Stretchable flexible sensors for smart tires based on laser-induced graphene technology
Abstract
Continuous feedback on a tire is an essential means to ensure tire safety. Smart tires are an important part of the future vehicle control system, which affects the safety and comfort of vehicles by combining sensors with traditional tires to achieve continuous monitoring of real-time dynamic parameters. A stretchable and flexible sensor made of laser-induced graphene (LIG) and PDMS, designed for use in smart tires, is presented in this work. The sensor is known as a LIG-PDMS sensor. Using transfer printing, LIG is formed on a commercial polyimide film under the scribing of a laser beam following the predesigned route before being transferred to a PDMS film. This technology is used to successfully prepare flexible sensors for measuring the tire road interaction at different driving speeds due to its flexibility and shape-following characteristics. The real-time monitoring of the wheel speed and the shape of the tire grounding mark during the driving process is realized by embedding multiple LIG sensors in the tire to monitor the strain information of the tire grounding. Results show that the tire deformation can be accurately feedbacked with the LIG sensors, demonstrating our method's capability for designing and manufacturing intelligent tires.
Keywords
INTRODUCTION
As the only interaction between the vehicle and the road, the tire affects the stability, dynamics, safety, and controllability of the vehicle. Considering that the forces required for the vehicle to move on the road are generated by the interaction between the tire and the road, the key directions in vehicle research are the mechanical properties of the tire and the contact state of tire and road. Monitoring tire movement is crucial because it is complex and involves considerable deformation, making it difficult to quantify. In response to these needs, the concept of smart tires has been proposed[1-3]. A smart tire is a highly intelligent tire product that integrates advanced technologies, such as smart material technology, sensing technology, signal conditioning, and communication technology, and even has various sensors embedded within the tire. The various tire parameters (such as tire pressure[4,5], tire temperature[6], tire strain[7-9], tire force[10,11], and road adhesion information[12-14]) measured by these sensors are transmitted to the central controller of the vehicle for real-time monitoring. Monitoring parameters in real-time and performing corresponding improvements can improve vehicle dynamics and fuel economy, and can prevent dangerous situations, such as blowouts, rollovers, and skidding, by warning in time before they occur. Efforts have been exerted globally to integrate wireless sensors within tires for measuring dynamic mechanical parameters. The in-tire sensors currently being developed need to fit well into the tire structure without interfering with the tire’s movement and be able to adapt to changes in the tire’s internal structure and environment under different operating conditions, which demands flexibility and form-following properties of the sensors. Traditionally, the most classic tire pressure monitoring system transmits tire pressure signals through an in-vehicular wireless device, which is employed to warn a driver of any loss in tire pressure[15,16].
At present, smart tire technology has flourished, and most methods are implemented by collecting the data generated by the tire tread deformation during the driving and actuating process of the vehicle and then calculating and predicting the tire force and road adhesion coefficient with the help of corresponding algorithms to obtain the specific vehicle movement state. The classification of smart tires is based on the type of sensors embedded in the tires, including acceleration sensors[17,18], optical sensors[19], acoustic sensors[20], and piezoelectric sensors[1,10,21,22].
Kim proposed an algorithm that can determine the road surface condition of a driving vehicle using an acceleration sensor attached to the inside of a tire[17]. Dasol[23] presented a new load estimation algorithm based on an acceleration sensor. This algorithm is analyzed by using a flexible annular tire model, which is a physical tire model and is constructed on the basis of the relationship between the load contact angle pressures. Alfred conducted tire deformation[24] research by using surface acoustic waves to monitor the strain on the inner surface of the tire and then determined the state of the tire under stress employing an algorithm. However, the sensor requires the insertion of a probe into the tire carcass, which can affect the regular use of the tire and increase the safety risks. Generally, the tire generates a sizeable centrifugal force when it rotates at high speed. The sensor suffers a significant acceleration when it passes through the contact area, which affects the contact quality between the tire and the ground, further increasing the safety risks. Optical sensors are noncontact measurement sensors that measure the lateral, longitudinal, and lateral deformation of tires with high accuracy. Tuononen et al. used optical sensors to measure the coefficient of friction of tires on the road[25], and Erdogan et al. used polyvinylidene fluoride piezoelectric sensors to monitor the deformation of the tire carcass[21], which are one of the few experimental cases where flexible sensors were used as in-tire sensing.
However, most of these sensors are rigid, require an external power supply, and are manufactured using a time-consuming, multistep process, which increases the complexity and cost. In the development and design process of the more mature technology of intelligent tires, the overall architecture is still highly complex. Its implementation requires the interaction of multiple subsystems in the vehicle and a number of sensor types. Flexible sensors can be used to capture a wide range of signals because of flexible and stretchable, such as pulse, respiration, tremor, and limb movements[26-29]. Due to these characteristics of flexible sensors, flexible sensors can be better used in smart tires. There are various substrates for flexible sensors, which can be stretched by structural design[30-32], or by using the mechanical properties of the substrate material itself[33-35]. The material of its conductive medium is also varied, and laser-induced graphene is mostly used for the preparation of flexible smart sensors due to its good properties[36-41]. The substrate of laser-induced graphene (LIG) flexible sensor is carbon-based silicone with good elasticity, which can fit well with the inner surface of the tire and can follow the deformation of the tire. LIG was first discovered accidentally during the laser cutting of commercial polyimide (PI) films[42]. LIG can be successfully prepared on many types of natural and synthetic materials with sufficient carbon sources[42-51]. The prepared LIG can be transferred from PI films to stretchable elastic substrates (e.g., polydimethylsiloxane or polyurethane), thus increasing the flexibility and stretchability of the sensor[52,53].
In this work, we report the design and fabrication of a flexible LIG-based sensor and its application in smart tires to record the ground trace and wheel speed. The tensile performance of PI-based and PDMS-based sensors is compared systematically to select the most suitable sensor for smart tires, where the PDMS-based sensor is obtained by transferring the prepared LIG from PI film to PDMS film. The results show that
EXPERIMENTAL
Preparation of the LIG-PI sensor
The PI film was first attached to the rectangular glass plate through the thermal release tapes, ensuring that the PI film would remain flat after the laser scan, and the thickness of the PI film was 75 μm. Then, the prepared PI film was placed on the commercial laser cutting platform (X-7050, G.U. Eagle Automation). The PI film was scanned with a CO2 laser generator, and the scanning parameters were 12% specific power, 20 kHz laser frequency, 300 mm/s scanning speed, and 1000 DPI. The LIG was prepared into a
Preparation of the stretchable LIG-PDMS sensor
The flexible LIG-PDMS sensor was obtained by transferring the LIG on PI film to the PDMS carrier. A rectangular pattern of 10 mm × 10 mm was scanned on the PI film by using the same method. The PDMS solution was spin-coated on the LIG pattern with a spin-coating speed of 150 RPM and a spin-coating time of 60 seconds before curing at 70 °C for 1 h. The PDMS to curing agent mass ratio in PDMS prepolymer is 10:1, and the thickness of the PDMS film is 1 mm. The conductive silver was coated into electrodes to connect the wires on two sides of the LIG region after stripping the PDMS from the PI substrate. The LIG was coated with PDMS solution after transferring it to the PDMS carrier by transfer printing technology; the thickness of PDMS film covered with LIG is about 30 μm. The fabrication of the stretchable LIG-PDMS sensor was completed after curing the PDMS at 70 °C for 1 h.
RESULTS AND DISCUSSION
Figure 1A depicts the method of generating LIG on PI films. A 10 × 10 mm serpentine LIG pattern was generated by scanning along a predesigned set path using a CO2 laser generator, and the conductive silver was coated into electrodes to connect the wires on two sides of the LIG region. The picture is shown in Figure 1C. However, the in-plane mechanical robustness of the PI substrate limits the stretchability of the LIG-PI sensor. For this reason, the transfer printing technology was used to transfer the LIG from PI films to the softer substrate PDMS surface. As shown in Figure 1B, the configured PDMS solution was
Figure 1. Fabrication of LIG-based strain sensor. (A) Schematic diagram of the preparation of the LIG in a specific pattern; (B) schematic illustration of the fabrication process of the LIG-based stretchable sensor; (C) optical images of LIG in PI film; (D) optical images of the LIG-PDMS composite prepared by transferring LIG from PI to PDMS; (E) the 100× and 1,000× SEM images of LIG.
Electrical performances of the LIG-based sensors under the tension loads
Figures 2A and B show the normalized resistance change of the LIG-based sensor with PI substrate and PDMS substrate under tensile loading, respectively. With the increase in tension, the normalized resistance of the LIG-PI increases from 0 to 47, corresponding to a strain of 3%. At the beginning of tension from 0% to 1%, the normalized resistance of the PI-LIG strain sensor increases slowly with gauge factor (GF) = 5 and then increases sharply with GF = 20. The normalized resistance of the LIG-PDMS increases linearly from 0 to 2,300 with GF = 31, which corresponds to a strain of 70% at this time. The GF, a critical parameter to evaluate the sensitivity of the sensors, is presented and calculated by using GF = (∆R/R0)/ε, where ∆R, R0, and ε denote the variation of the resistance, original resistance, and the applied strain, respectively. PDMS has better stretchable performance with more stable linear resistance change by comparing the normalized resistance change during the stretching of two flexible piezoresistive sensors, LIG-PI and LIG-PDMS, so LIG-PDMS is used as the smart tire sensor. During the driving of the car, the tire will rotate at different speeds, which requires the sensor to have stable performance under different strain rates. Figure 2C shows the variation of LIG-PDMS normalized resistance at different strain rates, from 0% to 20%. The normalized resistance corresponding to 20% tensile strain is stable with the increase in the strain rate, indicating that this sensor can be used at different strain rates and has extremely high stability. Figure 2D shows that the LIG-PDMS strain sensor exhibits excellent durability in the 20% tensile strain range, and the partial magnification shows that this sensor has an extremely short response time. This finding indicates that
Figure 2. Electrical performances of LIG-based strain sensor. (A) The normalized resistance versus the tensile strain for the LIG-PI sensor; (B) the normalized resistance versus the tensile strain for the LIG-PDMS sensor; (C) the normalized resistance values of the LIG-PDMS sensor during tensile experiments with different strain rates; (D) relative change in resistance under repeated loading and unloading of 20% strain for 200 cycles.
The feedback mechanism of the smart tire
During the movement of the car, the grounding of the tire will be flattened by the extrusion of the rigid ground. At this time, the center of the tire circle will be lowered, so the actual effective radius of wheel travel Re is slightly smaller than the actual radius of the tire Rr. Figure 3A shows the mechanical deformation model of the tire grounding[54]. The pressure distributed within the tire grounding imprint during motion is a highly critical object of study. In previous studies of the mechanical properties of tires, researchers assumed the tire contact force as a symmetric parabolic distribution, and the equation of force distribution can be expressed as

where a is the tire grounding imprint half-length, Fz is the vertical load, and qz(x) is the tire distribution force at distance x from the center point.
Figure 3. The feedback mechanism of the smart tie with smart sensors. (A) Grounding deformation and mechanical model of the moving tire; (B) schematic diagram of tire deformation under different strain types and corresponding waveform.
However, this symmetrical parabolic pressure distribution can only approximate the tire force distribution under some small load states. When the wheel is under heavy load, the movement causes the wheel’s center of gravity to deviate due to increased inertia, and the peak force within the tire’s grounding imprint is shifted. The entire pressure distribution takes the form of a two-end distribution. In this case, the researchers improved the description of the pressure distribution by establishing an arbitrary pressure distribution form of the tire, which can be expressed as Equation (2).

where u is the relative coordinate of the grounding trace, marked as x/a, and η(u) is the corresponding distribution function.
As shown in Figure 3B, the tire will go through three deformation stages when rolling on the road, and the strain sensor will produce different types of deformation when it enters these phases. The tire compresses because of pressure before the grounding phase, and the sensor enters the compression state with this compressive strain. The LIG fibers are more tightly connected, resulting in a decrease in sensor resistance. When entering the grounding phase, the tire will gradually stretch and the sensor resistance will increase until the sensor is completely in the grounding phase[1]. The strain state of the sensor changes from tension to compression and then returns to normal when the sensor is disconnected from the grounding phase. This condition is reflected in the resistance signal, which becomes smaller, then larger, and finally smoother. The three phases are defined as the P, Q, and R zones, where P and R are the compression zones, and Q is the stretch zone. TA is the time between entering the grounding phase and leaving the grounding phase. TC is the period of the sensor’s strain waveform, that is, the time elapsed when the tire rolls over a week. HC is the change in the resistance of sensor in the fully stretched zone, and the strain can be obtained by combining the sensitivity curve of LIG-PDMS sensor.
Applications of LIG-PDMS sensors in smart tire
The grounding length of the tire is an essential parameter in tire load estimation. The analysis of the grounding imprint is simple when the tire is in a static state, whereas measuring the grounding length of the tire is difficult when the tire is rolling. A LIG-PDMS strain sensor is inserted inside the wheel. When the sensor enters and exits, the deformation region can be reflected by the feedback resistance signal waveform so that the length of the grounding can be easily obtained. The calculation equation can be expressed as
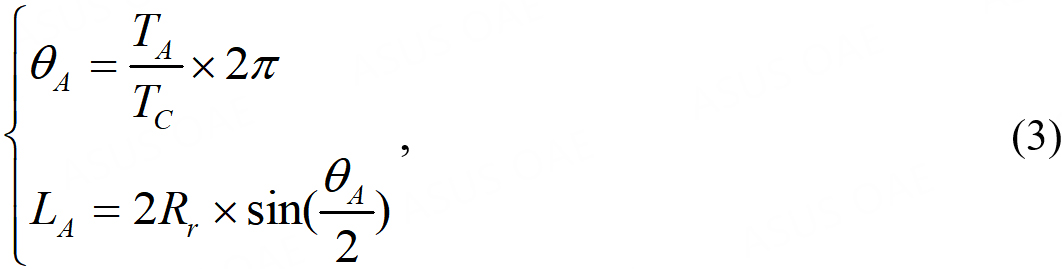
where θA is the tire ground angle, Tc is the time of tire roll over a week experienced, and LA is the length of grounding phase.
The prediction of the ground impression can be achieved by arranging multiple LIG sensors horizontally, and the ground impression of the tire can be obtained by connecting the ground length predicted by each LIG sensor. The LIG sensor arrangement is shown in Figure 4A, the distance between each sensor is 10 mm, the tire width is 10 cm, and the dimension of LIG-PDMS sensor is 20 × 12 mm2. The 2D grounding imprint can be obtained after measuring and calculating the ground length values of all LIG-PDMS sensors. The specific steps are as follows: (i) the length values obtained from the LIG sensor test and calculation are expressed in line segments; (ii) the corresponding line segments are arranged in accordance with the sensor distribution; (iii) the grounding imprint pattern is obtained by connecting the end points of adjacent grounding line segments, as shown in Figure 4B. In accordance with the above experimental method, several experiments were conducted, and the results were obtained, as shown in Figure 4C. The predicted results of some tire grounding marks are close to the topography map of grounding marks (actual results), which proves the accuracy of the LIG-PDMS sensor in predicting the grounding marks of tires.
Figure 4. The prediction of the tire grounding trace based on LIG-based sensor. (A) The arrangement of LIG-based sensor; (B) the grounding trace rubbing and the 2d figure of the ground segment of the LIG-based sensor; (C) comparison between different grounding imprinting experiments and rubbing.
The tire speed information can be read directly by attaching the LIG strain sensor to the inside of the tire. When the tire rolls on the ground, a wave signal can be obtained for each rolling revolution. Therefore, the rotation speed of the tire can be obtained by combining the distance that the tire has experienced for each turn and the time between each wave crest. The calculation equation can be expressed as
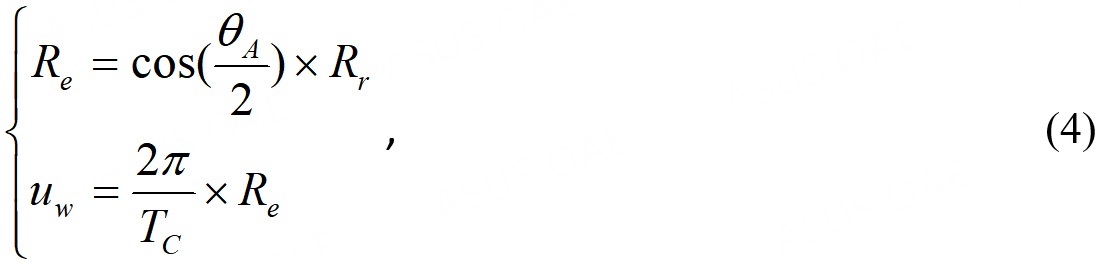
where Re is the running radius of the tire, and uw is the wheel speed.
Figure 5A shows the attachment position of the LIG-PDMS sensors in the tire, and a sensor is installed every 25 mm to prevent the connection failure of a sensor due to fast tire speed during the driving process. The car was driven smoothly with variable speeds from 20 km/h to 60 km/h, and each speed stage lasted for 1 min with gentle acceleration and deceleration during the variable speed process. Figure 5B shows the signal feedback curve of the LIG-PDMS sensor during the driving process. A section with a similar peak shape in each speed level (duration 3 s) is randomly selected. Tc and TA of each cycle are measured, and the corresponding wheel speeds are calculated in accordance with Equations (3) and (4). The results are shown in Figure 5C. The calculated wheel speeds are 21.272, 41.713, and 58.980 km/h, and the real-time speeds read from the speedometer are 22, 40, and 59 km/h. Comparing the theoretical speed calculation with the actual speedometer speed, the calculation of wheel speed using LI-PDMS sensors has a high degree of accuracy.
CONCLUSIONS
In this work, we systematically completed the design, fabrication of LIG-based sensors, and their applications in smart tires. Two LIG-based sensors were fabricated with PI film and PDMS substrates, and the better flexibility, stretchability, and excellent linearity with remarkable durability of LIG-PDMS sensor were determined by tensile experiments for smart tire applications. The feasibility of LIG embedded in the tire to monitor the tire deformation was confirmed through the experiment, and the time and strain of LIG sensors entering and leaving the tire grounding area were analyzed to achieve the estimation of the tire grounding length. The prediction of tire grounding marks and vehicle speed in an accurate manner was achieved by the arrangement of multiple LIG sensors.
DECLARATIONS
Authors’ contributionsConceived the research idea and supervised the overall project: Guo X
Wrote the manuscript with contributions from all authors: Yue Y, Guo X
Conducted the composite preparation and electrical characterization experiments: Wang H, Zhao Z
Fabricated the sensors and studied their applications: Li X, Zhao Z, Wang H
The manuscript was written through the contributions of all authors. All authors have given approval for the final version of the manuscript.
Availability of data and materialsNot applicable.
Financial support and sponsorshipThis work was supported by the National Natural Science Foundation of China (Grant No. 12072030).
Conflicts of interestAll authors declared that there are no conflicts of interest.
Ethical approval and consent to participateNot applicable.
Consent for publicationNot applicable.
Copyright© The Author(s) 2023.
REFERENCES
1. Maurya D, Khaleghian S, Sriramdas R, et al. 3D printed graphene-based self-powered strain sensors for smart tires in autonomous vehicles. Nat Commun 2020;11:5392.
2. Xiong Y, Tuononen A. A laser-based sensor system for tire tread deformation measurement. Meas Sci Technol 2014;25:115103.
3. Xiong Y, Yang X. A review on in-tire sensor systems for tire-road interaction studies. SR 2018;38:231-8.
4. Zhang J, Wang C, Xie X, Li M, Li L, Mao X. Development of MEMS composite sensor with temperature compensation for tire pressure monitoring system. J Micromech Microeng 2021;31:125015.
5. Boada M, Lazaro A, Villarino R, Gil-dolcet E, Girbau D. Battery-less NFC bicycle tire pressure sensor based on a force-sensing resistor. IEEE Access 2021;9:103975-87.
6. Wang C, Taylor BD. SansEC temperature sensor for tire safety monitoring application. 2011 Future of Instrumentation International Workshop (FIIW) Proceedings. IEEE ;2011:146-9.
7. Mendoza-Petit MF, García-Pozuelo D, Díaz V, Olatunbosun O. A strain-based intelligent tire to detect contact patch features for complex maneuvers. Sensors 2020;20:1750.
8. Agliullin TA, Gubaidullin RR, Morozov OG, Sahabutdinov A, Ivanov V. Tire strain measurement system based on addressed FBG-structures. 2019 Systems of Signals Generating and Processing in the Field of on Board Communications. IEEE 2019:1-5.
9. Agliullin TA, Gubaidullin RR, Ivanov V, Morozov O, Sakhabutdinov A. Addressed FBG-structures for tire strain measurement. Optical Technologies for Telecommunications 2018. SPIE 2019;11146:392-7.
10. Sui Z, Wang Z, Zhang X, et al. Piezoelectric based smart tire for vehicle speed and load detection and energy harvesting. 2021 IEEE International Conference on Flexible and Printable Sensors and Systems (FLEPS). IEEE ;2021:1-4.
11. Andrews JB, Cardenas JA, Lim CJ, Noyce SG, Mullett J, Franklin AD. Fully printed and flexible carbon nanotube transistors for pressure sensing in automobile tires. IEEE Sensors J 2018;18:7875-80.
12. Wang Y, Hu J, Wang F, et al. Tire road friction coefficient estimation: review and research perspectives. Chin J Mech Eng 2022:35.
13. Leng B, Jin D, Xiong L, Yang X, Yu Z. Estimation of tire-road peak adhesion coefficient for intelligent electric vehicles based on camera and tire dynamics information fusion. Mech Syst Signal Pr 2021;150:107275.
14. Tian C, Leng B, Hou X, Xiong L, Huang C. Multi-sensor fusion based estimation of tire-road peak adhesion coefficient considering model uncertainty. Remote Sens 2022;14:5583.
15. Formentin S, Onesto L, Colombo T, Pozzato A, Savaresi SM. h-TPMS: a hybrid tire pressure monitoring system for road vehicles. Mechatronics 2021;74:102492.
16. Lee D, Yoon D, Kim G. New indirect tire pressure monitoring system enabled by adaptive extended kalman filtering of vehicle suspension systems. Electronics 2021;10:1359.
17. Kim H, Han J, Lee S, et al. A road condition classification algorithm for a tire acceleration sensor using an artificial neural network. Electronics 2020;9:404.
18. Gu T, Li B, Quan Z, et al. The vertical force estimation algorithm based on smart tire technology. WEVJ 2022;13:104.
19. Fontaine M, Coiret A, Cesbron J, Baltazart V, Bétaille D. In-tire distributed optical fiber (DOF) sensor for the load assessment of light vehicles in static conditions. Sensors 2021;21:6874.
20. Kim M, Park J, Choi S. Road type identification ahead of the tire using D-CNN and reflected ultrasonic signals. Int J Automot Technol 2021;22:47-54.
21. Erdogan G, Alexander L, Rajamani R. A novel wireless piezoelectric tire sensor for the estimation of slip angle. Meas Sci Technol 2010;21:015201.
22. den Ende DA, van de Wiel HJ, Groen WA, van der Zwaag S. Direct strain energy harvesting in automobile tires using piezoelectric PZT-polymer composites. Smart Mater Struct 2012;21:015011.
23. Jeong D, Lee J, Choi S, Kim M. Load estimation of intelligent tires equipped with acceleration sensors. 2019 IEEE Sensors Applications Symposium (SAS). IEEE ;2019:1-5.
24. Pohl A, Ostermayer G, Reindl L, Seifert F. Monitoring the tire pressure at cars using passive SAW sensors. 1997 IEEE Ultrasonics Symposium Proceedings. An International Symposium (Cat. No. 97CH36118). IEEE 1997;1:471-4.
25. Tuononen AJ. Laser triangulation to measure the carcass deflections of a rolling tire. Meas Sci Technol 2011;22:125304.
26. Gao Y, Yu L, Yeo JC, Lim CT. Flexible hybrid sensors for health monitoring: materials and mechanisms to render wearability. Adv Mater 2020;32:e1902133.
27. Zhang Y, Zhang F, Yan Z, et al. Printing, folding and assembly methods for forming 3D mesostructures in advanced materials. Nat Rev Mater 2017:2.
28. Ma Y, Choi J, Hourlier-Fargette A, et al. Relation between blood pressure and pulse wave velocity for human arteries. Proc Natl Acad Sci USA 2018;115:11144-9.
29. Carvalho AF, Fernandes AJS, Martins R, Fortunato E, Costa FM. Laser-induced graphene piezoresistive sensors synthesized directly on cork insoles for gait analysis. Adv Mater Technol 2020;5:2000630.
30. Yan D, Chang J, Zhang H, et al. Soft three-dimensional network materials with rational bio-mimetic designs. Nat Commun 2020;11:1180.
31. Bai K, Cheng X, Xue Z, et al. Geometrically reconfigurable 3D mesostructures and electromagnetic devices through a rational bottom-up design strategy. Sci Adv 2020;6:eabb7417.
32. Ma Q, Zhang Y. Mechanics of fractal-inspired horseshoe microstructures for applications in stretchable electronics. J Appl Mech 2016;83:111008.
33. Dal H, Açıkgöz K, Badienia Y. On the performance of isotropic hyperelastic constitutive models for rubber-like materials: a state of the art review. Appl Mech Rev 2021;73:020802.
34. Liu T, Asheghi M, Goodson KE. Performance and manufacturing of silicon-based vapor chambers. Appl Mech Rev 2021;73:010802.
35. Firooz S, Steinmann P, Javili A. Homogenization of composites with extended general interfaces: comprehensive review and unified modeling. Appl Mech Rev 2021;73:040802.
36. Zhang C, Peng Z, Huang C, et al. High-energy all-in-one stretchable micro-supercapacitor arrays based on 3D laser-induced graphene foams decorated with mesoporous ZnP nanosheets for self-powered stretchable systems. Nano Energy 2021;81:105609.
37. Araujo WR, Frasson CMR, Ameku WA, Silva JR, Angnes L, Paixão TRLC. Single-step reagentless laser scribing fabrication of electrochemical paper-based analytical devices. Angew Chem Int Ed Engl 2017;56:15113-7.
38. Santos NF, Rodrigues J, Pereira SO, Fernandes AJS, Monteiro T, Costa FM. Electrochemical and photoluminescence response of laser-induced graphene/electrodeposited ZnO composites. Sci Rep 2021;11:17154.
39. Carvalho AF, Kulyk B, Fernandes AJS, Fortunato E, Costa FM. A review on the applications of graphene in mechanical transduction. Adv Mater 2022;34:e2101326.
40. Romero FJ, Salinas-Castillo A, Rivadeneyra A, et al. In-depth study of laser diode ablation of kapton polyimide for flexible conductive substrates. Nanomaterials 2018;8:517.
41. Ehsani H, Boyd JD, Wang J, Grady ME. Evolution of the laser-induced spallation technique in film adhesion measurement. Appl Mech Rev 2021;73:030802.
42. Lin J, Peng Z, Liu Y, et al. Laser-induced porous graphene films from commercial polymers. Nat Commun 2014;5:5714.
43. Rodriguez RD, Shchadenko S, Murastov G, et al. Ultra-robust flexible electronics by laser-driven polymer-nanomaterials integration. Adv Funct Mater 2021;31:2008818.
44. Cao L, Zhu S, Pan B, et al. Stable and durable laser-induced graphene patterns embedded in polymer substrates. Carbon 2020;163:85-94.
45. Wang H, Wang H, Wang Y, et al. Laser writing of Janus graphene/Kevlar textile for intelligent protective clothing. ACS Nano 2020;14:3219-26.
46. Li Z, Lu L, Xie Y, et al. Preparation of laser-induced graphene fabric from silk and its application examples for flexible sensor. Adv Eng Mater 2021;23:2100195.
47. Kulyk B, Matos M, Silva BF, et al. Conversion of paper and xylan into laser-induced graphene for environmentally friendly sensors. Diam Relat Mater 2022;123:108855.
48. Mendes LF, Pradela-filho LA, Paixão TR. Polyimide adhesive tapes as a versatile and disposable substrate to produce CO2 laser-induced carbon sensors for batch and microfluidic analysis. Microchem J 2022;182:107893.
49. Getachew BA, Bergsman DS, Grossman JC. Laser-induced graphene from polyimide and polyethersulfone precursors as a sensing electrode in anodic stripping voltammetry. ACS Appl Mater Interf 2020;12:48511-7.
50. Martins L, Kulyk B, Theodosiou A, et al. Laser-induced graphene from commercial polyimide coated optical fibers for sensor development. Opt Laser Technol 2023;160:109047.
51. Kulyk B, Silva BFR, Carvalho AF, et al. Laser-induced graphene from paper for mechanical sensing. ACS Appl Mater Interf 2021;13:10210-21.
52. Sun B, McCay RN, Goswami S, et al. Gas-permeable, multifunctional on-skin electronics based on laser-induced porous graphene and sugar-templated elastomer sponges. Adv Mater 2018;30:e1804327.
53. Dallinger A, Keller K, Fitzek H, Greco F. Stretchable and skin-conformable conductors based on polyurethane/laser-induced graphene. ACS Appl Mater Interf 2020;12:19855-65.
Cite This Article
Export citation file: BibTeX | EndNote | RIS
OAE Style
Yue Y, Li X, Zhao Z, Wang H, Guo X. Stretchable flexible sensors for smart tires based on laser-induced graphene technology. Soft Sci 2023;3:13. http://dx.doi.org/10.20517/ss.2023.02
AMA Style
Yue Y, Li X, Zhao Z, Wang H, Guo X. Stretchable flexible sensors for smart tires based on laser-induced graphene technology. Soft Science. 2023; 3(2): 13. http://dx.doi.org/10.20517/ss.2023.02
Chicago/Turabian Style
Yang Yue, Xuyang Li, Zifen Zhao, Hao Wang, Xiaogang Guo. 2023. "Stretchable flexible sensors for smart tires based on laser-induced graphene technology" Soft Science. 3, no.2: 13. http://dx.doi.org/10.20517/ss.2023.02
ACS Style
Yue, Y.; Li X.; Zhao Z.; Wang H.; Guo X. Stretchable flexible sensors for smart tires based on laser-induced graphene technology. Soft. Sci. 2023, 3, 13. http://dx.doi.org/10.20517/ss.2023.02
Comments
Comments must be written in English. Spam, offensive content, impersonation, and private information will not be permitted. If any comment is reported and identified as inappropriate content by OAE staff, the comment will be removed without notice. If you have any queries or need any help, please contact us at support@oaepublish.com.