fig7
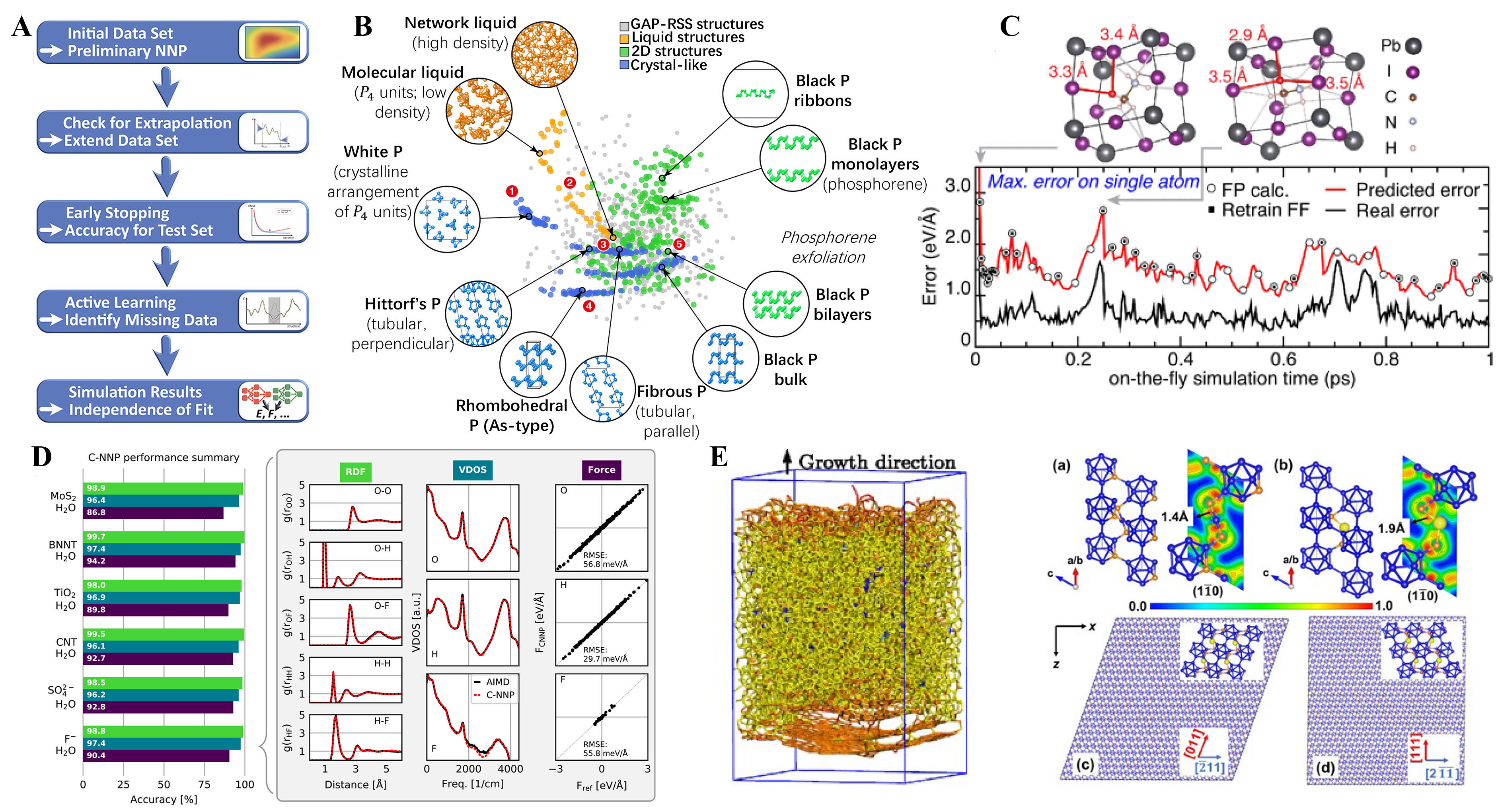
Figure 7. Development and typical application of the MLFF. (A) The MLFF constructed by beginning with an initial dataset (AIMD simulation generally). Subsequently, the MLFF is validated through a multistep process (i.e., force root mean square error (RMSE), radial distribution functions (RDFs) of the selected structures randomly). If the potential quality is deemed insufficient, problematic structures are identified and included in the training set until the final potential is achieved. Reproduced with permission[148]. Copyright 2021, American Chemical Society; (B) The MLFF fitting database for elemental phosphorus by using the many-body Smooth Overlap of Atomic Positions (SOAP) model. Reproduced with permission[148]. Copyright 2021, American Chemical Society; (C) The application of active learning molecular dynamics approach for the perovskite. The black curve represents the actual error, while the red curve represents the estimated error from MLFF. When the estimated error exceeds a certain threshold, it triggers DFT calculations to generate new data, which is then used to retrain the MLFF. The top structures highlight the hydrogen atom with the highest error in red for two different snapshots. Reproduced with permission[148]. Copyright 2021, American Chemical Society; (D)The figure on the left shows the performance assessment of the committee NNP (c-NNP) for six different systems. The figure on the right is a bar plot that summarizes the accuracy of the RDFs, VDOS, and force predictions for each system. Reproduced with permission[153]. Copyright 2021, National Academy of Science; (E) (Left) Slab model of amorphous carbon from MLFF-based simulation. (Right) Crystal structures and electron localization function (ELF) of (a) B4C and (b) Al-doped boron carbide (labeled as B12 -CAlC), and models of the B12-CAlC sliding system along different (c, d) slip directions. The blue, orange, and yellow spheres represent B, C, and Al atoms, respectively. Reproduced with permission[155]. Copyright 2018, American Physical Society. Reproduced with permission[156]. Copyright 2023, American Physical Society.