Artificial intelligence and image guidance in minimally invasive pancreatic surgery: current status and future challenges
Abstract
Artificial intelligence (AI), machine learning (ML), and image guidance are increasingly being used to support surgeons in preoperative, intraoperative, and postoperative decision making and optimized patient care. Surgery is the cornerstone of curative treatment in pancreatic diseases, and a large amount of perioperative data are becoming available with the widespread application of minimally invasive surgical techniques. AI is showing promise in the prediction of malignancy and resectability from preoperative images. A further clinical focus is the prediction of postoperative complications, especially pancreatic fistula, and several AI algorithms now outperform conventional fistula risk scores. Future research will be directed toward refinement of intraoperative decision support systems, individualization of surgical training, and improvement of pre- and postoperative oncologic risk stratification to personalize the sequence of surgery and chemotherapy. This review summarizes recent developments in AI and image guidance for pancreatic surgery.
Keywords
INTRODUCTION
Minimally invasive surgery (MIS) has found wide acceptance in various surgical fields; however, implementation of MIS in pancreatic surgery (MIPS) has been slow[1]. Even at highly specialized pancreatic centers, MIPS is still performed in only about 20%-40% of cases, and due to oncologic concerns, particularly resections for pancreatic ductal adenocarcinoma (PDAC) are traditionally performed via an open approach[2-5]. Although the first laparoscopic pancreatoduodenectomy (LPD) was performed by Gagner as long ago as 1994, this technique has still not gained broad acceptance due to its complexity and the steep learning curve[6]. Meanwhile, high-volume centers have mainly demonstrated slight benefits for laparoscopic pancreatic resection by experienced surgeons with regard to postoperative recovery, but high-level evidence from studies comparing oncologic outcomes is scarce[7-9]. More recently, interest has shifted toward robotic-assisted surgery, mainly due to its advantages over the laparoscopic technique, such as enhanced versatility of the robotic instruments, elimination of surgeon tremor, and better three-dimensional (3D) vision[10]. With increasing experience in robotic surgery, compelling short-term results have been demonstrated both for robotic pancreaticoduodenectomy (RPD) and robotic distal pancreatectomy (RDP)[11-13].
Artificial intelligence (AI), the simulation of intelligent behavior by computers, has emerged as a promising resource in the field of healthcare. AI is applied to extract and classify information from complex data, and its utility has been demonstrated above all for image recognition tasks[14]. As formulated by John McCarthy in 1955, AI aims “to make machines use language, form abstractions and concepts, solve kind of problems now reserved for humans, and improve themselves[15]”. Machine learning (ML), a subfield of AI, imitates human learning using computer algorithms. ML algorithms can be trained to classify data or to identify patterns within a given data set. Recently, AI has been progressively implemented in surgical practice, specifically aiming to improve clinical decision making and surgical procedures and predict and reduce human error through preoperative risk stratification and intraoperative monitoring[16].
Together with the introduction of AI, different methods for 3D real-time visualization of anatomical structures and landmarks (LM) have been implemented in various specialties[17-20]. This is of particular interest in MIPS, since the combination of AI and 3D image guidance has the potential to improve intraoperative decision making, including the assessment of resectability or the extent of surgery. Given the large amount of data thrown up by surgical cases, the introduction and application of AI in MIPS has the potential to improve both short-term postoperative outcomes and long-term oncologic survival, with particular impacts on the following aspects of MIPS [Figure 1][21]:
Figure 1. Artificial intelligence applications along the surgical treatment path of pancreatic cancer.
(1) Improved surgical technique through better orientation and presentation of structures at risk by image guidance;
(2) Enhanced intraoperative decision making by context-aware AI assistant systems, helping to determine the feasibility of complete oncologic resection;
(3) Reduction of the learning curve through novel teaching methods, including the application of augmented reality (AR) and AI-assisted skill assessment.
Together, these three aspirations comprise the overarching goal of improving both the short-term perioperative outcomes and the long-term oncologic outcomes after MIPS.
In this narrative literature review, we summarize the current state of AI and image-guided surgery in MIPS and focus on their influence on perioperative results and oncologic outcomes.
CURRENT STATUS OF MINIMALLY INVASIVE PANCREATIC SURGERY
Laparoscopic pancreatic surgery
The international guidelines on MIPS recommend minimally invasive distal pancreatectomy (MIDP) in preference to open distal pancreatectomy (ODP) for benign and low-grade malignant tumors in the body and tail of the pancreas, while advanced malignant tumors are traditionally approached via open access[22-25]. These recommendations were recently confirmed by level 1 evidence from the randomized controlled non-inferiority DIPLOMA trial, comparing MIDP with ODP[26]. This trial demonstrated the non-inferiority of MIDP regarding R0 resection rates (73% vs. 69%) and lymph node yield [22 (16-30) vs. 23 (14-32)][26]. Furthermore, the hazard ratios for overall survival [0.99 (0.67-1.46)] and disease-free survival [0.97 (0.67-1.42)] confirmed comparable long-term oncologic outcomes.
Other than in distal pancreatectomy, the safety and oncological effectiveness of laparoscopic pancreatic head resection remain debatable[27,28]. The findings of four randomized controlled trials (RCTs) indicate that at high-volume centers with adequate surgical experience, LPD may be an alternative to OPD in selected patients with comparable short-term perioperative outcomes. However, one of those trials, LEOPARD-2, had to be terminated early due to a 15% rate of postoperative mortality in the LPD group[29]. In the highly selected PDAC patients included in those RCTs, oncological outcomes have been comparable, although neither were the studies powered for those outcomes, nor do the patient cohorts reflect a real-world population, leaving the question of oncologic comparability to an open approach unanswered[30-32].
Robotic pancreatic surgery
RDP was first reported by Giulianotti et al. in 2003[33]. A recent meta-analysis of non-randomized studies revealed that RDP was associated with a lower conversion rate [OR = 0.44 (0.36-0.55)] and a lower rate of unplanned splenectomies [OR = 0.32 (0.24-0.42)] than LDP[34]. Additionally, a subgroup analysis of patients with PDAC demonstrated that RDP was associated with a higher lymph node yield [MD = 3.95 (1.67-6.23)] and a similar R0 resection rate [OR = 0.96 (0.67-1.37)] compared with LDP[34].
In contrast to RDP, RPD has been slow to gain acceptance, with the exception of a few early adopters[4,29]. No RCTs on this topic have yet not been published, and the highest level of evidence comes from a large Chinese propensity-matched multicenter study including a selected cohort of low-risk patients whose operations were performed after completion of the surgeons’ learning curves[35]. A total of 982 patients were included after propensity score matching. The RPD group had less blood loss (190 mL vs. 260 mL; P < 0.001) and a shorter length of hospital stay (LOS) [12 (9-16) days vs. 15 (11-19) days; P < 0.001] than the OPD group. No significant differences in major morbidity or 90-day mortality were found. A subgroup analysis of patients with PDAC showed no significant differences in median disease-free survival (15 months vs. 14 months, P = 0.94) or median overall survival (24 months vs. 24 months, P = 0.88). Nevertheless, this evidence gap urgently needs to be filled by high-quality studies comparing the long-term oncologic outcomes of patients with unselected tumors to determine the oncologic non-inferiority of RPD to OPD.
IMAGE GUIDANCE FOR MIPS
The intraoperative integration of various preoperative imaging modalities such as magnetic resonance imaging (MRI) or CT, resulting in image guidance, promises to enable more precise and patient-tailored MIPS. In theory, image guidance offers enhanced visualization and improved safety by providing real-time feedback to the surgeon. Hitherto, the intraoperative safety and effectiveness of MIPS have depended on detailed knowledge of the anatomy of the pancreas and its surrounding structures[36]. To date, only 2D preoperative imaging studies have been routinely available during surgery.
A crucial, as yet unsolved challenge in image guidance is the precise matching of preoperative images with the patient's actual anatomy in the operating room. Compared with the liver, the retroperitoneal localization of the pancreas makes the organ less prone to intraoperative deformation after mobilization and, therefore, more suitable for navigation. In an experimental study in which pancreatic parenchyma was resected from inside the pancreatic duct (“endopancreatic surgery”), a commercially available computer-assisted navigation system (CAScination AG, Bern, Switzerland) achieved excellent registration accuracy of
Figure 2. Image-guided endopancreatic resection with ultrasound-guided landmark registration (A and B). With the help of image guidance, accurate resections from inside the pancreatic duct are possible (C and D).
Current clinical applications of image guidance in pancreatic surgery are scarce. Pilot studies have shown image guidance to be useful for the detection of the pancreatic duct, for the depiction of the vascular anatomy in artery-first approaches, and in patients with locally advanced tumors with vascular infiltration; however, the registration accuracies were no better than 15-20 mm[39-42]. These image-to-patient registrations are mainly based on surface LM (e.g., selection of prominent structures such as large vessels or the pancreatic border) and need to be improved before clinical benefit can be expected from improvement of either technical aspects or oncologic radicality. Especially accurate intraoperative 3D visualization of the major abdominal vessels in relation to the tumor would enable the surgeon to assess the feasibility of complete tumor resection or the necessity for vascular reconstruction[43-45]. With the increasing application of robotic surgery, 3D image guidance should be integrated to achieve a real-time AR environment to display patient anatomy and therefore increase the accuracy of complete tumor resection. Currently, the main limitation for clinical application is the lack of precise image-to-patient registration with accuracy better than 10 mm.
Apart from 3D image guidance, fluorescence-guided surgery (FGS), where tumor-specific agents in combination with fluorophores are linked to tumor cells, could lead to more precise surgical cancer removal[46]. FGS enhances the identification of cancer tissue and could, therefore, help to achieve tumor-free resection margins. Given the lack of tactile feedback in MIPS, FGS, in combination with frozen sections, may have a role to play in the radical removal of advanced pancreatic tumors[46]. In a recent study, highlighting human pancreatic cancer cells by means of fluorophore-conjugated antibodies achieved a higher complete tumor resection rate in the FGS group than in the conventional resection group in a preclinical model (92% vs. 46%)[47]. Furthermore, with FGS, an additional 14% of metastases were detected. This may be pivotal in robotic pancreatic surgery, where the limited tactile feedback impedes the detection of liver metastases compared with open surgery[48].
A recent study demonstrated the utility of intraoperative angiography by means of indocyanine green (ICG) fluorescence imaging in evaluating the quality of vascular reconstructions, particularly following left gastric artery (LGA) reconstruction during distal pancreatectomy with celiac axis resection[49]. The study showed that ICG imaging effectively assessed arterial flow and tissue perfusion, enabling early identification of poor flow. This allowed for prompt surgical adjustments, e.g., re-anastomosis, thus contributing to improved postoperative outcomes. These findings underscore the potential of ICG fluorescence imaging not only in guiding surgical decisions but also in optimizing vascular reconstructions to enhance short-term outcomes.
INTRAOPERATIVE DECISION MAKING
In MIPS, a large amount of intraoperative data are becoming available and can be processed for AI applications. This includes intraoperative video data, use of instruments, and anesthesiologic data over the course of the procedure. The sum of these intraoperative data has recently been summarized with the term “surgomics”[50].
One of the most important steps - indeed, the basis for AI-guided intraoperative decision making - is the partition of the surgical procedure into clearly defined phases. Surgical phase recognition divides huge data sets generated during a surgical procedure into standardized, clearly defined smaller units, which form the backbone of intraoperative assistance or workflow optimization. ML has been applied for automated phase recognition and forms the basis for context-aware intraoperative AI applications[51]. To date, however, ML has been successful mainly in automatic phase recognition of less extensive and less complex operations such as laparoscopic cholecystectomy, colorectal surgery, and upper gastrointestinal surgery[51-55]. Nevertheless, ML has achieved high accuracy in differentiating surgical phases in those procedures, but it was often dependent on the annotation of data by humans beforehand. This concept has recently been challenged by autonomic ML systems that have accurately identified surgical steps such as dissection, suturing, or the critical view of safety in cholecystectomy[56,19]. A team from Strasbourg has successfully trained an AI algorithm to identify the critical view of safety with an accuracy of 71%.
In pancreatic surgery, complex intraoperative decisions are currently based wholly on previous human experience. As mentioned above, AI applications have the potential to learn from the vast amount of surgomic data to assist in improved decision making[21]. While current AI applications mainly focus on decision support for postoperative events (such as POPF, see below), intraoperative assistance of surgical steps would be desirable. In particular, intraoperative evaluation of resectability, resection margins, and the need for vascular resection would facilitate MIPS. In line with those suggestions, an AI model has been developed to predict the resectability of PDAC and periampullary tumors in 80 patients using 3D preoperative CT scans[41]. The model performed excellently, with 100% accuracy in the prediction of resectability. The same group then simulated the peripancreatic vessels in 43 patients to aid the surgeon with vascular resection and reconstruction, demonstrating less blood loss and a lower operating time than the conventional approach[42]. However, the application was based on preoperative imaging and did not address the intraoperative deformation of the pancreas after surgical mobilization. More recently, intraoperative decision support has been successfully implemented in other surgical fields such as orthopedics and ophthalmic surgery. In spinal cord surgery, algorithms have been trained to predict somatosensory evoked potentials, to intraoperatively assess the neurological function and, therefore, accurately detect intraoperative spinal trauma while reducing false warnings[57]. To date, similar intraoperative applications of AI-guided decision support are lacking in MIPS. However, AI will soon be able to automatically divide the MIPS procedures into different surgical steps, enabling precise, phase-dependent intraoperative support for important steps such as the transection of the pancreas or division of vessels[58].
POSTOPERATIVE DECISION MAKING
Early identification and treatment of postoperative complications is vital to improve patient outcomes, particularly in high-risk procedures such as pancreatic resection. To address this, a nationwide stepped-wedge cluster-randomized trial was conducted using an algorithm for early complication recognition[59]. Patients in the algorithm group had lower rates of both organ failure (5% vs. 10%) and 90-day mortality (3% vs. 5%). In line with this study, Merath et al. trained a ML algorithm to predict postoperative complications after pancreatic, hepatic, and colorectal resections. After processing 34 preoperative patient characteristics, the algorithm predicted 13 general complications with high accuracy (AUC > 80%); however, the study did not assess procedure-specific complications[60].
Another ML algorithm was developed using data from the National Surgical Quality Improvement Program (n = 38,209 patients) to predict unexpected mortality after pancreatectomy in low-risk patients. Age, preoperative albumin level, vascular resection, and the presence of a bleeding disorder were found to be the most important factors contributing to the ML decision-tree model. After including these procedure-specific variables, the ML model achieved an excellent AUC of 88% for the prediction of unexpected mortality[61].
With the aim of preoperatively stratifying the risk of POPF, the single most important postoperative complication after pancreatic resections, Kambakamba et al. and Skawran et al. used preoperative CT and MRI radiomics to predict this adverse event[62,63]. Based on plain CT scans, ML-based texture analysis revealed excellent accuracy in predicting POPF (AUC 95%). The proposed ML algorithm exceeded the prediction performance of currently applied fistula risk scores. From the same research group,
MODERN MIPS TRAINING
MIPS is technically complex and is associated with extensive learning curves between 20-40 procedures, dependent on the surgeon’s previous experience[66-68]. The Pittsburgh group and the LAELAPS-3 training program in the Netherlands have demonstrated that structured training with stepwise learning objectives reduces the learning curve, especially for younger and less experienced surgeons[69,70]. AI-assisted training programs have the potential to dramatically improve the quality of surgical training by implementing automated learning curve assessment and thus surgeon-tailored feedback[71,72]. In virtual orthopedic procedures, AI achieved a 97.6% accuracy in the identification of surgical training level (senior vs. junior) based on 12 procedure-related metrics[73]. AI-assisted surgical training will, therefore, automatically assess trainees’ strengths and weaknesses and could potentially reduce training time while improving the efficiency of surgical education[74].
Automated motion and instrument tracking can provide individual, contextually relevant feedback, as suggested by the “iSurgeon” training system[75]. This system implements surgical telestration, in which the mentor can highlight anatomical target structures to guide a less experienced surgeon through a procedure [Figure 3]. Mentors using such a system could provide guidance remotely, enabling persons in remote locations to access structured, high-quality surgical training. In the next step, AR can provide a real-time overlay of the patient's anatomy or even the movements of expert surgeons directly on the laparoscopic screen or the robotic console for simulation-based training[76]. Trainees can then practice procedures in a virtual environment, allowing them to gain experience without putting patients at risk[74]. Furthermore, AI has been shown to automatically decode surgical activity into individual steps with high accuracy (AUC > 0.9) and to assess the quality of the different movements from surgical videos. The ML system reliably decoded the skill level of surgical activity, such as needle handling and needle driving (AUC 0.849 and 0.821). Such an algorithm can already provide automatic feedback to surgeons and comment on their operating skills, enabling the identification of limitations in surgical behavior[56].
VALIDITY AND REPRODUCIBILITY OF AI MODELS
AI models have demonstrated the potential to assist surgeons with various tasks; however, most studies are limited by a small sample size and lack of external validation[77]. Models perform well in controlled environments, but struggle with real-world applications, where variables such as patient diversity and surgical conditions are less controlled. Moreover, the reproducibility of AI models is often compromised by inconsistent data quality and variations in surgical procedures, underscoring the need for standardized protocols for data collection and model evaluation[78]. Many AI systems rely on retrospective datasets, which may not capture real-time clinical complexity, and there is considerable variability in model accuracy due to differences in training data and evaluation methods[79]. The absence of universally accepted benchmarks further complicates the assessment of AI models' efficacy and safety. Thus, there is an urgent need for large-scale, multicenter prospective studies to rigorously validate AI tools in diverse clinical environments[80].
CHALLENGES IN THE INTEGRATION OF AI MODELS
The integration of AI in healthcare faces key challenges, including ethical concerns, data privacy risks, and uncertain cost-effectiveness. Ethical issues include algorithmic biases that could exacerbate inequalities, while data privacy concerns arise from the handling of sensitive patient information[81]. Additionally, the financial viability of AI implementation remains unclear, raising questions about the return on investment in terms of patient care and operational efficiency[82]. Addressing these issues will be essential for the responsible adoption of AI technologies in healthcare.
CONCLUSION
Early applications of AI and ML in MIPS show promise in enhancing intraoperative support through 3D image guidance and AI-driven decision making. However, most applications are still in the early stages, lack external validation, and have yet to demonstrate their efficacy beyond controlled study environments.
Future efforts should prioritize large-scale, multicenter validation studies to improve generalizability, together with addressing regulatory and ethical challenges. Training surgeons in AI tools and leveraging multimodal data integration will be critical for advancing decision support. Progress in AR, robotics, and cost-effective solutions holds the potential to enhance accessibility and precision, while standardized metrics and collaborative platforms will drive benchmarking and continuous improvement. In the near future, AI algorithms will enhance surgeons' expertise, potentially improving perioperative and oncologic outcomes.
DECLARATIONS
Authors’ contributions
Manuscript preparation and writing: Müller PC, Erdem S
Conceived and designed the analysis of the literature: Müller PC, Erdem S
Manuscript revision: Kuemmerli C, Lavanchy JL, Kraljevic M, Steinemann DC, Billeter AT, Müller BP
Availability of data and materials
Not applicable.
Financial support and sponsorship
None.
Conflicts of interest
Müller PC is an Editorial Board member of the journal Artificial Intelligence Surgery. Müller PC was not involved in any steps of editorial processing, notably including reviewer selection, manuscript handling, and decision making. The other authors declared that there are no conflicts of interest.
Ethical approval and consent to participate
Not applicable.
Consent for publication
Not applicable.
Copyright
© The Author(s) 2025.
REFERENCES
1. Pfister M, Probst P, Müller PC, et al. Minimally invasive versus open pancreatic surgery: meta-analysis of randomized clinical trials. BJS Open. 2023;7:zrad087.
2. Wellner UF, Küsters S, Sick O, et al. Hybrid laparoscopic versus open pylorus-preserving pancreatoduodenectomy: retrospective matched case comparison in 80 patients. Langenbecks Arch Surg. 2014;399:849-56.
3. Mendoza AS 3rd, Han HS, Yoon YS, Cho JY, Choi Y. Laparoscopy-assisted pancreaticoduodenectomy as minimally invasive surgery for periampullary tumors: a comparison of short-term clinical outcomes of laparoscopy-assisted pancreaticoduodenectomy and open pancreaticoduodenectomy. J Hepatobiliary Pancreat Sci. 2015;22:819-24.
4. Ramshorst TME, van Hilst J, Bannone E, et al; 2022 first Internationally validated European Guidelines Meeting on Minimally Invasive Pancreatic Surgery and the IHPBA Innovation and Research Committees. International survey on opinions and use of robot-assisted and laparoscopic minimally invasive pancreatic surgery: 5-year follow up. HPB. 2024;26:63-72.
5. der Heijde N, Vissers FL, Manzoni A, et al; European Consortium on Minimally Invasive Pancreatic Surgery. Use and outcome of minimally invasive pancreatic surgery in the European E-MIPS registry. HPB. 2023;25:400-8.
6. Gagner M, Pomp A. Laparoscopic pylorus-preserving pancreatoduodenectomy. Surg Endosc. 1994;8:408-10.
7. Palanivelu C, Senthilnathan P, Sabnis SC, et al. Randomized clinical trial of laparoscopic versus open pancreatoduodenectomy for periampullary tumours. Br J Surg. 2017;104:1443-50.
8. Poves I, Burdío F, Morató O, et al. Comparison of perioperative outcomes between laparoscopic and open approach for pancreatoduodenectomy: the PADULAP randomized controlled trial. Ann Surg. 2018;268:731-9.
9. Wang M, Pan S, Qin T, et al. Short-term outcomes following laparoscopic vs open pancreaticoduodenectomy in patients with pancreatic ductal adenocarcinoma: a randomized clinical trial. JAMA Surg. 2023;158:1245-53.
10. Baker EH, Ross SW, Seshadri R, et al. Robotic pancreaticoduodenectomy for pancreatic adenocarcinoma: role in 2014 and beyond. J Gastrointest Oncol. 2015;6:396-405.
11. Shyr YM, Wang SE, Chen SC, Shyr BU, Shyr BS. Robotic pancreaticoduodenectomy for pancreatic head cancer and periampullary lesions. Ann Gastroenterol Surg. 2021;5:589-96.
12. Nickel F, Wise PA, Müller PC, et al. Short-term outcomes of robotic versus open pancreatoduodenectomy: propensity score-matched analysis. Ann Surg. 2024;279:665-70.
13. Müller PC, Breuer E, Nickel F, et al. Robotic distal pancreatectomy: a novel standard of care? Ann Surg. 2023;278:253-9.
14. Yu KH, Beam AL, Kohane IS. Artificial intelligence in healthcare. Nat Biomed Eng. 2018;2:719-31.
15. McCarthy MLM, Nathaniel Rochester, Claude E. A proposal for the dartmouth summer research project on artificial intelligence. AI Magazine. 1995;27:4.
16. De Simone B, Chouillard E, Gumbs AA, Loftus TJ, Kaafarani H, Catena F. Artificial intelligence in surgery: the emergency surgeon's perspective (the ARIES project). Discov Health Syst. 2022;1:9.
17. Madani A, Namazi B, Altieri MS, et al. Artificial intelligence for intraoperative guidance: using semantic segmentation to identify surgical anatomy during laparoscopic cholecystectomy. Ann Surg. 2022;276:363-9.
18. Sadda P, Imamoglu M, Dombrowski M, Papademetris X, Bahtiyar MO, Onofrey J. Deep-learned placental vessel segmentation for intraoperative video enhancement in fetoscopic surgery. Int J Comput Assist Radiol Surg. 2019;14:227-35.
19. Mascagni P, Vardazaryan A, Alapatt D, et al. Artificial intelligence for surgical safety: automatic assessment of the critical view of safety in laparoscopic cholecystectomy using deep learning. Ann Surg. 2022;275:955-61.
20. Kitaguchi D, Takeshita N, Matsuzaki H, et al. Computer-assisted real-time automatic prostate segmentation during TaTME: a single-center feasibility study. Surg Endosc. 2021;35:2493-9.
21. Kuemmerli C, Rössler F, Berchtold C, et al. Artificial intelligence in pancreatic surgery: current applications. Journal of Pancreatology. 2023;6:74-81.
22. Asbun HJ, Moekotte AL, Vissers FL, et al; International Study Group on Minimally Invasive Pancreas Surgery (I-MIPS). The miami international evidence-based guidelines on minimally invasive pancreas resection. Ann Surg. 2020;271:1-14.
23. Yan Y, Hua Y, Chang C, Zhu X, Sha Y, Wang B. Laparoscopic versus open pancreaticoduodenectomy for pancreatic and periampullary tumor: a meta-analysis of randomized controlled trials and non-randomized comparative studies. Front Oncol. 2022;12:1093395.
24. Abu Hilal M, van Ramshorst TME, Boggi U, et al; Collaborators. The Brescia internationally validated European guidelines on minimally invasive pancreatic surgery (EGUMIPS). Ann Surg. 2024;279:45-57.
25. de Rooij T, van Hilst J, van Santvoort H, et al; Dutch Pancreatic Cancer Group. Minimally invasive versus open distal pancreatectomy (LEOPARD): a multicenter patient-blinded randomized controlled trial. Ann Surg. 2019;269:2-9.
26. Korrel M, Jones LR, van Hilst J, et al; European Consortium on Minimally Invasive Pancreatic Surgery (E-MIPS). Minimally invasive versus open distal pancreatectomy for resectable pancreatic cancer (DIPLOMA): an international randomised non-inferiority trial. Lancet Reg Health Eur. 2023;31:100673.
27. Yin T, Qin T, Wei K, et al. Comparison of safety and effectiveness between laparoscopic and open pancreatoduodenectomy: a systematic review and meta-analysis. Int J Surg. 2022;105:106799.
28. Nickel F, Haney CM, Kowalewski KF, et al. Laparoscopic versus open pancreaticoduodenectomy: a systematic review and meta-analysis of randomized controlled trials. Ann Surg. 2020;271:54-66.
29. van Hilst J, de Rooij T, Bosscha K, et al; Dutch Pancreatic Cancer Group. Laparoscopic versus open pancreatoduodenectomy for pancreatic or periampullary tumours (LEOPARD-2): a multicentre, patient-blinded, randomised controlled phase 2/3 trial. Lancet Gastroenterol Hepatol. 2019;4:199-207.
30. Choi M, Hwang HK, Rho SY, Lee WJ, Kang CM. Comparing laparoscopic and open pancreaticoduodenectomy in patients with pancreatic head cancer: oncologic outcomes and inflammatory scores. J Hepatobiliary Pancreat Sci. 2020;27:124-31.
31. Sharpe SM, Talamonti MS, Wang CE, et al. Early national experience with laparoscopic pancreaticoduodenectomy for ductal adenocarcinoma: a comparison of laparoscopic pancreaticoduodenectomy and open pancreaticoduodenectomy from the national cancer data base. J Am Coll Surg. 2015;221:175-84.
32. Yan JF, Pan Y, Chen K, Zhu HP, Chen QL. Minimally invasive pancreatoduodenectomy is associated with lower morbidity compared to open pancreatoduodenectomy: an updated meta-analysis of randomized controlled trials and high-quality nonrandomized studies. Medicine. 2019;98:e16730.
33. Giulianotti PC, Coratti A, Angelini M, et al. Robotics in general surgery: personal experience in a large community hospital. Arch Surg. 2003;138:777-84.
34. van Ramshorst TME, van Bodegraven EA, Zampedri P, Kasai M, Besselink MG, Abu Hilal M. Robot-assisted versus laparoscopic distal pancreatectomy: a systematic review and meta-analysis including patient subgroups. Surg Endosc. 2023;37:4131-43.
35. Liu Q, Zhao Z, Zhang X, et al. Perioperative and oncological outcomes of robotic versus open pancreaticoduodenectomy in low-risk surgical candidates: a multicenter propensity score-matched study. Ann Surg. 2023;277:e864-71.
36. Tao HS, Lin JY, Luo W, et al. Application of real-time augmented reality laparoscopic navigation in splenectomy for massive splenomegaly. World J Surg. 2021;45:2108-15.
37. Müller PC, Haslebacher C, Steinemann DC, et al. Image-guided minimally invasive endopancreatic surgery using a computer-assisted navigation system. Surg Endosc. 2021;35:1610-7.
38. Müller PC, Steinemann DC, Nickel F, et al. Transduodenal-transpapillary endopancreatic surgery with a rigid resectoscope: experiments on ex vivo, in vivo animal models and human cadavers. Surg Endosc. 2017;31:4131-5.
39. Miyamoto R, Oshiro Y, Nakayama K, et al. Three-dimensional simulation of pancreatic surgery showing the size and location of the main pancreatic duct. Surg Today. 2017;47:357-64.
40. Lin C, Gao J, Zheng H, et al. Three-dimensional visualization technology used in pancreatic surgery: a valuable tool for surgical trainees. J Gastrointest Surg. 2020;24:866-73.
41. Fang CH, Zhu W, Wang H, et al. A new approach for evaluating the resectability of pancreatic and periampullary neoplasms. Pancreatology. 2012;12:364-71.
42. Fang CH, Kong D, Wang X, et al. Three-dimensional reconstruction of the peripancreatic vascular system based on computed tomographic angiography images and its clinical application in the surgical management of pancreatic tumors. Pancreas. 2014;43:389-95.
43. Bari H, Wadhwani S, Dasari BVM. Role of artificial intelligence in hepatobiliary and pancreatic surgery. World J Gastrointest Surg. 2021;13:7-18.
44. Onda S, Okamoto T, Kanehira M, et al. Identification of inferior pancreaticoduodenal artery during pancreaticoduodenectomy using augmented reality-based navigation system. J Hepatobiliary Pancreat Sci. 2014;21:281-7.
45. Tang R, Yang W, Hou Y, et al. Augmented reality-assisted pancreaticoduodenectomy with superior mesenteric vein resection and reconstruction. Gastroenterol Res Pract. 2021;2021:9621323.
46. Mieog JSD, Achterberg FB, Zlitni A, et al. Fundamentals and developments in fluorescence-guided cancer surgery. Nat Rev Clin Oncol. 2022;19:9-22.
47. Metildi CA, Kaushal S, Pu M, et al. Fluorescence-guided surgery with a fluorophore-conjugated antibody to carcinoembryonic antigen (CEA), that highlights the tumor, improves surgical resection and increases survival in orthotopic mouse models of human pancreatic cancer. Ann Surg Oncol. 2014;21:1405-11.
48. Juhl K, Christensen A, Rubek N, Karnov KKS, von Buchwald C, Kjaer A. Improved surgical resection of metastatic pancreatic cancer using uPAR targeted in vivo fluorescent guidance: comparison with traditional white light surgery. Oncotarget. 2019;10:6308-16.
49. Oba A, Inoue Y, Sato T, et al. Impact of indocyanine green-fluorescence imaging on distal pancreatectomy with celiac axis resection combined with reconstruction of the left gastric artery. HPB. 2019;21:619-25.
50. Wagner M, Brandenburg JM, Bodenstedt S, et al. Surgomics: personalized prediction of morbidity, mortality and long-term outcome in surgery using machine learning on multimodal data. Surg Endosc. 2022;36:8568-91.
51. Garrow CR, Kowalewski KF, Li L, et al. Machine learning for surgical phase recognition: a systematic review. Ann Surg. 2021;273:684-93.
52. Kitaguchi D, Takeshita N, Matsuzaki H, et al. Real-time automatic surgical phase recognition in laparoscopic sigmoidectomy using the convolutional neural network-based deep learning approach. Surg Endosc. 2020;34:4924-31.
53. Kitaguchi D, Takeshita N, Matsuzaki H, et al. Deep learning-based automatic surgical step recognition in intraoperative videos for transanal total mesorectal excision. Surg Endosc. 2022;36:1143-51.
54. Ramesh S, Dall’Alba D, Gonzalez C, et al. Multi-task temporal convolutional networks for joint recognition of surgical phases and steps in gastric bypass procedures. Int J Comput Assist Radiol Surg. 2021;16:1111-9.
55. Ward TM, Hashimoto DA, Ban Y, et al. Automated operative phase identification in peroral endoscopic myotomy. Surg Endosc. 2021;35:4008-15.
56. Kiyasseh D, Ma R, Haque TF, et al. A vision transformer for decoding surgeon activity from surgical videos. Nat Biomed Eng. 2023;7:780-96.
57. Fan B, Li HX, Hu Y. An intelligent decision system for intraoperative somatosensory evoked potential monitoring. IEEE Trans Neural Syst Rehabil Eng. 2016;24:300-7.
58. Navarrete-Welton AJ, Hashimoto DA. Current applications of artificial intelligence for intraoperative decision support in surgery. Front Med. 2020;14:369-81.
59. Smits FJ, Henry AC, Besselink MG, et al; Dutch Pancreatic Cancer Group. Algorithm-based care versus usual care for the early recognition and management of complications after pancreatic resection in the Netherlands: an open-label, nationwide, stepped-wedge cluster-randomised trial. Lancet. 2022;399:1867-75.
60. Merath K, Hyer JM, Mehta R, et al. Use of machine learning for prediction of patient risk of postoperative complications after liver, pancreatic, and colorectal surgery. J Gastrointest Surg. 2020;24:1843-51.
61. Sahara K, Paredes AZ, Tsilimigras DI, et al. Machine learning predicts unpredicted deaths with high accuracy following hepatopancreatic surgery. Hepatobiliary Surg Nutr. 2021;10:20-30.
62. Kambakamba P, Mannil M, Herrera PE, et al. The potential of machine learning to predict postoperative pancreatic fistula based on preoperative, non-contrast-enhanced CT: a proof-of-principle study. Surgery. 2020;167:448-54.
63. Skawran SM, Kambakamba P, Baessler B, et al. Can magnetic resonance imaging radiomics of the pancreas predict postoperative pancreatic fistula? Eur J Radiol. 2021;140:109733.
64. Verma A, Balian J, Hadaya J, et al. Machine learning-based prediction of postoperative pancreatic fistula following pancreaticoduodenectomy. Ann Surg. 2024;280:325-31.
65. Ashraf Ganjouei A, Romero-Hernandez F, Wang JJ, et al. A machine learning approach to predict postoperative pancreatic fistula after pancreaticoduodenectomy using only preoperatively known data. Ann Surg Oncol. 2023;30:7738-47.
66. Haney CM, Karadza E, Limen EF, et al. Training and learning curves in minimally invasive pancreatic surgery: from simulation to mastery. Journal of Pancreatology. 2020;3:101-10.
67. Müller PC, Kuemmerli C, Cizmic A, et al. Learning curves in open, laparoscopic, and robotic pancreatic surgery: a systematic review and proposal of a standardization. Ann Surg Open. 2022;3:e111.
68. Müller PC, Müller-Stich BP, Hackert T, Nickel F. Robotic pancreaticoduodenectomy after the learning curve-a new hope. Hepatobiliary Surg Nutr. 2022;11:489-91.
69. Rice MK, Hodges JC, Bellon J, et al. Association of mentorship and a formal robotic proficiency skills curriculum with subsequent generations’ learning curve and safety for robotic pancreaticoduodenectomy. JAMA Surg. 2020;155:607-15.
70. Zwart MJW, Nota CLM, de Rooij T, et al; Dutch Pancreatic Cancer Group. Outcomes of a multicenter training program in robotic pancreatoduodenectomy (LAELAPS-3). Ann Surg. 2022;276:e886-95.
71. Fazlollahi AM, Bakhaidar M, Alsayegh A, et al. Effect of artificial intelligence tutoring vs expert instruction on learning simulated surgical skills among medical students: a randomized clinical trial. JAMA Netw Open. 2022;5:e2149008.
72. Kiyasseh D, Laca J, Haque TF, et al. A multi-institutional study using artificial intelligence to provide reliable and fair feedback to surgeons. Commun Med. 2023;3:42.
73. Bissonnette V, Mirchi N, Ledwos N, Alsidieri G, Winkler-Schwartz A, Del Maestro RF; Neurosurgical Simulation & Artificial Intelligence Learning Centre. Artificial intelligence distinguishes surgical training levels in a virtual reality spinal task. J Bone Joint Surg Am. 2019;101:e127.
74. Satapathy P, Hermis AH, Rustagi S, Pradhan KB, Padhi BK, Sah R. Artificial intelligence in surgical education and training: opportunities, challenges, and ethical considerations - correspondence. Int J Surg. 2023;109:1543-4.
75. Kowalewski KF, Hendrie JD, Schmidt MW, et al. Development and validation of a sensor- and expert model-based training system for laparoscopic surgery: the iSurgeon. Surg Endosc. 2017;31:2155-65.
76. Nickel F, Cizmic A, Chand M. Telestration and augmented reality in minimally invasive surgery: an invaluable tool in the age of COVID-19 for remote proctoring and telementoring. JAMA Surg. 2022;157:169-70.
77. Pedrett R, Mascagni P, Beldi G, Padoy N, Lavanchy JL. Technical skill assessment in minimally invasive surgery using artificial intelligence: a systematic review. Surg Endosc. 2023;37:7412-24.
78. Arora A, Alderman JE, Palmer J, et al. The value of standards for health datasets in artificial intelligence-based applications. Nat Med. 2023;29:2929-38.
79. Kelly CJ, Karthikesalingam A, Suleyman M, Corrado G, King D. Key challenges for delivering clinical impact with artificial intelligence. BMC Med. 2019;17:195.
80. Plana D, Shung DL, Grimshaw AA, Saraf A, Sung JJY, Kann BH. Randomized clinical trials of machine learning interventions in health care: a systematic review. JAMA Netw Open. 2022;5:e2233946.
81. Hasan HE, Jaber D, Khabour OF, Alzoubi KH. Ethical considerations and concerns in the implementation of AI in pharmacy practice: a cross-sectional study. BMC Med Ethics. 2024;25:55.
Cite This Article
How to Cite
Download Citation
Export Citation File:
Type of Import
Tips on Downloading Citation
Citation Manager File Format
Type of Import
Direct Import: When the Direct Import option is selected (the default state), a dialogue box will give you the option to Save or Open the downloaded citation data. Choosing Open will either launch your citation manager or give you a choice of applications with which to use the metadata. The Save option saves the file locally for later use.
Indirect Import: When the Indirect Import option is selected, the metadata is displayed and may be copied and pasted as needed.
About This Article
Special Issue
Copyright
Data & Comments
Data
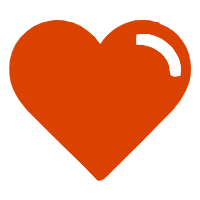
Comments
Comments must be written in English. Spam, offensive content, impersonation, and private information will not be permitted. If any comment is reported and identified as inappropriate content by OAE staff, the comment will be removed without notice. If you have any queries or need any help, please contact us at [email protected].