Figure1
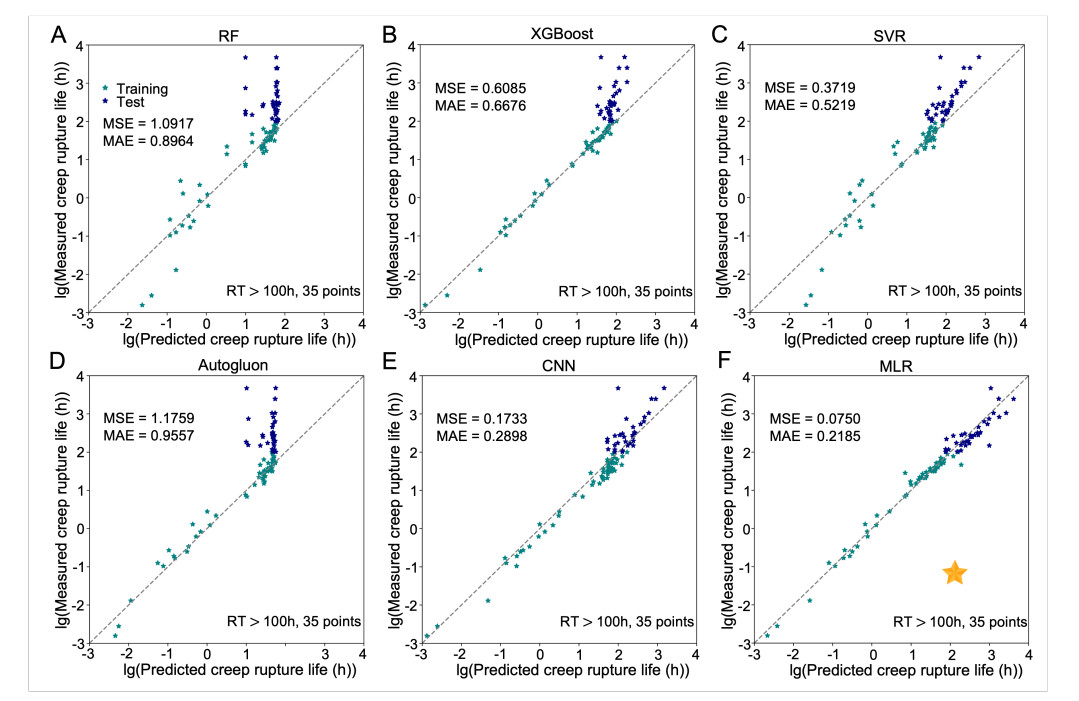
Figure 1. Extrapolation performance of the six surrogate models on both training and testing data, the data division with RT
Figure 1. Extrapolation performance of the six surrogate models on both training and testing data, the data division with RT
All published articles are preserved here permanently:
https://www.portico.org/publishers/oae/