The application of artificial intelligence-based tools in the management of hepatocellular carcinoma: current status and future perspectives
Abstract
Artificial intelligence (AI) is rapidly advancing in hepatocellular carcinoma (HCC) management, offering promising applications across diagnosis, prognosis, and treatment. In histopathology, deep learning models have shown impressive accuracy in differentiating liver lesions and extracting prognostic information from tissue samples. For biomarker discovery, AI techniques applied to multi-omics data have identified novel prognostic signatures and predictors of immunotherapy response. In radiology, convolutional neural networks have demonstrated high performance in classifying hepatic lesions, grading tumors, and predicting microvascular invasion from computed tomography (CT) and magnetic resonance imaging (MRI) images. Multimodal AI models integrating histopathology, genomics, and clinical data are emerging as powerful tools for risk stratification. Large language models (LLMs) show potential to support clinical decision making and patient education, though concerns about accuracy remain. While AI holds immense promise, several challenges must be addressed, including algorithmic bias, data privacy, and regulatory compliance. The successful implementation of AI in HCC care will require ongoing collaboration between clinicians, data scientists, and ethicists. As AI technologies continue to evolve, they are expected to enable more personalized approaches to HCC management, potentially improving diagnosis, treatment selection, and patient outcomes. However, it is crucial to recognize that AI tools are designed to assist, not replace, clinical expertise. Continuous validation in diverse, real-world settings will be essential to ensure the reliability and generalizability of AI models in HCC care.
Keywords
INTRODUCTION
Hepatocellular carcinoma (HCC) represents the most common primary liver tumor and is the third leading cause of cancer-related deaths worldwide[1]. It is expected that the number of new cases of HCC will increase by 55% in the next two decades, representing a major public health issue[2]. The majority of HCC arises from a background of chronic liver disease and cirrhosis, and it is associated with risk factors such as viral hepatitis, alcohol consumption, and metabolic syndrome. Metabolic dysfunction-associated steatotic liver disease (MASLD) is currently the leading cause of chronic liver disease and HCC, with obesity and diabetes representing independent risk factors for HCC[3]. The common coexistence between HCC and cirrhosis makes the prognosis of this neoplasm strictly related to that of the underlying liver disease and, therefore, to the degree of liver dysfunction. In this line, multidisciplinary management of underlying chronic liver disease, in addition to the tumor, is crucial to improve survival outcomes[4,5]. Radiological diagnosis using contrast enhancement computed tomography (CT), magnetic resonance imaging (MRI), and contrast enhancement ultrasound (CEUS) for HCC is recommended, provided that the Liver Imaging Reporting and Data System (Li-RADS) criteria can be applied (e.g., in patients with cirrhosis of any etiology and in patients with chronic hepatitis B)[6,7]. However, due to the advent of highly effective systemic therapies for advanced stages, current guidelines now suggest considering biopsy in order to identify different tumor immune profiles and to guide personalized management and treatment[7,8]. Several histopathological and molecular subtypes have been recently identified and associated with different prognoses and responses to treatments[9,10], although their application to daily clinical practice is not yet universally accepted. Although curative treatments (including surgical resection and ablation) offer a significant survival benefit[11], most cases are diagnosed in advanced stages, where systemic therapies are indicated.
Artificial Intelligence (AI) has rapidly progressed in the last decade, with a broad potential application in the healthcare setting[12]. AI includes different technologies that can be applied to lesion detection, diagnostic processes, personalized treatment, drug discovery, administrative tasks, remote patient monitoring, predictive medicine, and medical research.
Different AI technologies, particularly deep learning and its variants such as convolutional neural networks (CNNs) and transformers, have shown promising results in medical image analysis and multimodal data integration. While CNNs first emerged as the best tools for image processing, transformer neural networks (or simply “transformers”) offer improved versatility and performance in various tasks, enhancing performance in medical image processing (e.g., predicting biomarkers from histopathology slides) and allowing better integration of multiple data types (multimodal data).
The impact of AI-based tools is expected to increase in several aspects of medicine, and healthcare professionals need to develop awareness of potential applications, advantages, and caveats of this innovative technology. In this narrative review, we aim to present and discuss the current status and future perspectives on the application of AI in the field of HCC.
METHODOLOGICAL FRAMEWORK
The evaluation and implementation of AI applications in HCC management requires a rigorous and structured methodological approach. AI implementation depends on several critical factors that must be carefully considered.
First, study design quality represents a fundamental element. The most robust studies in this field have demonstrated the importance of adequate sample size: the most significant experiences have included large multicenter cohorts, as demonstrated by Cheng et al. (n = 738)[13] and Shi et al. (n = 1,500)[14], which allowed for solidly validated results. The quality assessment of AI studies has been established by guidelines such as TRIPOD-AI and SPIRIT-AI[15,16]. The assessment of study design and data quality encompasses the methodology specification, sample size justification, and data collection rigor, with particular attention to ground truth label quality and missing data management. Model development evaluation focuses on the transparency of AI architecture reporting, validation methodology, and comprehensive performance metrics. Studies must clearly describe their data partitioning strategy for training, validation, and testing, while demonstrating external validation efforts. Guidelines emphasize the importance of reporting beyond traditional accuracy metrics to include sensitivity, specificity, and area under the curve (AUC) measurements. Clinical integration assessment examines the relevance of chosen endpoints, model interpretability measures, and computational efficiency considerations. Furthermore, studies are evaluated on their reproducibility potential through code availability, documentation quality, and demonstrated model generalizability across varied clinical settings.
Image preprocessing and standardization represent other crucial initial steps, particularly for histopathological and radiological applications. Standardized protocols for whole slide image digitization and balanced patch distribution are essential for reliable model training[17] Moreover, multi-institutional data quality control measures ensure model generalizability.
We have already mentioned how different types of technologies are more efficient depending on the required clinical task. For example, CNNs perform better in medical image analysis, while transformers are more useful in multimodal data integration[18]. The training process involves several critical phases that determine the model’s ultimate performance and clinical utility.
The initial data preparation phase requires careful consideration of multiple factors. Pre-segmentation of regions of interest (ROIs) from whole slide images (WSIs) is performed following standardized protocols[19]. Studies typically utilize between 25,000-43,000 balanced patches, employing data augmentation techniques such as rotation, flipping, and color adjustment to enhance model robustness.
The training strategy employs sophisticated approaches, including transfer learning from pre-trained networks (ResNet50, InceptionV3, Xception). Cross-validation using Monte Carlo methods helps assess model stability, while dropout layers prevent overfitting. Learning rates are dynamically adjusted based on validation performance, with early stopping implemented when validation metrics plateau.
Validation follows a structured framework with internal validation on held-out data (typically 20% of the primary dataset) and external validation on independent cohorts. Multi-institutional testing assesses generalizability, with expert comparison studies providing benchmarking against pathologist/radiologist performance. Model architecture selection must be guided by the specific clinical objective: CNNs have demonstrated excellent performance in lesion classification, with accuracy exceeding 90%, as reported in the setting of HCC[20], while transformers have proven particularly effective in multimodal data integration in different cancers[21]. The ensemble approach, combining different models, has often allowed for further performance optimization.
Performance optimization involves feature selection through different techniques (for example, LASSO regression, recursive feature elimination or support vector machine[22]), methods combining multiple models, and integration of clinical variables to improve accuracy. Regular model retraining with new data ensures continued performance. These methods follow established regulatory frameworks[23] and require continuous validation in real-world settings.
Finally, clinical implementation constitutes another crucial element. The integration of AI tools into clinical workflow requires careful evaluation of practical feasibility, required resources, and time efficiency. Particular attention must be paid to cost-effectiveness analysis, an increasingly relevant element in modern healthcare systems.
LITERATURE SEARCH
To ensure a comprehensive review, we have performed a literature search on Pubmed by using a string search reported in Supplementary Materials. The flow-chart of the literature screening process is reported in Supplementary Figure 1. Studies were included and discussed in the review if they met the following inclusion criteria: (1) peer-reviewed studies published in English, focusing on the application of AI in HCC management; (2) full-text studies. Exclusion criteria encompassed non-peer-reviewed articles and studies without validation cohorts.
HISTOPATHOLOGY
Digitalization of histopathology offers the possibility to overcome several limitations associated with conventional histopathology, potentially increasing efficiency and storage capacity and sparing time and costs. Digital pathology is based on scanning glass slides in order to obtain WSIs, which can be subsequently stored, managed, and digitally analyzed. This approach can allow pathologists to view and interpret slides on computer screens rather than through conventional microscopes. This approach could facilitate remote consultations, enabling expert review of cases from anywhere in the world. The digital format can also support the application of AI, with deep learning algorithms representing a promising tool for improving diagnostic accuracy and efficiency. Moreover, digital pathology systems can be potentially integrated with laboratory information systems, streamlining workflow and data management. This technology is currently transforming pathology practice by enhancing collaboration, enabling quantitative analysis, and supporting research and education in the field of pathology.
For example, AI can play a role in the differentiation of liver lesions [Table 1]. This could be helpful given that the differentiations of dysplastic nodules from well-differentiated HCC can be challenging, even for experienced pathologists in some instances. In this line, Cheng et al. developed a deep learning system aiming to improve the diagnosis of hepatocellular lesions (including dysplastic nodules and well-differentiated HCC) and background liver tissue[13]. This multicentre study on 738 patients assessed the diagnostic performance of 4 deep neural networks (ResNet50, InceptionV3, Xception, and the Ensemble) on surgical and biopsy specimens and the predictive performance of models was further reviewed by expert pathologists. The best model showed an AUC of 0.93 in the independent external validation cohort and the agreement rate was higher for the deep learning-based model than 9 pathologists.
Application of artificial intelligence to histopathology of hepatocellular carcinoma
Study first name, year of publication | Number of participants | Main results |
Cheng et al., 2022[13] | 738 | Prediction of cancer risk (AUC 0.93) |
Kiani et al., 2020[20] | 70 | Prediction of cancer risk (AUC 0.88) |
Saillard et al., 2020[24] | 522 | C-index for survival prediction between (0.78-0.75) |
Shi et al., 2021[14] | 1,500 | TRS > clinical-bioumoral classification |
Yamashita et al., 2021[25] | 299 | risk stratification of recurrence (AUC 0.95) |
Similar results were obtained when the AI was asked to differentiate between 2 primary liver tumors, HCC and Cholangiocarcinoma, in a study where 11 pathologists with different levels of experience were supported by an AI model[20]. The authors developed a deep-learning-based assistant trained with aeosin-colored images, and the impact of this model on the diagnostic performance of 11 pathologists with different degrees of expertise was assessed. The model showed an AUC of 0.88 in the derivation set and 0.84 in the test set. A significant improvement in accuracy was observed in the categories of non-specialized gastroenterology pathologists and pathology trainees, highlighting how AI models can also be used to aid decision-making processes, especially in the absence of extensive expertise in specialized subfields.
The application of deep learning to digital pathology is not only useful for diagnostic purposes but also for extracting prognostic information from histopathology data. For example, a study trained two deep learning algorithms through histopathological images of HCC patients undergoing liver resection[24]. The first algorithm evaluated specific areas of the histologic findings indicated by the pathologist, while the second one was independent of the physician’s experience, not requiring human expertise. Both the algorithms were able to predict the survival of 2 independent cohorts of patients with a respective c-index of 0.78 and 0.75, and they were better in predicting survival compared with conventional variables. In another study, an AI framework based on a weakly supervised deep learning model was trained with more than 2,700 WSIs from 2 independent cohorts of about 1,500 patients[14]. A tumor risk index (TRS) was extrapolated from AI that was superior to current clinical-bioumoral classifications in dividing patients into 5 different prognosis groups, with sinusoidal capillarization, prominent nucleoli and karyotheca, the nucleus/cytoplasm ratio, and infiltrating inflammatory cells emerging as the main features of TRS.
Yamashita et al. used a large number of digitized images of HCC surgical specimens to train a CNN model to accurately predict the risk of recurrence in patients following resection, achieving performance that either overlaps or even exceeds that of clinical laboratory assessments alone[25]. Particularly, this model reached concordance indices of 0.724 and 0.683 on the internal and external test cohorts, respectively, showing a better performance of the standard Tumor-Node-Metastasis classification system. This may enable better stratification of postoperative recurrence risk, the possibility of tailoring follow-up programs while saving healthcare costs, and the potential incorporation of AI into routine histological interpretation.
These studies open several potential uses of AI as a tool for prognostic staging but also, more importantly, for personalizing follow-up and therapies for individual patients, which to date is difficult to implement with conventional data.
BIOMARKERS
In biomarker discovery, AI has been explored to decode different sources of data, including - omics data [Table 2]. For example, Chaudhary et al. built a deep-learning-based model using data from 360 patients with HCC in the Cancer Genome Atlas (TCGA) multi-omics cohort, including mRNA expression, miRNA expression, CpG methylation, and clinical information[26]. The model showed a good discriminating ability in identifying two subgroups of patients with different overall survival, with good model fitness (C-index = 0.68). The authors found that TP53 inactivation mutations, higher expression of KRT19, EPCAM, and BIRC5, and the activation of Wnt and Akt signaling pathways were associated with a higher tumor aggressiveness. More importantly, the multi-omics model has been validated on 5 external datasets, with C-index values ranging from 0.67 to 0.82.
Application of artificial intelligence to biomarker research for hepatocellular carcinoma
Study first name, year of publication | Number of participants | Main results |
Chaudhary et al., 2021[26] | 360 | C-index for stratification of overall survival subgroups 0.67-0.82 |
Zeng et al., 2022[27] | 336 | Identification of genetic “signature” of sensitivity to Immunotherapy AUC 0.81-0.92 |
Zeng et al., 2023[28] | 122 | Identification of genetic “signature” of sensitivity to Immunotherapy and PFS |
Liu et al., 2023[29] | - | Identification of 12 transcriptional biomarkers associated with progression |
Another relevant unmet need is represented by the identification of patients with HCC displaying certain genetic “signatures” associated with higher sensitivity to immunotherapy. Zeng et al. developed and validated an AI-based pathology model able to predict the activation of 6 immune gene signatures in 2 independent cohorts of patients with HCC treated with surgical resection[27]. The AI models were trained by exploiting RNA sequencing on surgical samples and they were able to recognize the overexpression of genetic signatures related to responses to immunotherapies with AUCs of 0.81 and 0.92 in the validation cohort. The authors found that tissue areas with high predictive value were particularly enriched in lymphocytes, plasma cells, and neutrophils.
The identification of the predictors of response to atezolizumab-bevacizumab, which is currently considered the standard of care for first-line systemic treatment of unresectable HCC, represents an unsolved medical need. A recently published multicenter retrospective study developed an AI-based model that was able to predict the presence of a molecular signature associated with response to atezolizumab-bevacizumab[28]. This model, named atezolizumab-bevacizumab response signature-prediction (ABRS-P), was trained using TCGA dataset and was subsequently validated on 2 independent series of samples. Finally, the predictive performance of the model was tested in 122 patients treated with atezolizumab-bevacizumab, showing that patients with ABRS-P-high tumors had significantly longer progression-free survival, compared to patients with ABRS-P low tumors. Moreover, spatial transcriptomics revealed that the expression of ABRS genes was higher in tumor areas with high ABRS-P values compared to those with low ABRS-P values. Another study by Liu et al. used deep learning techniques to analyze RNA-seq data and successfully identified a set of transcriptional biomarkers associated with HCC progression[29]. This approach revealed 12 key genes with potential prognostic value, some of which were not previously associated with HCC.
MULTIMODAL AI MODELS
Multimodal AI models combine different sources of data, such as clinical data, genomic data, pathological information, and radiological images, to create an integrated system that enhances diagnostic and prognostic accuracy. These features prove to be very useful in the management of patients with cancer[30].
Researchers employed a multimodal algorithm to integrate histopathological images and genomic data from 5,470 patients diagnosed with the principal 14 types of cancer, including HCC[21]. This study demonstrated that combining both WSI and genomic data resulted in more accurate risk stratification for HCC patients compared to using either modality alone. The proposed multimodal fusion model (MMF) achieved better performance metrics (c-index and survival AUC) in predicting outcomes for HCC compared to unimodal models based solely on either histology or molecular features, suggesting that the combination of histopathology with genomic profiling could provide a better prognostic stratification for HCC patients. Moreover, this study identified histopathological features, such as the tumor microenvironment and immune cell infiltration, particularly tumor-infiltrating lymphocytes (TILs), as prognostic factors for better outcomes in HCC, consistently with their emerging role as biomarkers for response to immune checkpoint inhibitors. On the other hand, from a molecular perspective, mutations in oncogenes like TP53 and PIK3CA, as well as immune-related genes, were identified as significant contributors to the risk stratification of HCC patients.
However, the implementation of multimodal models is associated with significant challenges. Data standardization is a key issue, as different modalities often come with different formats, resolutions, and scales, making integration difficult. For example, while genomic data are highly dimensional, imaging data require spatial context, necessitating complex preprocessing and alignment methods. Harmonization across datasets is also critical to ensure compatibility. Variability in imaging protocols or sequencing platforms can introduce noise and bias, which multimodal AI systems may amplify rather than mitigate. Computational complexity may further complicate integration, limiting accessibility, particularly in resource-constrained settings. Interpretability represents another issue, given that combining modalities can obscure the contribution of individual data sources to the final predictions. This is especially problematic in HCC, where clinicians rely on clear and explainable evidence to make decisions regarding complex treatments such as surgery, locoregional therapies, or systemic options.
RADIOLOGICAL IMAGING
AI can be applied to radiology at both the diagnostic and prognostic stages [Table 3]. Deep Neural Networks (DNNs) have been successfully used in radiological imaging for liver tumor classification and tumor segmentation. Models such as CNNs can analyze MRI and CT images to identify and delineate liver lesions, improving diagnostic accuracy and reducing analysis time. Moreover, in different clinical settings, AI-based algorithms have been employed to detect radiological features that are predictive of specific mutation status[31].
Application of artificial intelligence to radiological imaging of hepatocellular carcinoma
Study first name, year of publication | Number of participants | Main results |
Hamm et al., 2019[32] | 494 | Sensitivity of 90% for classifying HCC from other hepatic lesions |
Zhen et al., 2020[33] | 1,411 | Discrimination of malignant from benign liver tumors AUC 0.95 |
Ding et al., 2021[34] | 1,234 | Prediction of preoperative HCC grading AUC 0.75-0.89 |
Xia et al., 2022[35] | 773 | Prediction of microvascular invasion AUC 0.72-0.86 |
Peng et al., 2023[37] | 562 | Prediction of response to TACE AUC 0.95-0.97 |
Particularly, the use of CNNs has been explored for different purposes, including the classification of hepatocellular lesions and metastatic tumors, the preoperative grading of HCC, and the prediction of microvascular invasion.
Hamm et al. evaluated multi-phasic MRI images from 494 hepatic lesions to develop and validate a CNN-based deep-learning model that was able to classify hepatocellular lesions[32]. They used augmentation techniques to generate 43,400 samples and performed Monte Carlo cross-validation. The final engineered model was compared for accuracy with 2 expert radiologists on a test cohort. The deep-learning model showed accuracy, sensitivity, and specificity higher than 90%, with a sensitivity of 90% for classifying HCC from other hepatic lesions, compared to 70% for radiologists. These data are particularly interesting given the potential of such a model for an accurate decision-support tool for radiologists, with extraordinarily short classification runtime of a few milliseconds.
Zhen et al. trained a CNNs-based deep learning model by using data from 1,210 patients with different liver tumors, with more than 30,000 images, validating the model in an external independent cohort of 201 patients[33]. CNN performed well in discriminating malignant from benign liver tumors on unenhanced images, with an AUC of 0.95. When CNN was combined with clinical data, AUCs for differentiating malignant lesions (including HCC, metastatic tumors and other primary liver tumors) were higher than 0.96, with 92% agreement with pathology. Moreover, the sensitivity and specificity of these models were as high as those of three experienced radiologists.
Ding et al. developed and validated a deep learning-based radiomics model for preoperative evaluation of HCC differentiation (low versus high grade) using CT images of 1,234 patients (Training and internal validation cohort: n = 1,047; external validation cohort: n = 187)[34]. They extracted 707 radiomic features, and after the exclusion of redundant and low reproducible features, 25 features were selected, showing AUCs of 0.82, 0.76, and 0.75 in the training, internal validation, and external test cohorts, respectively. Based on radiomic features, the authors developed a deep-learning model that showed AUCs of 0.85, 0.81, and 0.75 in the training, internal validation, and test cohorts, respectively. Interestingly, after the fusion of the radiomic model with a deep-learning model and a clinical model, they reached better AUCs of 0.89, 0.83, and 0.80 in the training, validation, and test cohorts, respectively. This study demonstrated that a fused model of deep learning, radiomics, and clinical data can serve as a non-invasive prediction tool for preoperative HCC grading.
The development of AI model-based algorithms to predict microvascular invasion has also been explored. Xia et al. retrospectively extracted radiomic CT data from tumors and peritumor regions of 773 HCC patients, and after feature reduction, they developed 5 radiomics models through logistic regression[35]. Interestingly, they combined the radiomic model with clinical-radiologic features to build a hybrid model. Radiomic and hybrid models demonstrated AUCs of 0.76 and 0.86 in the internal test set and 0.72 and 0.84 in the external test set, respectively. When the model-derived score was dichotomized as low versus high, it was able to predict early recurrence-free survival and overall survival.
Another potential application of deep learning-based models could be reducing radiation exposure by using algorithms capable of deriving the same, if not superior, information from images acquired from low-exposure CT scans[36]. These results have been obtained for the identification of liver metastases, but not yet for HCC.
In addition to radiological diagnosis and classification, AI also has the potential for prognosis prediction after treatment. Prognostic applications of AI in liver cancer represent a rapidly developing area of research with potentially significant implications for patient management and outcomes.
Peng et al. trained and validated a residual CNN-based model for the preoperative prediction of the response of patients with intermediate-stage HCC undergoing transarterial chemoembolization (TACE)[37]. They observed an accuracy of 83% for the CNN model in predicting response to TACE with AUCs of 0.97, 0.96, 0.95, and 0.96 for complete response, partial response, stable disease, and progressive disease, respectively. This could have implications for a more targeted selection of patients to undergo TACE, as well as avoidance of unnecessary treatments with improved cost-effectiveness.
LLMs
LLMs have emerged in recent years, demonstrating the ability to understand and develop natural language, opening up new scenarios for their use in clinical practice[23,38,39]. This potentially makes it possible to use AI at every clinical stage. In recent years, significant progress has been made in treating HCC, offering several therapeutic options to physicians and patients. AI could be utilized to identify patients who are better suited for a particular therapy by analyzing radiological, genomic, and clinical data. These data are often not fully understood by healthcare professionals themselves[40-42].
Moreover, the ability of LLMs to answer clinical questions based on complex medical notes with accuracy that is similar to that of experts could allow the automation of medical record management and the extraction of useful data for clinical decisions[23]. Finally, systems like GPT-4 (OpenAI) and Claude (Anthropic) can read and interpret scientific articles in real time, aiding in understanding, generating new hypotheses, and facilitating evidence-based practice.
In the setting of HCC, the accuracy and reproducibility of ChatGPT in answering questions regarding cirrhosis and liver cancer have been assessed[43]. In this study, ChatGPT answered 164 questions assessed by two experts plus a third reviewer. The answers were then compared with those of physicians using validated questionnaires. The parameters studied were: knowledge of cirrhosis, knowledge of hepatocarcinoma, and finally, the ability of ChatGPT to provide emotional support. The results demonstrated a solid grasp of AI, particularly in the areas of cirrhosis (79% of correct answers) and hepatocarcinoma (74%). However, less than half of the answers were rated as complete, and there was a notable challenge in specifying decision-making criteria and treatment options. Nevertheless, the chatbot was able to provide practical guidance to both patients and caregivers in the management and adaptation to the diagnosis. It is clear that integrating a chatbot into clinical practice can be useful as a support for patients and caregivers, providing education in disease management.
Similar results were observed in another study conducted to assess the performance of ChatGPT in answering questions related to liver cancer screening, surveillance, and diagnosis. Overall, 48% of the answers were considered accurate, with mean scores typically higher for questions on general HCC risk factors and preventive measures. On the other side, 25% of the answers were considered inaccurate, mostly regarding Li-RADS categories. The study shows that ChatGPT often provided inaccurate information regarding liver cancer surveillance and diagnosis. It frequently gave contradictory, falsely reassuring, or outright incorrect responses to questions about specific LI-RADS categories. This could potentially influence management decisions and patient outcomes[44].
ETHICAL, PRIVACY, AND REGULATORY CHALLENGES
Bias in AI models
Algorithmic bias in AI represents a significant concern that can perpetuate or exacerbate existing healthcare disparities. Current AI models are predominantly trained on datasets from large academic medical centers in developed regions, potentially leading to suboptimal performance when applied to diverse patient populations or resource-limited settings. For instance, training data often underrepresent certain ethnic groups, socioeconomic classes, and geographical regions, which can result in models that perform less accurately for these underrepresented populations. This is particularly relevant for HCC, given its varying epidemiology and risk factors across different populations, such as the higher prevalence of HBV-related HCC in Asian populations versus HCV- and alcohol-related HCC in Western countries[45]. Socioeconomic disparities in access to advanced imaging modalities constitute another significant source of bias. This is particularly relevant given that tertiary centers, which typically provide training datasets for AI models, utilize more advanced imaging protocols compared to peripheral centers. This disparity can lead to significant performance worsening when models are deployed in resource-limited settings. This issue could affect the performance of AI model for early HCC detection in underserved populations, as observed in different clinical settings[46]. Moreover, recent studies have also identified potential gender and age-related biases in AI model performance[47]. Finally, the algorithms might inadvertently learn and perpetuate existing biases in clinical decision making, potentially affecting screening recommendations, treatment selection, and prognosis prediction for different patient subgroups.
Patient data protection
Data privacy concerns in AI-driven HCC management encompass complex challenges in data sharing, storage, and processing, extending beyond traditional medical confidentiality requirements, encompassing complex challenges in data sharing, storage, and processing. The development of robust AI models requires large-scale, multi-institutional datasets containing sensitive patient information, including clinical data, medical imaging, and genetic data. This raises significant concerns about patient consent, data anonymization, and the potential for re-identification through advanced data mining techniques. It is necessary that healthcare institutions navigate stringent regulatory frameworks such as Health Insurance Portability and Accountability Act (HIPAA) in the United States or the General Data Protection Regulation (GDPR) in Europe while facilitating necessary data sharing for AI development. Additionally, the increasing integration of AI systems with electronic health records and real-time clinical decision support tools creates potential vulnerabilities for data breaches and unauthorized access. These privacy concerns can also impact patient trust and willingness to participate in AI-driven healthcare initiatives, potentially limiting the diversity and comprehensiveness of training datasets. Another critical issue is the acknowledgment of the potential implications of the company’s integration of AI systems in the medical domain.
In the setting of the application of AI to medical imaging, techniques such as federated learning, differential privacy, and homomorphic encryption have been proposed to safeguard data while enabling research and clinical applications[48]. However, risks such as re-identification attacks and algorithmic vulnerabilities necessitate continuous innovation to ensure ethical and secure data usage without compromising patient privacy or research potential.
Regulatory landscape
Recent regulatory frameworks have significantly changed the approach to AI management in healthcare. The 2023 WHO guidelines[49] emphasize that patient data protection is central to the ethical and effective deployment of AI systems, particularly in sensitive areas like oncology. It highlights frameworks like GDPR and HIPAA as essential for developers to navigate privacy laws and ensure compliance. WHO report advocates for privacy-preserving technologies such as anonymization, differential privacy, and secure multiparty computation during AI development. Moreover, WHO guidance stresses the importance of robust documentation and transparency throughout the AI lifecycle. This includes recording datasets used, validation metrics, and justifications for system modifications to enable traceability and accountability. To enhance ethical standards, stakeholder collaboration and the creation of AI regulatory sandboxes to evaluate AI systems in controlled environments are recommended. Post-market surveillance and adaptive learning capabilities are emphasized to monitor ongoing performance and mitigate emergent risks. These insights underline the necessity for a structured approach to integrating AI in healthcare while safeguarding patient data.
The Food and Drug Administration (FDA) emphasizes a risk-based regulatory framework tailored to different AI complexities, such as simple algorithms versus advanced models like generative AI[50]. Key concepts include life cycle management, continuous post-market monitoring, and the integration of real-world performance data to maintain AI reliability in diverse clinical contexts. Ethical concerns such as bias, cybersecurity, and patient privacy are addressed, advocating for harmonized global standards and collaboration among regulators, developers, and clinicians. The FDA highlights the need for recurring local validation to assess AI performance within specific settings and underlines the balance between financial incentives and patient health outcomes. These insights stress the importance of oversight and innovation to ensure AI’s transformative potential aligns with patient safety and healthcare equity. In order to raise awareness of the importance of regulatory bodies, a comprehensive and open-access database of FDA-approved AI-based medical technologies has been recently launched[51].
A collaborative framework between the FDA, Health Canada, and the United Kingdom’s Medicines and Healthcare products Regulatory Agency (MHRA) identified ten guiding principles to establish Good Machine Learning Practices (GMLP) for AI-driven medical devices, focusing on safety, efficacy, and high-quality standards[52]. These principles highlight the importance of multidisciplinary expertise throughout the product lifecycle, rigorous software engineering, representative datasets, and independent training/test datasets to ensure robust AI model development. The guidelines also emphasize tailored model design to mitigate risks, optimize human-AI interactions, and support clinical relevance. Moreover, this framework advocates for transparent communication with users, detailing model limitations, updates, and integration within clinical workflows. This initiative underscores the need for adaptable regulatory and ethical standards to meet evolving challenges in healthcare, ensuring patient safety and advancing responsible innovation across global markets.
The FDA has also introduced the concept of “Continual learning’’, a technique in which the decision logic of mathematical models is updated through new data while retaining previously learned knowledge[53]. Continual learning can create more advanced and updated AI-based medical devices based on new data that enable performance improvement. This differs from locked systems, where algorithms are trained on a specific dataset. The collection of post-approval, real-world data is essential to updating AI-based medical devices, potentially improving health outcomes.
In the high-stakes context of cancer treatment, ethical AI deployment requires a robust framework to balance innovation with patient safety and autonomy. A fundamental principle is the “human-in-the-loop” approach[54], where AI systems serve as decision-support tools rather than autonomous decision-makers. Clinicians must maintain final oversight of all treatment decisions, ensuring AI recommendations align with patient-specific factors and preferences. In this line, healthcare providers must transparently communicate both the role of AI in decision making and its limitations, explaining when and how AI influences diagnosis, prognosis, or treatment recommendations. Patients need to understand that AI systems are probability-based tools trained on population data, which may not capture all individual circumstances. The concept of “algorithmic transparency” is particularly relevant, given that patients may not need to understand the technical details, but they should be informed about the general basis for AI-driven recommendations affecting their care. Quality assurance and validation standards must be particularly rigorous for oncology AI applications. This includes regular performance monitoring, systematic documentation of AI-influenced decisions, and clear protocols for handling AI system errors or disagreements between AI recommendations and clinical judgment.
Technical limitations and critical considerations
The implementation of AI in HCC management, while promising, should consider several important limitations that warrant careful consideration.
Despite the promising technological advances in AI applications for HCC, there remains a significant gap between theoretical capabilities and demonstrated real-world clinical utility. Most current studies are retrospective, single-center, and lack external validation in diverse clinical settings. Large-scale, prospective studies with clearly defined clinical outcomes are notably scarce in the field. Furthermore, while many AI models show impressive performance metrics in controlled research environments, their impact on patient-centered outcomes, clinical workflow efficiency, and healthcare economics remains largely undemonstrated. Future research should prioritize prospective, multicenter trials that assess not only technical performance but also meaningful clinical endpoints such as survival rates, quality of life, and cost-effectiveness in real-world clinical practice.
Data quality and bias are critical challenges: as previously discussed, AI models trained on non-representative populations may fail to generalize, leading to suboptimal diagnostic or prognostic performance in studies of underrepresented groups. In the setting of HCC, geographic heterogeneity in risk factors, clinical presentation, and prevalence of underlying comorbidities can bias training data, leading to disparities in care.
Algorithmic opacity and interpretability represent additional potential barriers. AI models, particularly deep learning systems, often function as “black boxes”, making it difficult for clinicians to understand or trust the rationale behind predictions. In a high-stakes condition like HCC, this lack of transparency can lead to hesitation in integrating AI into clinical workflows or misinterpretation of results, potentially compromising patient outcomes. In this line, potential strategies to mitigate the risks of misdiagnosis associated with prioritizing or disregarding AI-generated diagnoses include the validation of AI outputs with clinical context, careful consideration of AI limitations, the incorporation of further opinions from alternative diagnostic tools, the engagement in continuous education, and the establishment of clear protocols for disagreements between human and AI. Moreover, in the specific setting of HCC, it should always be considered that the clinical courses of many patients, such as those treated with surgical resection and locoregional therapy, would be influenced by the technical skills of the clinicians.
Ethical concerns include the potential for exacerbating healthcare inequalities. In this line, resource-limited settings may lack the infrastructure to deploy advanced AI tools, further widening the gap in HCC care between high-income and low-income regions. Additionally, issues of data privacy and security that have been previously discussed, as well as the sensitive nature of patient health data used to train AI models, need to be considered.
These limitations underscore the need for careful dataset curation, transparent validation, robust regulatory oversight, and human-AI collaboration to ensure that AI augments, rather than undermines, care in HCC management.
FUTURE PERSPECTIVES
The future of AI in the management of HCC holds immense promise across various domains [Figure 1]. In differential diagnosis, AI is expected to revolutionize both histopathological and radiological assessments. Advanced deep learning models will likely enhance the accuracy of discriminating HCC from other liver lesions and improve the detection of subtle features indicative of early-stage HCC. Specific developmental milestones for the next years have to include the integration of automated quality control systems for image acquisition, the development of real-time lesion characterization tools, and the implementation of standardized reporting frameworks. These models may integrate multimodal data, combining histopathological images with genomic profiles and clinical data to provide more precise diagnoses. This is particularly important considering the need for adjuvant and neoadjuvant treatments after and before curative treatments, which is still unmet[55].
Figure 1. Potential applications of artificial intelligence to the management of hepatocellular carcinoma.
In prognostication, AI algorithms are expected to evolve, offering more accurate predictions of disease progression, recurrence, and progression risks, as well as overall survival by analyzing complex patterns in imaging, molecular, and clinical data. Key areas for development include novel trial designs incorporating AI-based patient stratification and integration of AI tools in treatment response assessment. The prediction of treatment response will likely become more sophisticated, with AI models guiding personalized therapy selection by predicting outcomes for different treatments, including locoregional and systemic therapies.
LLMs will play a crucial role in supporting both patients and physicians. These models could offer real-time, evidence-based guidance for clinical decision making, assist in interpreting complex medical literature, and provide patients with personalized information and support. LLMs may also revolutionize how guidelines are consulted and applied, offering instant, context-aware recommendations tailored to individual patient scenarios.
In the setting of precision medicine, the implementation of AI-based tools can support the advancement of personalized approaches. The development of integrated biomarker discovery platforms and the implementation of molecular profiling systems may represent crucial steps for the future.
In the setting of liver transplantation, AI applications are expected to refine the management of waitlists by more accurately predicting post-transplant survival, optimizing organ allocation, and assessing the risk of HCC recurrence post-transplant. Given that rejection after liver transplantation remains a significant challenge[56], AI models may also enhance the prediction and early detection of transplant rejection, potentially improving long-term outcomes.
These developments require specific infrastructure and validation frameworks. Improving the management of HCC by applying AI-based tools requires the development of automated quality control pipelines that ensure consistent data harmonization across institutions, while simultaneously integrating molecular and genomic data with imaging through standardized protocols. Model architectures should evolve to combine CNNs and transformers, incorporating attention mechanisms specifically designed for HCC features, enabling better handling of multiple imaging modalities.
Training strategies need refinement through HCC-specific pre-training on extensive liver imaging datasets, implementing learning approaches that progress from straightforward to complex cases. The integration of dynamic weighting schemes can optimize the contribution of different data modalities based on their predictive value. Clinical implementation can be enhanced by developing interpretable models that provide reasoning for their predictions while adapting to real-time clinical workflows and incorporating treatment response data.
Validation protocols require standardization across diverse geographic populations, supported by continuous performance monitoring systems and HCC-specific benchmark datasets. Performance evaluation should extend beyond traditional accuracy measures to include clinical outcomes and account for tumor heterogeneity and temporal changes. These modifications would collectively strengthen the robustness and clinical utility of AI tools in HCC management while maintaining regulatory compliance and ensuring reliable performance in real-world settings.
CONCLUSIONS
The integration of AI in the management of HCC represents a paradigm shift in hepato-oncology, offering new opportunities to enhance diagnosis, treatment selection, and patient outcomes. While AI demonstrates remarkable potential in areas such as pathologic and image analysis, prognostication, and decision support, it is crucial to recognize that these tools are designed to augment and support, not replace, clinical expertise. The successful implementation of AI in HCC management will require ongoing collaboration between clinicians, data scientists, and ethicists to address the several challenges related to data privacy, algorithmic bias, and regulatory compliance. As we move forward, continuous validation and refinement of AI models in heterogeneous, real-world clinical settings will be essential to ensure their reliability and generalizability. Ultimately, the judicious application of AI technologies, coupled with human clinical judgment, holds the promise of more precise, personalized, and effective care for patients with HCC, potentially improving survival rates and quality of life.
DECLARATIONS
Authors’ contributions
Served as the guarantors of the integrity of the entire study and contributed to the manuscript drafting and revision for important intellectual content: Celsa C, Quartararo A, Cammà C
Contributed to the manuscript editing and had full control over the preparation of the manuscript: Celsa C, Quartararo A, Maida M, Giusino G, Gaudioso V, Sparacino A, Cusimano G, Rao S, Grova A, Ciccia R, Salvato M, Mercurio F, La Mantia C, Di Maria G, Cabibbo G, Cammà C
Availability of data and materials
Not applicable.
Financial support and sponsorship
Celsa C is funded by the European Union-FESR or FSE, PON Research and Innovation (2014-2020-DM 1062/2021). Cammà C has received funding from the European Union - NextGenerationEU through the Italian Ministry of University and Research under PNRR M4C2I1.3 project PE_00000019 “HEAL ITALIA” (CUPB73C22001250006).
Conflicts of interest
Celsa C received speaker fees and advisory board honoraria from AstraZeneca, Eisai, Merck Sharp and Dohme, Ipsen and travel support from Roche. Cabibbo G has served as a consultant or on advisory boards for Bayer, Eisai, Ipsen, MSD, AstraZeneca, and Roche. Cammà C has served as a consultant or on advisory boards for Bayer, Eisai, Ipsen, MSD, AstraZeneca, and Roche. The other authors declared that there are no conflicts of interest.
Ethical approval and consent to participate
Not applicable.
Consent for publication
Not applicable.
Copyright
© The Author(s) 2025.
Supplementary Materials
REFERENCES
1. Sung H, Ferlay J, Siegel RL, et al. Global cancer statistics 2020: GLOBOCAN estimates of incidence and mortality worldwide for 36 cancers in 185 countries. CA Cancer J Clin. 2021;71:209-49.
2. Rumgay H, Arnold M, Ferlay J, et al. Global burden of primary liver cancer in 2020 and predictions to 2040. J Hepatol. 2022;77:1598-606.
3. Rodriguez LA, Schmittdiel JA, Liu L, et al. Hepatocellular carcinoma in metabolic dysfunction-associated steatotic liver disease. JAMA Netw Open. 2024;7:e2421019.
4. Reig M, Cabibbo G. Antiviral therapy in the palliative setting of HCC (BCLC-B and -C). J Hepatol. 2021;74:1225-33.
5. Cabibbo G, Aghemo A, Lai Q, Masarone M, Montagnese S, Ponziani FR; Italian Association for the Study of the Liver (AISF). Optimizing systemic therapy for advanced hepatocellular carcinoma: the key role of liver function. Dig Liver Dis. 2022;54:452-60.
6. Association for the Study of the Liver. EASL clinical practice guidelines: management of hepatocellular carcinoma. J Hepatol. 2018;69:182-236.
7. Singal AG, Llovet JM, Yarchoan M, et al. AASLD practice guidance on prevention, diagnosis, and treatment of hepatocellular carcinoma. Hepatology. 2023;78:1922-65.
8. Llovet JM, Villanueva A, Marrero JA, et al; AASLD Panel of Experts on Trial Design in HCC. Trial design and endpoints in hepatocellular carcinoma: AASLD consensus conference. Hepatology. 2021;73 Suppl 1:158-91.
9. Calderaro J, Couchy G, Imbeaud S, et al. Histological subtypes of hepatocellular carcinoma are related to gene mutations and molecular tumour classification. J Hepatol. 2017;67:727-38.
10. Sia D, Jiao Y, Martinez-Quetglas I, et al. Identification of an immune-specific class of hepatocellular carcinoma, based on molecular features. Gastroenterology. 2017;153:812-26.
11. Pecorelli A, Lenzi B, Gramenzi A, et al; Italian LiverCancer (ITA.LI.CA) group. Curative therapies are superior to standard of care (transarterial chemoembolization) for intermediate stage hepatocellular carcinoma. Liver Int. 2017;37:423-33.
12. Davenport T, Kalakota R. The potential for artificial intelligence in healthcare. Future Healthc J. 2019;6:94-8.
13. Cheng N, Ren Y, Zhou J, et al. Deep learning-based classification of hepatocellular nodular lesions on whole-slide histopathologic images. Gastroenterology. 2022;162:1948-61.e7.
14. Shi JY, Wang X, Ding GY, et al. Exploring prognostic indicators in the pathological images of hepatocellular carcinoma based on deep learning. Gut. 2021;70:951-61.
15. Collins GS, Moons KGM, Dhiman P, et al. TRIPOD+AI statement: updated guidance for reporting clinical prediction models that use regression or machine learning methods. BMJ. 2024;385:e078378.
16. Rivera SC, Liu X, Chan AW, Denniston AK, Calvert MJ; SPIRIT-AI and CONSORT-AI Working Group. Guidelines for clinical trial protocols for interventions involving artificial intelligence: the SPIRIT-AI Extension. BMJ. 2020;370:m3210.
17. Chan HP, Samala RK, Hadjiiski LM, Zhou C. Deep learning in medical image analysis. Adv Exp Med Biol. 2020;1213:3-21.
18. Mall PK, Singh PK, Srivastav S, et al. A comprehensive review of deep neural networks for medical image processing: recent developments and future opportunities. Healthc Anal. 2023;4:100216.
19. Rodriguez JPM, Rodriguez R, Silva VWK, et al. Artificial intelligence as a tool for diagnosis in digital pathology whole slide images: a systematic review. J Pathol Inform. 2022;13:100138.
20. Kiani A, Uyumazturk B, Rajpurkar P, et al. Impact of a deep learning assistant on the histopathologic classification of liver cancer. NPJ Digit Med. 2020;3:23.
21. Chen RJ, Lu MY, Williamson DFK, et al. Pan-cancer integrative histology-genomic analysis via multimodal deep learning. Cancer Cell. 2022;40:865-78.e6.
22. Le NQK, Li W, Cao Y. Sequence-based prediction model of protein crystallization propensity using machine learning and two-level feature selection. Brief Bioinform. 2023;24:bbad319.
23. Singhal K, Azizi S, Tu T, et al. Large language models encode clinical knowledge. Nature. 2023;620:172-80.
24. Saillard C, Schmauch B, Laifa O, et al. Predicting survival after hepatocellular carcinoma resection using deep learning on histological slides. Hepatology. 2020;72:2000-13.
25. Yamashita R, Long J, Saleem A, Rubin DL, Shen J. Deep learning predicts postsurgical recurrence of hepatocellular carcinoma from digital histopathologic images. Sci Rep. 2021;11:2047.
26. Chaudhary K, Poirion OB, Lu L, Garmire LX. Deep learning-based multi-omics integration robustly predicts survival in liver cancer. Clin Cancer Res. 2018;24:1248-59.
27. Zeng Q, Klein C, Caruso S, et al. Artificial intelligence predicts immune and inflammatory gene signatures directly from hepatocellular carcinoma histology. J Hepatol. 2022;77:116-27.
28. Zeng Q, Klein C, Caruso S, et al; HCC-AI study group. Artificial intelligence-based pathology as a biomarker of sensitivity to atezolizumab-bevacizumab in patients with hepatocellular carcinoma: a multicentre retrospective study. Lancet Oncol. 2023;24:1411-22.
29. Liu Y, Xun Z, Ma K, et al. Identification of a tumour immune barrier in the HCC microenvironment that determines the efficacy of immunotherapy. J Hepatol. 2023;78:770-82.
30. Lipkova J, Chen RJ, Chen B, et al. Artificial intelligence for multimodal data integration in oncology. Cancer Cell. 2022;40:1095-110.
31. Nguyen HS, Ho DKN, Nguyen NN, Tran HM, Tam KW, Le NQK. Predicting EGFR mutation status in non-small cell lung cancer using artificial intelligence: a systematic review and meta-analysis. Acad Radiol. 2024;31:660-83.
32. Hamm CA, Wang CJ, Savic LJ, et al. Deep learning for liver tumor diagnosis part I: development of a convolutional neural network classifier for multi-phasic MRI. Eur Radiol. 2019;29:3338-47.
33. Zhen SH, Cheng M, Tao YB, et al. Deep learning for accurate diagnosis of liver tumor based on magnetic resonance imaging and clinical data. Front Oncol. 2020;10:680.
34. Ding Y, Ruan S, Wang Y, et al. Novel deep learning radiomics model for preoperative evaluation of hepatocellular carcinoma differentiation based on computed tomography data. Clin Transl Med. 2021;11:e570.
35. Xia TY, Zhou ZH, Meng XP, et al. Predicting microvascular invasion in hepatocellular carcinoma using CT-based radiomics model. Radiology. 2023;307:e222729.
36. Jensen CT, Gupta S, Saleh MM, et al. Reduced-dose deep learning reconstruction for abdominal CT of liver metastases. Radiology. 2022;303:90-8.
37. Peng J, Kang S, Ning Z, et al. Residual convolutional neural network for predicting response of transarterial chemoembolization in hepatocellular carcinoma from CT imaging. Eur Radiol. 2020;30:413-24.
38. Maida M, Celsa C, Lau LHS, et al. The application of large language models in gastroenterology: a review of the literature. Cancers. 2024;16:3328.
39. Maida M, Ramai D, Mori Y, Dinis-Ribeiro M, Facciorusso A, Hassan C; and the AI-CORE (Artificial Intelligence COlorectal cancer Research) Working Group. The role of generative language systems in increasing patient awareness of colon cancer screening. Endoscopy. 2024;Online ahead of print.
40. Truhn D, Reis-Filho JS, Kather JN. Large language models should be used as scientific reasoning engines, not knowledge databases. Nat Med. 2023;29:2983-4.
41. Clusmann J, Kolbinger FR, Muti HS, et al. The future landscape of large language models in medicine. Commun Med. 2023;3:141.
42. Cheng K, Guo Q, He Y, et al. Artificial intelligence in sports medicine: could GPT-4 make human doctors obsolete? Ann Biomed Eng. 2023;51:1658-62.
43. Yeo YH, Samaan JS, Ng WH, et al. Assessing the performance of ChatGPT in answering questions regarding cirrhosis and hepatocellular carcinoma. Clin Mol Hepatol. 2023;29:721-32.
44. Cao JJ, Kwon DH, Ghaziani TT, et al. Accuracy of information provided by ChatGPT regarding liver cancer surveillance and diagnosis. AJR Am J Roentgenol. 2023;221:556-9.
45. Singal AG, Lampertico P, Nahon P. Epidemiology and surveillance for hepatocellular carcinoma: new trends. J Hepatol. 2020;72:250-61.
46. Seyyed-Kalantari L, Zhang H, McDermott MBA, Chen IY, Ghassemi M. Underdiagnosis bias of artificial intelligence algorithms applied to chest radiographs in under-served patient populations. Nat Med. 2021;27:2176-82.
47. Chu CH, Donato-Woodger S, Khan SS, et al. Age-related bias and artificial intelligence: a scoping review. Humanit Soc Sci Commun. 2023;10:510.
48. Kaissis GA, Makowski MR, Rückert D, et al. Secure, privacy-preserving and federated machine learning in medical imaging. Nat Mach Intell. 2020;2:305-11.
49. World Health Organization. Regulatory considerations on artificial intelligence for health: World Health Organization 2023. Available from: https://books.google.com/books?hl=zh-CN&lr=&id=YHoOEQAAQBAJ&oi=fnd&pg=PR5&dq=+Regulatory+considerations+on+artificial+intelligence+for+health:+World+Health+Organization+&ots=WpgouRrmWW&sig=GNSP3CzM51dMuoZ-AVVwV6C25jc#v=onepage&q=Regulatory%20considerations%20on%20artificial%20intelligence%20for%20health%3A%20World%20Health%20Organization&f=false. [Last accessed on 19 Dec 2024].
50. Warraich HJ, Tazbaz T, Califf RM. FDA perspective on the regulation of artificial intelligence in health care and biomedicine. JAMA. 2024;Online ahead of print.
51. Benjamens S, Dhunnoo P, Meskó B. The state of artificial intelligence-based FDA-approved medical devices and algorithms: an online database. NPJ Digit Med. 2020;3:118.
52. U.S. Food and Drug Administration. Good machine learning practice for medical device development: guiding principles. Available from: https://www.fda.gov/medical-devices/software-medical-device-samd/good-machine-learning-practice-medical-device-development-guiding-principles. [Last accessed on 19 Dec 2024].
53. Vokinger KN, Feuerriegel S, Kesselheim AS. Continual learning in medical devices: FDA’s action plan and beyond. Lancet Digit Health. 2021;3:e337-8.
54. Mosqueira-Rey E, Hernández-Pereira E, Alonso-Ríos D, et al. Human-in-the-loop machine learning: a state of the art. Artif Intell Rev. 2023;56:3005-54.
55. Stefanini B, Manfredi GF, D'Alessio A, et al. Delivering adjuvant and neoadjuvant treatments in the early stages of hepatocellular carcinoma. Expert Rev Gastroenterol Hepatol. 2024;18:647-60.
Cite This Article
How to Cite
Download Citation
Export Citation File:
Type of Import
Tips on Downloading Citation
Citation Manager File Format
Type of Import
Direct Import: When the Direct Import option is selected (the default state), a dialogue box will give you the option to Save or Open the downloaded citation data. Choosing Open will either launch your citation manager or give you a choice of applications with which to use the metadata. The Save option saves the file locally for later use.
Indirect Import: When the Indirect Import option is selected, the metadata is displayed and may be copied and pasted as needed.
About This Article
Copyright
Data & Comments
Data
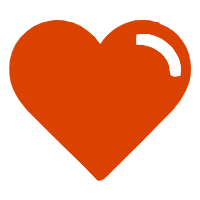
Comments
Comments must be written in English. Spam, offensive content, impersonation, and private information will not be permitted. If any comment is reported and identified as inappropriate content by OAE staff, the comment will be removed without notice. If you have any queries or need any help, please contact us at [email protected].