fig15
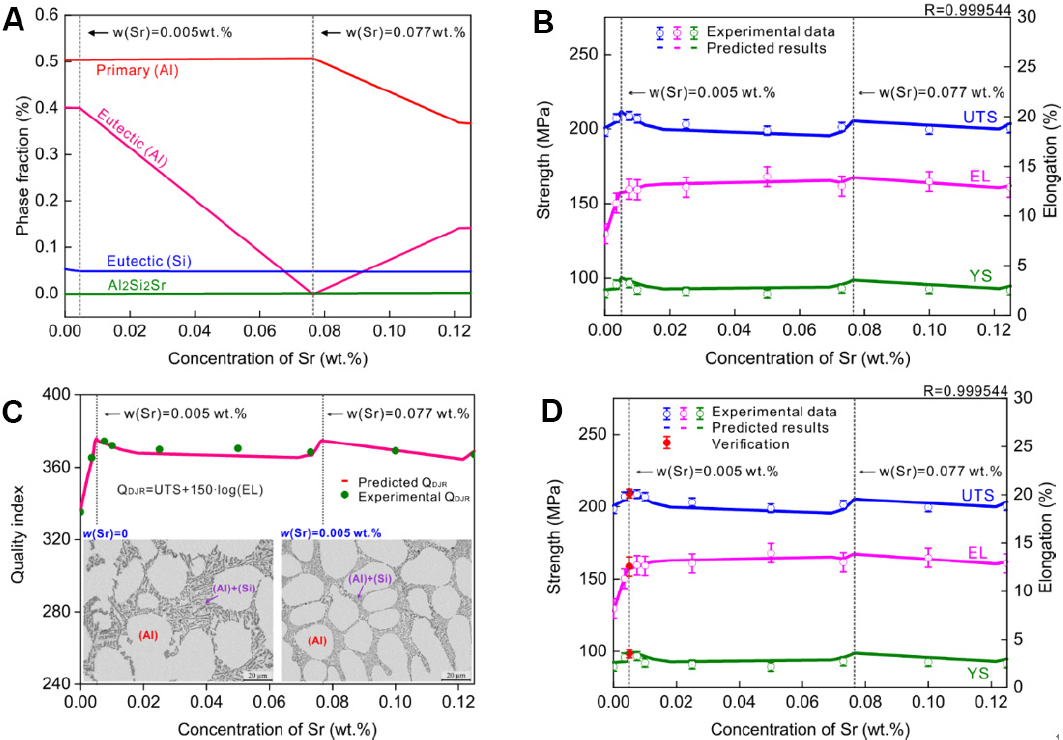
Figure 15. Alloy design of Sr-modified A356 alloys driven by combing computational thermodynamic and machine learning techniques. (A) Fraction of different phases/structures in Sr-modified A356 obtained by CT; (B) measured and training mechanical properties of A356-xSr alloys. (C) Quality index [QDJR = UTS + 150·log(EL)] of Sr-modified A356 alloys computed from the predicted and the experimental data. (D) Experimental verification of the predicted optimal Sr additional content (i.e., 0.005 wt%) and corresponding best mechanical properties. Figure reproduced from Ref.[168] with permission from Elsevier.