Figure3
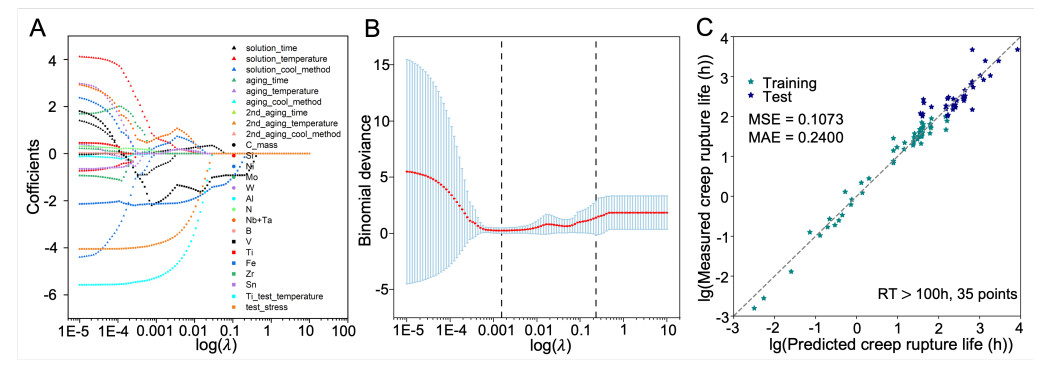
Figure 3. Feature selection via Lasso and model performance with reduced features, the data division with RT
Figure 3. Feature selection via Lasso and model performance with reduced features, the data division with RT
All published articles are preserved here permanently:
https://www.portico.org/publishers/oae/