Three key domains for optimizing preoperative preparedness in plastic surgery with AI
Abstract
Artificial Intelligence (AI) is reshaping preoperative preparation for both patients and surgeons in plastic surgery, offering innovative tools that improve efficiency, precision, and outcomes. This manuscript examines the growing role of AI in preoperative patient education, surgeon preparation, and clinical workflows, and provides an overview of its main domains of impact. AI-powered tools show great promise in consultations, providing personalized, clear patient education, simplifying administrative tasks such as drafting consultation letters, and enhancing communication. For surgeons, AI can support preoperative planning with reinforcement learning, advanced imaging algorithms, and virtual simulations. These technologies assist in accurate surgical decision making, as seen in deep inferior epigastric artery perforator (DIEP) flap breast reconstruction, where AI may be able to reduce perforator analysis times while maintaining precision. In aesthetic surgery, AI-driven 3D imaging and generative algorithms enable realistic postoperative simulations, improving communication and helping patients set realistic expectations. From a patient communication perspective, AI promotes patient-centered care by simplifying medical jargon, making information more accessible, and fostering better understanding and adherence. Despite challenges such as ethical considerations, data security, and algorithmic bias, AI’s integration into preoperative workflows highlights its potential to improve patient and surgeon experiences. By enhancing patient education, refining surgical strategies, and optimizing preparation, AI is redefining how care is delivered in plastic surgery, aiming for better patient satisfaction and outcomes. Collaboration among clinicians, engineers, and ethicists remains crucial to ensure the responsible use of AI, balancing innovation with the core values of medical care.
Keywords
INTRODUCTION
The term Artificial Intelligence (AI), introduced by John McCarthy - the “Father of AI” - has driven a technological revolution across countless industries, including surgery. McCarthy’s pioneering programming language laid the foundation for AI’s rapid evolution, enabling machines to analyze vast amounts of data and recognize patterns with remarkable accuracy. In healthcare, these capabilities have had an impact on both diagnostics and treatments[1]. In orthopedic surgery, AI tools can create detailed 3D models from CT or MRI scans, providing surgeons with clear visualizations of the hip joint[2]. AI can enhance kidney stone management in urology by assisting with procedure planning, such as lithotripsy. It predicts stone fragmentation patterns and determines optimal treatment strategies, leading to more efficient and personalized care[3-5]. Additionally, in plastic surgery and microsurgery, there has been an increased interest in using AI to improve efficiency, especially in the preoperative planning phase. Algorithms have given life to personalized preoperative assessment, surgical planning and outcome simulation, and postoperative monitoring[6-9].
Plastic surgery has always been a field driven by innovation, with surgeon-innovators leading advancements in areas like regenerative medicine, craniofacial surgery, transplantation, and hand surgery. Today, we have an opportunity to take this spirit of innovation further by embracing technology to improve patient care. Robotic surgery can enhance precision and safety in the operating room, while AI offers even broader possibilities. Unlike robotic surgery, AI spans the entire patient journey - from the first consultation and preoperative planning to surgery itself and postoperative recovery. By integrating these tools, we can deliver better outcomes and greater efficiency for our patients.
With increasing awareness, we have seen a considerable number of research on the use of AI in medicine. However, there is limited literature available specifically addressing its application in the preoperative setting. Bearing in mind the existing literature, as well as our own experience[6,10], we have identified three key domains where AI has an impact on preoperative awareness [Table 1]:
Overview of three main domains where AI has an influence preoperatively
Aspect | Key features | AI applications |
Patient communication | Builds trust, simplifies information, enhances consent | AI consultations, automated documentation, predictive models |
Surgical planning | Optimizes decisions, reduces variability | Reinforcement learning, imaging for perforator mapping, simulations |
Education and preparation | Trains surgeons, sets patient expectations | AI simulators, 3D imaging, integration in training programs |
· Patient communication: Where AI tools enhance workflows, improve informed consent, and reduce administrative tasks.
· Preoperative surgical planning: Where we focused on surgical decision making, breast reconstruction, microsurgery, aesthetic surgery and head and neck.
· Preoperative education and preparation.
This manuscript explores and explains how AI can impact the preoperative phase in plastic surgery, focusing on its benefits, current applications, and challenges.
METHODS
We conducted a systematic literature search following the Preferred Reporting Items for Systematic Reviews and Meta-Analyses (PRISMA) guidelines to evaluate the role of AI specifically within the preoperative phase of plastic surgery. Two major medical databases, PubMed and Embase, were systematically searched to identify relevant literature.
In PubMed, the search utilized Medical Subject Headings (MeSH) terms combined with keywords. The structured search strategy included the following terms: [“Surgery, Plastic”(Mesh) OR “Plastic Surgery Procedures”(Mesh)] AND [“Artificial Intelligence”(Mesh) OR “Deep Learning”(Mesh)] AND “preoperative planning”.
In Embase, we employed Emtree terms in our systematic search, incorporating the terms (“plastic surgery”/exp) AND (“artificial intelligence”/exp OR “deep learning”/exp OR “machine learning”/exp) AND “preoperative evaluation”/exp.
Initial screening involved the evaluation of titles and abstracts to identify articles relevant to our research question, specifically targeting the application of AI within the preoperative phase of plastic surgery. Articles clearly irrelevant to these criteria were excluded at this stage.
Full-text articles deemed potentially relevant during initial screening were subsequently reviewed in detail to assess their eligibility based on our inclusion and exclusion criteria. Articles were included if they addressed the direct use or implications of AI in patient communication, surgical planning, or education and preparation within the preoperative context of plastic surgery. Exclusions included articles focused on AI applications exclusively in intraoperative or postoperative phases, articles unrelated to plastic surgery, or those lacking sufficient detail on preoperative AI applications.
Data extraction was performed independently by two reviewers, and any discrepancies were resolved by consensus.
RESULTS
The combined database search initially yielded a total of 210 articles (141 from PubMed, 69 from Embase). After removing duplicates (n = 4), 206 unique articles remained for screening. Based on titles and abstracts screening, 86 articles were excluded due to irrelevance, leaving 120 articles eligible for full-text assessment. Upon detailed full-text evaluation, an additional 103 articles were excluded for reasons including exclusive focus on intra- or postoperative applications, lack of relevance to plastic surgery, or insufficient detail about preoperative AI applications. Ultimately, 17 articles met the inclusion criteria and were selected for analysis [Figure 1].
The 17 selected articles were systematically categorized into three specific subdomains relevant to preoperative preparedness. Articles often overlapped and could be fit into more than 1 subdomain
Articles per subdomain from the systematic search
Articles used in “Patient communication and preoperative preparation” | Authors | Publication date |
Machine learning, deep learning, artificial intelligence and aesthetic plastic surgery: a qualitative systematic review | Nogueira R, Eguchi M, Kasmirski J, de Lima BV, Dimatos DC, Lima DL, Glatter R, Tran DL, Piccinini PS | 2025 Jan |
MRA for preoperative planning and postoperative management of perforator flap surgeries: a review Artificial intelligence in facial plastics and reconstructive surgery | Nanda Deepa Thimmappa | 2023 Aug 17 |
Evaluating artificial intelligence’s role in teaching the reporting and interpretation of computed tomographic angiography for preoperative planning of the deep inferior epigastric artery perforator flap | Lim B, Cevik J, Seth I, Sofiadellis F, Ross RJ, Rozen WM, Cuomo R | 2024 Apr 5 |
The ability of artificial intelligence chatbots ChatGPT and Google Bard to accurately convey preoperative information for patients undergoing ophthalmic surgeries | Patil NS, Huang R, Mihalache A, Kisilevsky E, Kwok J, Popovic MM, Nassrallah G, Chan C, Mallipatna A, Kertes PJ, Muni RH | 2024 Jun 1 |
The future of artificial intelligence in facial plastic surgery | Fortune-Ely M, Achanta M, Song MSH | 2023 Dec 1 |
Evaluating the quality and readability of ChatGPT-generated patient-facing medical information in rhinology | Fazilat AZ, Brenac C, Kawamoto-Duran D, Berry CE, Alyono J, Chang MT, Liu DT, Patel ZM, Tringali S, Wan DC, Fieux M | 2025 Apr |
A history of innovation: tracing the evolution of imaging modalities for the preoperative planning of microsurgical breast reconstruction | Cevik J, Seth I, Hunter-Smith DJ, Rozen WM | 2023 Aug 11 |
Current and future photography techniques in aesthetic surgery | Parsa S, Basagaoglu B, Mackley K, Aitson P, Kenkel J, Amirlak B | 2021 Nov 29 |
Articles used in “AI in preoperative surgical planning” | Authors | Publication date |
Machine learning, deep learning, artificial intelligence and aesthetic plastic surgery: a qualitative systematic review | Nogueira R, Eguchi M, Kasmirski J, de Lima BV, Dimatos DC, Lima DL, Glatter R, Tran DL, Piccinini PS | 2025 Jan |
MRA for preoperative planning and postoperative management of perforator flap surgeries: a review | Nanda Deepa Thimmappa | 2023 Aug 17 |
Artificial intelligence in maxillofacial and facial plastic and reconstructive surgery | Fung E, Patel D, Tatum S | 2024 Aug 1 |
Simulation and artificial intelligence in rhinoplasty: a systematic review | Eldaly AS, Avila FR, Torres-Guzman RA, Maita K, Garcia JP, Palmieri Serrano L, Forte AJ | 2022 Oct |
Artificial intelligence-enabled simulation of gluteal augmentation: a helpful tool in preoperative outcome simulation? | Knoedler L, Odenthal J, Prantl L, Oezdemir B, Kehrer A, Kauke-Navarro M, Matar DY, Obed D, Panayi AC, Broer PN, Chartier C, Knoedler S | 2023 May |
Automatic detection of perforators for microsurgical reconstruction | Mavioso C, Araújo RJ, Oliveira HP, Anacleto JC, Vasconcelos MA, Pinto D, Gouveia PF, Alves C, Cardoso F, Cardoso JS, Cardoso MJ | 2020 Apr |
Artificial intelligence in facial plastics and reconstructive surgery | Park KW, Diop M, Willens SH, Pepper JP | 2024 Oct |
Applications of artificial intelligence in facial plastic and reconstructive surgery: a systematic review | Souza S, Bhethanabotla RM, Mohan S | 2024 Aug 1 |
Artificial intelligence applications and ethical challenges in oral and maxillo-facial cosmetic surgery: a narrative review | Rokhshad R, Keyhan SO, Yousefi P | 2023 Mar 13 |
A history of innovation: tracing the evolution of imaging modalities for the preoperative planning of microsurgical breast reconstruction | Cevik J, Seth I, Hunter-Smith DJ, Rozen WM | 2023 Aug 11 |
Current and future photography techniques in aesthetic surgery | Parsa S, Basagaoglu B, Mackley K, Aitson P, Kenkel J, Amirlak B | 2021 Nov 29 |
Analysis of surgical outcome after upper eyelid surgery by computer vision algorithm using face and facial landmark detection | Bahçeci Şimşek İ, Şirolu C | 2021 Oct |
Predicting patient-reported outcomes following mastectomy and breast reconstruction: application of machine learning techniques to real patient data | Hassan AM, Biaggi-Ondina A, Rajesh A | 2022 Jun 18 |
Articles used in “Preoperative education and preparation” | Authors | Publication date |
Machine learning, deep learning, artificial intelligence and aesthetic plastic surgery: a qualitative systematic review | Nogueira R, Eguchi M, Kasmirski J, de Lima BV, Dimatos DC, Lima DL, Glatter R, Tran DL, Piccinini PS | 2025 Jan |
Artificial intelligence in facial plastics and reconstructive surgery | Park KW, Diop M, Willens SH, Pepper JP | 2024 Oct |
Preoperative patient guidance and education in aesthetic breast plastic surgery: a novel proposed application of artificial intelligence large language models | Abi-Rafeh J, Bassiri-Tehrani B, Kazan R, Furnas H, Hammond D, Adams WP Jr, Nahai F | 2024 Aug 13 |
A history of innovation: tracing the evolution of imaging modalities for the preoperative planning of microsurgical breast reconstruction | Cevik J, Seth I, Hunter-Smith DJ, Rozen WM | 2023 Aug 11 |
Current and future photography techniques in aesthetic surgery | Parsa S, Basagaoglu B, Mackley K, Aitson P, Kenkel J, Amirlak B | 2021 Nov 29 |
· Patient communication (n = 9)
· Preoperative surgical planning (n = 13)
· Preoperative education and preparation (n = 5)
Several articles provided insights across multiple subdomains, reflecting the interconnectedness of AI applications within the preoperative context of plastic surgery. Data from these articles was critically analyzed, highlighting current AI applications, benefits, limitations, and potential for further development.
Below, we further outline the three main domains in which AI can help operative preparedness in plastic surgery.
Patient communication and preoperative preparedness
Consultations play a vital role in establishing accurate diagnoses, formulating management plans, and creating trust between patients and their medical teams. While AI demonstrates considerable potential in this area, its current capabilities highlight both promises and limitations[11].
AI tools, such as the widely recognized Chat Generative Pre-trained Transformer (ChatGPT), have shown impressive knowledge in clinical scenarios. For instance, ChatGPT has performed comparably on knowledge to a first-year plastics trainee on in-service examinations, achieving a score in the 49th percentile[12]. With focused training in plastic surgery, AI could potentially perform at much higher levels.
Recent research further emphasizes AI’s strengths. One investigation into ChatGPT’s use in blepharoptosis consultations revealed that it provided more comprehensive information than experienced plastic surgeons[13]. Similarly, its responses to microsurgery-related queries were rated superior to those found on the website of the American Society of Reconstructive Microsurgery, according to feedback from both medical professionals and laypeople[14]. Furthermore, a study examining ChatGPT’s ability to obtain informed consent for plastic surgery showed it could provide shorter, more readable, and comparably reliable information regarding common plastic surgery procedures[15,16].
However, AI’s limitations remain evident. Tian et al. observed that while ChatGPT shows the potential in improving clinical workflows and patient education, its inclination to provide generalized responses highlights the importance of surgeon oversight, particularly for procedure-specific nuances[17]. This underscores the need for careful consideration of ethical challenges. Additionally, it is important to remember to see AI as a supportive tool, not as a replacement[6,15]. The prospect of AI fully replacing clinicians in consultations is currently difficult to envision. Such a transition would require rigorous testing, validation, and safety assessments before gaining widespread acceptance. Instead, AI’s supportive role in consultations appears more plausible and beneficial.
AI has demonstrated its ability to enhance clinical workflows. For example, it outperforms traditional predictors, such as the American Society of Anesthesiologists (ASA) score, in predicting postoperative morbidity and mortality, which can help patients make more informed decisions during the consent process[18]. Additionally, AI scribes provide the ability to draft consultation letters in a smooth manner. This can significantly reduce the plastic surgeon’s administrative burden and makes it possible to better inform the patient and his General Practitioner[19]. AI can efficiently scribe patient encounters, enabling clinicians to focus on patient-centered interactions without being distracted by screens, typing, and saving hours of clinician time and administrative workload[20]. This efficiency not only improves patient and clinician satisfaction but also streamlines outpatient services, allowing physicians to see more patients without delays caused by documentation.
Furthermore, AI systems can help patients better understand complex medical information. They translate complex medical terms into understandable language, giving patients a better understanding of predetermined treatments[15]. Moreover, diagnoses and treatment options can be personalized by linking patients’ personal information, as well as their medical history and patient wishes. As we all know, a well-informed patient has much higher adherence[21].
While AI has shown remarkable potential in clinical consultations, significant challenges and limitations persist. Its most likely role in the future is as a complementary tool, augmenting clinician capabilities rather than replacing them[17]. By enhancing prediction accuracy, documentation efficiency, and patient engagement, AI has the potential to revolutionize consultations while maintaining the centrality of human judgment and compassion in patient care.
AI in preoperative surgical planning
Surgical decision making
Reinforcement learning is a specialized form of machine learning (ML) that focuses on optimizing actions to achieve a specific goal. The model learns through rewards and punishments: successful actions are rewarded, while less effective actions are discouraged. This iterative learning allows reinforcement learning to develop a strategy that maximizes the probability of achieving a predefined goal.
In the medical world, specifically in surgery, reinforcement learning offers promising opportunities to improve decision making. For example, it can be used to make recommendations to surgeons at predetermined times based on dynamic analysis of the situation. This could range from recommending a surgical technique to determining the optimal time for a particular intervention.
A key advantage of reinforcement learning is its ability to process complex, multidimensional input data. This includes not only textual and visual information, such as medical reports and radiological images, but also temporal data, such as changes in vital signs or the progress of an operation in real time. By combining these different types of data, reinforcement learning can form a comprehensive picture of the situation and generate context-specific recommendations.
Through its flexibility and intelligence, it can not only help streamline surgical processes, but also contribute to more accurate and personalized care. Its use can lead to improved patient outcomes by supporting surgeons in making complex decisions under time pressure. Although this technology is still under development, it represents a promising step toward integrating AI into modern medicine[22,23].
Breast reconstruction
AI’s use in medical imaging dates to 1992, with computer systems detecting microcalcifications in mammography[24]. Today, AI systems often outperform experienced radiologists in interpreting mammograms, reducing false negatives and positives[25]. In 2017, the Food and Drug Administration (FDA) approved an AI-based radiology reporting algorithm, marking a milestone in clinical AI adoption. AI has since advanced across specialties like ophthalmology, cardiology, and stroke care, with augmented reality aiding real-time surgical planning. However, AI’s role in preoperative planning for free flaps remains underdeveloped, requiring further research and validation[26].
Among reconstructive options, the deep inferior epigastric artery perforator (DIEP) flap is the gold standard in reconstructing a breast. However, its success is often complicated by the variable vascular anatomy of the deep inferior epigastric artery (DIEA), including differences in location, size, and number of perforators. Preoperative imaging, particularly computed tomography angiography (CTA), is commonly used to map the vascular architecture, aiding in perforator selection and reducing operative times. Interpreting CTA scans is labor-intensive, requiring expertise and prone to variability due to the subjective nature of identifying DIEA branches[27]. To enhance surgical planning, techniques such as 3D reconstructions and virtual/augmented reality have been explored[28-30].
Studies by Mavioso et al. demonstrated that AI could dramatically reduce perforator analysis time. Using a semi-automated image processing algorithm, the study reduced analysis times from 2-3 h to just 30 min per scan, saving approximately 80 h across 40 patients[31]. Their study implemented a semi-automatic pathway to perforator detection.
Moreover, AI demonstrated comparable accuracy to manual methods in locating perforators. However, Mavioso et al. reported challenges in assessing smaller perforators (≤ 1.5 mm), where the algorithm introduced errors. The software excelled in evaluating larger perforators but showed a significant vertical location error, though with a small effect size[31]. These results suggest that while AI can optimize preoperative planning, further refinement is needed to enhance its reliability across all perforator types. Currently, at our center, we are working on fully automated AI preoperative perforator detection. Improving and developing such systems highlights AI’s potential in improving time efficiency and streamlining preoperative workflows[6].
Microsurgery
Besides the potential of AI to improve DIEP flap surgical planning by detecting the perforators and helping in flap design, there are also possibilities for monitoring the flap perfusion postoperatively. A study by
Aesthetic surgery
AI can also play an important role in the preoperative simulation of various procedures, creating three-dimensional simulations. A well-known example of 3D surface imaging technology is Vectra®, which allows surgeons to create a virtual representation of the patient on which to base their plans. Whereas these original companies are also increasingly incorporating AI algorithms in their simulations, we are seeing new programs that fully use generative AI to develop postoperative outcome images based on ML[33-35].
It is likely that in the future, we will increasingly see a combination of three-dimensional surface imaging technology with increasingly better AI algorithms. This makes it much easier for the patient to receive a realistic picture of the expected results of the surgery, which helps in getting a better idea of the patient’s goals. This not only contributes to a better match between what the patient expects and what is possible but may also be able to reduce misunderstandings and disappointments after surgery. It increases surgical precision, protects the surgeon from unforeseen complications and helps set realistic expectations, ultimately leading to better outcomes[36].
Head and neck surgery
AI is playing an increasing role in virtual surgical planning (VSP). Although research on the integration of AI in pattern recognition and model development is still in its infancy, the use of AI for mandibular reconstructions in daily clinical practice remains limited for now. This is because the final design still needs to be manually modified by surgeons and engineers. Despite these limitations, AI is already being successfully used in the preoperative segmentation of skull CTs[37].
Manual segmentation of CT images is, as mentioned above, a time-consuming task that relies heavily on the surgeon’s subjective interpretation. AI offers a solution here by enabling more objective and standardized reconstruction planning. For complex mandibular defects, such as those caused by tumors or congenital disorders, AI can help in analyzing the morphology of the jaw. Generative adversarial networks (GANs) can supplement this by generating authentic images of normal jaw structure[38].
Although AI offers impressive possibilities, surgeons’ anatomical expertise remains essential. Structures such as the inferior alveolar nerve canal, teeth, condyle head, and glenoid fossa must be carefully considered during planning. Moreover, metallic artefacts from, for example, crowns, implants, or orthodontic appliances can complicate image segmentation, placing an additional burden on surgical planning. AI can handle these challenges, but still benefits from refinements based on anatomical knowledge and accurate image analysis[39,40].
By using ML and deep learning (DL), AI can extract information from large data sets and continuously improve itself. These technologies enhance AI’s capabilities and increase its potential for application in VSP. Thus, AI not only supports segmentation, but can also contribute to clinical decision support (CDS). This is particularly valuable in radiological incidental findings, where AI can help with detection and interpretation.
AI combined with advanced imaging techniques such as diffusion-weighted MRI and radiomics can offer significant improvements in patient care. It is particularly useful for tumor segmentation and assessment of perineural invasion, which is crucial for R0 resections. Applications of DL for image segmentation are already being used successfully in other disciplines, such as cardiology, neurosurgery, and oncology[37].
Within maxillofacial surgery, current research focuses on AI models for the segmentation of mandibular and maxillary bone structures, the mandibular canal, condyle head, maxillary sinus, natural and treated teeth, and implants[41].
Although AI is making tremendous progress in VSP, technical improvements in 3D imaging and anatomical accuracy are necessary. However, the potential of AI to improve efficiency, objectivity, and patient care is undeniable and remains a focus for future research[8,42].
Preoperative education and preparation
Simulation was initially introduced in general surgery, particularly for laparoscopic and endoscopic procedures, and has since been embraced by various surgical specialties. It covers a wide range of models, including synthetic, animal, human, and virtual simulations[43,44]. The aim is to mimic the operating theatre environment, allowing students to practice in a safe, controlled environment. As a result, they develop both physical and cognitive skills through repetition and visualization of anatomical structures.
In plastic surgery, simulation is used to evaluate basic knowledge, such as operative anatomy, and to measure progress in surgical skills. Simulation provides a standardized approach to the variables in the operative experience and provides objective, quantitative evaluations of skills, in addition to qualitative assessments in the operating theatre. Thanks to its proven effectiveness and the increasing availability of validated models, simulation is increasingly used in training and fellowship programs[35,45].
In addition, several studies have shown that AI can significantly improve the quality and efficiency of plastic surgery training programs. For example, AI systems that convert text to images are used to generate clinical photographs, enriching medical and plastic surgery education. Moreover, AI-powered simulators provide trainees with a safe and controlled environment to practice surgical techniques, minimizing the risk of errors and complications during real procedures. This technology can further enhance training programs, from medical studies to residencies, and ensure that plastic surgeons are well prepared and skilled in the most advanced techniques[46,47].
Lastly, we believe that meaningful change and widespread adoption of robotics and AI in surgery can only be achieved through comprehensive training and early exposure during a surgeon’s formative years. Early engagement not only fosters familiarity but also inspires innovation and expands the potential applications of these technologies. Introducing robotics and AI into surgical training curricula at an early stage provides greater opportunities for research, development, and advancements in the field, laying a strong foundation for future progress[10,48].
DISCUSSION
To ensure that AI is effectively and responsibly applied in healthcare, it is essential that healthcare professionals, such as doctors and surgeons, take a leading role in identifying future healthcare priorities. Physicians must not only understand the technical aspects of AI, but also recognize the specific needs and constraints of the healthcare sector. They should work closely with computer scientists, data scientists, and engineers to develop AI algorithms that are both clinically relevant and understandable to healthcare professionals. This requires a joint effort combining both medical and engineering expertise to create AI solutions that are not only scientifically robust but also practically applicable in daily clinical practice[49].
This is exactly what we are doing in our department. Since 2023, we have started a close collaboration with our university’s engineering department and are exploring multiple ways in which AI and other technological innovations can enhance our efficiency, accuracy, and outcome of care that we provide to our patients.
Moreover, robust methods must be developed to collect, digitize, and process health data ethically and efficiently. It is crucial that the data are presented in a standardized and consistent manner so that they are suitable for use by AI systems. This involves pre-cleaning, validating, and standardizing the data so that AI algorithms can provide reliable and actionable insights. Ensuring data quality is critical to the success of AI in healthcare, as unreliable or inconsistent data can compromise the accuracy of AI models[6,17]. If these basic principles are adhered to, then a continuous circle of AI development can be aimed for [Figure 2].
In addition, comprehensive frameworks need to be developed to ensure the cost-effectiveness, safety, and security of AI applications. It is essential that the deployment of AI in healthcare is measured not only by technological advances, but also by the impact on patient safety and efficiency of healthcare delivery. AI systems must meet strict data privacy and security standards, as patient data often contain sensitive information. This requires implementing robust cybersecurity measures and complying with data protection laws and regulations, such as the General Data Protection Regulation (GDPR) in Europe. In addition, AI systems need to be carefully validated, with evidence of their safety and effectiveness. This evidence should be thorough and publicly available so that both healthcare professionals and patients can be confident that the technology is safe to use[50,51].
Another important aspect is that data shared with companies developing AI algorithms must always be kept secure and confidentiality must be ensured. Companies using health data to develop AI must adhere strictly to ethical standards. This means that they must treat patient data responsibly and ensure that the innovations derived from this data benefit the wider healthcare system and patients themselves, without putting commercial interests above patient care. It is essential that patients’ interests are protected, and that AI solutions do not lead to misuse of personal data or unwarranted access to sensitive information[52].
Using patient data to improve health outcomes through AI also brings with it the responsibility to adhere to the ethical standards that apply to doctors. The standards for automated systems should at least meet, or even exceed, those required of human healthcare providers. Automated systems that make decisions about medical treatments, diagnostic processes, or surgical procedures must meet the highest ethical and professional standards, as the consequences of errors in care can be serious. This requires continuous evaluation and updating of AI systems so that they remain consistently compliant with medical ethics and patient care requirements[52-54].
Integrating AI into the clinical practice of plastic surgeons and other medical specialties introduces several ethical and practical challenges[50,51,54]. AI systems that claim to objectively classify attractiveness, for example, raise significant ethical dilemmas. Since beauty is subjective and culturally dependent, there is a risk that AI models promote discrimination by unfairly generalizing about what is considered “attractive”. There is also a potential risk of bias in AI systems, whereby algorithms may develop a preference for certain ethnic groups, genders, or body shapes, leading to unequal treatment or even reinforcing existing social stereotypes. For example, models trained primarily on images of individuals from one ethnic group may produce suboptimal or discriminatory outcomes for others. This is called algorithmic bias and can be particularly problematic in cosmetic surgery, where perceptions of aesthetics play a key role. AI should, therefore, be carefully developed and monitored to ensure that it does not contribute to inequality or discrimination based on external features.
Moreover, the use of AI in aesthetic treatments raises questions about its impact on patients’ beauty standards and self-image. AI-driven decisions on surgery or cosmetic procedures may further increase the pressure on patients to meet certain beauty standards, which may have psychological consequences and can unintentionally reinforce narrow and culturally specific beauty standards, which may not reflect the diversity of human appearance. It is important that AI systems are not only based on technical efficiency but also take into account the broader social and psychological impact of aesthetic treatments[55,56].
Therefore, it is essential to have transparent collaborations between clinicians, AI developers, and ethics committees. This collaboration will help address the potential risks of AI in healthcare and ensure that technology is deployed in a way that benefits both patients and the healthcare system as a whole. It is important that AI is developed and implemented in an ethical way, considering both the technical possibilities and the societal implications for patients and healthcare providers. Only in this way can AI contribute to a just, inclusive, and responsible future for healthcare.
In the interest of fairness and reducing bias in AI systems used in plastic surgery, it would be advisable to prioritize inclusive data collection. This could entail utilizing diverse and representative datasets that encompass individuals of various ages, ethnicities, genders, and body types, as well as a spectrum of skin colors, facial structures, and cultural beauty standards. In addition, it would be advisable for AI systems to undergo regular audits and testing to identify any potential biases. These audits could be conducted using datasets designed to highlight inequalities. Implementing fairness metrics could help assess whether the AI delivers fair results for all demographic groups. In addition, developers may wish to consider documenting the sources of their training data and disclosing potential limitations. Collaboration with experts in cultural studies, anthropology, and medicine could be beneficial in identifying how societal biases might potentially affect AI systems. In addition, regulatory oversight is critical to ensure ethical standards for the development and deployment of AI in medical and aesthetic fields. Independent reviews could be considered to verify the fairness and accountability of these systems. Finally, human supervision is crucial. Surgeons and physicians must combine AI recommendations with their professional judgment, carefully evaluate results, and offer culturally sensitive advice to patients.
FUTURE PERSPECTIVES
The integration of AI with other emerging technologies such as augmented reality, virtual reality and robotics is of paramount importance. The future of AI in Plastic surgery has the potential to offer valuable opportunities, particularly in the areas of medical training, enhancing surgical precision, and personalizing patient care. AI has the potential to enhance preoperative planning through advanced imaging techniques and 3D modeling. These technologies have the potential to provide surgeons with a more detailed view of complex anatomical structures, which may, in turn, help them to make better, more informed decisions before surgery.
In surgical training, AI has the potential to play a significant role by offering AI-powered simulators and virtual reality, which can provide more realistic and safer practice experiences. These technologies allow them to practice various surgical techniques without substantial risk to patients, accelerating the learning curve and improving skills.
Additionally, AI can provide valuable support in postoperative care. By analyzing patient data, AI can predict the expected outcomes of surgery and help monitor the recovery process. This can help doctors intervene in a timely manner in case of any complications and thus optimize recovery. Furthermore, the integration of medical data and personal preferences through AI can facilitate the creation of customized treatment plans, enhancing the personalization of care.
Chatbots, such as ChatGPT, have the potential to play an increasing role in plastic surgery by providing patients with quick access to information about procedures, risks, and recovery. They have the potential to support informed consent processes and facilitate postoperative follow-up, contributing to enhanced communication and reduced waiting times. While it is important to recognize that chatbots are not a substitute for human interaction, they can support surgeons by reducing administrative burdens and enhancing patient care. They have the potential to enhance efficiency and personalize the patient experience.
DECLARATIONS
Authors’ contributions
Created the concept, wrote and produced the manuscript: Kapila AK
Guided all the steps of preparing the manuscript, revised the drafts critically for important intellectual content, and gave final approval for publication of the version: Kapila AK
Made substantial contributions to writing the work, and is shared first author: De Vleminck L
Made substantial contributions to analyzing and revising the work: Hamdi M
All authors read and approved the final manuscript.
Availability of data and materials
Not applicable.
Financial support and sponsorship
None.
Conflicts of interest
All authors declared that there are no conflicts of interest.
Ethical approval and consent to participate
Not applicable.
Consent for publication
Not applicable.
Copyright
© The Author(s) 2025.
REFERENCES
2. Wu L, Zhao X, Lu ZD, Yang Y, Ma L, Li P. Accuracy analysis of artificial intelligence-assisted three-dimensional preoperative planning in total hip replacement. Jt Dis Relat Surg. 2023;34:537-47.
3. Hameed BMZ, Shah M, Naik N, et al. The ascent of artificial intelligence in endourology: a systematic review over the last 2 decades. Curr Urol Rep. 2021;22:53.
4. Deol ES, Kavoussi NL. Artificial intelligence applications in kidney stone disease. Artificial intelligence in urology. Elsevier; 2025. pp. 169-91.
5. Shah N, Khalid U, Kavia R, Batura D. Current advances in the use of artificial intelligence in predicting and managing urological complications. Int Urol Nephrol. 2024;56:3427-35.
6. Kapila AK, Georgiou L, Hamdi M. Decoding the impact of AI on microsurgery: systematic review and classification of six subdomains for future development. Plast Reconstr Surg Glob Open. 2024;12:e6323.
7. Kiwan O, Al-Kalbani M, Rafie A, Hijazi Y. Artificial intelligence in plastic surgery, where do we stand? JPRAS Open. 2024;42:234-43.
8. Fortune-Ely M, Achanta M, Song MSH. The future of artificial intelligence in facial plastic surgery. JPRAS Open. 2024;39:89-92.
9. Knoedler L, Odenthal J, Prantl L, et al. Artificial intelligence-enabled simulation of gluteal augmentation: a helpful tool in preoperative outcome simulation? J Plast Reconstr Aesthet Surg. 2023;80:94-101.
10. Kapila AK, Hamdi M. Time to evolve plastic surgery education? Plast Reconstr Surg Glob Open. 2024;12:e5778.
11. Nogueira R, Eguchi M, Kasmirski J, et al. Machine learning, deep learning, artificial intelligence and aesthetic plastic surgery: a qualitative systematic review. Aesthetic Plast Surg. 2025;49:389-99.
12. Humar P, Asaad M, Bengur FB, Nguyen V. ChatGPT is equivalent to first-year plastic surgery residents: evaluation of ChatGPT on the plastic surgery in-service examination. Aesthet Surg J. 2023;43:NP1085-9.
13. Shiraishi M, Tanigawa K, Tomioka Y, et al. Blepharoptosis consultation with artificial intelligence: aesthetic surgery advice and counseling from chat generative pre-trained transformer (ChatGPT). Aesthetic Plast Surg. 2024;48:2057-63.
14. Berry CE, Fazilat AZ, Lavin C, et al. Both patients and plastic surgeons prefer artificial intelligence-generated microsurgical information. J Reconstr Microsurg. 2024;40:657-64.
15. Patel I, Om A, Cuzzone D, Garcia Nores G. Comparing ChatGPT vs surgeon-generated informed consent documentation for plastic surgery procedures. Aesthet Surg J Open Forum. 2024;6:ojae092.
16. Patil NS, Huang R, Mihalache A, et al. The ability of artificial intelligence chatbots chatgpt and google bard to accurately convey preoperative information for patients undergoing ophthalmic surgeries. Retina. 2024;44:950-3.
17. Tian WM, Sergesketter AR, Hollenbeck ST. The role of ChatGPT in microsurgery: assessing content quality and potential applications. J Reconstr Microsurg. 2024;40:e1-2.
18. Hassan AM, Rajesh A, Asaad M, et al. Artificial intelligence and machine learning in prediction of surgical complications: current state, applications, and implications. Am Surg. 2023;89:25-30.
19. Rotenstein L, Melnick ER, Iannaccone C, et al. Virtual scribes and physician time spent on electronic health records. JAMA Netw Open. 2024;7:e2413140.
20. van Buchem MM, Boosman H, Bauer MP, Kant IMJ, Cammel SA, Steyerberg EW. The digital scribe in clinical practice: a scoping review and research agenda. NPJ Digit Med. 2021;4:57.
21. Park KW, Diop M, Willens SH, Pepper JP. Artificial intelligence in facial plastics and reconstructive surgery. Otolaryngol Clin North Am. 2024;57:843-52.
23. Loftus TJ, Vlaar APJ, Hung AJ, et al. Executive summary of the artificial intelligence in surgery series. Surgery. 2022;171:1435-9.
24. Driver CN, Bowles BS, Bartholmai BJ, Greenberg-Worisek AJ. Artificial intelligence in radiology: a call for thoughtful application. Clin Transl Sci. 2020;13:216-8.
25. McKinney SM, Sieniek M, Godbole V, et al. International evaluation of an AI system for breast cancer screening. Nature. 2020;577:89-94.
26. Cevik J, Seth I, Rozen WM. Transforming breast reconstruction: the pioneering role of artificial intelligence in preoperative planning. Gland Surg. 2023;12:1271-5.
27. Kapila AK, Kempny T, Knoz M, Holoubek J, Lipovy B, Hamdi M. An algorithm in managing deep inferior epigastric vessel interruption in free flap breast reconstruction. Plast Reconstr Surg Glob Open. 2023;11:e4938.
28. Thimmappa ND. MRA for preoperative planning and postoperative management of perforator flap surgeries: a review. J Magn Reson Imaging. 2024;59:797-811.
29. Lim B, Cevik J, Seth I, et al. Evaluating artificial intelligence’s role in teaching the reporting and interpretation of computed tomographic angiography for preoperative planning of the deep inferior epigastric artery perforator flap. JPRAS Open. 2024;40:273-85.
30. Cevik J, Seth I, Hunter-Smith DJ, Rozen WM. A history of innovation: tracing the evolution of imaging modalities for the preoperative planning of microsurgical breast reconstruction. J Clin Med. 2023;12:5246.
31. Mavioso C, Araújo RJ, Oliveira HP, et al. Automatic detection of perforators for microsurgical reconstruction. Breast. 2020;50:19-24.
32. Kim J, Lee SM, Kim DE, et al. Development of an automated free flap monitoring system based on artificial intelligence. JAMA Netw Open. 2024;7:e2424299.
33. Chartier C, Watt A, Lin O, Chandawarkar A, Lee J, Hall-Findlay E. BreastGAN: artificial intelligence-enabled breast augmentation simulation. Aesthet Surg J Open Forum. 2022;4:ojab052.
34. Parsa S, Basagaoglu B, Mackley K, Aitson P, Kenkel J, Amirlak B. Current and future photography techniques in aesthetic surgery. Aesthet Surg J Open Forum. 2022;4:ojab050.
35. Eldaly AS, Avila FR, Torres-Guzman RA, et al. Simulation and artificial intelligence in rhinoplasty: a systematic review. Aesthetic Plast Surg. 2022;46:2368-77.
36. Şimşek İ, Şirolu C. Analysis of surgical outcome after upper eyelid surgery by computer vision algorithm using face and facial landmark detection. Graefes Arch Clin Exp Ophthalmol. 2021;259:3119-25.
37. Baecher H, Hoch CC, Knoedler S, et al. From bench to bedside - current clinical and translational challenges in fibula free flap reconstruction. Front Med. 2023;10:1246690.
38. Liang Y, Huan J, Li JD, Jiang C, Fang C, Liu Y. Use of artificial intelligence to recover mandibular morphology after disease. Sci Rep. 2020;10:16431.
39. Yang WF, Su YX. Artificial intelligence-enabled automatic segmentation of skull CT facilitates computer-assisted craniomaxillofacial surgery. Oral Oncol. 2021;118:105360.
40. Rokhshad R, Keyhan SO, Yousefi P. Artificial intelligence applications and ethical challenges in oral and maxillo-facial cosmetic surgery: a narrative review. Maxillofac Plast Reconstr Surg. 2023;45:14.
41. Fung E, Patel D, Tatum S. Artificial intelligence in maxillofacial and facial plastic and reconstructive surgery. Curr Opin Otolaryngol Head Neck Surg. 2024;32:257-62.
42. Fazilat AZ, Brenac C, Kawamoto-Duran D, et al. Evaluating the quality and readability of ChatGPT-generated patient-facing medical information in rhinology. Eur Arch Otorhinolaryngol. 2025;282:1911-20.
44. Sutherland LM, Middleton PF, Anthony A, et al. Surgical simulation: a systematic review. Ann Surg. 2006;243:291-300.
45. Agrawal N, Turner A, Grome L, et al. Use of simulation in plastic surgery training. Plast Reconstr Surg Glob Open. 2020;8:e2896.
46. Farid Y, Fernando Botero Gutierrez L, Ortiz S, et al. Artificial intelligence in plastic surgery: insights from plastic surgeons, education integration, ChatGPT’s survey predictions, and the path forward. Plast Reconstr Surg Glob Open. 2024;12:e5515.
47. Abi-Rafeh J, Bassiri-Tehrani B, Kazan R, et al. Preoperative patient guidance and education in aesthetic breast plastic surgery: a novel proposed application of artificial intelligence large language models. Aesthet Surg J Open Forum. 2024;6:ojae062.
48. Souza S, Bhethanabotla RM, Mohan S. Applications of artificial intelligence in facial plastic and reconstructive surgery: a systematic review. Curr Opin Otolaryngol Head Neck Surg. 2024;32:222-33.
49. Murphy DC, Saleh DB. The annals of the royal college of surgeons of england. 2020;102:577. Available from: https://scholar.google.com/scholar?cluster=6979955655115776717&hl=zh-CN&as_sdt=5,48&sciodt=0,48. [Last accessed on 24 Apr 2025].
50. Cobianchi L, Verde JM, Loftus TJ, et al. Artificial intelligence and surgery: ethical dilemmas and open issues. J Am Coll Surg. 2022;235:268-75.
51. Power D. Ethical considerations in the era of AI, automation, and surgical robots: there are plenty of lessons from the past. Discov Artif Intell. 2024;4:166.
52. Copeland-Halperin LR, Oppikofer C. Artificial intelligence, data protection, privacy, and doxxing. Aesthet Surg J. 2025;45:223-4.
53. Capelli G, Verdi D, Frigerio I, et al; Artificial Intelligence Surgery Editorial Board Study Group on Ethics. White paper. Ethics and trustworthiness of artificial intelligence in clinical surgery. 2023. Available from: https://iris.uniroma1.it/handle/11573/1685251. [Last accessed on 24 Apr 2025].
54. O’Sullivan S, Nevejans N, Allen C, et al. Legal, regulatory, and ethical frameworks for development of standards in artificial intelligence (AI) and autonomous robotic surgery. Int J Med Robot. 2019;15:e1968.
55. Kenig N, Monton Echeverria J, Muntaner Vives A. Human beauty according to artificial intelligence. Plast Reconstr Surg Glob Open. 2023;11:e5153.
56. Bernal IC, Andre J, Patel M, Newman MI. Beauty re-defined: a comparative analysis of artificial intelligence-generated ideals and traditional standards. Cureus. 2024;16:e71026. Available from: https://assets.cureus.com/uploads/original_article/pdf/248904/20241108. [Last accessed on 24 Apr 2025].
Cite This Article

How to Cite
Download Citation
Export Citation File:
Type of Import
Tips on Downloading Citation
Citation Manager File Format
Type of Import
Direct Import: When the Direct Import option is selected (the default state), a dialogue box will give you the option to Save or Open the downloaded citation data. Choosing Open will either launch your citation manager or give you a choice of applications with which to use the metadata. The Save option saves the file locally for later use.
Indirect Import: When the Indirect Import option is selected, the metadata is displayed and may be copied and pasted as needed.
About This Article
Special Issue
Copyright
Data & Comments
Data
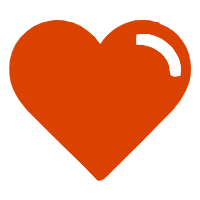
Comments
Comments must be written in English. Spam, offensive content, impersonation, and private information will not be permitted. If any comment is reported and identified as inappropriate content by OAE staff, the comment will be removed without notice. If you have any queries or need any help, please contact us at [email protected].