fig6
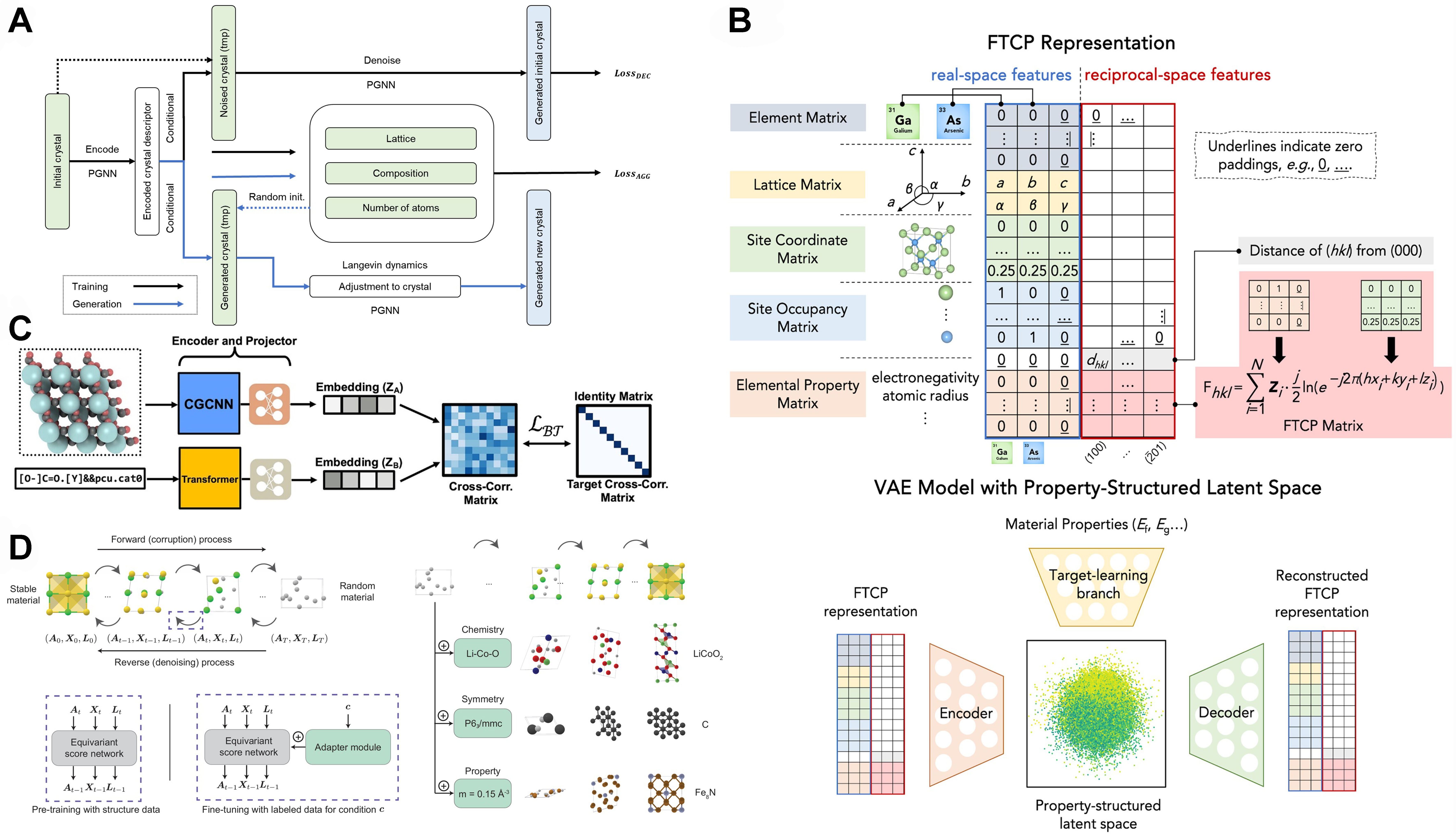
Figure 6. Encoding and embedding enhanced crystal descriptors for crystal generation tasks. (A) CDVAE model for crystalline material generation[16], using conditional denoising technique to generate new structures with requirements; (B) FTCP model using VAE framework for generating novel crystalline structures. Reprinted with permission[79], Copyright 2022, CellPress; (C) MOFormer, encoding framework integrating self-supervised learning framework with CGCNN and transformer. Reprinted with permission[52], Copyright 2023, American Chemical Society; (D) MatterGen, a diffusion model for crystal generation. Reprinted with permission from Zeni et al.[80]. CDVAE: Crystal diffusion variational autoencoder; FTCP: Fourier-transformed crystal properties; VAE: variational autoencoder; CGCNN: crystal graph convolutional neural networks.