fig5
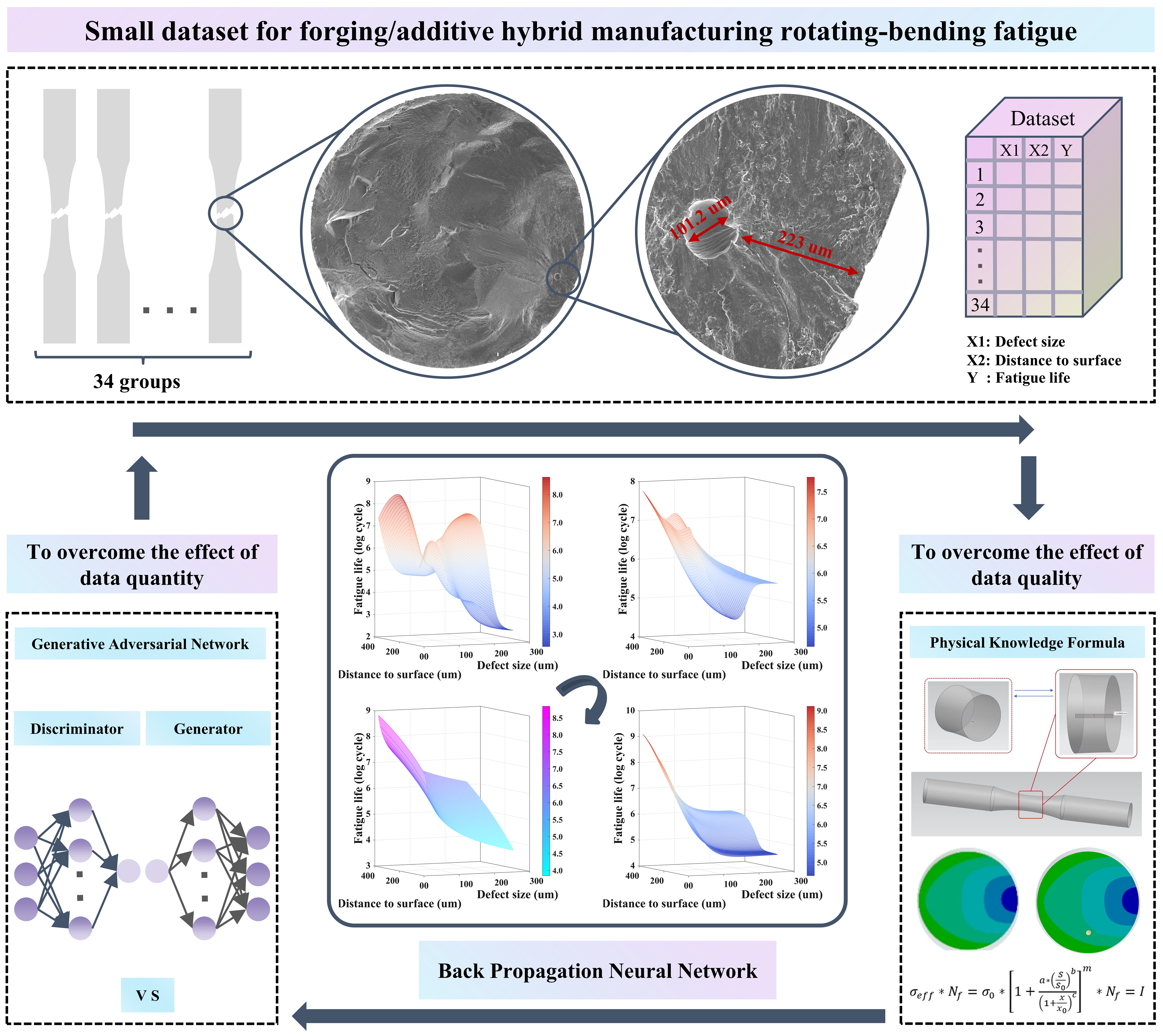
Figure 5. The overall flow chart of the present work. A total of 34 sets of defect size, defect to distance and fatigue life data were obtained from fracture photos after forging/additive components experienced rotating-bending fatigue fracture. The sparse and limited data set then prevented further analysis of the work. In order to overcome the influence of data quality, the corresponding fatigue life equation is established on the basis of physical knowledge, and it is applied to data processing and model interpretation. In order to overcome the influence of data quantity, a GAN based on Pytorch deep learning framework is constructed that conforms to the laws of defect size, distance to surface and fatigue life. Finally, a machine learning framework with physical knowledge predicts the fatigue life to be smooth by defect size and defect to distance.