Blood-based sex-differential DNA methylation in Alzheimer’s disease
Abstract
Aim: We aim to explore the blood-based sex-differential DNA methylation profiles in patients with Alzheimer’s disease (AD).
Methods: The same analytical pipeline and meta-analysis procedure were applied to 254 patients with AD and 261 matched healthy controls (HC) from four blood-based datasets to identify sex-differentially methylated positions (sex-DMPs) and their biological functions. We further reviewed brain-based sex-DMPs previously reported in AD and intersected with blood-based sex-DMPs.
Results: We identified 134 sex-DMPs in AD, 88 were unique to AD, and 46 were shared with HC. Eleven novel sex-DMPs and 28 sex-DMPs consistent across blood and brain were recognized for the first time. Differentially methylated genes, such as COL25A1, MSUT2, ELAVL4, SLC17A7, EPHA4, and PPP2CB, were closely related to the pathogenesis of AD. In addition, immune-related processes were enriched in the enrichment analysis.
Conclusion: Sex-differential DNA methylation in blood provides potential biomarkers for AD pathogenesis, and also points to potential drug targets for precision medicine.
Keywords
INTRODUCTION
Alzheimer’s disease (AD) is a progressive neurodegenerative disease and the leading cause of dementia in older adults. AD is characterized by a gradual decline in memory, behavioral, and social skills, significantly impacting daily life and functioning. With the global population aging, the socioeconomic burden of AD is escalating sharply[1,2]. Notably, pronounced sex differences are evident in AD risk, prevalence, and clinical presentation[3,4]. Women face a higher risk of developing AD, and more women than men are affected by dementia, with an estimated 27.0 million women compared to 16.8 million men living with dementia in 2016 (age-standardized)[2]. Clinical manifestations also vary substantially between males and females, particularly regarding neuropsychiatric symptoms[3,4]. However, the molecular mechanisms underlying these sex differences in AD remain largely unknown.
DNA methylation is an important form of epigenetic modification, which plays a key role in determining genomic structure and function, regulating gene expression and cell differentiation without altering the DNA sequence[5]. Environmental factors throughout life can drive DNA methylation changes, some of which exhibit sex-specific patterns[6-9]. Sex differences in DNA methylation have gained attention across various life stages, from newborns to the elderly, as well as in diseases such as cancers, psychiatric disorders, and neurodegenerative diseases[6-13]. Studies regarding sex-related differential methylation in AD have primarily focused on brain tissue[12,13], while peripheral blood has received relatively little attention, despite the tissue-specific nature of DNA methylation[14-16]. One study approached this gap by using sex-stratified and methylation-by-sex interaction analyses to detect DNA methylation differences in peripheral blood from AD patients, though it did not directly compare male and female AD patients[17].
Therefore, further elucidating blood-based, sex-differential DNA methylation will enhance our understanding of sex differences in AD. Additionally, integrated analyses of sex-related DNA methylation patterns across both brain and blood tissues in AD patients may reveal valuable epigenetic biomarkers, offering insights into the disease’s progression and potential therapeutic targets.
METHODS
Study cohorts
To systematically explore blood-based sex-differential DNA methylation profiles in AD, we obtained data from 254 patients with AD and 261 healthy controls (HC) from three independent datasets: the Australian Imaging, Biomarker & Lifestyle (AIBL) cohort (GSE153712, the Nabais dataset containing 161 AD patients)[18], the AddNeuroMed cohort (GSE144858, the Lunnon dataset containing 93 AD patients and 96 HC)[19], and the third cohort was previously described by Li et al. (GSE53740, containing 165 HC)[20]. Patients with AD were diagnosed according to the NINCDS-ADRDA criteria[21]. Age and sex for AD and HC were matched individually.
Data pre-processing
DNA methylation in the peripheral blood of all participants was measured using the Infinium Human Methylation 450K Bead-Chip, except for the AIBL cohort[18], where the Infinium Human Methylation EPIC (850K) Bead-Chip was applied. We used the R package ChAMP[22] (version 2.21.1) to process the β-value matrix for each dataset separately. Probes with a β value of 0 were replaced with 1.00 × 10-6, and probes with missing β values were imputed using the k-nearest neighbor algorithm by impute.knn function in impute package[23]. Quality control (QC) was performed using the champ.filter function[24]. Probes meeting the following criteria were removed: (1) probes with detection P < 0.01 in one or more samples; (2) probes with < 3 beads in at least 5% of samples per probe; (3) non-CpG probes as identified by Chen et al.[25]; (4) probes located close to single nucleotide polymorphism (SNP) (an SNP with minor allele frequency ≥ 0.01 was present in the last five base pairs of the probe); (5) multi-hit probes as identified by Zhou et al.[24]; (6) probes located on X and Y chromosomes. The number of probes filtered at each QC step is listed in Supplementary Table 1. We then applied the β-mixture quantile normalization (BMIQ) method[26] to normalize the β values of type 1 and type 2 design probes within the Illumina arrays. A quantile-quantile (QQ) plot was generated and the genomic inflation factor (λ) was estimated for each dataset using the R qqman package[27].
Sex-differentially methylated positions and regions
The remaining probe data were compared between males and females to identify sex-differentially methylated positions (sex-DMPs) using the limma package[28], as implemented in the champ.DMP function. A multiple-testing adjustment was performed using the Benjamini-Hochberg false discovery rate (FDR) method[29]. We selected probes with FDR under a threshold of 0.1 (adjusted P value < 0.10) for further meta-analysis, given the different platforms (450K and 850K microarrays) of the two AD datasets. Differentially methylated regions (DMRs) were extended segments of the genome that showed quantitative alterations in DNA methylation levels between the two groups. Sex-DMRs were detected by applying the Bumphunter algorithm[30] implemented in the champ.DMR function, which clustered the groups containing more than seven continuous probes as DMR (FDR < 0.05).
Meta-analysis
Sample size weighted meta-analysis for common DMPs across individual AD cohorts was conducted in METAL, converting the direction of effect and adjusting the P value into a signed Z-score[31]. The same meta-analysis procedure was performed for HC. To further characterize the results, we then intersected the meta-analysis outcomes of AD and HC to identify the unique sex-DMPs in AD and the shared sex-DMPs in both AD and HC.
Gene set enrichment analysis
Before enrichment analysis was performed, significant DMPs in the meta-analysis were annotated according to their locations with respect to genes (TSS1500, TSS200, 5’UTR, 1stExon, body, 3’UTR, and intergenic) or CpG islands (island, shore, shelf, and open sea), using the IlluminaHumanMethylation450kanno.ilmn12.hg19 package. Kyoto Encyclopedia of Genes and Genomes (KEGG) pathway analysis was conducted for these differentially methylated genes using the KOBAS-i
Comparison to previous brain tissue-based sex-DMPs
Recent studies have suggested sex-specific differences in DNA methylation in the brain tissues of patients with AD. Pellegrini et al. identified 77 sex-dependent DMPs consistent across four brain regions (frontal cortex, temporal cortex, entorhinal cortex, and cerebellum)[13]. Additionally, Zhang et al. found that 381 CpGs in females and 76 CpGs in males were significantly associated with the AD Braak stage by analyzing more than 1,000 postmortem prefrontal cortex brain samples[12]. To assess the consistency of sex-DMPs in the brain and peripheral blood, we intersected blood-based DMPs identified in our meta-analysis and brain-based DMPs reported by Pellegrini et al. and Zhang et al.[12,13].
RESULTS
Study cohort characteristics
The characteristics of participants from the four datasets are presented in Supplementary Table 2. Our meta-analysis included 254 AD patients [153 females, 101 males; mean age 74.7 years with a standard deviation (SD) of 6.9 years] and 261 matched HC (161 females, 100 males; mean age 70.1 years with an SD of 8.1 years). The overview of study design is shown in Figure 1.
Sex-DMPs and regions
After data pre-processing, QC and normalization of DNA methylation, the number of autosomal probes used for analyses in each dataset ranged from 262K to 384K, except for the AIBL cohort (EPIC array), for which 747K probes passed QC [Supplementary Table 1]. No evident inflation of the test statistics was observed in the AD datasets [Figure 2]. Significant sex-DMPs at FDR < 0.10 identified in the individual dataset are shown in Supplementary Tables 3-6 and Figure 3A. A total of 216 sex-DMPs were common to both HC datasets, and 134 sex-DMPs were common to both AD datasets. A Venn diagram of the DMPs obtained from the four datasets is shown in Figure 3A.
Figure 2. QQ plots of observed and expected distributions of P-values for each dataset. λ is the genomic inflation factor. QQ: Quantile-quantile.
Figure 3. Discovery and annotation of sex-DMPs in AD. (A) Venn diagram of sex-DMPs obtained by four datasets; (B) Annotation in gene features (TSS1500, TSS200, 5’UTR, 1stExon, body, 3’UTR, intergenic) and CpG features (island, shore, shelf, open sea) of 134 sex-DMPs identified in AD meta-analysis. AD: Alzheimer’s disease; sex-DMPs: sex-differentially methylated positions; HC: healthy controls.
Six sex-DMRs were significant in both AD datasets, but not in any HC dataset [Supplementary Table 7, Supplementary Figures 1-6]. The average number of CpGs per DMR was 7.7 ± 0.5. Six genes (PM20D1, LOC441666, AURKC, PCDHGA4, H2AFJ, CLDN9) were mapped to the above six sex-DMRs. Among these, PCDHGA4 and H2AFJ were hypermethylated in males compared to females, and the remaining four genes were hypomethylated in males compared to females. We found no CpGs overlapped between 134 sex-DMPs and six sex-DMRs in AD, suggesting complementary evidence from DMPs and DMRs for sex-associated differences in DNA methylation in AD.
Meta-analysis
To prioritize unique sex-DMPs in AD, we conducted meta-analysis for AD and HC, respectively. For the convenience of classification, we defined the DMP with higher methylation levels in males than in females as “hypermethylated” and “hypomethylated” otherwise. Our meta-analysis identified 216 sex-DMPs [44 (20.4%) hypermethylated and 172 (79.6%) hypomethylated] in HC [Supplementary Table 8] and 134 sex-DMPs [40 (29.9%) hypermethylated and 94 (70.1%) hypomethylated] in AD [Supplementary Table 9]. Compared with HC, 88 sex-DMPs were unique in AD (defined as AD-sex-DMPs), whereas 46 sex-DMPs were shared in both AD and HC (defined as AD-HC-sex-DMPs) [Supplementary Table 10].
With annotation, the AD-sex-DMPs were mapped to 52 genes that contained 12 (23.1%) hypermethylated and 40 (76.9%) hypomethylated genes [Supplementary Table 9]. The AD-HC-sex-DMPs were mapped to 28 genes: 9 (32.1%) were hypermethylated, and 19 (67.9%) were hypomethylated, which also showed a similar pattern of lower rates of hypermethylated genes in males than in females. In terms of gene features, the hypermethylated CpGs in males were enriched in intergenic regions, gene body, and TSS200 but under-represented in 3’UTR, and the hypomethylated CpGs were enriched in intergenic regions, TSS1500, and gene body [Figure 3B and Supplementary Table 9]. Regarding CpG features, both hypermethylated and hypomethylated CpGs were overrepresented in CpG island and shore [Figure 3B and Supplementary Table 9]. Similar distribution characteristics were observed in the AD-sex-DMPs and AD-HC-sex-DMPs
Specifically, 11 novel CpGs (cg18355337, hypomethylated, P = 5.08 × 10-5; cg24592500, hypomethylated, P = 1.25 × 10-4; cg22364668, hypermethylated, P = 1.60 × 10-4; cg19653417, hypomethylated, P = 4.63 × 10-4; cg17394530, hypomethylated, P = 5.84 × 10-4; cg05697849, hypermethylated, P = 0.002; cg19893929, hypermethylated, P = 0.004; cg12873476, hypomethylated, P = 0.006; cg12964741, hypermethylated, P = 0.006; cg07592361, hypermethylated, P = 0.008; cg13772414, hypermethylated, P = 0.015) with sex specificity were identified for the first time [Table 1], based on trait records in the epigenome-wide association studies (EWAS) Atlas (https://ngdc.cncb.ac.cn/ewas/atlas), an updated public EWAS knowledgebase covering more than 617 018 high-quality EWAS associations[34]. The other 91.8% of CpGs (77/88 in AD-sex-DMPs and 46/46 in AD-HC-sex-DMPs) [Supplementary Table 10] were consistently replicated in direction with previous sex-related EWAS results of health population[6-9,35]. Impressively, among genes mapped to these 11 novel CpGs, ELAVL4, SLC17A7, and EPHA4 were all hypermethylated in males and were closely related to AD based on search records in the PubMed [Alzheimer’s disease (All Fields) AND “Gene Name” (All Fields)]. The details are developed in the discussion section.
Eleven novel AD-sex-DMPs
CpG | Chr | Position | Gene | Gene features | CpG features | Direction | P value |
cg18355337 | 19 | 55549722 | GP6 | TSS200 | Opensea | -- | 5.08E-05 |
cg24592500 | 1 | 2863801 | IGR | Opensea | -- | 0.0001248 | |
cg22364668 | 19 | 49944826 | SLC17A7 | TSS200 | Shore | ++ | 0.0001598 |
cg19653417 | 12 | 132654924 | IGR | Shore | -- | 0.0004633 | |
cg17394530 | 6 | 106434623 | IGR | Shore | -- | 0.0005838 | |
cg05697849 | 1 | 50513766 | ELAVL4 | 1stExon | Island | ++ | 0.00232 |
cg19893929 | 2 | 16105823 | IGR | Opensea | ++ | 0.004802 | |
cg12873476 | 8 | 142402728 | IGR | Shore | -- | 0.005545 | |
cg12964741 | 18 | 77160221 | NFATC1 | Body | Island | ++ | 0.006405 |
cg07592361 | 2 | 241496830 | ANKMY1 | 5’UTR | Island | ++ | 0.00872 |
cg13772414 | 2 | 222383060 | EPHA4 | Body | Opensea | ++ | 0.01497 |
Enrichment and pathway analysis implicates immune-related processes
To investigate the potential biological pathways enriched with significant sex-differentially methylated genes unique to AD, KEGG pathway enrichment analysis was conducted. For 52 genes mapped from 88 AD-sex-DMPs, we identified 53 significant terms (at FDR < 0.25[33]), which were grouped into six clusters based on the correlation among gene sets [Figure 4 and Supplementary Table 11]. The immune-related cluster (cluster 5) was independent of the other clusters [Figure 4] and contained multiple aspects of immunity, such as inflammatory bowel disease (P = 0.062, FDR = 0.187), B cell receptor signaling pathway (P = 0.077, FDR = 0.187), Th1 and Th2 cell differentiation (P = 0.086, FDR = 0.187), T cell receptor signaling pathway
Figure 4. cirFunMap visualization of KEGG pathway enrichment analysis for 52 genes mapped from 88 AD-sex-DMPs. (A) The circular network view (the node color represents different clusters and the node size represents six levels of P-value) showed the immune-related cluster (cluster 5) was enriched and independent from the other clusters; (B) The barplot of the P-value for terms in different clusters. AD: Alzheimer’s disease; sex-DMPs: sex-differentially methylated positions; KEGG: Encyclopedia of Genes and Genomes.
Comparison to previous brain tissue-based sex-DMPs
We intersected the 134 blood-based sex-DMPs of AD in our meta-analysis and the 77 brain-based sex-dependent DMPs of AD reported by Pellegrini et al.[13], identifying 28 common probes with the same sex-independent direction of changes (hyper or hypomethylated) across the blood and brain [Table 2]. Among these, 14 were classified as the AD-sex-DMPs, and the other 14 were classified as the AD-HC-sex-DMPs
Twenty-eight sex-DMPs exhibiting consistent methylation changes in both blood and brain
CpG | Chr | Position | Gene | Gene features | CpG features | Direction | P value |
cg23719534 | 15 | 101099284 | IGR | Island | -- | 1.13E-110 | |
cg22345911 | 17 | 80231263 | CSNK1D | 5’UTR | Island | -- | 2.80E-33 |
cg13346869 | 8 | 37605517 | LOC728024 | Body | Opensea | -- | 1.59E-28 |
cg22266749 | 4 | 110223959 | COL25A1 | TSS200 | Island | ++ | 7.06E-25 |
cg23001456 | 17 | 2615074 | KIAA0664 | TSS200 | Island | -- | 7.30E-20 |
cg22794378 | 14 | 89029563 | ZC3H14 | TSS200 | Island | -- | 4.28E-18 |
cg15817705 | 1 | 209406063 | IGR | Shore | ++ | 1.57E-15 | |
cg27645294 | 17 | 21795257 | IGR | Opensea | -- | 1.14E-14 | |
cg10546176 | 5 | 34929404 | DNAJC21 | TSS1500 | Island | -- | 1.52E-11 |
cg05020125 | 8 | 37605552 | LOC728024 | TSS200 | Opensea | -- | 9.52E-10 |
cg26516287 | 7 | 12629275 | SCIN | Body | Opensea | -- | 3.88E-08 |
cg17561891 | 7 | 86849173 | C7orf23 | TSS200 | Island | -- | 2.41E-07 |
cg22105158 | 19 | 3480672 | C19orf77 | TSS200 | Shore | ++ | 1.51E-05 |
cg19311244 | 4 | 77341912 | IGR | Shore | -- | 0.0002931 | |
cg04946709 | 16 | 59789030 | LOC644649 | Body | Island | ++ | 1.19E-85 |
cg05100634 | 18 | 45457604 | SMAD2 | TSS200 | Island | -- | 1.16E-72 |
cg06710937 | 13 | 23489940 | IGR | Island | -- | 9.23E-53 | |
cg09971754 | 16 | 89557657 | ANKRD11 | TSS1500 | Island | ++ | 1.23E-32 |
cg09725915 | 2 | 70369583 | IGR | Island | -- | 5.02E-28 | |
cg14030268 | 10 | 119135296 | PDZD8 | TSS1500 | Island | -- | 1.58E-22 |
cg03894796 | 8 | 144361315 | IGR | Island | ++ | 5.37E-12 | |
cg15148078 | 19 | 3480561 | C19orf77 | TSS200 | Shore | ++ | 6.01E-07 |
cg02530860 | 8 | 144371537 | IGR | Island | ++ | 7.30E-07 | |
cg11841231 | 2 | 205543309 | PARD3B | Body | Opensea | ++ | 1.01E-06 |
cg06666376 | 19 | 3480596 | C19orf77 | TSS200 | Shore | ++ | 1.25E-05 |
cg11174255 | 4 | 1513259 | IGR | Shore | ++ | 0.0002916 | |
cg11565911 | 12 | 72233249 | TBC1D15 | TSS1500 | Shore | -- | 0.007048 |
cg07645761 | 16 | 2892518 | TMPRSS8 | Body | Shore | ++ | 0.01495 |
There was no overlap between our 134 sex-DMPs and 457 sex-stratified CpGs of the brain reported by Zhang et al.[12] The former was DMPs between male and female patients, while the latter offered AD Braak stage-associated differentially methylated CpGs in male and female patients, respectively. Not surprisingly, no overlapped DMPs were identified due to the focus on different aspects of sex on AD and the use of different analysis methods.
DISCUSSION
Blood-based epigenetic biomarkers for AD have gained significant attention in recent years[43-45]. Although sex differences have been of interest in AD-related studies, they have often been overlooked as key factors influencing patient responses to treatments in clinical trials, typically treated as covariates or subgroups[46]. In this study, we utilized DNA methylation datasets of AD and HC to identify sex-differential DNA methylation profiles in AD, using a three-step approach. Overall, 88 AD-sex-DMPs and 46 AD-HC-sex-DMPs were identified. Of these, 91.8% had been previously reported in healthy populations[6-9,35], while 11 were identified for the first time in AD. Notably, 28 sex-DMPs showed consistent methylation patterns across both brain and blood. Our findings highlight specific genes, genomic regions, and immune-related processes with sex-differential DNA methylation, which may serve as potential modifiers of AD progression.
To minimize the influence of age on DNA methylation[7], we included age-matched controls to identify sex-differential methylation patterns in AD. We observed higher methylation levels in females than in males, both in AD (70.1%) and in HC (79.6%), consistent with prior blood-based studies in the healthy population[7,8]. Similarly, higher methylation levels in females (73%) were noted in brain tissues of AD patients[13]. These findings underscore the substantial sex differences in DNA methylation observed in both blood and brain, highlighting the need for further investigations and emphasizing the importance of considering sex differences in future research designs and analyses.
In addition to corroborating previous findings of sex-related differences in DNA methylation, our meta-analysis identified several novel sex-differential methylated genes in AD. For example, ELAVL4 (cg05697849 at gene promoter, hypermethylated in males), which encodes a neuronal-specific RNA binding protein involved in neural development and glutamate regulation, was linked to AD pathogenesis[47,48]. ELAVL4 has also been implicated in accelerating tau pathology and glutamatergic neuron maturation through aberrant splicing[49]. Furthermore, the expression of the ELAVL4 gene was different between males and females in the brain tissue of AD patients[47]. Another notable gene, SLC17A7/VGLUT1 (cg22364668 at gene promoter, hypermethylated in males), regulates synaptic glutamate transport and long-term potentiation[50,51], and interacts with the Aβ precursor[52]. EPHA4 (cg13772414 at gene body, hypermethylated in males) modulates Aβ production[53,54], with its downregulation leading to increased Aβ and BACE1 expression[54]. PPP2CB (cg26631144 at gene promoter, hypomethylated in males), encoding the protein phosphatase 2A catalytic subunit, has been identified as a key protein in the protective effect of apolipoprotein E ɛ2 against AD[55]. These findings are promising; however, further investigation is needed to elucidate the functional roles of these methylated genes in AD progression. It is also essential to determine whether these novel DMPs can serve as biomarkers for early diagnosis or prognosis in AD. Additionally, analyzing the relationship between DNA methylation changes and gene expression will help better understand the clinical relevance of these epigenetic modifications.
These sex-differential methylations may reveal molecular mechanisms underlying sex disparities in AD. Our enrichment analysis highlighted immune processes modified by DNA methylation showed sex differences. Many studies have strongly implicated a critical role played by immune dysregulation and neuroinflammation in the pathogenesis of AD[56]. In fact, evidence from clinical[57] and basic[58] studies also supports sex-specific differences contributing to this complexity. A gene expression meta-analysis revealed consistent immune signatures in the blood and brain in female AD patients but was absent in males[57]. Longitudinal studies showed that compared with male 5xFAD mice, female 5xFAD mice displayed an earlier and more robust induction of multiple inflammatory mediators such as CXCL10, CCL2, IL-1β, and TNFα[58]. These findings underscore the need for integrating omics analyses to better understand sex differences in AD[46].
Our results, which compare blood-brain methylation and previous cross-tissue DNA methylation analyses[16,17], indicated that DNA methylation changes are not entirely consistent between blood and brain tissue. Considering the tissue-specific nature of DNA methylation, future studies simultaneously analyzing blood and brain tissue methylation changes will help to comprehensively evaluate the potential of blood-based DNA methylation as an AD biomarker, and uncover the association of DNA methylation alterations between blood and brain tissue in AD.
This study has several limitations. First, although we applied the same analytical pipeline and meta-analysis procedure for matched AD and HC groups, we were unable to fully adjust for potential confounders, such as lifestyle factors and chronic diseases (e.g., diabetes), which may affect DNA methylation patterns. Therefore, our findings should be interpreted with caution. Validation in future studies with larger sample sizes and more rigorous control for these confounders is needed. Second, insufficient clinical data (disease duration and degree of dementia at the time of blood collection) of patients with AD were extracted, impeding further interpretation of the results. Third, DNA methylation exhibits racial differences[59,60], and our study primarily included subjects from Caucasian populations, which may introduce racial bias and limit the generalizability of the findings to other populations, such as Asians. Future studies should aim to include more diverse populations to validate and broaden the applicability of our findings. Fourth, the lack of a non-AD dementia control group limits our ability to distinguish whether the identified sex-DMPs are specific to AD or may be associated with other forms of dementia. We recognize that including non-AD dementia controls in future studies would help clarify whether these methylation patterns are uniquely associated with AD or are more broadly linked to dementia pathology.
In conclusion, our findings highlight significant sex differences in blood DNA methylation in patients with AD and address the DNA methylation associations between blood and brain tissues. These blood-based sex-differential DNA methylation profiles not only provide valuable insights into the epigenetic mechanisms underlying sex bias in AD, but also bring new opportunities to identify potential sex-specific biomarkers which could help in personalized medicine for AD.
DECLARATIONS
Acknowledgments
The authors thank Nabais MF (University of Queensland), Lunnon K (University of Exeter) and Coppola G (University of California, Los Angeles) et al. for generously sharing their DNA methylation data in the Gene Expression Omnibus database. The authors also thank Dr. Jianming Zeng (University of Macau) and all the members of his bioinformatics team, biotrainee, for generously sharing their experience and codes.
Authors’ contributions
Contributed to the conception and design of the study: Yang T, Li C, Shang H
Organized the datasets: Yang T, Li C, Wei Q, Cheng Y, Xiao Y
Performed the statistical analysis: Yang T, Li C, Wei Q, Cheng Y
Wrote the manuscript: Yang T, Shang H
All authors contributed to the manuscript revision and have read and approved the submitted version.
Availability of data and materials
All datasets analyzed in this study are publicly available, as described in the “Methods” section. The Australian Imaging, Biomarker & Lifestyle (AIBL) cohort (GSE153712), the AddNeuroMed cohort (GSE144858), and the third cohort were previously described by Li et al. (GSE53740).
Financial support and sponsorship
This study was supported by the National Natural Science Foundation of China (Grant No. 82371430).
Conflicts of interest
Shang H is Guest Editors for the Exploring Biomarkers in Neurodegenerative Diseases: Recent Advances and Clinical Implications. Shang H was not involved in any steps of edtorial processing, notably including reviewers' selection, manuscript handling and decision making. The other authors declare that there are no conflicts of interest.
Ethics approval and consent to participate
Ethics approval for the Nabais dataset, the Lunnon dataset, and the Li dataset is based on the corresponding original studies that have been published. The Nabais dataset was approved by the University of Queensland Human Research Ethics Committee (HREC): HREC/14/QRBW/ 495 (RBWH). The Lunnon dataset, which is part of the AddNeuroMed cohort, was approved by the AddNeuroMed/DCR (SLaM/IOP 30/07/2006/SLaM/IOP 30/04/2008). The Li dataset was approved by the Institutional Review Boards for human research at the University of California, San Francisco (UCSF) and the University of California, Los Angeles (UCLA).
Consent for publication
Not applicable.
Copyright
© The Author(s) 2024.
Supplementary Materials
REFERENCES
1. Kließ M, Martins R, Connolly MP. Major cost drivers in assessing the economic burden of Alzheimer’s disease: a structured, rapid review. J Prev Alzheimers Dis. 2021;8:362-70.
2. GBD 2016 Dementia Collaborators. Global, regional, and national burden of Alzheimer’s disease and other dementias, 1990-2016: a systematic analysis for the Global Burden of Disease Study 2016. Lancet Neurol. 2019;18:88-106.
3. Mielke MM, Aggarwal NT, Vila-Castelar C, et al; Diversity and Disparity Professional Interest Area Sex and Gender Special Interest Group. Consideration of sex and gender in Alzheimer’s disease and related disorders from a global perspective. Alzheimers Dement 2022;18:2707-24.
4. Eikelboom WS, Pan M, Ossenkoppele R, et al. Sex differences in neuropsychiatric symptoms in Alzheimer’s disease dementia: a meta-analysis. Alzheimers Res Ther. 2022;14:48.
5. Moore LD, Le T, Fan G. DNA methylation and its basic function. Neuropsychopharmacology. 2013;38:23-38.
6. McCartney DL, Zhang F, Hillary RF, et al. An epigenome-wide association study of sex-specific chronological ageing. Genome Med. 2019;12:1.
7. Yusipov I, Bacalini MG, Kalyakulina A, et al. Age-related DNA methylation changes are sex-specific: a comprehensive assessment. Aging. 2020;12:24057-80.
8. Yousefi P, Huen K, Davé V, Barcellos L, Eskenazi B, Holland N. Sex differences in DNA methylation assessed by 450 K BeadChip in newborns. BMC Genomics. 2015;16:911.
9. Singmann P, Shem-Tov D, Wahl S, et al. Characterization of whole-genome autosomal differences of DNA methylation between men and women. Epigenetics Chromatin. 2015;8:43.
10. Ye W, Siwko S, Tsai RYL. Sex and race-related DNA methylation changes in hepatocellular carcinoma. Int J Mol Sci. 2021;22:3820.
11. Xia Y, Dai R, Wang K, et al. Sex-differential DNA methylation and associated regulation networks in human brain implicated in the sex-biased risks of psychiatric disorders. Mol Psychiatry. 2021;26:835-48.
12. Zhang L, Young JI, Gomez L, et al. Sex-specific DNA methylation differences in Alzheimer’s disease pathology. Acta Neuropathol Commun. 2021;9:77.
13. Pellegrini C, Pirazzini C, Sala C, et al. A meta-analysis of brain DNA methylation across sex, age, and Alzheimer’s disease points for accelerated epigenetic aging in neurodegeneration. Front Aging Neurosci. 2021;13:639428.
14. Braun PR, Han S, Hing B, et al. Genome-wide DNA methylation comparison between live human brain and peripheral tissues within individuals. Transl Psychiatry. 2019;9:47.
15. Wei X, Zhang L, Zeng Y. DNA methylation in Alzheimer’s disease: In brain and peripheral blood. Mech Ageing Dev. 2020;191:111319.
16. Silva TC, Young JI, Zhang L, et al. Cross-tissue analysis of blood and brain epigenome-wide association studies in Alzheimer’s disease. Nat Commun. 2022;13:4852.
17. Silva TC, Zhang W, Young JI, et al. Distinct sex-specific DNA methylation differences in Alzheimer’s disease. Alzheimers Res Ther. 2022;14:133.
18. Nabais MF, Laws SM, Lin T, et al; Australian Imaging Biomarkers and Lifestyle study, Alzheimer’s Disease Neuroimaging Initiative. Meta-analysis of genome-wide DNA methylation identifies shared associations across neurodegenerative disorders. Genome Biol 2021;22:90.
19. Roubroeks JAY, Smith AR, Smith RG, et al. An epigenome-wide association study of Alzheimer’s disease blood highlights robust DNA hypermethylation in the HOXB6 gene. Neurobiol Aging. 2020;95:26-45.
20. Li Y, Chen JA, Sears RL, et al. An epigenetic signature in peripheral blood associated with the haplotype on 17q21.31, a risk factor for neurodegenerative tauopathy. PLoS Genet. 2014;10:e1004211.
21. McKhann G, Drachman D, Folstein M, Katzman R, Price D, Stadlan EM. Clinical diagnosis of Alzheimer’s disease: report of the NINCDS-ADRDA Work Group under the auspices of Department of Health and Human Services Task Force on Alzheimer’s Disease. Neurology. 1984;34:939-44.
22. Morris TJ, Butcher LM, Feber A, et al. ChAMP: 450k chip analysis methylation pipeline. Bioinformatics. 2014;30:428-30.
23. Troyanskaya O, Cantor M, Sherlock G, et al. Missing value estimation methods for DNA microarrays. Bioinformatics. 2001;17:520-5.
24. Zhou W, Laird PW, Shen H. Comprehensive characterization, annotation and innovative use of Infinium DNA methylation BeadChip probes. Nucleic Acids Res. 2017;45:e22.
25. Chen YA, Lemire M, Choufani S, et al. Discovery of cross-reactive probes and polymorphic CpGs in the Illumina Infinium HumanMethylation450 microarray. Epigenetics. 2013;8:203-9.
26. Teschendorff AE, Marabita F, Lechner M, et al. A beta-mixture quantile normalization method for correcting probe design bias in Illumina Infinium 450 k DNA methylation data. Bioinformatics. 2013;29:189-96.
27. Turner SD. qqman: an R package for visualizing GWAS results using Q-Q and manhattan plots. J Open Source Softw. 2018;3:731.
28. Smyth GK. Linear models and empirical bayes methods for assessing differential expression in microarray experiments. Stat Appl Genet Mol Biol. 2004;3:Article3.
29. Benjamini Y, Hochberg Y. Controlling the false discovery rate: a practical and powerful approach to multiple testing. J R Stat Soc B. 1995;57:289-300.
30. Jaffe AE, Murakami P, Lee H, et al. Bump hunting to identify differentially methylated regions in epigenetic epidemiology studies. Int J Epidemiol. 2012;41:200-9.
31. Willer CJ, Li Y, Abecasis GR. METAL: fast and efficient meta-analysis of genomewide association scans. Bioinformatics. 2010;26:2190-1.
32. Bu D, Luo H, Huo P, et al. KOBAS-i: intelligent prioritization and exploratory visualization of biological functions for gene enrichment analysis. Nucleic Acids Res. 2021;49:W317-25.
33. GSEA/MSigDB Team. GSEA FAQ. 2022. Available from: https://software.broadinstitute.org/cancer/software/gsea/wiki/index.php/FAQ#Why_does_GSEA_use_a_false_discovery_rate_.28FDR.29_of_0.25_rather_than_the_more_classic_0.05.3F. [Last accessed on 12 Dec 2024].
34. Xiong Z, Yang F, Li M, et al. EWAS Open Platform: integrated data, knowledge and toolkit for epigenome-wide association study. Nucleic Acids Res. 2022;50:D1004-9.
35. Zaghlool SB, Al-Shafai M, Al Muftah WA, Kumar P, Falchi M, Suhre K. Association of DNA methylation with age, gender, and smoking in an Arab population. Clin Epigenetics. 2015;7:6.
36. Rudolf R, Busch R, Patra AK, et al. Architecture and expression of the nfatc1 gene in lymphocytes. Front Immunol. 2014;5:21.
37. Hashimoto T, Wakabayashi T, Watanabe A, et al. CLAC: a novel Alzheimer amyloid plaque component derived from a transmembrane precursor, CLAC-P/collagen type XXV. EMBO J. 2002;21:1524-34.
38. Drummond E, Kavanagh T, Pires G, et al. The amyloid plaque proteome in early onset Alzheimer’s disease and Down syndrome. Acta Neuropathol Commun. 2022;10:53.
39. Tong Y, Xu Y, Scearce-Levie K, Ptácek LJ, Fu YH. COL25A1 triggers and promotes Alzheimer’s disease-like pathology in vivo. Neurogenetics. 2010;11:41-52.
40. Wheeler JM, McMillan P, Strovas TJ, et al. Activity of the poly(A) binding protein MSUT2 determines susceptibility to pathological tau in the mammalian brain. Sci Transl Med. 2019;11:eaao6545.
41. McMillan PJ, Strovas TJ, Baum M, et al. Pathological tau drives ectopic nuclear speckle scaffold protein SRRM2 accumulation in neuron cytoplasm in Alzheimer’s disease. Acta Neuropathol Commun. 2021;9:117.
42. Tsiknia AA, Edland SD, Sundermann EE, et al; Alzheimer’s Disease Neuroimaging Initiative. Sex differences in plasma p-tau181 associations with Alzheimer’s disease biomarkers, cognitive decline, and clinical progression. Mol Psychiatry 2022;27:4314-22.
43. Walker RM, Bermingham ML, Vaher K, et al. Epigenome-wide analyses identify DNA methylation signatures of dementia risk. Alzheimers Dement. 2020;12:e12078.
44. Fransquet PD, Lacaze P, Saffery R, et al. Blood DNA methylation signatures to detect dementia prior to overt clinical symptoms. Alzheimers Dement. 2020;12:e12056.
45. Li QS, Vasanthakumar A, Davis JW, et al; Alzheimer’s Disease Neuroimaging Initiative (ADNI). Association of peripheral blood DNA methylation level with Alzheimer’s disease progression. Clin Epigenetics 2021;13:191.
46. Guo L, Zhong MB, Zhang L, Zhang B, Cai D. Sex differences in Alzheimer’s disease: insights from the multiomics landscape. Biol Psychiatry. 2022;91:61-71.
47. Hu RT, Yu Q, Zhou SD, et al. Co-expression network analysis reveals novel genes underlying Alzheimer’s disease pathogenesis. Front Aging Neurosci. 2020;12:605961.
48. Popovitchenko T, Park Y, Page NF, et al. Translational derepression of Elavl4 isoforms at their alternative 5’ UTRs determines neuronal development. Nat Commun. 2020;11:1674.
49. Bowles KR, Silva MC, Whitney K, et al. ELAVL4, splicing, and glutamatergic dysfunction precede neuron loss in MAPT mutation cerebral organoids. Cell. 2021;184:4547-63.e17.
50. Balschun D, Moechars D, Callaerts-Vegh Z, et al. Vesicular glutamate transporter VGLUT1 has a role in hippocampal long-term potentiation and spatial reversal learning. Cereb Cortex. 2010;20:684-93.
51. Du X, Li J, Li M, et al. Research progress on the role of type I vesicular glutamate transporter (VGLUT1) in nervous system diseases. Cell Biosci. 2020;10:26.
52. Zhou JW, Zhao M, Rang WL, et al. Proteome profiling identified amyloid-β protein precursor as a novel binding partner and modulator of VGLUT1. J Alzheimers Dis. 2021;81:981-1038.
53. Vargas LM, Cerpa W, Muñoz FJ, Zanlungo S, Alvarez AR. Amyloid-β oligomers synaptotoxicity: the emerging role of EphA4/c-Abl signaling in Alzheimer’s disease. Biochim Biophys Acta Mol Basis Dis. 2018;1864:1148-59.
54. Tamura K, Chiu YW, Shiohara A, Hori Y, Tomita T. EphA4 regulates Aβ production via BACE1 expression in neurons. FASEB J. 2020;34:16383-96.
55. Jun GR, You Y, Zhu C, et al; Alzheimer’s Disease Genetics Consortium. Protein phosphatase 2A and complement component 4 are linked to the protective effect of APOE ɛ2 for Alzheimer’s disease. Alzheimers Dement 2022;18:2042-54.
56. Wu KM, Zhang YR, Huang YY, Dong Q, Tan L, Yu JT. The role of the immune system in Alzheimer’s disease. Ageing Res Rev. 2021;70:101409.
57. Paranjpe MD, Belonwu S, Wang JK, et al. Sex-specific cross tissue meta-analysis identifies immune dysregulation in women with Alzheimer’s disease. Front Aging Neurosci. 2021;13:735611.
58. Manji Z, Rojas A, Wang W, Dingledine R, Varvel NH, Ganesh T. 5xFAD mice display sex-dependent inflammatory gene induction during the prodromal stage of Alzheimer’s disease. J Alzheimers Dis. 2019;70:1259-74.
59. Maunakea AK, Phankitnirundorn K, Peres R, et al. Socioeconomic status, lifestyle, and DNA methylation age among racially and ethnically diverse adults: NIMHD social epigenomics program. JAMA Netw Open. 2024;7:e2421889.
Cite This Article

How to Cite
Download Citation
Export Citation File:
Type of Import
Tips on Downloading Citation
Citation Manager File Format
Type of Import
Direct Import: When the Direct Import option is selected (the default state), a dialogue box will give you the option to Save or Open the downloaded citation data. Choosing Open will either launch your citation manager or give you a choice of applications with which to use the metadata. The Save option saves the file locally for later use.
Indirect Import: When the Indirect Import option is selected, the metadata is displayed and may be copied and pasted as needed.
About This Article
Special Issue
Copyright
Data & Comments
Data
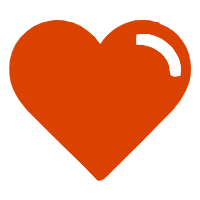
Comments
Comments must be written in English. Spam, offensive content, impersonation, and private information will not be permitted. If any comment is reported and identified as inappropriate content by OAE staff, the comment will be removed without notice. If you have any queries or need any help, please contact us at [email protected].