Figure10
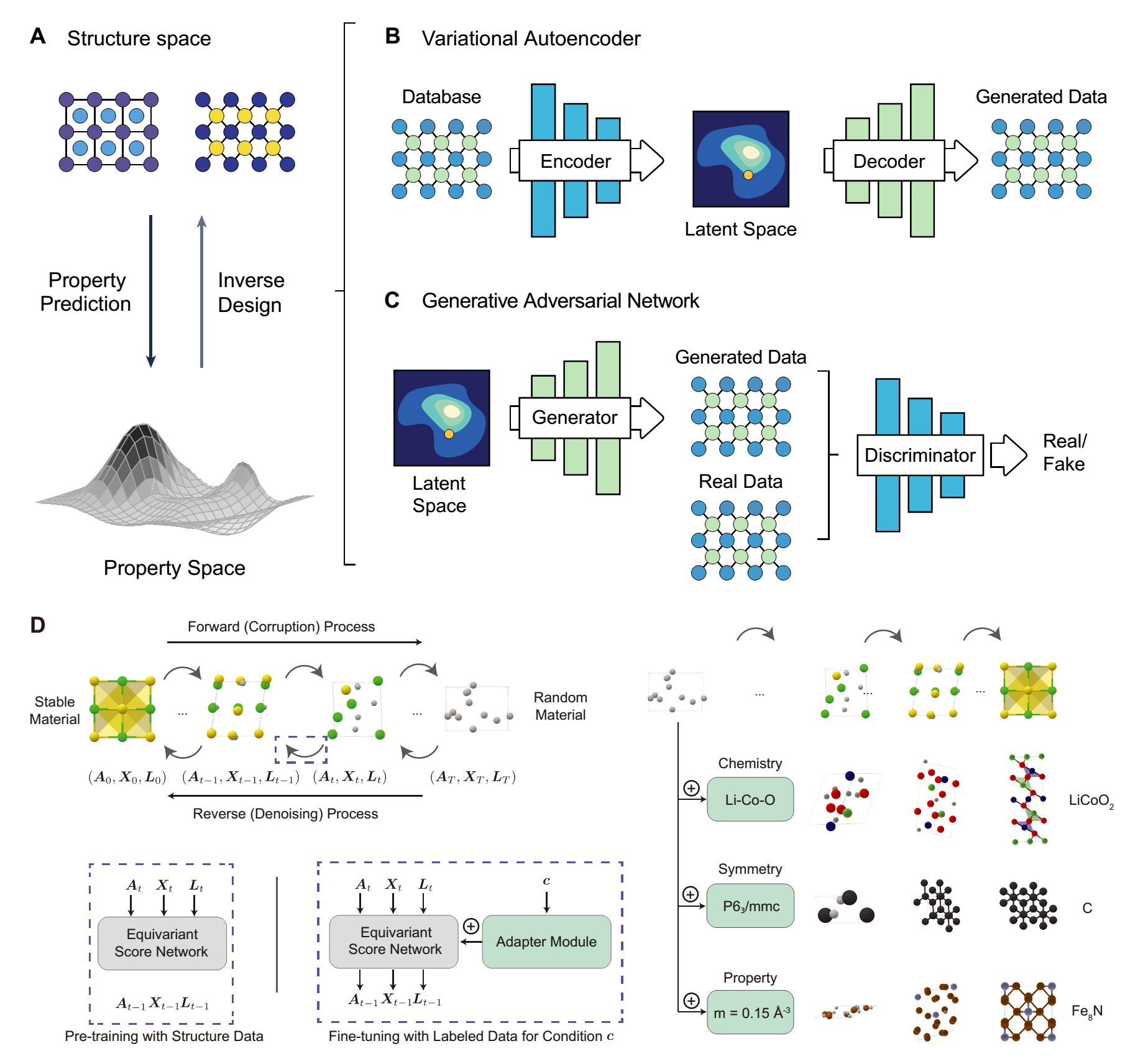
Figure 10. Material property prediction and inverse design by generative models. (A) Schematic showing material property prediction from the structure space to the property space (downward arrow), and inverse material design from the property space back to the structure space (upward arrow). Reproduced from Ref.[111]. CC BY-NC 4.0; (B) VAE. The VAE consists of an encoder that transforms the input sample feature vector to a latent distribution space, and a decoder that reconstructs the sample given the hidden distribution. The VAE also models the latent space vector