fig1
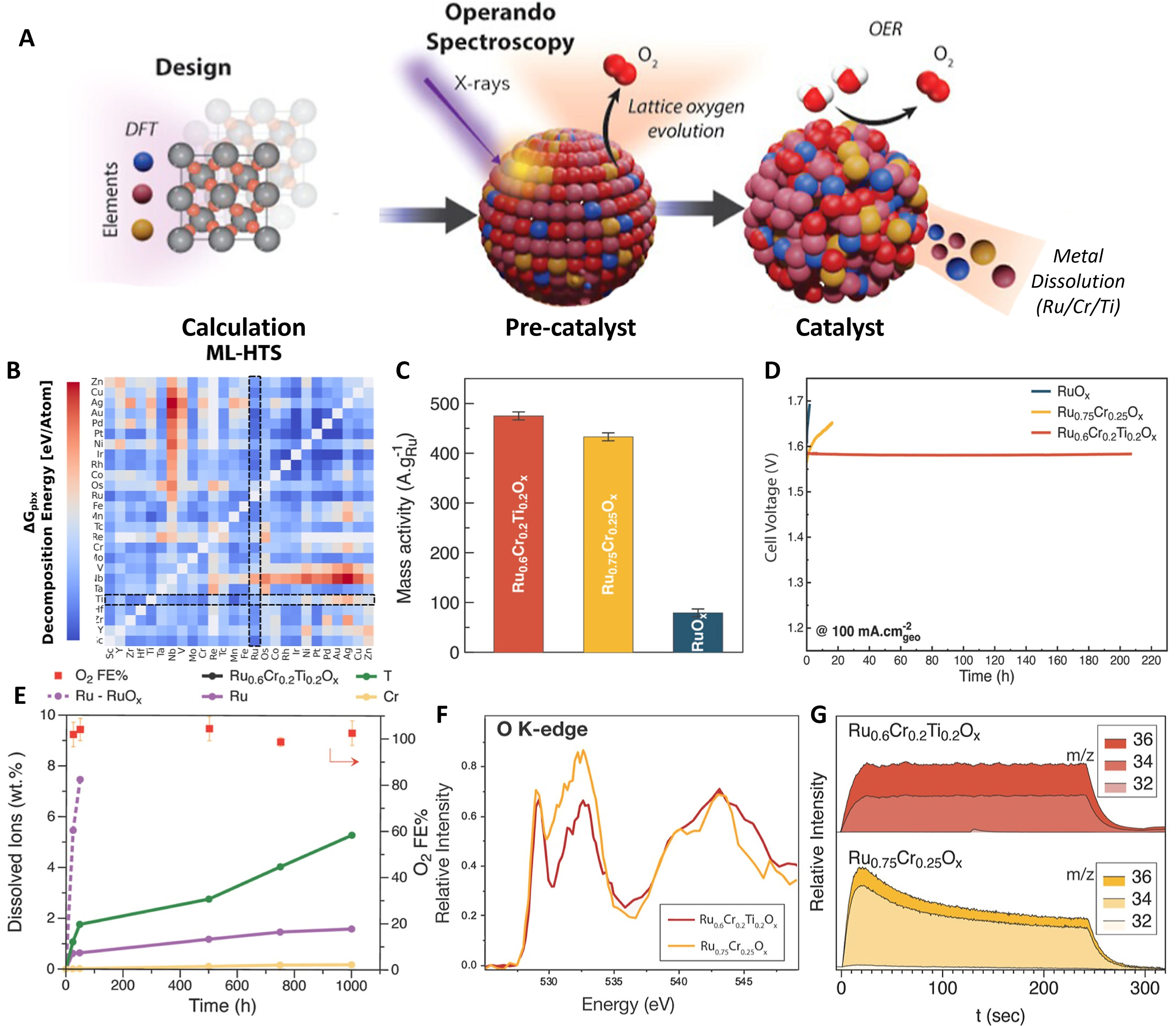
Figure 1. ML-aided framework identifies Ru-based acidic water oxidation catalysts. (A) Schematic of the design process of Ru-based catalysts from the ML-aided calculation model to the real catalyst; (B) The model named CGCNN-HD’s heatmaps of Pourbaix electrochemical stability (ΔGpbx) for the subset; (C) Mass activity at 1.48 V vs. RHE for Ru0.6Cr0.2Ti0.2Ox, Ru0.75Cr0.25Ox, and RuOx; (D) Stability test at a constant current density of 100 mA·cmgeo-2; (E) Accumulative total dissolved ions for RuOx and Ru0.6Cr0.2Ti0.2Ox; (F) O K-edge STXM spectroptychography XANES comparison between Ru0.75Cr0.25Ox and Ru0.6Cr0.2Ti0.2Ox; (G) DEMS signals of the reaction products using a H218O aqueous sulfuric acid electrolyte. This figure is quoted with permission from Abed et al.[9]. ML: Machine learning; CGCNN-HD: crystal graph convolutional neural network modified with a hyperbolic tangent activation function and dropout algorithm; RHE: reversible hydrogen electrode; STXM: scanning transmission X-ray microscopy; XANES: X-ray absorption near-edge structure; DEMS: differential electrochemical mass spectroscopy measurements.