fig4
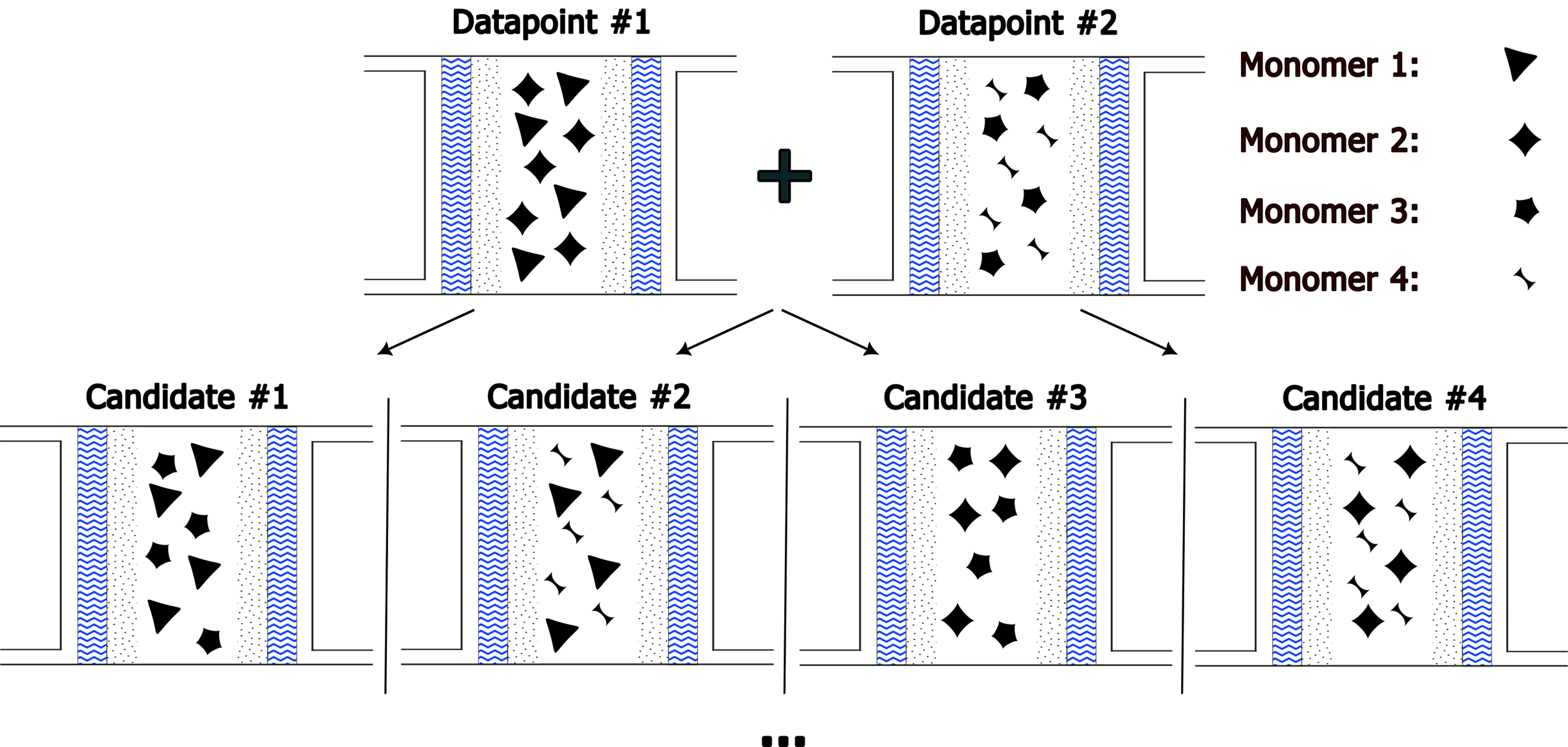
Figure 4. Illustration of the candidate generation process: unique monomer units are extracted from the training dataset and combined in various ratios to generate novel random copolymer candidates. Each candidate’s composition is varied in 10% increments, resulting in a search space of approximately 11 million candidates. The copolymers are then screened based on theoretical IEC to filter for candidates with IEC values between 0.5 and 5 meq/g. The selected candidates undergo ML-based property predictions for hydroxide conductivity, WU, and SR for further screening. IEC: Ion exchange capacity; ML: machine learning; WU: water uptake; SR: swelling ratio.