fig7
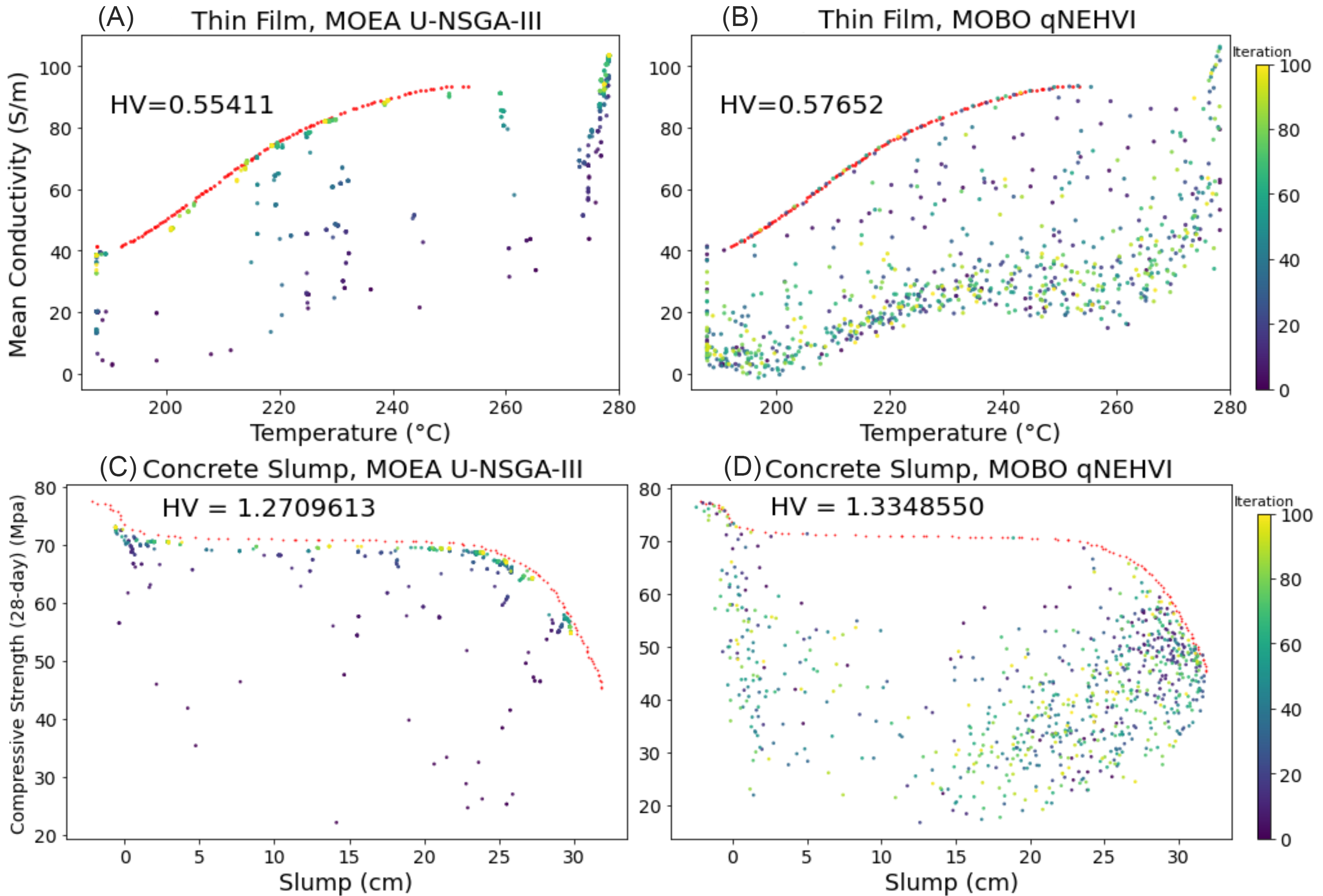
Figure 7. Optimization trajectory in objective space for a single run of 100 iterations × 8 points per batch. (A and B) Thin Film; (C and D) Concrete Slump. Across objective space for a single run of 100 iterations × 8 points per batch. The red line represents the PF. PFs for real-world datasets were virtually generated using NSGA-II for 500 generations with a population size of 100. The color of each experiment refers to the number of iterations. The results here corroborate the “wastage” of solutions in qNEHVI, although which algorithm is superior appears to be problem dependent. HV: hypervolume; MOBO: multi-objective Bayesian optimization; MOEA: multi-objective evolutionary algorithms; PF: Pareto Front; qNEHVI: q-Noisy Expected Hypervolume Improvement; U-NSGA-III: Unified Non-dominated Sorting Genetic Algorithm III.