Predictive value of m6A regulators in prognosis and immunotherapy response of clear cell renal cell carcinoma: a bioinformatics and radiomics analysis
Abstract
Aim: This study aimed to develop an m6A-related gene signature for predicting the prognosis of clear cell renal cell carcinoma (ccRCC) patients and explore its value in predicting the immunotherapy response.
Methods: In total, 530 ccRCC patients with gene expression data in the TCGA cohort were included and classified into the training (n = 371) and validation (n = 159) sets. Differential expression analyses of 23 m6A regulators between survivors and non-survivors were performed. Subsequently, an m6A-related gene signature was developed via LASSO Cox regression. All patients were categorized into two groups of m6A subtypes, i.e., low or high m6A score group. The Kaplan-Meier survival curves and Tumor Immune Dysfunction and Exclusion (TIDE) scores of the two m6A subtype groups were compared to measure the gene signature’s predictive value in prognosis and potential immunotherapy response, respectively.
Results: Eighteen m6A regulators were significantly differentially expressed between the survivors and non-survivors, and were also related to overall survival (OS). A gene signature containing five selected m6A methylation regulators (KIAA1429, METTL14, IGF2BP2, IGF2BP3, and SRSF2) was developed and showed favorable discrimination in the training (C-index 0.708) and validation (C-index 0.689) sets. Patients with low m6A scores had significantly better OS and lower TIDE scores than those with high m6A scores. Moreover, a robust MRI-based radiomic signature was developed to noninvasively predict the m6A subtype for each patient.
Conclusion: We demonstrated the prognostic value of five m6A regulators and constructed a gene signature for prognosis and immunotherapy response prediction among ccRCC patients. In addition, a radiomic signature was developed for noninvasive prediction of the m6A subtype. These findings may promote precision medicine and provide novel insights into the regulation of tumor immune microenvironment.
Keywords
INTRODUCTION
Renal cell carcinoma (RCC) is among the most common types of cancer globally, holding a position within the top 10. RCC encompasses a group of highly heterogeneous cancers, of which clear cell RCC (ccRCC) is the most common subtype[1]. Although localized RCC can be managed through ablation, partial or radical nephrectomy or active surveillance[2], more than 30% of individuals eventually present with or develop metastases, which are refractory to conventional chemotherapy and are associated with high mortality[3]. Targeted agents against vascular endothelial growth factor (VEGF) and mammalian target of rapamycin (mTOR) pathways have been created for treating metastatic RCC, but the treatment response is varied, and most cases eventually progress[4]. Downregulation of lactotransferrin promotes metastasis in ccRCC and also renders the ccRCC tumor cells more responsive to mTOR inhibitors, suggesting its potential as a predictor for therapeutic effectiveness[5]. Over the past decade, marked advances in the treatment of cancers have been made, with immunotherapies being the fourth therapeutic technique for cancer therapy after surgery, chemotherapy, and radiotherapy[6]. To date, immune checkpoint inhibitors have been demonstrated to bring survival benefits for metastatic RCC[7]. Nevertheless, most treated patients do not experience effective clinical relief after immunotherapy. For example, the response rate of advanced RCC to nivolumab is only 25%[8]. For a substantial proportion of patients who are not suitable for immunotherapy, tyrosine kinase inhibitors (TKI) monotherapy remains an appropriate first-line therapy, and may bring lifestyle benefits with treatment breaks[9]. Therefore, it is urgently needed to predict the prognosis and response to immunotherapy of ccRCC patients and provide individual therapy.
N6-methyladenosine (m6A) modification is a common modification in mRNA of most eukaryotes. The modification is a reversible process regulated by the balanced activities of “writers” (methyltransferases including WTAP, METTL3, and METTL14), “readers” (binding proteins including YTHDF1, YTHDF2, YTHDF3, YTHDC1, and YTHDC2), and “erasers” (demethylases including ALKBH5 and FTO)[10]. Research has demonstrated that m6A modification plays a pivotal role in the regulation of mRNA metabolism, encompassing various stages such as gene expression, nuclear pre-mRNA processing, cytoplasmic translation, and mRNA decay[11-13]. In addition, previous studies have indicated that aberrant m6A modification is related to various pathophysiological processes, including developmental defects, dysregulation of cell proliferation, differentiation, and death, as well as tumor progression[14-16]. Additionally, m6A methylation plays important roles in the innate and adaptive immune response as well as the tumor immune microenvironment[17]. Moreover, besides exosomes, circulating tumor DNA, and other novel noninvasive tumor biomarkers, substantial evidence has revealed that m6A methylation regulators could be reliable biomarkers for the prediction of the prognosis and/or treatment response of many types of tumors[18-20]. However, whether m6A methylation regulators could be utilized to predict prognosis and immunotherapy response among patients with ccRCC has not been systematically and rigorously investigated.
In the presented study, we developed and validated an m6A-related gene signature based on the pretreatment expression of m6A regulators for predicting the prognosis in ccRCC patients and explored the value of the gene signature in predicting immunotherapy response.
METHODS
Data acquisition
The clinical data and gene expression profiles of ccRCC patients were downloaded from The Cancer Genome Atlas (TCGA), and the biological data were normalized. In total, 530 ccRCC cases from the TCGA-KIRC project were involved in the analysis. A total of 23 m6A regulators were extracted, including nine writers (METTL3, RBM15, WTAP, ZC3H13, METTL14, METTL16, RBM15, RBM15B, and KIAA1429), twelve readers (YTHDF1, YTHDF2, YTHDF3, IGF2BP1, IGF2BP3, YTHDC1, YTHDC2, HNRNPC, HNRNPA2B1, SRSF2, FMR1, and ELF3), and two erasers (FTO and ALKBH5).
Association between prognosis and m6A regulators
To explore the prognosis value of m6A regulators in ccRCC patients, differentially expressed gene (DEG) analyses were performed to assess their differential expression between survivors and non-survivors among patients with ccRCC. Additionally, we used Wilcoxon’s tests to compare the expression levels of m6A regulators between the survivors and non-survivors.
Construction of the gene signature
The gene signature construction flowchart is shown in Figure 1. All cases were randomly split into a training set (n = 371) and a validation set (n = 159) at a ratio of 7:3. The potential associations between the m6A regulators and overall survival (OS) were estimated with univariable Cox regression analyses in the training set. Among 23 m6A regulators, prognosis-related genes with nonzero coefficients were identified via the least absolute shrinkage and selection operator (LASSO) Cox regression algorithm[21]. The log-rank tests were used to compare the OS of the low or high expression of the selected prognosis-related m6A regulators, whose thresholds were selected by the “Survminer” package in the R language.
Figure 1. The study flowchart. TCGA: The Cancer Genome Atlas; TCIA: the Cancer Imaging Archive; MRI: magnetic resonance imaging; LASSO: least absolute shrinkage and selection operator.
According to the results of the LASSO Cox regression, we developed an m6A-related gene signature for OS prediction among patients with ccRCC, and for individual patients, a risk score (m6A score) was calculated using the subsequent regression equation:
where Gi is the selected prognosis-related m6A regulator and bi is the regression coefficient of Gi.
Performance evaluation of the m6A-related gene signature
We evaluated the discrimination of the m6A-related gene signature by using Harrell’s C-index in the training and the validation sets. We also performed time-dependent receiver operating characteristic (ROC) analyses to estimate the predictive accuracy of gene signature. All patients were classified into two m6A subtypes, i.e., low or high m6A score groups, according to their m6A scores, whose threshold was determined using X-tile software in the training set[22]. The Kaplan-Meier OS curves of the two m6A subtypes were compared by the log-rank tests. Subsequently, a series of stratified analyses were conducted to evaluate the potential modification effects according to age, sex, T stage, N stage, M stage, and tumor grade. To determine whether the m6A subtype is an independent predictor of prognosis in ccRCC, the candidate variables were subjected to multivariable analysis based on backward stepwise selection. The discrimination of the significant predictors, as well as their combination, were measured by Harrell’s
The predictive value of the m6A subtype in clinical benefit of immunotherapy
The underlying clinical benefit of immunotherapy for ccRCC patients in each m6A subtype was assessed according to the Tumor Immune Dysfunction and Exclusion (TIDE) score. The TIDE score was calculated through the online website (http://tide.dfci.harvard.edu/)[23].
Furthermore, we also investigated the role of prognosis-related m6A regulators in immunity. The CIBERSORT algorithm was used to evaluate the infiltration of 22 types of immunocytes[24]. Subsequently, the relationship between the identified prognosis-related m6A genes and different types of immunocytes was investigated.
MR images acquisition, images segmentation, and radiomic features extraction
To predict the m6A subtypes individually and noninvasively, we developed an MRI-based radiomic signature. Radiomics is an emerging field of research aiming to extract high-dimensional image-based features from medical images, and to estimate the correlations between the features and potential pathophysiology[25,26]. Radiomics has recently drawn widespread interest in many cancer studies due to its potential to predict prognosis and treatment outcomes and aid in diagnosis[25,27,28]. Figure 1 depicts the radiomics workflow of this study. In total, 47 ccRCC patients from the TCIA-KIRC project were enrolled for radiomics analysis. We downloaded the preoperative MRI images from the TCIA (http://www.cancerimagingarchive.net/). Tumor image segmentation was performed by using 3D Slicer software
Radiomics signature development and performance evaluation
The m6A subtype-related radiomic features were identified by using the LASSO logistic regression algorithm [Supplementary Method 2]. According to the LASSO logistic regression results, we constructed a radiomics signature to measure the probability of the m6A subtype for individual patients. Based on the LASSO regression formula, a radiomics score for each patient could be calculated. The radiomics signature performance was evaluated according to the area under the ROC curve (AUC). The prognostic value of the radiomic signature in ccRCC patients was estimated by performing univariable Cox regression analysis.
Statistical analysis
R statistical software (v 4.0.4) was used to perform statistical tests. Supplementary Method 3 listed the R packages used in our study. P values < 0.05 indicated significant differences.
RESULTS
Association between prognosis and m6A regulators
Baseline characteristics of the enrolled ccRCC patients in the training and validation sets were provided in Supplementary Table 2. Eighteen m6A methylation regulatory genes (YTHDF1, YTHDF2, YTHDF3, YTHDC1, YTHDC2, RBM15, RBM15B, ALKBH5, WTAP, KIAA1429, METTL14, METTL16, ZC3H13, FMR1, FTO, IGF2BP1, IGF2BP2, and IGF2BP3) were differentially expressed between the survivors and non-survivors [Figure 2A]. Among the survivors, the expression levels of YTHDF1, YTHDF2, YTHDF3, YTHDC1, YTHDC2, RBM15, RBM15B, ALKBH5, WTAP, KIAA1429, METTL14, METTL16, ZC3H13, FTO, and FMR1 were significantly decreased compared to the non-survivors, while expression levels of IGF2BP1, IGF2BP2, and IGF2BP3 were significantly increased [Figure 2B].
Figure 2. Association between the expression of m6A methylation regulators and prognosis in ccRCC patients. (A) The heatmap exhibits the expression patterns of the 23 m6A methylation regulators between the survivors and non-survivors. (B) The violin plots show the differential expression of the 23 m6A methylation regulators in the survivors (blue) and the non-survivors (red). ccRCC: Clear cell renal cell carcinoma. *P < 0.05, **P < 0.01, ***P < 0.001.
Construction of the gene signature
As shown in Figure 3A, YTHDF2, YTHDF3, YTHDC1, YTHDC2, RBM15, RBM15B, KIAA1429, METTL14, METTL16, ZC3H13, IGF2BP1, IGF2BP2, IGF2BP3, FMR1, FTO, SRSF2, and HNRNPC were related to the OS in the univariable Cox regression analysis. In addition, five prognosis-related genes (KIAA1429, METTL14, IGF2BP2, IGF2BP3, and SRSF2) were selected by the LASSO Cox regression analysis
Figure 3. Development of the m6A-based gene signature. (A) Univariable Cox regression analyses measuring the predictive value of m6A methylation regulators for overall survival of ccRCC patients. (B) Tuning parameter (λ) selection in the LASSO model used 10-fold cross-validation via minimum criteria. Partial likelihood deviances from the LASSO regression cross-validation procedure were drawn as a function of log(λ). The numbers along the upper x-axis represent the average number of predictors. The red dots show the average deviance values for each model with a given λ, and the vertical bars through the red dots indicate the upper and lower values of the deviance. The dotted vertical lines are plotted at the optimal values where the model provides its best fit to the data. The optimal λ value of 0.038 with log (λ) = -3.279 was identified. (C) LASSO coefficient profiles of the 23 m6A methylation regulators. The dotted vertical line is plotted at the value determined by using 10-fold cross-validation in (B), where optimal λ resulted in 5 nonzero coefficients. (D) Histogram showing the coefficients of the selected m6A regulators in the gene signature. ccRCC: Clear cell renal cell carcinoma; LASSO: least absolute shrinkage and selection operator.
Performance evaluation of the m6A-related gene signature
C-index for the gene signature was 0.708 in the training set (95% confidence interval [CI]: 0.660-0.756) and 0.689 in the validation set (95%CI: 0.611-0.767). The time-ROC curve analysis also demonstrated satisfactory predictive accuracy for the gene signature. In the 3-year and 5-year ROC curves, the AUCs were 0.715 (95%CI: 0.657-0.772) and 0.746 (95%CI: 0.689-0.803) in all patients, 0.724 (95%CI: 0.656-0.792) and 0.744 (95%CI: 0.677-0.812) in the training set, and 0.698 (95%CI: 0.590-0.806) and 0.766
Figure 4. Correlation between the gene signature and prognosis in ccRCC patients. (A-C) Time-ROC curve analyses of the m6A score in all patients (A), the training set (B), and the validation set (C), respectively. (D-F) Kaplan-Meier survival curves of patients divided into low and high m6A score groups in all patients (D), the training set (E), and the validation set (F), respectively. ccRCC: Clear cell renal cell carcinoma; ROC: receiver operator characteristic curve.
Figure 5. Stratified analyses of the m6A subtype. Stratified analyses were applied to determine the performance of the m6A subtype in various subgroups according to age (A and B), sex (C and D), T stage (E and F), N stage (G and H), M stage (I and J), and tumor grade (K and L).
Based on the multivariable Cox regression analyses, five variables were determined as the independent predictors of OS, including age, tumor grade, N stage, M stage, and m6A subtype [Supplementary Table 3]. Moreover, the m6A score achieved better discrimination than other clinical predictors
Genetic mutation landscape analysis
A total of 391 ccRCC patients with genetic mutation data were enrolled to analyze the genetic mutation landscape for ccRCC lesions, including 105 patients with high m6A scores and 286 patients with low m6A scores. The identified somatic mutations were compared between the two different m6A subtypes
The predictive value of the m6A subtype in clinical benefit of immunotherapy
To further assess the predictive value of the m6A subtype in immunotherapy efficacy, the TIDE score, T cell dysfunction score, microsatellite instability (MSI) score, and T cell exclusion score were calculated for each patient. As shown in Figures 6A and B, the TIDE score and T cell dysfunction score were significantly lower in patients with low m6A scores than those with high m6A scores. Meanwhile, a lower m6A score was associated with a higher MSI score [Figure 6C]. According to these findings, patients in the low m6A score group were more likely to achieve a positive immunotherapy response. However, the T cell exclusion score did not differ significantly between the two groups [Figure 6D].
Figure 6. The probable benefit of patients for immunotherapy in different m6A subtypes. (A) TIDE score, (B) T cell dysfunction score, (C) MSI, and (D) T cell exclusion score in different groups of m6A subtypes. The scores between the two groups were compared through the Wilcoxon tests. TIDE: Tumor Immune Dysfunction and Exclusion; MSI: microsatellite instability.
Moreover, as shown in Figure 7, among those selected prognosis-related m6A regulators, IGF2BP3 was positively correlated with activated CD4+ memory T cells. METTL14 was negatively correlated with follicular helper T cells and regulatory T cells. KIAA1429 was negatively correlated with follicular helper T cells, regulatory T cells, and activated NK cells.
Figure 7. The relationship of the selected m6A regulators with different types of immune cells. (A) The heatmap shows the relationship between the expression levels of the selected m6A regulators and different types of immunocytes. (B-G) Correlation plots exhibit the relationship between the selected m6A regulators and different types of immunocytes. Only the relationship with the absolute value of correlation coefficients no less than 0.3 was displayed.
Radiomics signature development and performance evaluation
In total, 7 prognosis-related features were determined by the LASSO logistic regression [Figures 8A and B]. The selected prognosis-related features’ coefficients are provided in Figure 8C. An AUC of 0.915 (95%CI: 0.835-0.996; Figure 8D) suggested that the radiomics signature had satisfactory discrimination for m6A subtypes prediction. Based on the maximum Youden index, an optimal radiomics score cutoff was chosen as -0.537. The waterfall plot illustrates the distribution of radiomics scores and m6A subtypes for all patients, with the dividing line delineated at the threshold value [Figure 8E]. Moreover, based on the univariable Cox regression, the radiomic signature was determined to be a significant predictor of the OS among patients with ccRCC (hazard ratio: 1.717, 95%CI: 1.080-2.730; P = 0.022).
Figure 8. Development and performance evaluation of the MRI-based radiomics signature. (A) Tuning parameter (λ) selection in the LASSO model used 10-fold cross-validation via minimum criteria. The optimal λ value of 0.096 with log (λ) = -2.339 was identified. (B) LASSO coefficient profiles of the 1,316 radiomic features. The dotted vertical line is drawn at the value selected using 10-fold cross-validation in (A), where optimal λ resulted in 7 nonzero coefficients. (C) Histogram showing the coefficients of the selected features in the radiomics signature. The radiomics score was calculated as a linear combination of the 7 selected features weighted by their respective coefficients. (D) ROC curve of the gene signature. (E) Waterfall plot for distribution of radiomics scores and m6A subtypes in all patients. LASSO: Least absolute shrinkage and selection operator; ROC: receiver operator characteristic curve; LoG: laplacian of gaussian; GLSZM: gray level size zone matrix; GLRLM: gray level run length matrix; GLCM: gray level cooccurence matrix; LRHGLE: long run high gray level emphasis; IDN: inverse difference normalized; LAHGLE: large area high gray level emphasis; IMC2: informal measure of correlation 2.
DISCUSSION
According to the pretreatment expression of 5 prognosis-related m6A methylation regulators, we developed and validated a gene signature to predict the ccRCC patient prognosis, and assessed the predictive value of the gene signature for immunotherapy response. According to the gene signature, patients could be categorized into two m6A subtypes, indicating different prognoses. Moreover, we developed an MRI-based radiomic signature, which can serve as a noninvasive tool for individualized prediction of the m6A subtypes. It would help develop therapeutic schemes and promote precision medicine.
Our study revealed that eighteen m6A regulators (YTHDF1, YTHDF2, YTHDF3, YTHDC1, YTHDC2, RBM15, RBM15B, ALKBH5, WTAP, KIAA1429, METTL14, METTL16, ZC3H13, FMR1, FTO, IGF2BP1, IGF2BP2, and IGF2BP3) were differentially expressed between survivors and non-survivors, whose expression was related to OS as well. Our findings preliminarily suggest that these eighteen m6A regulators may affect the malignant behavior of renal carcinoma cells.
Subsequently, by incorporating five selected m6A regulators (KIAA1429, METTL14, IGF2BP2, IGF2BP3, and SRSF2), we developed a gene signature to predict OS among ccRCC patients. The gene signature can accurately predict the prognosis of ccRCC patients. Moreover, both the TIDE score and T-cell dysfunction score were lower in the low m6A score group, while the MSI score was higher. These scores can indicate the potential ability of immune evasion of cancer and could be used to predict immunotherapy response[23]. In addition, three prognosis-related m6A regulators (IGF2BP3, METTL14, and KIAA1429) were found to be correlated with specific immune cell types. These results indicate that the m6A modification plays a vital role in the tumor immune microenvironment. In light of this, our results demonstrated that patients with low m6A scores may have a lower probability of tumor cells escaping from the surveillance of the immune system and, thus, would be more likely to achieve a positive immunotherapy response. This also indicates the favorable value of the m6A subtype in predicting immunotherapy benefits. Our study will provide novel insights into the regulation of tumor immune microenvironment. Additionally, the presented gene signature will aid clinicians in determining whether the prognosis or immunotherapy response of a patient is good or bad, thus facilitating the development of more appropriate treatment plans that are more conducive to the allocation of medical and health resources.
In the present study, based on LASSO Cox regression analysis, five m6A regulators, including METTL14, KIAA1429, IGF2BP2, IGF2BP3, and SRSF2, were identified as critical predictors of OS in ccRCC patients. Among them, METTL14 is a close counterpart of METTL3 and an integral part of the multiprotein methyltransferase complex[30], which can stabilize METTL3, maintain complex integrity, and facilitate RNA binding[31]. Compared to each protein alone, the stabilized complex formed by the METTL3-METTL14 heterodimer exhibits enhanced methyltransferase activity[30,32]. Previous studies revealed the low expression of METTL14 in ccRCC, and the downregulation of METTL14 is related to poor OS[33,34], which was also found in our study. In addition to ccRCC, decreased expression of METTL14 was demonstrated in glioblastoma stem cells[35], whereas upregulation of METTL14 in acute myeloid leukemia promoted leukemogenesis[36].
The RNA-binding protein KIAA1429 is localized in nuclear speckles[37]. Knockdown of KIAA1429 leads to a diminished expression of m6A, with a decrease that is more pronounced compared to depletion observed by the knockdown of METTL3 or METTL4 alone, indicating the important role of KIAA1429 in the multiprotein methyltransferase complex[37]. Consistent with our study, a previous study revealed that the KIAA1429 expression is decreased in ccRCC patients, and downregulation is associated with shortened survival following nephrectomy[33]. However, a few studies supposed that KIAA1429 might enhance the generation and progression of tumors, such as hepatocellular carcinoma and breast cancer[38,39].
In agreement with our study, several previous studies also demonstrated that elevated expression of IGF2BP2 and IGF2BP3 was significantly related to shortened OS of ccRCC patients[34,40,41]. Moreover, the expression of IGF2BP2 and IGF2BP3 was positively related to activated central memory CD8+ T cells and CD4+ T cells[40]. Therefore, we hypothesized that the dysregulation of IGF2BP2 and IGF2BP3 may influence the associated immune cells and thus affect the survival of ccRCC patients. In addition, IGF2BP2 was reported to be differentially expressed in pancreatic cancer, and its high expression promotes tumor growth by stimulating the PI3K/Akt pathway[42].
The SRSF2 protein belongs to the SR family of proteins that regulate constitutive and alternative splicing[43]. SRSF2 is a key predictor of OS in ccRCC patients in this study. SRSF2 causes apoptosis by regulating the alternative splicing of some apoptosis-associated genes in lung cancer[44], suggesting that SRSF2 can lead to tumorigenesis. Several studies also revealed that silencing of SRSF2 resulted in the upregulation of TP53, which generally functions as an apoptotic protein[45,46]. However, p53 can also be considered an activator of anti-apoptosis in several tumor cells[47-49]. In renal cancer, upregulation of p53 is related to tumor metastasis and worse prognosis in patients[50].
To further elucidate the biological implications of our m6A-related gene signature, it is noteworthy to focus on the behavior of cancer stem cells (CSCs) in ccRCC, given their significant impact on tumor relapse and treatment resistance. A recent study discovered a subset of CD133+/CD24+ CSCs in ccRCC, which exhibited self-renewal and clonogenic capabilities, leveraging pathways such as Notch, Wnt, and Hedgehog - commonly involved in early embryogenesis and also affected by m6A modifications[51]. Investigating the intersection of m6A methylation regulators with these CSC pathways could provide novel insights into their roles in sustaining CSC populations and affecting tumor heterogeneity, thereby potentially improving the predictive accuracy of our gene signature for both prognosis and therapeutic responses. Other significant biomarkers such as MUC1, a glycoprotein known for its role in epithelial tumor biology, may also play a vital role in how m6A modifications influence ccRCC pathology. MUC1 is frequently overexpressed in RCC and influences crucial processes including cell proliferation and metabolic reprogramming[52,53]. The interaction between MUC1 overexpression and m6A methylation patterns could provide deeper insights into the regulatory networks that drive ccRCC progression and resistance to therapy. Exploring these connections might uncover new layers of complexity within the tumor microenvironment and highlight potential synergies between epigenetic modifications and membrane protein dynamics, offering novel avenues for targeted therapeutic interventions[52].
Notably, the m6A subtypes can only be determined in an invasive way. For patients who are planning to receive neoadjuvant immunotherapy or patients who are unable to undergo surgical treatment in an advanced stage, a tumor biopsy is required to obtain information about m6A subtypes; however, this procedure is prone to some adverse effects such as bleeding and tumor dissemination. Therefore, we developed a reliable radiomic signature to predict the m6A subtypes noninvasively in ccRCC patients. The high-throughput features could provide more comprehensive information about tumors and would be more sensitive to determining the m6A subtype, which will be helpful in estimating the prognosis and immunotherapy response. Additionally, our research design may be applicable to other cancer studies. The study results may also offer new perspectives on cancer diagnosis, treatment, or patient management in other tumor types.
There are several limitations to our study. First, even though substantial evidence has shown that m6A regulatory genes are crucial for predicting the prognosis of ccRCC patients, the underlying mechanisms remain unknown and warrant further exploration by experiments on cell lines and human tissues. Second, due to the retrospective design of the study, potential biases are present, such as selection bias, information bias, and confounding bias. Third, because of the shortcomings inherent in bioinformatics analysis performed in this study, in future clinical applications, data from different sources need to be standardized to ensure effective integration, and medical professionals need to receive appropriate training to understand and apply the analysis results of this study. Moreover, the prediction efficacy of the gene signature and radiomic signature needs to be further externally validated to guarantee the generality and reliability of this study.
CONCLUSIONS
In our study, we identified five critical prognosis-related m6A regulators in ccRCC patients. Additionally, the presented m6A-related gene signature could aid in predicting the OS and immunotherapy response of ccRCC patients. Moreover, the radiomic signature can be used as a noninvasive tool for predicting the m6A subtypes individually. It is necessary to verify the robustness of the two signatures by multicenter validation before they are applied in clinical settings.
DECLARATIONS
Acknowledgments
We thank the Cancer Genome Atlas (TCGA) for the gene and clinical datasets. We also thank the Cancer Imaging Archive (TCIA) for the MRI datasets. We also thank Figdraw for the assistance in drawing graphical abstract (www.figdraw.com).
Authors’ contributions
Designed the study: Chen W, Lin T, Yang G, Huang W
Processed the images: Wang Z, Zeng L, Lin H
Supervised the study: Yang G, Huang W
All the authors analyzed and interpreted the data, and wrote, reviewed, and/or revised the manuscript, and approved the submitted version
Availability of data and materials
Publicly available datasets were analyzed in this study. This data can be found here: the Cancer Genome Atlas (TCGA, https://portal.gdc.cancer.gov/); the Cancer Imaging Archive (TCIA, http://www.cancerimagingarchive.net/).
Financial support and sponsorship
None.
Conflicts of interest
All authors declare that there are no conflicts of interest.
Ethical approval and consent to participate
Not applicable.
Consent for publication
Not applicable.
Copyright
© The Author(s) 2024.
Supplementary Materials
REFERENCES
2. Ljungberg B, Bensalah K, Canfield S, et al. EAU guidelines on renal cell carcinoma: 2014 update. Eur Urol 2015;67:913-24.
3. Wolff I, May M, Hoschke B, et al. Do we need new high-risk criteria for surgically treated renal cancer patients to improve the outcome of future clinical trials in the adjuvant setting? Results of a comprehensive analysis based on the multicenter CORONA database. Eur J Surg Oncol 2016;42:744-50.
4. Motzer RJ, Hutson TE, McCann L, Deen K, Choueiri TK. Overall survival in renal-cell carcinoma with pazopanib versus sunitinib. N Engl J Med 2014;370:1769-70.
5. Aweys H, Lewis D, Sheriff M, et al. Renal cell cancer - insights in drug resistance mechanisms. Anticancer Res 2023;43:4781-92.
6. Hsieh JJ, Purdue MP, Signoretti S, et al. Renal cell carcinoma. Nat Rev Dis Primers 2017;3:17009.
7. Choueiri TK, Motzer RJ. Systemic therapy for metastatic renal-cell carcinoma. N Engl J Med 2017;376:354-66.
8. Motzer RJ, Escudier B, McDermott DF, et al. Nivolumab versus everolimus in advanced renal-cell carcinoma. N Engl J Med 2015;373:1803-13.
9. Brown JE, Royle KL, Gregory W, et al. Temporary treatment cessation versus continuation of first-line tyrosine kinase inhibitor in patients with advanced clear cell renal cell carcinoma (STAR): an open-label, non-inferiority, randomised, controlled, phase 2/3 trial. Lancet Oncol 2023;24:213-27.
10. Yang Y, Hsu PJ, Chen YS, Yang YG. Dynamic transcriptomic m6A decoration: writers, erasers, readers and functions in RNA metabolism. Cell Res 2018;28:616-24.
11. Paramasivam A, Priyadharsini JV, Raghunandhakumar S. Implications of m6A modification in autoimmune disorders. Cell Mol Immunol 2020;17:550-1.
12. Paramasivam A, Vijayashree Priyadharsini J. Novel insights into m6A modification in circular RNA and implications for immunity. Cell Mol Immunol 2020;17:668-9.
13. Paramasivam A, Vijayashree Priyadharsini J, Raghunandhakumar S. N6-adenosine methylation (m6A): a promising new molecular target in hypertension and cardiovascular diseases. Hypertens Res 2020;43:153-4.
14. Fu Y, Dominissini D, Rechavi G, He C. Gene expression regulation mediated through reversible m6A RNA methylation. Nat Rev Genet 2014;15:293-306.
15. Tong J, Cao G, Zhang T, et al. m6A mRNA methylation sustains Treg suppressive functions. Cell Res 2018;28:253-6.
16. Pinello N, Sun S, Wong JJ. Aberrant expression of enzymes regulating m6A mRNA methylation: implication in cancer. Cancer Biol Med 2018;15:323-34.
17. Guo L, Yang H, Zhou C, Shi Y, Huang L, Zhang J. N6-methyladenosine RNA modification in the tumor immune microenvironment: novel implications for immunotherapy. Front Immunol 2021;12:773570.
18. Wang P, Wang X, Zheng L, Zhuang C. Gene signatures and prognostic values of m6A regulators in hepatocellular carcinoma. Front Genet 2020;11:540186.
19. Zhao R, Li B, Zhang S, et al. The N6-methyladenosine-modified pseudogene HSPA7 correlates with the tumor microenvironment and predicts the response to immune checkpoint therapy in glioblastoma. Front Immunol 2021;12:653711.
20. Xu W, Tian X, Liu W, et al. m6A regulator-mediated methylation modification model predicts prognosis, tumor microenvironment characterizations and response to immunotherapies of clear cell renal cell carcinoma. Front Oncol 2021;11:709579.
21. Tibshirani R. Regression shrinkage and selection via the lasso: a retrospective. J R Stat Soc Series B 2011;73:273-82.
22. Camp RL, Dolled-Filhart M, Rimm DL. X-tile: a new bio-informatics tool for biomarker assessment and outcome-based cut-point optimization. Clin Cancer Res 2004;10:7252-9.
23. Jiang P, Gu S, Pan D, et al. Signatures of T cell dysfunction and exclusion predict cancer immunotherapy response. Nat Med 2018;24:1550-8.
24. Chen B, Khodadoust MS, Liu CL, Newman AM, Alizadeh AA. Profiling tumor infiltrating immune cells with CIBERSORT. In: von Stechow L, editor. Cancer Systems Biology. New York: Springer; 2018. pp. 243-59.
25. Lambin P, Leijenaar RTH, Deist TM, et al. Radiomics: the bridge between medical imaging and personalized medicine. Nat Rev Clin Oncol 2017;14:749-62.
26. Zheng J, Yu H, Batur J, et al. A multicenter study to develop a non-invasive radiomic model to identify urinary infection stone in vivo using machine-learning. Kidney Int 2021;100:870-80.
27. Cai J, Zheng J, Shen J, et al. A radiomics model for predicting the response to bevacizumab in brain necrosis after radiotherapy. Clin Cancer Res 2020;26:5438-47.
28. Zheng J, Kong J, Wu S, et al. Development of a noninvasive tool to preoperatively evaluate the muscular invasiveness of bladder cancer using a radiomics approach. Cancer 2019;125:4388-98.
29. van Griethuysen JJM, Fedorov A, Parmar C, et al. Computational radiomics system to decode the radiographic phenotype. Cancer Res 2017;77:e104-7.
30. Cao G, Li HB, Yin Z, Flavell RA. Recent advances in dynamic m6A RNA modification. Open Biol 2016;6:160003.
31. Wang X, Feng J, Xue Y, et al. Structural basis of N6-adenosine methylation by the METTL3-METTL14 complex. Nature 2016;534:575-8.
32. Liu J, Yue Y, Han D, et al. A METTL3-METTL14 complex mediates mammalian nuclear RNA N6-adenosine methylation. Nat Chem Biol 2014;10:93-5.
33. Gundert L, Strick A, von Hagen F, et al. Systematic expression analysis of m6A RNA methyltransferases in clear cell renal cell carcinoma. BJUI Compass 2021;2:402-11.
34. Xu T, Gao S, Ruan H, et al. METTL14 acts as a potential regulator of tumor immune and progression in clear cell renal cell carcinoma. Front Genet 2021;12:609174.
35. Cui Q, Shi H, Ye P, et al. m6A RNA methylation regulates the self-renewal and tumorigenesis of glioblastoma stem cells. Cell Rep 2017;18:2622-34.
36. Weng H, Huang H, Wu H, et al. METTL14 inhibits hematopoietic stem/progenitor differentiation and promotes leukemogenesis via mRNA m6A modification. Cell Stem Cell 2018;22:191-205.e9.
37. Xie W, Wei L, Guo J, Guo H, Song X, Sheng X. Physiological functions of Wilms’ tumor 1-associating protein and its role in tumourigenesis. J Cell Biochem 2019;120:10884-92.
38. Qu N, Qin S, Zhang X, et al. Multiple m6A RNA methylation modulators promote the malignant progression of hepatocellular carcinoma and affect its clinical prognosis. BMC Cancer 2020;20:165.
39. Qian JY, Gao J, Sun X, et al. KIAA1429 acts as an oncogenic factor in breast cancer by regulating CDK1 in an N6-methyladenosine-independent manner. Oncogene 2019;38:6123-41.
40. Li J, Cao J, Liang C, Deng R, Li P, Tian J. The analysis of N6-methyladenosine regulators impacting the immune infiltration in clear cell renal cell carcinoma. Med Oncol 2022;39:41.
41. Xing Q, Luan J, Liu S, Ma L, Wang Y. Six RNA binding proteins (RBPs) related prognostic model predicts overall survival for clear cell renal cell carcinoma and is associated with immune infiltration. Bosn J Basic Med Sci 2022;22:435-52.
42. Xu X, Yu Y, Zong K, Lv P, Gu Y. Up-regulation of IGF2BP2 by multiple mechanisms in pancreatic cancer promotes cancer proliferation by activating the PI3K/Akt signaling pathway. J Exp Clin Cancer Res 2019;38:497.
43. Long JC, Caceres JF. The SR protein family of splicing factors: master regulators of gene expression. Biochem J 2009;417:15-27.
44. Merdzhanova G, Edmond V, De Seranno S, et al. E2F1 controls alternative splicing pattern of genes involved in apoptosis through upregulation of the splicing factor SC35. Cell Death Differ 2008;15:1815-23.
45. Haupt S, Berger M, Goldberg Z, Haupt Y. Apoptosis - the p53 network. J Cell Sci 2003;116:4077-85.
46. Kędzierska H, Popławski P, Hoser G, et al. Decreased expression of SRSF2 splicing factor inhibits apoptotic pathways in renal cancer. Int J Mol Sci 2016;17:1598.
47. Garner E, Martinon F, Tschopp J, Beard P, Raj K. Cells with defective p53-p21-pRb pathway are susceptible to apoptosis induced by p84N5 via caspase-6. Cancer Res 2007;67:7631-7.
48. Mercer J, Figg N, Stoneman V, Braganza D, Bennett MR. Endogenous p53 protects vascular smooth muscle cells from apoptosis and reduces atherosclerosis in ApoE knockout mice. Circ Res 2005;96:667-74.
49. Amin AR, Thakur VS, Gupta K, et al. Restoration of p53 functions protects cells from concanavalin A-induced apoptosis. Mol Cancer Ther 2010;9:471-9.
50. Haitel A, Wiener HG, Baethge U, Marberger M, Susani M. mdm2 expression as a prognostic indicator in clear cell renal cell carcinoma: comparison with p53 overexpression and clinicopathological parameters. Clin Cancer Res 2000;6:1840-4.
51. Lasorsa F, Rutigliano M, Milella M, et al. Cancer stem cells in renal cell carcinoma: origins and biomarkers. Int J Mol Sci 2023;24:13179.
52. Lucarelli G, Netti GS, Rutigliano M, et al. MUC1 expression affects the immunoflogosis in renal cell carcinoma microenvironment through complement system activation and immune infiltrate modulation. Int J Mol Sci 2023;24:4814.
Cite This Article
Export citation file: BibTeX | EndNote | RIS
OAE Style
Chen W, Lin T, Wang Z, Zeng L, Lin H, Yang G, Huang W. Predictive value of m6A regulators in prognosis and immunotherapy response of clear cell renal cell carcinoma: a bioinformatics and radiomics analysis. J Cancer Metastasis Treat 2024;10:21. http://dx.doi.org/10.20517/2394-4722.2024.43
AMA Style
Chen W, Lin T, Wang Z, Zeng L, Lin H, Yang G, Huang W. Predictive value of m6A regulators in prognosis and immunotherapy response of clear cell renal cell carcinoma: a bioinformatics and radiomics analysis. Journal of Cancer Metastasis and Treatment. 2024; 10: 21. http://dx.doi.org/10.20517/2394-4722.2024.43
Chicago/Turabian Style
Wanqi Chen, Tuanyu Lin, Zhenshan Wang, Liting Zeng, Haitao Lin, Guisheng Yang, Weipeng Huang. 2024. "Predictive value of m6A regulators in prognosis and immunotherapy response of clear cell renal cell carcinoma: a bioinformatics and radiomics analysis" Journal of Cancer Metastasis and Treatment. 10: 21. http://dx.doi.org/10.20517/2394-4722.2024.43
ACS Style
Chen, W.; Lin T.; Wang Z.; Zeng L.; Lin H.; Yang G.; Huang W. Predictive value of m6A regulators in prognosis and immunotherapy response of clear cell renal cell carcinoma: a bioinformatics and radiomics analysis. J. Cancer. Metastasis. Treat. 2024, 10, 21. http://dx.doi.org/10.20517/2394-4722.2024.43
About This Article
Special Issue
Copyright
Data & Comments
Data
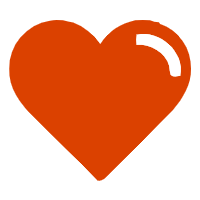
Comments
Comments must be written in English. Spam, offensive content, impersonation, and private information will not be permitted. If any comment is reported and identified as inappropriate content by OAE staff, the comment will be removed without notice. If you have any queries or need any help, please contact us at support@oaepublish.com.