关键自噬相关生物标志物与HBV/HCV感染在肝细胞癌存活预后上的相互作用效应(translate by chatgpt 4.0)
摘要 (translate by chatgpt 4.0)
方法:提取了来自癌症基因组图谱(TCGA)、人类蛋白质图谱(HPA)、国际癌症基因组共济会(ICGC)和TIMER数据库中差异表达的ATGs(DEAs)的表达谱。确定与DEAs相关的生物功能和富集通路。在TCGA训练队列中,使用单变量Cox回归定义HCC亚型,并将预后性ATGs提交至LASSO Cox分析,生成总生存(OS)、无进展生存(PFS)、无疾病生存(DFS)和疾病特异性生存(DSS)相关模型。ICGC验证队列(肝癌-RIKEN JP,LIRI-JP)用于检查预测模型。使用Kaplan-Meier、核树图和受试者工作特征(ROC)分析来确认预测的准确性。通过多个数据库还检查了预后标志物与临床病理参数、基因突变、肿瘤微环境和细胞亚位注释之间的关系。
结果:共鉴定了50个DEAs,并富集在自噬体膜、程序性细胞死亡和PI3K-Akt信号通路中。BIRC5、GAPDH、FKBP1A和RAC1与较差预后显著相关,并被确定为HCC OS和DSS的独立预测因子(HR > 1.8,P < 0.05)。预后模型的风险评分证实了BIRC5是独立的预后因子。校准曲线验证了核树图对生存年限的准确性和可靠性,以及ICGC验证使用相同的风险模型。BIRC5可能影响乙型和丙型肝炎患者的死亡率。免疫组化显示BIRC5蛋白在HCC中表达适中,可能影响肿瘤检测和基因突变。高BIRC5的放大可能抑制免疫浸润物,如CD4 T细胞、巨噬细胞和中性粒细胞。
结论:BIRC5在HCC组织中高表达,表明其预后差,可能是HCC的新预后生物标志物、治疗靶点和诊断标志。
关键词 (translate by chatgpt 4.0)
INTRODUCTION
The international Agency for Research on Cancer (IARC) reported that more than 19.3 million novel tumor cases and 10 million cancer-related deaths occurred all around the world in 2020[1]. Hepatocellular carcinoma (HCC) is the third leading cause of cancer-related deaths and the sixth in terms of incidence among malignancies[2], accounting for 90% of all primary liver cancers[3,4]. Its pathological changes are determined by epigenetic and genetic alterations, including genomic insertions, mutations, and deletions[5,6]. Globally, HCC has become a serious public health issue, with rising morbidity and mortality. As reported, the 5-year survival rate of HCC patients only reached 18% in the United States[7]. Every year, more than 850,000 new instances of liver cancer are diagnosed globally, with 782,000 fatalities[8]. It is estimated that by 2030, globally, HCC-associated mortality will reach 1 million cases annually[9]. Hepatitis B virus (HBV), Hepatitis C virus (HCV), and alcoholism are all substantial risk factors for HCC development, and they are all linked to a poor prognosis and high aggressivity[10,11]. Tumors are heterogeneous and drug-resistant, and despite the advances in therapy, such as anti-viral drugs, surgical interventions, immunotherapeutic agents, and targeted therapies, HCC remains a formidable threat and a great public health challenge[12]. Therefore, it is crucial to identify suitable prognostic and diagnostic biomarkers for early HCC stages to guide effective treatment.
Autophagy is a highly conserved intracellular degradation mechanism, which is required for cellular homeostasis, protein quality management, and pathogen defense as well[13]. Autophagy plays various roles in the maintenance of liver homeostasis by eliminating damaged mitochondria and promoting cellular processes that preserve genomic stability, prevent malignant transformation, and mitigate chronic cell damage[14,15], especially in the early stages of tumorigenesis[16]. Under the regulation of autophagy-related genes (ATGs), the autophagy degradation process delivers basic nutrients and energy to liver cells[12,16], all of which are commonly interrupted during carcinogenesis and cancer treatment[13]. Currently, preclinical findings have offered circumstantial evidence of a link between autophagy-related activities and HCC prognostic outcomes[17]. For instance, Fang et al. reported that autophagy suppression reduces HCV replication in human hematoma cells[18]. In hepatoma cells, autophagy-related 5 (ATG 5) siRNA inhibits autophagy while increasing norcantharidin-induced apoptosis[19]. Suppressed autophagy elevates the levels of the autophagy protein p62, which stimulates the production of the Nrf2, which promotes liver fibrosis and liver cancer progression[5,20] while also lessening HCC cell sensitivity to sorafenib[21]. Over recent years, the ATG signature has been developed to predict prognosis in diverse types of tumors, such as resected breast tumors [22], pancreatic cancer[23], and glioma[24]. AFP, VEGF, and ANG-2 were indicated as prognostic biomarkers for HCC by the European Association for the Study of the Liver (EASL), whereas Keratin-19 and EpCAM were presented as prognostic options for their link with poor prognosis outcomes for HCC patients[17,25]. Nevertheless, the predictive efficacy and diagnostic significance of ATG signature have not been examined for HCC.
In this study, we constructed signatures of ATGs through RNA expression levels of differentially expressed autophagy-related genes (DEAs) related to HCC, which were obtained from The Cancer Genome Atlas (TCGA) cohort and the Human Autophagy Database (HADb). Notably, the autophagy-related prognostic signature related to HCC survival prediction was also determined using clinical prognosis prediction models according to the risk score cutoff value. Following that, the International Cancer Genome Consortium (ICGC) dataset [the Liver Cancer-RIKEN JP (LIRI-JP)] was used to verify the HCC prognostic signature. Notably, the models exhibit promising prognostic ability and might be used to help HCC patients make healthcare decisions.
METHODS
Data source and processing
Transcription profiling RNA-seq data, along with the corresponding clinical data for 374 HCC samples and 50 adjacent normal tissues, were acquired from the “TCGA-LIHC” dataset in the TCGA public resource portal (https://tcga-data.nci.nih.gov/tcga/)[26].
Meanwhile, the samples extracted from the TCGA cohort were used as the training group, and those from the ICGC (http://dcc.icgc.org/releases/current/Projects) portals served as the validation cohort, which provided 202 normal tissues and 243 HCC tissues.
The HADb (https://autophagy.lu/v1/clustering/), a specific database for conserving genes related to human autophagy, yielded a total of 232 ATGs[27].
Notably, raw data were filtered to remove duplicated and missing findings. First, raw data were normalized by log2(TPM +1) transformation using “RMA” (version 4.1.3) in R. Then, 19,654 protein-coding genes were annotated. The “affy” package was used to perform robust multi-array average normalization and quantile normalization on the microarray data.
Identification and functional enrichment analysis of DEGs in HCC
The R package “DESeq2” was used to identify the differentially expressed genes (DEGs) related to HCC, |log2FC| >1, and false discovery rate (FDR) < 0.05 were deemed significant, and the P value adjusted by FDR.
GO and KEGG analyses performed functional and enrichment pathways related to DEGs. KEGG is a massive database that contains information about chemical, genetic, and system activities, while GO functional annotation is a database for characterizing and defining input genes as well as protein activities in numerous species[28].
Identification of differentially expressed ARGs
The expression profile of DEAs between HCC cases and normal controls was extracted from the HADb database and the TCGA transcription profiling data. The Wilcoxon test was applied to evaluate the significance of the variation in gene expression, with a significant threshold of |log2FC| >1 and FDR < 0.05. The Kruskal-Wallis test was utilized to compare gene expression significances in various tissues.
To predict protein functions, the STRING (version 11.5, https://string-db.org/) database was used, with a combined score > 0.6 as the criterion. Cytoscape (version 3.8.0, https://cytoscape.org/) was applied to display the protein-protein interaction (PPI) network[29]. GO and KEGG analyses were completed using “GOplot” and “ClusterProfiler” packages in R to execute the enriched ontologies, biological features, and disease pathways.
Prognostic significance of DEAs in HCC
Overall survival (OS), disease-free survival (DFS), progression-free survival (PFS), and disease-specific survival (DSS) for 374 HCC samples from the TCGA-LIHC dataset were analyzed to assess whether DEAs were associated with prognostic significance in HCC patients. Using Kaplan-Meier survival curves based on the median risk score, as well as hazard ratio (HR) values with a 95% Confidence Interval (95%CI) and
Construction of the risk signature for survival prediction of HCC patients
The prognostic risk signature was produced using a LASSO Cox regression model and the R packages, “glmnet” and “survival”, to avoid false-positive outcomes and overfitting of the prognostic signature. Then, the prognostic relevance of OS-, DFS-, PFS-, and DSS-related prognostic signatures of HCC was determined using univariate and LASSO Cox regression analyses (FDR < 0.05). Running cross-validation 1,000 times yielded the best value for the penalization coefficient lambda (λ) computed in multivariate Cox regression, with the risk score =
Establishment of the prognostic signature
Hence, four DAE signatures were optimized based on the coincidence of two prognostic models, OS and DSS. Then, to determine whether four DEA signatures, age, gender, pathological grade, and tumor types were independent prognostic factors for HCC tumorigenesis, all factors were included in the parameters of univariate and multivariate Cox regression. HR and 95%CI were used for the calculation of prognostic signature, and P < 0.05 was deemed significant. The “rms” in the R package was applied to develop a nomogram that was utilized to validate the patients’ OS, PFS, DSS, and DFS probability as well as the prognostic value. The C-index was generated through “survcomp” to assess the accuracy of the Cox regression models in predicting the prognostic outcomes of HCC patients (normal: 0.50-0.70, medium accuracy: 0.71-0.90; high accuracy: > 0.90). Notably, the calibration curve, which presents the areas under the curve (AUC) values of HCC patients at 1-, 2-, 3-, and 5-years, was constructed and utilized to test the accuracy and reliability of the nomogram.
Validation of the prognostic signature
To validate the efficiency of prognostic signatures, LASSO-Cox regression analysis has been established to calculate the optimal score of prognostic signatures. The clinical trait data, LIRI-JP, related to prognostic signature were retrieved from the ICGC cohort for HCC. The risk score was generated using the same algorithm used in the TCGA training cohort, and the mRNA expression levels of the prognostic signature were standardized using the “scale” function. Using the optimal cutoff value of Kaplan-Meier analysis obtained from the ICGC cohort, a total of 243 HCC patients were separated into two groups: high-risk and low-risk. Then, a log-rank test was performed to compare survival curves between the two groups. Additionally, the specificity and sensitivity of the prognostic signature in predicting outcomes at 1, 3, and 4 years were demonstrated using ROC curve analysis.
Crosstalk between BIRC5 expression and hepatitis B and C
Hepatitis B and C are major pathogenic factors in HCC. Clinical data from 372 HCC patients in the TCGA cohort were analyzed to better understand the influence of the interaction between prognostic signature expression and hepatitis B and C on survival outcomes in HCC. Patients were divided into infected (n = 60) and uninfected (n = 312) groups. The individuals were also classified as HCV infected (n = 19) and uninfected (n = 353). Based on the median signature score, patients with hepatitis B or C ere further categorized into high-and low-risk groups, as were the uninfected patients. The effect of high and low expression of a signature on survival and prognosis were also evaluated using the Log-rank test with hepatitis C data. Kaplan-Meier curves were constructed using the “survminer” package in R, and survival predictions were made using the “survival” R package.
Expression of prognostic signature
To compare the levels of signature expression in normal tissues and malignancies, the GEPIA (http://gepia.cancer-pku.cn/) database, which integrates TCGA normal and GTEx data, was used to investigate the mRNA expression of the prognostic signature, setting the threshold as |log2FC| > 1 and P < 0.05. The “PATHOLOGY” and “TISSUE” modules of the Human Protein Atlas (HPA, https://www.proteinatlas.org/) were employed to examine protein spatial distribution at the cellular and subcellular levels in normal tissues compared to HCC tissues[30].
Association between signature, genetic alterations, and immunotherapy biomarkers
The c-Bioportal (https://www.cbioportal.org/) database presently contains multidimensional cancer genomics data for approximately 5,000 samples across 20 cancer types[31]. The c-Bioportal database discovered gene mutations, copy number variations (CNV), and mRNA expressions among the genetic alterations for prognostic signature. The “OncoPrint” tab presented a trademark summary of genetic changes discovered in each HCC sample. Tumor mutational burden (TMB) is a new cancer characteristic that measures the number of somatic mutations per 1 million bases in a tumor[32]. Surprisingly, neoantigens produced by elevated TMB trigger spontaneous antitumor immune responses and potentially predict responses to cancer immunotherapies[33,34]. In a variety of malignancies, microsatellite instability (MSI) is an efficient prognostic biomarker and immunotherapeutic response predictor[35]. The SangerBox database
Tumor immune microenvironment and subcellular location annotation
Six types of immunological infiltrates - B cells, CD4 T cells, CD8 T cells, macrophages, neutrophils, and dendritic cells - were examined using the “SCNA” module in the TIMER database (http://timer.cistrome.org/)[36]. Box plots were utilized to illustrate the distribution of each immune cell type across different mutation states in HCC, and the Wilcoxon rank test (P < 0.05) was applied to assess differences in infiltration levels in each category. The HPA database was utilized to evaluate the signature expression of proteins in all cell lines (n = 69). Based on available gene characterization data from the HPA, each subcellular location was immunofluorescently annotated, and immunohistochemistry (IHC)-stained tissues were graded according to the reliability of the analyzed protein expression[30].
Statistical analysis
The statistical analysis was performed using the R software (v4.1.3) and GraphPad Prism (v9.2). To predict and validate the prognostic significance of the autophagy-related risk signature, the “survival ROC” and “rms” R packages were employed. For each of the biomarkers and particular combinations, the diagnostic performance was examined and compared using ROC analysis. Qualitative data were given as a percentage, whereas quantitative data were expressed as mean ± standard deviation
RESULTS
Identification and functional annotations of DEGs in HCC
A total of 2,915 DEGs were screened from HCC tissues and normal controls [Figure 1A and B]. Of these, 2,371 upregulated DEGs (81.34%) were considerably enriched in phagosomes, non-alcoholic fatty liver disease (NAFLD), mRNA catabolic processes, and pathways involved in oxidative phosphorylation, and bacterial invasion of epithelial cells. In contrast, 544 downregulated DEGs (18.66%) were significantly enriched in cellular amino acid metabolism, chemical carcinogenesis, small molecule catabolic processes, pathways related to epoxygenase P450, and the PPAR signaling pathway [Figure 1C].
Identification of differentially expressed ARGs
A total of 50 DEAs were identified, consisting of 42 upregulated and 8 downregulated genes [Figure 2A]. The largest fold differences were observed in BIRC5 (Log2FC = 2.7) and SPNS1 (Log2FC = -2.5). Most of these DEAs showed significant associations with the MAPK3 and TP53 proteins in the PPI network [Figure 2B], which were also primarily enriched in the lysosome, autophagosome membrane, and endoplasmic reticulum, where they were mainly involved in processes such as autophagy, programmed cell death, and the regulation of phosphate metabolism [Figure 2C]. Additionally, they played roles as structural constituents in catalytic activities, enzyme regulator activities, and protein domain-specific binding. Meanwhile, KEGG analysis revealed that these DEAs were predominantly enriched in pathways related to apoptosis, HCC, autophagy in animals, and signaling pathways like cancer, PI3K-Akt, and NOD-like receptor [Figure 2D].
Prognostic significance of DEAs in HCC
A total of 26 DEAs were linked to a poor HCC OS [Figure 3A], whereas 15 DEAs were substantially related to a poor HCC DSS [Figure 3B]. Then, BIRC5, FKBP1A, GAPDH, and RAC1 (HR > 1.8) were identified as potential independent risk factors for OS and DSS models that were substantially associated with poor prognosis for HCC [Figures 4 and 5].
Figure 3. The forestplot determined by the OS-related risk model (A) and DSS-related risk model (B). OS: Overall survival; DSS: disease-specific survival.
Figure 4. (A) The Venn diagram for the screening of HCC OS- and DSS-related signature genes, including BIRC5, FKBP1A, GAPDH, and RAC1; (B) The identification of prognostic signature for OS-, DSS-, DFS-, and PFS-related risk models. HCC: Hepatocellular carcinoma; OS: overall survival; DSS: disease-specific survival; DFS: disease-free survival; PFS: progression-free survival.
Risk signature for survival prediction of HCC patients
The LASSO Cox models revealed that 18 potential prognostic ATGs were correlated with HCC OS when the minimum λ value was 0.0195 [P < 0.05, Figure 6A-C]. The K-M method showed that the high BIRC5 expression group had worse OS [Figure 6D], implying that a high risk score is an adverse prognostic factor
Figure 6. Establishment of the DEAs prognostic classifier according to the OS of HCC based on the TCGA database. (A and B) LASSO Cox regression analysis of ATGs that can significantly affect the HCC OS; (C) The expression of 18 prognosis-related risk genes and the distribution of risk scores and survival status; (D) The overall survival in the high-risk and low-risk groups was analyzed using the K-M method; (E) ROC curves revealed the BIRC5’s prediction effectiveness for HCC. DEAs: Differentially expressed autophagy-related genes; OS: overall survival; HCC: hepatocellular carcinoma; TCGA: The Cancer Genome Atlas; ATGs: autophagy-related genes; ROC: receiver operating characteristic.
Construction of the prognostic signature
Univariate and multivariate Cox models were constructed for the screened significant signatures. The forest plots determined by Univariate Cox showed that VEGFA, SQSTM1, FKBP1A, and BIRC5 are potential prognostic signatures related to HCC OS [Figure 7A]. However, multivariate Cox analyses showed that only BIRC5 was filtered as an independent prognostic signature for the HCC OS [Figure 7B]. Increased risk scores in patients were associated with shorter survival times and higher mortality. The nomograms were used to calculate the total scores predicting 1-, 2-, 3-, and 5-year survival probabilities by aggregating the scores for each HCC patient. The C-index for OS [Figure 7C] was calculated as 0.682, 0.631, 0.686, and 0.634, respectively. Notably, the prediction accuracy improves as the calibration curve approaches the diagonal. The result demonstrated good consistencies between the predicted survival outcomes of the monogram and the observed actual survival outcomes [Figure 7D].
Figure 7. Forest plots and prognostic model affecting OS in HCC. (A and B) Univariate and multivariate Cox regression analysis; (C) A nomogram for predicting 1-, 2-, 3- and 5-year survival rates of HCC patients; (D) The calibration curves for predicting patients’ survival at 1, 2, 3 and 5 years. OS: Overall survival; HCC: hepatocellular carcinoma.
Validation of the prognostic signature BIRC5
The prognostic value and clinical relevance of the signature were subsequently validated in the ICGC external validation cohort. Based on the minimum criteria, a risk model consisting of TP53, ARSA, ATIC, GAPDH, BIRC5, and CDKN2A was established [Figure 8A-C]. A total of 240 HCC patients were divided into two groups: high BIRC5 expression (n = 120) and low BIRC5 expression (n = 120). Analysis showed that the low BIRC5 expression group tended to have a higher mortality rate and worse clinical outcomes compared to the high BIRC5 expression group [HR = 5.605, Figure 8D]. Furthermore, ROC analysis indicated that the validated model exhibited excellent predictive performance at 1 year (AUC = 0.779), 3 years (AUC = 0.83), and 4 years [AUC = 0.872, Figure 8E]. The results from the ICGC cohort validation were consistent with those observed in the TCGA cohort, suggesting the stability of the validation.
Figure 8. (A and B) The LASSO Cox regression model was built using the six predictive genes discovered in the ICGC training cohort; (C) The expression of six prognosis-related risk genes and the distribution of risk scores and survival status; (D) The overall survival in the high-risk and low-risk groups was analyzed using the K-M method; (E) Based on ICGC cohorts, ROC curves revealed the BIRC5’s prediction effectiveness for HCC. ICGC: International Cancer Genome Consortium; ROC: receiver operating characteristic; HCC: hepatocellular carcinoma.
Crosstalk of BIRC5 expression with hepatitis B and C
To investigate the impact of BIRC5 expression on the OS of patients with hepatitis B and C, six predictive models were developed. The results revealed that patients with hepatitis B [HR = 1.78, Figure 9A] exhibited poorer prognostic outcomes compared to healthy individuals. Based on median BIRC5 expression levels, patients with HCC were classified into high and low BIRC5 expression groups. High BIRC5 expression was associated with reduced survival in hepatitis B patients [HR = 2.63, Figure 9B] and shortened survival in non-infected individuals [HR = 1.47, Figure 9C]. Additionally, patients with hepatitis C [HR = 2.35, Figure 9D] also demonstrated poorer prognostic outcomes compared to healthy individuals. Moreover, elevated BIRC5 expression was linked to a poor prognosis in both HCV-infected [HR = 2.31, Figure 9E] and non-HCV-infected patients [HR = 1.78, Figure 9F]. These findings suggest that high BIRC5 expression was a bad prognostic signature, contributing to increased mortality in patients with hepatitis B and hepatitis C.
Figure 9. (A) Survival curves for HBV-infected and uninfected patients using Kaplan-Meier analysis. Survival curves for high and low expression of BIRC5 in HBV-infected group (B) and uninfected group (C) using Kaplan-Meier analysis. Survival curves for HCV-infected and uninfected patients (D). Survival curves for high and low expression of BIRC5 in HCV-infected group (E) and uninfected group (F) using Kaplan-Meier analysis. HBV: Hepatitis B virus; HCV: hepatitis C virus.
BIRC5 expression in normal tissues and HCC tissues
A total of 529 samples were gathered from TCGA and GTEx databases, including 369 HCC cases and 160 normal samples. The results indicated that BIRC5 protein expression was significantly higher in the HCC group compared to the control group [P < 0.05, Figure 10A]. The “Tissue Atlas” from the HPA database illustrated the distribution of proteins across diverse malignant and normal tissues, while the “Pathology Atlas” employed IHC to assess the impact of protein expression on the survival probability of tumor patients. Notably, IHC analysis revealed that BIRC5 protein expression was moderately elevated in HCC compared to normal tissues, based on cytoplasmic immunoreactivity [P < 0.05, Figure 10B].
Association between BIRC5, genetic alterations, and immunotherapy biomarkers
As a prognostic signature biomarker in HCC patients, BIRC5 was implicated with genetic pathology parameters. A total of 1,617 HCC samples from the c-Bioportal database were analyzed, revealing that genomic alterations in BIRC5 occurred in 4.07% of 369 cases, including 3.79% amplification and 0.27% deep deletion [Figure 11A]. Genetic alterations in BIRC5 occurred in 2.7% of cases (P < 0.05), with the mutation spectrum, cancer type details, and somatic status observed in nearly 100% of the HCC samples [Figure 11B]. Furthermore, the relationship between BIRC5 and TMB, MSI, and immune neoantigens scores was analyzed. The results revealed that BIRC5 expression had minimal impact on TMB and neoantigen scores, but it was positively related to MSI in the HCC microenvironment (P = 0.005, R = 0.15), suggesting that while BIRC5 may influence tumor detection and genetic mutations, it is not likely to be applicable as a biomarker for immunotherapy [Figure 11C-E].
Tumor immune microenvironment and subcellular location annotation
The underlying relationship between BIRC5 and immune infiltration levels in the HCC microenvironment was assessed using the TIMER online tool. The results showed a strong positive correlation between BIRC5 and various immune cell types, including B cells (R = 0.31, P = 1.72 × 10-9), CD4 T cells (R = 0.13, P = 0.01), CD8 T cells (R = 0.20, P = 0), neutrophil cells (R = 0.18, P = 0), macrophages (R = 0.27, P = 0.72 × 10-8), and dendritic cells (R = 0.27, P = 1.75 × 10-7) [Figure 12A]. High BIRC5 amplification was commonly associated with immune infiltrates, such as CD4 T cells, macrophages, and neutrophils [Figure 12B].
Figure 12. The expression of BIRC5 in immune cell infiltration. (A) The relationship between BIRC5 and six different levels of immune cell infiltration; (B) Six immune cell infiltration levels in terms of copy number (the copy number contains deep deletion, arm-level deletion, diploid/normal, arm-level gain, and high amplification).
For the cell lines summarized, the RNA expression of Hep-G2 in the liver was derived from the “RNA EXPRESSION” module of the HPA database, which reported an expression level of 123.1 nTPM, suggesting low cell line specificity [Figure 13A].
Figure 13. (A) The RNA expression of Hep-G2 in the liver was derived from the “RNA EXPRESSION” module of the HPA database; (B-E) Subcellular location of BIRC5 was evaluated at multiple levels, revealing its presence in the cancer cell cytoplasm and nuclei; (F and G) The levels of BIRC5 expression in liver cell cycle. HPA: Human Protein Atlas; OS: overall survival; U-251MG: human astrocytoma cells.
In the “HUMAN CELLS” module analysis, subcellular localization of BIRC5 was determined using the antibody CAB004270, revealing its presence in the cytokinetic bridge at multiple levels, with expression values of 139.1 nTPM, 152.6 nTPM, and 150.4 nTPM [Figure 13B-E]. Furthermore, the “SINGLE CELL VARIATION” analysis demonstrated that variations in BIRC5 transcript expression were closely correlated with the cell cycle, particularly peaking in the G1 phase [Figure 13F and G]. The “SINGLE CELL TYPE” analysis identified BIRC5 expression across 16 single-cell types in the liver, with particularly high expression in T cells (n = 103, 487.9 nTPM), erythroid cells (n = 91, 261.9 nTPM), and B cells types (n = 520, 174.4 nTPM) [Figure 14A-C].
DISCUSSION
Despite significant advancements in HCC therapy, more than half of Chinese patients remain at high risk of recurrence and metastasis[37]. The effective implementation of precision medicine continues to be a challenge worldwide. Autophagy plays a crucial role in the occurrence and progression of HCC[38-40], maintaining a dynamic equilibrium among genome integrity, cellular metabolism, and homeostasis[41]. Several pathways contribute to HCC metastasis and proliferation, including Wnt/-catenin-mediated autophagy[41,42], the stimulation of epithelial-mesenchymal transition[41,43], and the activation of autophagy via the JNK1/Bcl-2 signaling pathway [41,44]. However, the prognostic significance of aberrantly expressed ATGs in HCC has not been fully investigated[45]. Therefore, this study aimed to determine an autophagy-related prognostic signature as a potential biomarker for HCC patients.
In this study, high-throughput transcriptomic data and corresponding clinical statistics of HCC patients from the “TCGA-LIHC” dataset were analyzed to identify potential prognostic ATGs. A total of 2,915 DEGs were found to be mainly correlated with autophagy, autophagosome membrane formation, programmed cell death, protein domain-specific binding, and enzyme regulator activities. Additionally, 50 potential ATGs were predominantly linked to several key signaling pathways, including apoptosis, necroptosis, hepatitis B, autophagy (animal), PI3K-Akt, IL-17, Toll-like receptor, and NOD-like receptor pathways. Regarding their prognostic significance in HCC, 26 potential ATGs were strongly associated with poor OS of HCC, while 15 ATGs were linked to poor DSS. However, only four ATGs (BIRC5, FKBP1A, GAPDH, and RAC1) were consistently expressed in both OS and DSS analyses. Among them, BIRC5 was the most upregulated ATG, while SPNS1 was the most downregulated. Notably, BIRC5 was found to be highly influenced by the activation of the PI3K/AKT signaling pathway, which inhibits autophagic cell death during HCC progression[46,47]. Additionally, BIRC5 was induced by IGF-1 signaling, promoting epithelial-mesenchymal transition[48,49]. The prognostic relevance of OS-, DFS-, PFS-, and DSS-related prognostic signatures in HCC was further evaluated using univariate Cox regression and LASSO Cox regression analyses. Based on the coefficient scores, BIRC5 emerged as a potential prognostic signature. Further screening through univariate and multivariate Cox analyses confirmed BIRC5 as an independent risk factor when combined with predictive signatures and clinical parameters. Subsequently, nomograms were constructed to predict OS, DFS, PFS, and DSS in HCC patients, demonstrating good concordance between the predicted and actual survival outcomes. Furthermore, validation using external ICGC cohorts, based on the same risk score model derived from the TCGA training cohort, revealed that TP53, ARSA, ATIC, GAPDH, BIRC5, and CDKN2A were strongly associated with poor HCC prognosis. Kaplan-Meier survival analysis with the log-rank test indicated that patients with high BIRC5 expression had significantly worse clinical outcomes compared to those with low BIRC5 expression. Additionally, ROC analysis demonstrated excellent predictive performance across multiple survival time points. In conclusion, through training and validation models, BIRC5 was identified as an independent prognostic signature for HCC.
BIRC5 is a crucial member of the apoptosis-inhibiting gene family, playing a key role in encoding regulatory molecules that suppress cell apoptosis[5,28]. Based on the above analysis, six models were constructed to investigate the impact of BIRC5 on the OS of patients infected with hepatitis B and C. Interestingly, low BIRC5 expression was associated with better clinical outcomes, leading to a reduction in hepatitis B and C patient mortality. In HCC tissues, BIRC5 expression was significantly higher than in healthy controls. BIRC5 not only promotes cell proliferation and invasion but also inhibits apoptosis and cycle arrest[50]. It plays a critical role in regulating cancer cell autophagy, contributing to chemotherapy and radiotherapy resistance, and accelerating metastasis and recurrence, all of which support cancer cell survival and tumor maintenance[51]. Additionally, BIRC5 serves as a negative regulator of apoptosis[12,17,52]. Regarding tumor angiogenesis, BIRC5 enhances the expression of VEGF, which, in turn, promotes angiogenesis in the tumor stroma[53]. Liu et al. reported that BIRC5 may play an essential part in the IGF-1 signaling pathway by regulating epithelial-mesenchymal transition (EMT) in HCC[49]. Notably, IHC analysis revealed that BIRC5 protein expression was moderately elevated in HCC compared to normal tissues, as indicated by cytoplasmic immunoreactivity. The study identified significant survival differences among patients with varying clinical characteristics. HCC cells acquire aggressive properties through a series of genomic changes[54,55]. Among 369 HCC patients, 3.79% exhibited gene amplification, 0.27% had deep deletions, and 2.7% carried other genetic alterations. Surprisingly, the mutation spectrum, cancer type details, and somatic status showed nearly 100% occurrence in HCC samples. BIRC5 appears to promote tumor growth in the HCC microenvironment, given its positive correlation with TMB, MSI, and neoantigen presence. According to Xiao et al., a new predictive score incorporating BIRC5 as a key component identified various tumor-infiltrating immune cells (TIICs) in HCC[56]. TIICs potentially contribute to tumor progression[57] and are predominantly enriched and clonally amplified in HCC [58]. Their accumulation is also linked to poor prognosis[59-61]. BIRC5 was significantly associated with the abundance of six types of TIICs, suggesting its potential as a diagnostic biomarker for HCC and its involvement in immune regulation. Furthermore, high BIRC5 amplification was frequently observed in immune infiltrates, such as CD4 T cells, macrophages, and neutrophils. The “SINGLE CELL TYPE” analysis showed that BIRC5 is highly expressed in 16 different cell types, particularly in T cells, erythroid cells, and B cells in the liver. BIRC5 expression levels were also examined in Hep-G2 cells, indicating that BIRC5 immunostaining was mainly localized in the cytoplasm and nuclei of cancer cells. Additionally, variations in BIRC5 transcript expression were strongly correlated with the cell cycle, potentially influencing the progression of the HCC cell cycle.
The relationship between the BIRC5 and HCC diagnosis and clinical treatment has garnered significant attention in recent research. BIRC5, also known as Survivin, is a member of the inhibitor of apoptosis protein (IAP) family and plays a crucial role in regulating cell survival, proliferation, and apoptosis. Its abnormal expression contributes to HCC tumorigenesis by promoting cancer cell proliferation and inhibiting apoptosis[62,63]. In terms of diagnosis, BIRC5 has shown potential as a biomarker for HCC, facilitating early detection and prognosis assessment. However, further validation in larger patient cohorts is required. BIRC5 expression levels correlate with tumor size, metastasis, and patient survival, making it a promising target for diagnostic applications. Clinically, BIRC5-targeted therapies, such as small molecule inhibitors and immunotherapies, are being explored as potential treatments to inhibit tumor growth and improve patient outcomes. The underlying mechanisms of BIRC5 in HCC involve its interaction with key signaling pathways, including the PI3K/AKT and NF-κB pathways, which regulate apoptosis and cell cycle progression. Understanding these mechanisms could provide valuable insights into novel therapeutic strategies for HCC.
The study has several limitations as follows: Firstly, it employs a descriptive study design, which cannot completely eliminate the influence of confounding factors; Secondly, the study may be limited by a small sample size, especially for specific patient groups (e.g., those with HBV or HCV infections), which could affect the generalizability of the conclusions; Thirdly, autophagy is a multifaceted and complex biological process, and a single biomarker may not fully capture its role in HCC. Future studies should comprehensively investigate the complex effects of autophagy on the prognosis of HBV/HCV-infected patients.
In conclusion, this study systematically integrates publicly available sequencing data with clinically validated datasets to establish risk prognostic models for identifying BIRC5 as a key prognostic signature. BIRC5 could effectively and independently predict the OS, PFS, DFS, and DSS in HCC patients. Importantly, the identification of BIRC5 as a prognostic factor provides a basis for the development of therapeutic interventions for HCC via autophagy-related mechanisms.
DECLARATIONS
Acknowledgments
The authors thank those who participated in the preparation and maintenance of the databases used in this study, and the School of Public Health, Shaanxi University of Chinese Medicine, for assistance.
Authors’ contributions
Conceived and designed the study: Li J, Zhang R
Performed bioinformatics analysis and experiments: Wang R
Wrote the manuscript: Guo H, Kou X
Reviewed and approved the final manuscript: Wang R, Guo H, Kou X, Chen R, Zhang R, Li J
Availability of data and materials
The TCGA and ICGC datasets were obtained from open databases. TCGA dataset can be obtained from the following website: http://cancergenome.nih.gov. ICGC dataset can be obtained from the data portal: https://dcc.icgc.org/. Additional raw data will be made available by the corresponding authors upon reasonable request.
Financial support and sponsorship
Project supported by the National Natural Science Foundation of China (Grant NO.82174330, 82374418); Shaanxi Provincial Department of Science and Technology Innovation Team Program (2022TD-55); Shaanxi Provincial Administration of Traditional Chinese Medicine Innovation Team Program (2022-SLRH-LJ-002); Shaanxi University of Traditional Chinese Medicine High-level Chinese Medicine Key Discipline Construction Program (2024XKZD19); Shaanxi Provincial Administration of Traditional Chinese Medicine Key Research Laboratory Program.
Conflicts of interest
All authors declared that there are no conflicts of interest.
Ethics approval and consent to participate
All data for this study are derived from publicly available databases and do not directly involve human participants. Therefore, ethical approval was not required for this article.
Consent for publication
Not applicable.
Copyright
© The Author(s) 2025.
REFERENCES
1. Sung H, Ferlay J, Siegel RL, et al. Global cancer statistics 2020: GLOBOCAN estimates of incidence and mortality worldwide for 36 cancers in 185 countries. CA Cancer J Clin. 2021;71:209-49.
3. Wang W, Wei C. Advances in the early diagnosis of hepatocellular carcinoma. Genes Dis. 2020;7:308-19.
4. Luo YD, Yu HQ, Liu XY, et al. Prognostic and predicted significance of Ubqln2 in patients with hepatocellular carcinoma. Cancer Med. 2020;9:4083-94.
5. Feng R, Li J, Xuan W, Liu H, Cheng D, Wang G. An autophagy-related gene-based prognostic risk signature for hepatocellular carcinoma: construction and validation. Comput Math Methods Med. 2021;2021:5770228.
6. Li X, Xu W, Kang W, et al. Genomic analysis of liver cancer unveils novel driver genes and distinct prognostic features. Theranostics. 2018;8:1740-51.
7. Liang JY, Wang DS, Lin HC, et al. A novel ferroptosis-related gene signature for overall survival prediction in patients with hepatocellular carcinoma. Int J Biol Sci. 2020;16:2430-41.
8. Ouyang G, Yi B, Pan G, Chen X. A robust twelve-gene signature for prognosis prediction of hepatocellular carcinoma. Cancer Cell Int. 2020;20:207.
9. Kim E, Viatour P. Hepatocellular carcinoma: old friends and new tricks. Exp Mol Med. 2020;52:1898-907.
10. Yang W, Niu L, Zhao X, et al. Development and validation of a survival model based on autophagy-associated genes for predicting prognosis of hepatocellular carcinoma. Am J Transl Res. 2020;12:6705-22.
11. Yang Y, Yao JH, Du QY, et al. Connexin 32 downregulation is critical for chemoresistance in oxaliplatin-resistant HCC cells associated with EMT. Cancer Manag Res. 2019;11:5133-46.
12. Zhu Y, Wang R, Chen W, Chen Q, Zhou J. Construction of a prognosis-predicting model based on autophagy-related genes for hepatocellular carcinoma (HCC) patients. Aging. 2020;12:14582-92.
13. Liang TT, Shao Q, Deng ZC, Wang T, Kang QZ. Systemic expression analysis reveals prognostic significance of WIPI3 in hepatocellular carcinoma. Front Genet. 2020;11:847.
14. Liu L, Liao JZ, He XX, Li PY. The role of autophagy in hepatocellular carcinoma: friend or foe. Oncotarget. 2017;8:57707-22.
15. Huang F, Wang BR, Wang YG. Role of autophagy in tumorigenesis, metastasis, targeted therapy and drug resistance of hepatocellular carcinoma. World J Gastroenterol. 2018;24:4643-51.
16. Fang Q, Chen H. Development of a novel autophagy-related prognostic signature and nomogram for hepatocellular carcinoma. Front Oncol. 2020;10:591356.
17. Xu W, Guo W, Lu P, Ma D, Liu L, Yu F. Identification of an autophagy-related gene signature predicting overall survival for hepatocellular carcinoma. Biosci Rep. 2021;41:BSR20203231.
18. Fang S, Su J, Liang B, et al. Suppression of autophagy by mycophenolic acid contributes to inhibition of HCV replication in human hepatoma cells. Sci Rep. 2017;7:44039.
19. Xiong X, Wu M, Zhang H, et al. Atg5 siRNA inhibits autophagy and enhances norcantharidin-induced apoptosis in hepatocellular carcinoma. Int J Oncol. 2015;47:1321-8.
20. Ni HM, Woolbright BL, Williams J, et al. Nrf2 promotes the development of fibrosis and tumorigenesis in mice with defective hepatic autophagy. J Hepatol. 2014;61:617-25.
21. Pan J, Lu C, Jun W, et al. The up-regulation of P62 levels is associated with resistance of sorafenib in hepatocarcinoma cells. Int J Clin Exp Pathol. 2019;12:2622-30.
22. Gu Y, Li P, Peng F, et al. Autophagy-related prognostic signature for breast cancer. Mol Carcinog. 2016;55:292-9.
23. Ko YH, Cho YS, Won HS, et al. Prognostic significance of autophagy-related protein expression in resected pancreatic ductal adenocarcinoma. Pancreas. 2013;42:829-35.
24. Zhang H, Lu X, Wang N, et al. Autophagy-related gene expression is an independent prognostic indicator of glioma. Oncotarget. 2017;8:60987-1000.
25. Association for the Study of the Liver. EASL clinical practice guidelines: management of hepatocellular carcinoma. J Hepatol. 2018;69:182-236.
26. Wang Z, Jensen MA, Zenklusen JC. A practical guide to the cancer genome atlas (TCGA). In: Mathé E, Davis S, Editors. Statistical genomics. New York: Springer; 2016. pp. 111-41.
27. Li C, Wu ZH, Yuan K. Autophagy-related signature for head and neck squamous cell carcinoma. Dis Markers. 2020;2020:8899337.
28. Zhang J, Zhang M, Huang J, et al. Development and validation of an autophagy-related gene signature for predicting the prognosis of hepatocellular carcinoma. Biomed Res Int. 2021;2021:7771037.
29. Doncheva NT, Morris JH, Gorodkin J, Jensen LJ. Cytoscape stringApp: network analysis and visualization of proteomics data. J Proteome Res. 2019;18:623-32.
30. Xu YY, Zhou H, Murphy RF, Shen HB. Consistency and variation of protein subcellular location annotations. Proteins. 2021;89:242-50.
31. Lin S, Zheng L, Lu Y, Xia Q, Zhou P, Liu Z. Comprehensive analysis on the expression levels and prognostic values of LOX family genes in kidney renal clear cell carcinoma. Cancer Med. 2020;9:8624-38.
32. Lv J, Zhu Y, Ji A, Zhang Q, Liao G. Mining TCGA database for tumor mutation burden and their clinical significance in bladder cancer. Biosci Rep. 2020;40:BSR20194337.
33. Ott PA, Hu Z, Keskin DB, et al. An immunogenic personal neoantigen vaccine for patients with melanoma. Nature. 2017;547:217-21.
34. Hu J, Qiu D, Yu A, et al. YTHDF1 is a potential pan-cancer biomarker for prognosis and immunotherapy. Front Oncol. 2021;11:607224.
35. Liu J, Chen Z, Zhao P, Li W. Prognostic and immune regulating roles of YIF1B in Pan-cancer: a potential target for both survival and therapy response evaluation. Biosci Rep. 2020;40:BSR20201384.
36. Li T, Fu J, Zeng Z, et al. TIMER2.0 for analysis of tumor-infiltrating immune cells. Nucleic Acids Res. 2020;48:W509-14.
37. Zhang Z, Zhang Y, Mo W. The autophagy related gene CHAF1B is a relevant prognostic and diagnostic biomarker in hepatocellular carcinoma. Front Oncol. 2020;10:626175.
38. Lin Z, Niu Y, Wan A, et al. RNA m6A methylation regulates sorafenib resistance in liver cancer through FOXO3-mediated autophagy. EMBO J. 2020;39:e103181.
39. Lu X, Paliogiannis P, Calvisi DF, Chen X. Role of the mammalian target of rapamycin pathway in liver cancer: from molecular genetics to targeted therapies. Hepatology. 2021;73:49-61.
40. Wei Q, Zhu R, Zhu J, Zhao R, Li M. E2-induced activation of the NLRP3 inflammasome triggers pyroptosis and inhibits autophagy in HCC cells. Oncol Res. 2019;27:827-34.
41. Huo X, Qi J, Huang K, et al. Identification of an autophagy-related gene signature that can improve prognosis of hepatocellular carcinoma patients. BMC Cancer. 2020;20:771.
42. Fan Q, Yang L, Zhang X, et al. Autophagy promotes metastasis and glycolysis by upregulating MCT1 expression and Wnt/β-catenin signaling pathway activation in hepatocellular carcinoma cells. J Exp Clin Cancer Res. 2018;37:9.
43. Li J, Yang B, Zhou Q, et al. Autophagy promotes hepatocellular carcinoma cell invasion through activation of epithelial-mesenchymal transition. Carcinogenesis. 2013;34:1343-51.
44. Deng G, Zeng S, Qu Y, et al. BMP4 promotes hepatocellular carcinoma proliferation by autophagy activation through JNK1-mediated Bcl-2 phosphorylation. J Exp Clin Cancer Res. 2018;37:156.
45. Song Z, Zhang G, Yu Y, Li S. A prognostic autophagy-related gene pair signature and small-molecule drugs for hepatocellular carcinoma. Front Genet. 2021;12:689801.
46. Wei Y, Chen X, Liang C, et al. A noncoding regulatory RNAs network driven by Circ-CDYL acts specifically in the early stages hepatocellular carcinoma. Hepatology. 2020;71:130-47.
47. Roca H, Varsos Z, Pienta KJ. CCL2 protects prostate cancer PC3 cells from autophagic death via phosphatidylinositol 3-kinase/AKT-dependent survivin up-regulation. J Biol Chem. 2008;283:25057-73.
48. Peng Y, Liu C, Li M, et al. Identification of a prognostic and therapeutic immune signature associated with hepatocellular carcinoma. Cancer Cell Int. 2021;21:98.
49. Liu F, Sun Y, Liu B, et al. Insulin-like growth factor-1 induces epithelial-mesenchymal transition in hepatocellular carcinoma by activating survivin. Oncol Rep. 2018;40:952-8.
51. Cao L, Li C, Shen S, et al. OCT4 increases BIRC5 and CCND1 expression and promotes cancer progression in hepatocellular carcinoma. BMC Cancer. 2013;13:82.
52. Xu M, Hu X, Zhang M, Ge Y. What is the impact of BIRC5 gene polymorphisms on urinary cancer susceptibility? Gene. 2020;733:144268.
53. Fernández JG, Rodríguez DA, Valenzuela M, et al. Survivin expression promotes VEGF-induced tumor angiogenesis via PI3K/Akt enhanced β-catenin/Tcf-Lef dependent transcription. Mol Cancer. 2014;13:209.
54. Cao D, Chen MK, Zhang QF, et al. Identification of immunological subtypes of hepatocellular carcinoma with expression profiling of immune-modulating genes. Aging. 2020;12:12187-205.
55. Dong LY, Zhou WZ, Ni JW, et al. Identifying the optimal gene and gene set in hepatocellular carcinoma based on differential expression and differential co-expression algorithm. Oncol Rep. 2017;37:1066-74.
56. Xiao H, Wang B, Xiong HX, et al. A novel prognostic index of hepatocellular carcinoma based on immunogenomic landscape analysis. J Cell Physiol. 2021;236:2572-91.
57. Domingues P, González-Tablas M, Otero Á, et al. Tumor infiltrating immune cells in gliomas and meningiomas. Brain Behav Immun. 2016;53:1-15.
58. Zheng C, Zheng L, Yoo JK, et al. Landscape of infiltrating T cells in liver cancer revealed by single-cell sequencing. Cell. 2017;169:1342-56.e16.
59. Sun H, Liu L, Huang Q, et al. Accumulation of tumor-infiltrating CD49a+ NK cells correlates with poor prognosis for human hepatocellular carcinoma. Cancer Immunol Res. 2019;7:1535-46.
60. Pourbagheri-Sigaroodi A, Fallah F, Bashash D, Karimi A. Unleashing the potential of gene signatures as prognostic and predictive tools: a step closer to personalized medicine in hepatocellular carcinoma (HCC). Cell Biochem Funct. 2024;42:e3913.
61. Zhou Y, Shan R, Xie W, et al. Role of autophagy-related genes in liver cancer prognosis. Genomics. 2024;116:110852.
62. Zhang H, Xia P, Liu J, Chen Z, Ma W, Yuan Y. ATIC inhibits autophagy in hepatocellular cancer through the AKT/FOXO3 pathway and serves as a prognostic signature for modeling patient survival. Int J Biol Sci. 2021;17:4442-58.
Cite This Article

How to Cite
Download Citation
Export Citation File:
Type of Import
Tips on Downloading Citation
Citation Manager File Format
Type of Import
Direct Import: When the Direct Import option is selected (the default state), a dialogue box will give you the option to Save or Open the downloaded citation data. Choosing Open will either launch your citation manager or give you a choice of applications with which to use the metadata. The Save option saves the file locally for later use.
Indirect Import: When the Indirect Import option is selected, the metadata is displayed and may be copied and pasted as needed.
About This Article
Copyright
Data & Comments
Data
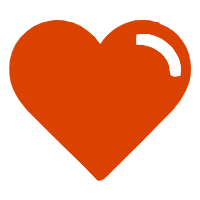
Comments
Comments must be written in English. Spam, offensive content, impersonation, and private information will not be permitted. If any comment is reported and identified as inappropriate content by OAE staff, the comment will be removed without notice. If you have any queries or need any help, please contact us at [email protected].