2: The coefficient of determination; NRMSE: normalized root mean squared error; MRE: mean relative error; ML: machine learning.
Comparison of performance improvement and corresponding statistical significance of NRMSE metrics across feature sets
Algorithm | NoGPF | GPFOnly | Improvement | P-value | GPFCombined | Improvement | P-value |
DTR | 0.21 ± 0.06 | 0.13 ± 0.01 | 39% | 0.002 | 0.19 ± 0.02 | 11% | 0.193 |
ETR | 0.14 ± 0.03 | 0.13 ± 0.01 | 8% | 0.037 | 0.16 ± 0.02 | 14% | 0.004 |
GBR | 0.14 ± 0.03 | 0.10 ± 0.03 | 29% | 0.065 | 0.14 ± 0.02 | 2% | 0.846 |
KNR | 0.16 ± 0.03 | 0.11 ± 0.01 | 32% | 0.002 | 0.14 ± 0.02 | 8% | 0.084 |
Ridge | 0.21 ± 0.02 | 0.19 ± 0.02 | 10% | 0.004 | 0.19 ± 0.02 | 9% | 0.004 |
RFR | 0.15 ± 0.03 | 0.09 ± 0.01 | 39% | 0.002 | 0.14 ± 0.02 | 6% | 0.322 |
SVR | 0.21 ± 0.02 | 0.19 ± 0.03 | 10% | 0.037 | 0.19 ± 0.02 | 10% | 0.002 |
Average | 0.17 ± 0.03 | 0.13 ± 0.02 | 24% | 0.021 | 0.16 ± 0.02 | 9% | 0.208 |
Among the GPFonly-trained models, DTR and RFR exhibit the lowest errors, with NRMSE values of 0.13 ± 0.01 and 0.09 ± 0.01, respectively. Their performances on NoGPF are 0.21 ± 0.06 and 0.15 ± 0.03, reflecting significant improvements after incorporating GPF features. Regarding the improvement among different algorithms, the 39% enhancement observed for DTR and RFR can be attributed to their ability to effectively capture complex nonlinear relationships. The 32% improvement seen with KNR suggests that the introduced features enhance prediction accuracy among neighboring samples.
Conversely, ETR and SVR exhibit smaller improvements of 8% and 10%, respectively, which may indicate lower sensitivity to changes in features. Lastly, ridge demonstrates only a 10% improvement, suggesting a reduced dependence on feature selection and a more limited capacity for handling complex data. Overall, the impact of GPFs varies across DTR, RFR, and KNR, demonstrating more significant performance enhancements, while Ridge models present relatively modest improvements. Based on the comprehensive analysis on the test performance on the HEA dataset, the RFR was selected to construct a model using the GPFonly, while ETR was selected to construct a reference model with NoGPF. The optimized hyperparameters of both ML models were listed in Supplementary Table 4.
Model transfer for RAHEA predictions
Unlike the model- or instance-based TL, we directly transferred the trained ML models discussed in Section “Construction of hardness prediction models” on RAHEAs for hardness prediction without a fine-tuning step[22], as the source and target domain have already been adapted. To quantify the improvement in prediction accuracy on RAHEAs, both ML models trained by NoGPF and GPFonly feature sets were employed for hardness prediction.
Figure 6 illustrates the predicted versus experimental values of samples from the testing sets of both the source and target domains, evaluated using two models trained on different feature sets. In Figure 6A, the prediction points for the testing set closely align with the line x = y (represented by the dashed lines). In contrast, the predictions for the target domain deviate from this line, resulting in a rapid increase of NRMSE from 0.09 to 0.29, indicating a dataset shift due to the absence of domain adaptation (NoGPF). Figure 6B shows that while the predictions from the GPFOnly on the source domain are not as close to the dashed line as those in Figure 6A, both source and target domain predictions are evenly distributed near it. The NRMSE slightly increased from 0.16 to 0.19, demonstrating the robustness of the model enhanced by GPFs.
Figure 6. The predicted vs. experimental values of samples from the testing set and the RAHEA dataset using (A) No-GPF trained model and (B) GPFOnly-trained model. RAHEA: Reduced-activation high-entropy alloy.
We performed a Wilcoxon test to statistically evaluate the performance improvement. The model was randomly excluded ten times with the same hyperparameters, generating predictions for both the source and target domains. The average NRMSE for the NoGPF-trained model on the target domain is 0.27 ± 0.02, while the GPFOnly-trained model achieves 0.19 ± 0.01. This represents a 30% reduction in average NRMSE (P-value = 0.004), indicating that GPFOnly significantly enhances model robustness.
Furthermore, SHAP analysis was conducted on both ML models to reveal the feature contribution and model decision. Figure 7A and B presents the mean absolute SHAP value of each initial element-based feature on both source and target domains, and Figure 7C illustrates the deviation of mean absolute SHAP values between the two domains. Similarly, Figure 7D and E shows that of each GPF in both domains. As shown in Figure 7B, the top three contributors, DRa, VHfus, DI1, are identified in both GPF1 and GPF2. Additionally, features with moderate contributions, such as Dχar, Mv and Mc12, are included in GPF1, which may help explain the significance of domain invariance from the perspective of feature contributions. Regarding the deviation between two domains in Figure 7C and F, the contributions of Dχar, VHfus and Fρ vary significantly, as they appear in both GPF1 and GPF3. The maximum value operator applied on Dχar and Fρ in GPF1 tends to reduce sensitivity to large changes in the importance of domain-invariant feature selection within the feature-based domain adaptation framework.
Figure 7. SHAP analysis of NoGPF and GPFOnly-trained model on both source and target domains. (A-C) Mean absolute SHAP value of each feature in NoGPF-trained model on the (A) source and (B) target, with (C) the deviation between two domains; (D-F) Mean absolute SHAP value of each feature in GPFOnly-trained model on the (D) source and (E) target, with (F) the deviation between two domains. SHAP: Shapley additive explanations.
CONCLUSIONS
In this work, we developed a framework that integrates SR and domain adaptation to enhance the accuracy of material property predictions on small datasets. The key conclusions and future expectations are summarized as follows:
(1) The framework effectively captured domain-invariant features related to hardness in both conventional HEAs and RAHEAs. The domain-invariant features reveal the physical insights for hardness in terms of crystal structure, bonding, and strengthening mechanisms.
(2) The accuracy of hardness predictions for both HEAs and RAHEAs was significantly improved, with NRMSE reduced by 24% for HEAs and 30% for RAHEAs compared to that of models trained with element-based features.
(3) SHAP analysis shows that the model performance by using the domain-invariant features is stable across various composition spaces.
The proposed framework can achieve robust and accurate ML prediction by extracting domain-invariant features, demonstrating its extendibility in various material designs with small datasets.
DECLARATIONS
Authors’ contributions
Conceptualization, methodology, software, data curation, visualization, writing - original draft preparation: Pan, H.
Writing - review and editing, supervision, project administration, funding acquisition: Zheng, M.
Writing - review and editing, supervision: Zhao, S.
Investigation, discussion: Li, X.
Availability of data and materials
The data and algorithms used in the current study will be available from the corresponding author based on reasonable request.
Financial support and sponsorship
This work was supported by the International Partnership Program for Grand Challenges of Chinese Academy of Sciences (Grant No. 145GJHZ2022055GC), Strategic Priority Research Program of Chinese Academy of Sciences (Grant No. XDA0410000), the HFIPS Director’s Fund (Grant No. YZJJ2024QN45), Enterprise Innovation Joint Fund of National Natural Science Foundation of China (Grant No. U22B2064), and Shenzhen Basic Research Program (JCYJ20230807114959029). We acknowledge the Hefei Advanced Computing Center for computational support and thank the Institutional Center for Shared Technologies and Facilities of INEST, HFIPS, and CAS for their technical assistance.
Conflicts of interest
All authors declared that there are no conflicts of interest.
Ethical approval and consent to participate
Not applicable.
Consent for publication
Not applicable.
Copyright
© The Author(s) 2025.
Supplementary Materials
REFERENCES
1. Yeh, J.; Chen, S.; Lin, S.; et al. Nanostructured high-entropy alloys with multiple principal elements: novel alloy design concepts and outcomes. Adv. Eng. Mater. 2004, 6, 299-303.
2. Li, Z.; Pradeep, K. G.; Deng, Y.; Raabe, D.; Tasan, C. C. Metastable high-entropy dual-phase alloys overcome the strength-ductility trade-off. Nature 2016, 534, 227-30.
3. Lei, Z.; Liu, X.; Wu, Y.; et al. Enhanced strength and ductility in a high-entropy alloy via ordered oxygen complexes. Nature 2018, 563, 546-50.
4. Wang, D.; Tan, J.; Li, C.; Qin, X.; Guo, S. Enhanced creep resistance of Ti30Al25Zr25Nb20 high-entropy alloy at room temperature. J. Alloys. Compd. 2021, 885, 161038.
5. Su, Z.; Shi, T.; Yang, J.; et al. The effect of interstitial carbon atoms on defect evolution in high entropy alloys under helium irradiation. Acta. Mater. 2022, 233, 117955.
7. Islam, N.; Huang, W.; Zhuang, H. L. Machine learning for phase selection in multi-principal element alloys. Comput. Mater. Sci. 2018, 150, 230-5.
8. Wen, C.; Zhang, Y.; Wang, C.; et al. Machine learning assisted design of high entropy alloys with desired property. Acta. Mater. 2019, 170, 109-17.
9. Xiong, J.; Shi, S.; Zhang, T. Machine learning of phases and mechanical properties in complex concentrated alloys. J. Mater. Sci. Technol. 2021, 87, 133-42.
10. Wen, C.; Wang, C.; Zhang, Y.; et al. Modeling solid solution strengthening in high entropy alloys using machine learning. Acta. Mater. 2021, 212, 116917.
11. Vazquez, G.; Singh, P.; Sauceda, D.; et al. Efficient machine-learning model for fast assessment of elastic properties of high-entropy alloys. Acta. Mater. 2022, 232, 117924.
12. Li, X.; Zheng, M.; Li, C.; Pan, H.; Ding, W.; Yu, J. Accelerated design of low-activation high entropy alloys with desired phase and property by machine learning. Appl. Mater. Today. 2024, 36, 102000.
13. Miracle, D.; Senkov, O. A critical review of high entropy alloys and related concepts. Acta. Mater. 2017, 122, 448-511.
14. Gorsse, S.; Nguyen, M. H.; Senkov, O. N.; Miracle, D. B. Database on the mechanical properties of high entropy alloys and complex concentrated alloys. Data. Brief. 2018, 21, 2664-78.
15. Xue, D.; Xue, D.; Yuan, R.; et al. An informatics approach to transformation temperatures of NiTi-based shape memory alloys. Acta. Mater. 2017, 125, 532-41.
16. Jones, R.; Heinisch, H.; Mccarthy, K. Low activation materials. J. Nucl. Mater. 1999, 271-272, 518-25.
17. Xian, X.; Zhong, Z.; Zhang, B.; et al. A high-entropy V35Ti35Fe15Cr10Zr5 alloy with excellent high-temperature strength. Mater. Design. 2017, 121, 229-36.
18. Barron, P.; Carruthers, A.; Fellowes, J.; Jones, N.; Dawson, H.; Pickering, E. Towards V-based high-entropy alloys for nuclear fusion applications. Scr. Mater. 2020, 176, 12-6.
19. Kareer, A.; Waite, J.; Li, B.; Couet, A.; Armstrong, D.; Wilkinson, A. Short communication: ‘Low activation, refractory, high entropy alloys for nuclear applications’. J. Nucl. Mater. 2019, 526, 151744.
20. Wang, Y.; Yao, Q.; Kwok, J. T.; Ni, L. M. Generalizing from a few examples: a survey on few-shot learning. ACM. Comput. Surv. 2021, 53, 1-34.
21. Zhang, Y.; Ling, C. A strategy to apply machine learning to small datasets in materials science. npj. Comput. Mater. 2018, 4, 81.
22. Pan, S. J.; Yang, Q. A survey on transfer learning. IEEE. Trans. Knowl. Data. Eng. 2010, 22, 1345-59.
23. Zhuang, F.; Qi, Z.; Duan, K.; et al. A comprehensive survey on transfer learning. Proc. IEEE. 2021, 109, 43-76.
24. Kim, Y.; Kim, Y.; Yang, C.; Park, K.; Gu, G. X.; Ryu, S. Deep learning framework for material design space exploration using active transfer learning and data augmentation. npj. Comput. Mater. 2021, 7, 609.
25. Wang, X.; Tran, N.; Zeng, S.; Hou, C.; Chen, Y.; Ni, J. Element-wise representations with ECNet for material property prediction and applications in high-entropy alloys. npj. Comput. Mater. 2022, 8, 945.
26. Wei, X.; Wang, C.; Jia, Z.; Xu, W. High-cycle fatigue S-N curve prediction of steels based on a transfer learning-guided convolutional neural network. J. Mater. Inf. 2022, 2, 9.
27. Quiñonero-Candela, J.; Sugiyama, M.; Schwaighofer, A.; Lawrence, N. D. Dataset shift in machine learning. MIT Press, 2009.
28. Li, K.; Decost, B.; Choudhary, K.; Greenwood, M.; Hattrick-Simpers, J. A critical examination of robustness and generalizability of machine learning prediction of materials properties. npj. Comput. Mater. 2023, 9, 1012.
29. Wang, J.; Lan, C.; Liu, C.; et al. Generalizing to unseen domains: a survey on domain generalization. IEEE. Trans. Knowl. Data. Eng. 2023, 35, 8052-72.
30. Motiian, S.; Jones, Q.; Iranmanesh, S. M.; Doretto, G. Few-shot adversarial domain adaptation. In Proceedings of the 31st International Conference on Neural Information Processing Systems, Long Beach, USA; Curran Associates Inc., 2017; pp 6673-83.
31. Long, M.; Wang, J.; Ding, G.; Pan, S. J.; Yu, P. S. Adaptation regularization: a general framework for transfer learning. IEEE. Trans. Knowl. Data. Eng. 2014, 26, 1076-89.
32. Stojanov, P.; Li, Z.; Gong, M.; Cai, R.; Carbonell, J. G.; Zhang, K. Domain adaptation with invariant representation learning: what transformations to learn? In Proceedings of the 35th International Conference on Neural Information Processing Systems; Curran Associates Inc., 2021.
33. Goetz, A.; Durmaz, A. R.; Müller, M.; et al. Addressing materials’ microstructure diversity using transfer learning. npj. Comput. Mater. 2022, 8, 703.
34. Hu, J.; Liu, D.; Fu, N.; Dong, R. Realistic material property prediction using domain adaptation based machine learning. Digit. Discov. 2024, 3, 300-12.
35. Zhao, H.; Combes, R. T. D.; Zhang, K.; Gordon, G. On learning invariant representations for domain adaptation. arXiv2019, arXiv:1901.09453. Available online: https://doi.org/10.48550/arXiv.1901.09453. (accessed 6 Feb 2025)
36. Huo, H.; Rupp, M. Unified representation of molecules and crystals for machine learning. Mach. Learn. Sci. Technol. 2022, 3, 045017.
37. Pan, S. J.; Tsang, I. W.; Kwok, J. T.; Yang, Q. Domain adaptation via transfer component analysis. IEEE. Trans. Neural. Netw. 2011, 22, 199-210.
38. Fernando, B.; Habrard, A.; Sebban, M.; Tuytelaars, T. Unsupervised visual domain adaptation using subspace alignment. In 2013 IEEE International Conference on Computer Vision, Sydney, Australia, December 01-08, 2013; IEEE, 2013; pp 2960-7.
39. Peng, H.; Long, F.; Ding, C. Feature selection based on mutual information: criteria of max-dependency, max-relevance, and min-redundancy. IEEE. Trans. Pattern. Anal. Mach. Intell. 2005, 27, 1226-38.
40. Koza, J. Genetic programming as a means for programming computers by natural selection. Stat. Comput. 1994, 4, BF00175355.
41. Chen, Y.; Hu, X.; Zhao, J.; Wang, Z.; Li, J.; Wang, J. Symbolic regression and two-point statistics assisted structure-property linkage based on irregular-representative volume element. Adv. Theory. Simul. 2023, 6, 2200524.
42. Xiong, J.; Shi, S.; Zhang, T. A machine-learning approach to predicting and understanding the properties of amorphous metallic alloys. Mater. Design. 2020, 187, 108378.
43. Huang, X.; Zheng, L.; Xu, H.; Fu, H. Predicting and understanding the ductility of BCC high entropy alloys via knowledge-integrated machine learning. Mater. Design. 2024, 239, 112797.
44. Lee, J. A.; Figueiredo, R. B.; Park, H.; Kim, J. H.; Kim, H. S. Unveiling yield strength of metallic materials using physics-enhanced machine learning under diverse experimental conditions. Acta. Mater. 2024, 275, 120046.
45. Feng, S.; Sun, X.; Chen, G.; Wu, H.; Chen, X. LBE corrosion fatigue life prediction of T91 steel and 316 SS using machine learning method assisted by symbol regression. Int. J. Fatigue. 2023, 177, 107962.
46. Couzinié, J. P.; Senkov, O. N.; Miracle, D. B.; Dirras, G. Comprehensive data compilation on the mechanical properties of refractory high-entropy alloys. Data. Brief. 2018, 21, 1622-41.
47. Carruthers, A.; Li, B.; Rigby, M.; et al. Novel reduced-activation TiVCrFe based high entropy alloys. J. Alloys. Compd. 2021, 856, 157399.
48. Ayyagari, A.; Salloom, R.; Muskeri, S.; Mukherjee, S. Low activation high entropy alloys for next generation nuclear applications. Materialia 2018, 4, 99-103.
49. Liu, Y.; Yao, Z.; Zhang, P.; et al. Enhanced strength without sacrificing ductility in FeCrMnVSix high entropy alloys via controlling the ratio of metallic to covalent bonding. Mater. Design. 2023, 225, 111565.
50. Carruthers, A.; Shahmir, H.; Hardwick, L.; Goodall, R.; Gandy, A.; Pickering, E. An assessment of the high-entropy alloy system VCrMnFeAlx. J. Alloys. Compd. 2021, 888, 161525.
52. Guo, S.; Liu, C. Phase stability in high entropy alloys: formation of solid-solution phase or amorphous phase. Prog. Nat. Sci. Mater. Int. 2011, 21, 433-46.
53. Wang, W. Y.; Shang, S. L.; Wang, Y.; et al. Atomic and electronic basis for the serrations of refractory high-entropy alloys. npj. Comput. Mater. 2017, 3, 24.
54. Weng, B.; Song, Z.; Zhu, R.; et al. Simple descriptor derived from symbolic regression accelerating the discovery of new perovskite catalysts. Nat. Commun. 2020, 11, 3513.
55. Lavalle, S. M.; Branicky, M. S.; Lindemann, S. R. On the relationship between classical grid search and probabilistic roadmaps. Int. J. Robot. Res. 2004, 23, 673-92.
56. Lundberg, S. M.; Lee, S. I. A unified approach to interpreting model predictions. In Proceedings of the 31st International Conference on Neural Information Processing Systems, Long Beach, USA; Curran Associates Inc., 2017; pp 4766-75.
57. Pedregosa, F.; Varoquaux, G.; Gramfort, A.; et al. Scikit-learn: machine learning in Python. arXiv2012, arXiv:1201.0490. Available online: https://doi.org/10.48550/arXiv.1201.0490. (accessed 6 Feb 2025)
58. Harris, C. R.; Millman, K. J.; van, W. S. J.; et al. Array programming with NumPy. Nature 2020, 585, 357-62.
59. Virtanen, P.; Gommers, R.; Oliphant, T. E.; et al; SciPy 1.0 Contributors. SciPy 1.0: fundamental algorithms for scientific computing in Python. Nat. Methods.2020, 17, 261-72.
61. Borgwardt, K. M.; Gretton, A.; Rasch, M. J.; Kriegel, H. P.; Schölkopf, B.; Smola, A. J. Integrating structured biological data by kernel maximum mean discrepancy. Bioinformatics 2006, 22, e49-57.
62. Du, Y. Y.; Lu, Y. P.; Li, T. J.; Wang, T. M.; Zhang, G. L. Effect of aluminium content of AlxCrFe1.5Ni0.5 multiprincipal alloys on microstructure and alloy hardness. Mater. Res. Innov. 2011, 15, 107-10.
63. Wang, W.; Wang, W.; Wang, S.; Tsai, Y.; Lai, C.; Yeh, J. Effects of Al addition on the microstructure and mechanical property of AlxCoCrFeNi high-entropy alloys. Intermetallics 2012, 26, 44-51.
Cite This Article
How to Cite
Download Citation
Export Citation File:
Type of Import
Tips on Downloading Citation
Citation Manager File Format
Type of Import
Direct Import: When the Direct Import option is selected (the default state), a dialogue box will give you the option to Save or Open the downloaded citation data. Choosing Open will either launch your citation manager or give you a choice of applications with which to use the metadata. The Save option saves the file locally for later use.
Indirect Import: When the Indirect Import option is selected, the metadata is displayed and may be copied and pasted as needed.
About This Article
Copyright
Data & Comments
Data
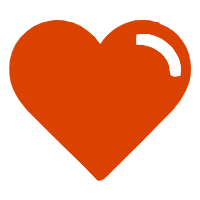
Comments
Comments must be written in English. Spam, offensive content, impersonation, and private information will not be permitted. If any comment is reported and identified as inappropriate content by OAE staff, the comment will be removed without notice. If you have any queries or need any help, please contact us at [email protected].