fig6
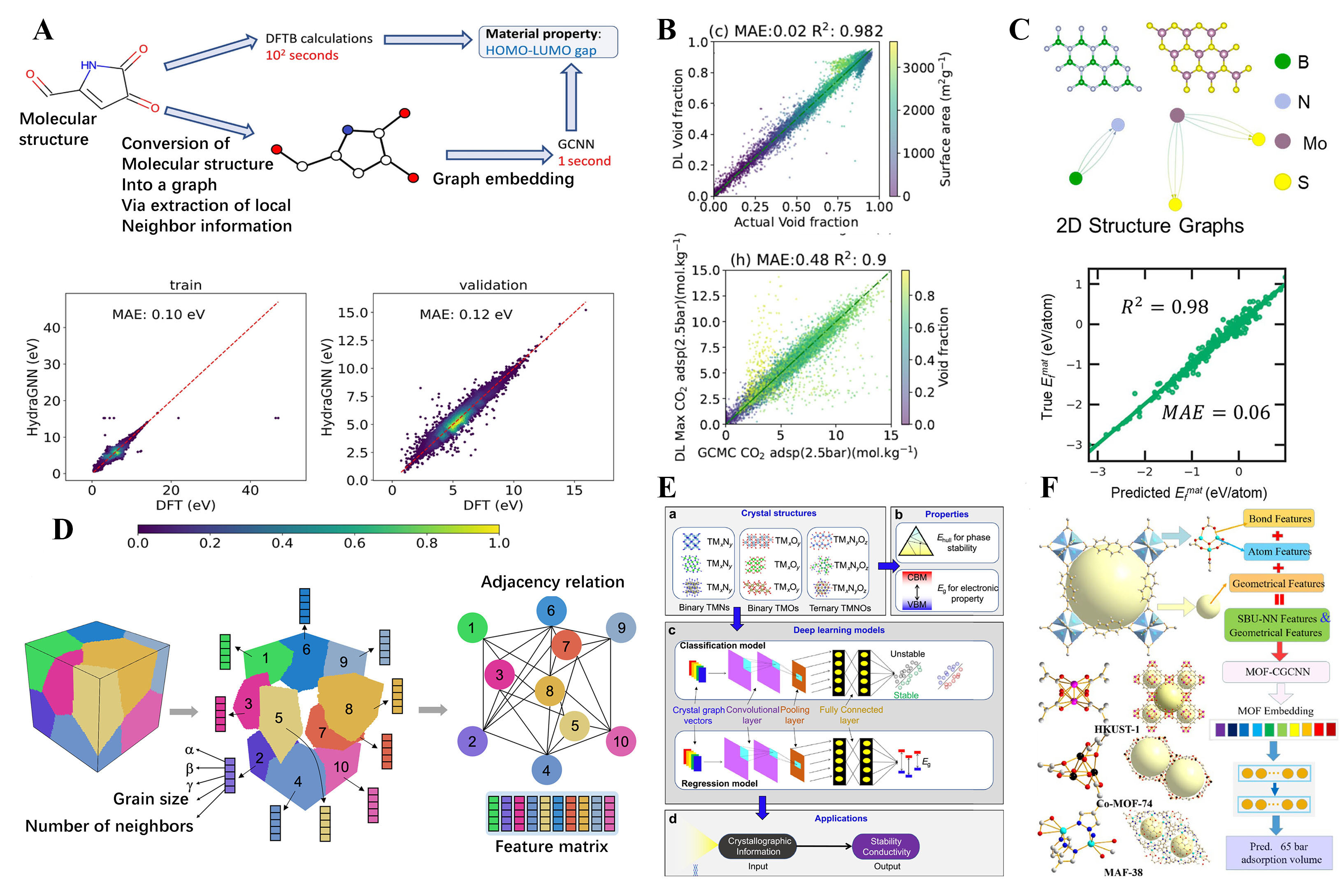
Figure 6. Selected examples of investigations based on ml-method predictions for targeted functional material design. (A) A computational workflow to predict the HOMO-LUMO gap. This workflow pits the conventional method against two alternative approaches: density functional-based tight binding (DFTB) calculations and a GCNN model. The latter leverages molecular structure as its input to estimate the HOMO-LUMO gap. Reproduced with permission[130]. Copyright 2022, BioMed Central; (B) GNN predictions and grand canonical Monte Carlo (GCMC) actual value comparisons of void fraction and adsorption at 2.5 bar using the Atomistic Line Graph Neural Network method. Reproduced with permission[130]. Copyright 2022, BioMed Central; (C) Schematic of the workflow of deep transfer learning for predicting 2D host material properties and identifying promising hosts (for example, 2D BN and 2D MoS2). Reproduced with permission[132]. Copyright 2020, American Chemical Society; (D) A graphical representation of a polycrystalline microstructure composed of N grains. Reproduced with permission[110]. Copyright 2021, Springer Nature Limited; (E) The proposed workflow diagram of a visual interactive software (DeepTMC) to targeted design doped transition metal compounds. Reproduced with permission[133]. Copyright 2022, Elsevier; (F) Proposed crystal graph convolutional neural network (CGCNN) method for CH4 adsorption in MOFs. Reproduced with permission[134]. Copyright 2022, Elsevier.