Figure4
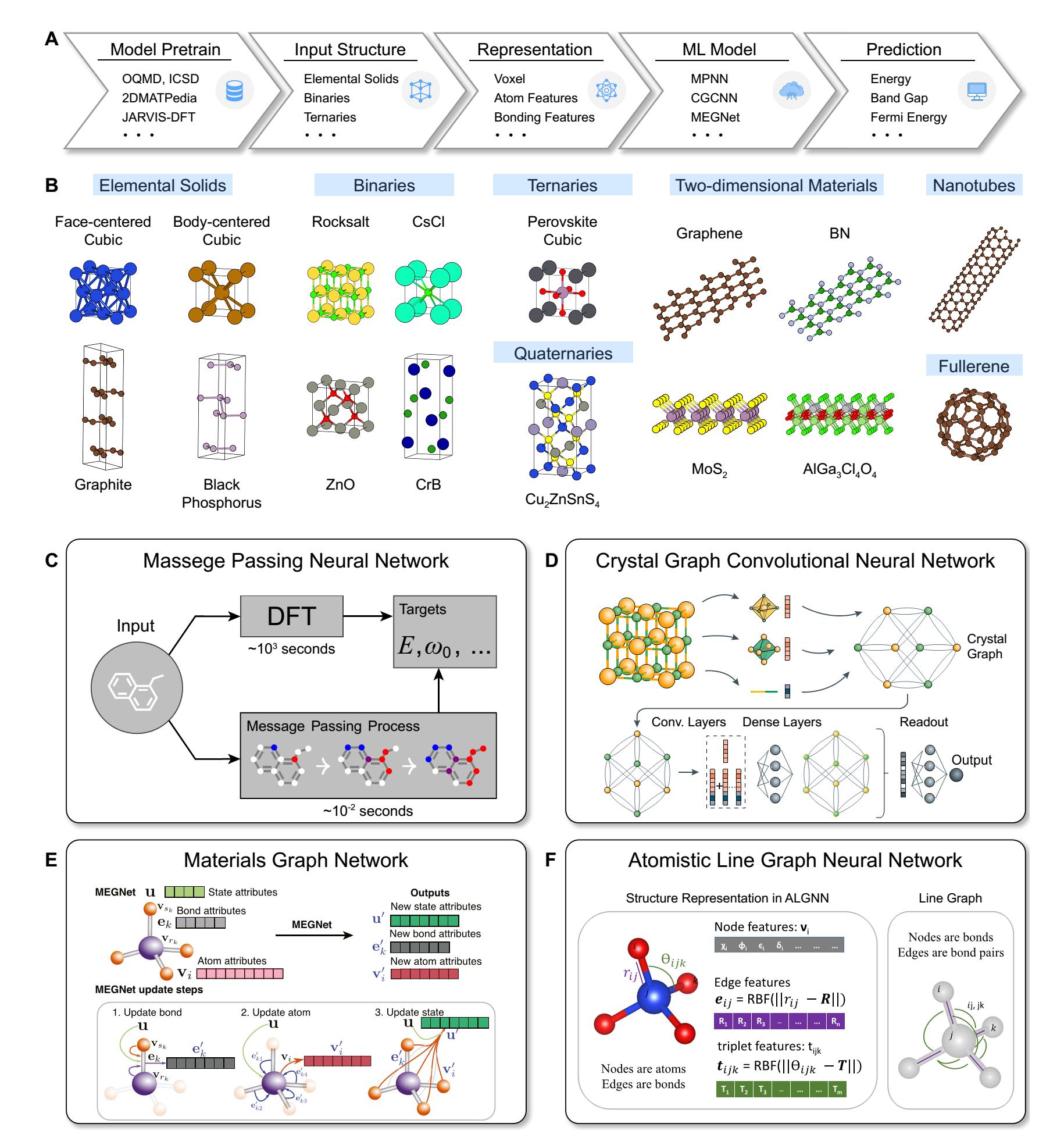
Figure 4. GNNs applied in CSP. (A) Prediction pipeline; (B) Examples of structures stored in database. Reproduced from Ref.[144]. CC BY 4.0; (C) MPNN predicts the quantum properties of an organic molecule. Reproduced from Ref.[128]. CC BY-NC 4.0; (D) Illustration of the CGCNN, including construction of the crystal graph and then building the structure of the convolutional neural network on top of the crystal graph. Reproduced with permission[107]. Copyright 2018, American Physical Society; (E) Overview of MEGNet. The initial graph is represented by the set of atomic attributes