The use of generative artificial intelligence in surgical education: a narrative review
Abstract
The introduction of generative artificial intelligence (AI) has revolutionized healthcare and education. These AI systems, trained on vast datasets using advanced machine learning (ML) techniques and large language models (LLMs), can generate text, images, and videos, offering new avenues for enhancing surgical education. Their ability to produce interactive learning resources, procedural guidance, and feedback post-virtual simulations makes them valuable in educating surgical trainees. However, technical challenges such as data quality issues, inaccuracies, and uncertainties around model interpretability remain barriers to widespread adoption. This review explores the integration of generative AI into surgical training, assessing its potential to enhance learning and teaching methodologies. While generative AI has demonstrated promise for improving surgical education, its integration must be approached cautiously, ensuring AI input is balanced with traditional supervision and mentorship from experienced surgeons. Given that generative AI models are not yet suitable as standalone tools, a blended learning approach that integrates AI capabilities with conventional educational strategies should be adopted. The review also addresses limitations and challenges, emphasizing the need for more robust research on different AI models and their applications across various surgical subspecialties. The lack of standardized frameworks and tools to assess the quality of AI outputs in surgical education necessitates rigorous oversight to ensure accuracy and reliability in training settings. By evaluating the current state of generative AI in surgical education, this narrative review highlights the potential for future innovation and research, encouraging ongoing exploration of AI in enhancing surgical education and training.
Keywords
INTRODUCTION
Artificial intelligence (AI) has been transformative in the healthcare and education sectors by enhancing workflow efficiency through the automation of tasks[1]. One notable form of AI that has garnered considerable attention is generative AI, encompassing models that autonomously create novel content, including text, images, audio, and video[2]. Generative AI tools achieve this by leveraging machine learning (ML) techniques, particularly large language models (LLMs) and generative adversarial networks (GANs)[3-5]. LLMs are trained on vast quantities of textual data from an array of sources, enabling them to learn associations between lexical items and syntactic patterns to produce contextually specific responses[5-7]. Among the most widely recognized LLMs is ChatGPT by OpenAI (San Francisco, USA), which rapidly attracted over 100 million users within the first two months of its inception[8]. GANs, another subset of generative AI, specialize in producing realistic visual data. These models utilize two neural networks to create visuals, with one network generating images and the other evaluating their realism[9].
The ability to generate new content has piqued the interest of those within the surgical field for its potential applications in enhancing surgical education[10,11]. Traditionally, surgical training has involved a blend of theoretical instruction, observation of procedures, and supervised practice[12,13]. However, these conventional approaches encounter challenges such as limited access to diverse training scenarios. Additionally, to maximize the benefits of these modes of teaching, regular quality and structured feedback is required for trainees to refine their techniques and enhance their performance, which is often difficult due to time constraints and reduced opportunities for senior supervision[14,15]. LLMs demonstrate significant promise in overcoming these barriers by delivering real-time personalized feedback, owing to their ability to comprehend and generate text that mirrors natural human writing[16]. Furthermore, by integrating AI with surgical simulators, feedback in the form of text and data can be produced, allowing trainees to gain valuable insights into their performance outside the operating room[17]. Generative AI tools can also serve as educational aids for trainees. Through their chatbot-like interface, LLMs can be employed to answer surgical queries, create study materials including practice questions and case studies, and add interactivity by enabling dialogue and discussion. Meanwhile, GANs can potentially develop anatomical, pathological, and procedural images[18].
The intersection between AI technology and surgical education has become a topical area of research, leading to the publication of multiple studies on this topic in recent years[19-22]. However, limited reviews specifically focus on generative AI models’ applications in surgical education. As such, this narrative review aims to bridge this gap by providing an overview of existing applications, limitations, and future directions to foster continued development in this area.
METHODS
From the databases’ inception until July 2024, two authors independently conducted an extensive literature search encompassing PubMed (January 1996), Scopus (March 2004), World of Science (1997), and Cochrane Library (April 1996) databases. The search strategy employed included: (“generative artificial intelligence” OR “generative AI” OR “AI-generat*” OR “AI generat*” OR “ChatGPT” OR “Dall-E” OR “Sora” OR “text-to-image” OR “text to image” OR “text-to-video” OR “text to video” OR “artificial intelligence” OR “AI” OR “AI technolog*” OR “AI model*” OR “AI system*” OR “AI technique*” OR “machine intelligence” OR “computer vision” OR “computer vision system*” OR “computer reasoning” OR “neural network*” OR “neural network model*” OR “computer neural network*” OR “large language model*” OR “LLM” OR “natural language processing” OR “generative adversarial network*” OR “machine learning” OR “machine learning algorithm*” OR “deep learning” OR “deep learning model*”) AND (“surgical training” OR “surgical education” OR “surgical competence*” OR “surgical trainee*” OR “ surgical expertise” OR “surgical resident*” OR “surgical registrar*” OR “surgical fellow*” OR “ surgical learning” OR “surgical curriculum*” OR “surgical preparation” OR “surgical exam*” OR “surgical skill*” OR “surgical technique*”). Titles and abstracts were initially screened, followed by a full-text review to assess eligibility. Figure 1 shows the PRISMA flow diagram of selected studies.
Figure 1. PRISMA flow diagram of selected studies. PRISMA: Preferred Reporting Items for Systematic Reviews and Meta-Analyses.
Inclusion criteria:
1. Primary research published in peer-reviewed journals, incorporating both experimental studies such as randomized controlled trials (RCTs) and non-randomized trials, as well as observational studies including cohort and case-control studies.
2. Studies focusing on generative AI systems capable of creating novel content and outputs.
3. Studies with clear applications to surgical training, including improving educational methods, surgical techniques, or the development of surgical skills.
Exclusion criteria:
1. Studies not published in the English language.
2. Review articles, pre-prints, case reports, conference proceedings, conference abstracts, and letters or editorial opinions.
3. Studies on non-generative AI systems, e.g., predictive models, diagnostic tools, and traditional ML algorithms.
4. Studies that do not discuss generative AI in the context of applications to surgical training.
Due to the significant heterogeneity between the studies included in our review, a formal meta-analysis could not be performed. The variability in study designs, AI models employed, educational outcomes measured, and surgical subspecialties investigated contributed to this heterogeneity. However, we extracted and presented the data in a flowchart and tabular format to provide a comprehensive overview of the existing evidence. This approach allows for a more precise comparison of the outcomes analyzed in each study, highlighting the current literature’s strengths and limitations [Table 1]. The tabulated data of included studies [Table 2] also serve as a valuable resource for identifying trends and gaps in the research, which could guide future investigations in this rapidly evolving field.
Limitations and challenges of integrating generative AI into surgical education
Prompt engineering | Prompt engineering involves crafting specific and clear inputs to guide AI models effectively [27]. The accuracy of AI-generated surgical content, such as procedural outlines, depends on the precision of these prompts, often requiring significant experimentation to achieve the desired results. |
“Black box” issue | The limited understanding of AI models leads to potential mistrust within the system and difficulty for trainees to critically evaluate AI-generated recommendations, increasing the risk of medical errors[32]. |
AI “Hallucination” | LLMs can occasionally produce information that appears logical but is factually incorrect, raising concerns about the validity of AI-generated content and the potential propagation of medical misinformation[29]. |
Over-reliance on AI | Over-reliance on AI systems could lead to a decline in trainees’ clinical judgment, decision-making, and critical thinking skills, which are necessary for navigating complex surgical cases. |
Traditional mentorship models may diminish AI integration, potentially limiting the real-world experiences essential for comprehensive trainee skill development. | |
Ethical considerations | When AI-generated recommendations lead to adverse patient outcomes, the unclear responsibility between the AI system and the supervising surgeon can result in liability concerns, compromised patient trust, and ethical dilemmas over the surgeon’s decision-making autonomy. Advances in AI may also be misused for military or criminal purposes - for instance, an AI-driven surgical robot tool recreating a surgical technique might be correctly used by a surgeon or “incorrectly” used in the hands of a criminal, e.g., illegal organ acquisition. Further, AI programs/tools may create the possibility for exploitation of trained workforce - e.g., “pressured” trainees working for free or minimally paid workers - to generate AI inputs for such programs/tools. |
Summary of included studies
Title | Study design | Type of AI | Outcomes examined and conclusions |
Evaluating chatbot efficacy for answering frequently asked questions in plastic surgery: a ChatGPT case study focused on breast augmentation | Case study | Chatbot/NLP | Outcomes assessed: qualitative assessment of ChatGPT accuracy by qualified plastic and reconstructive surgeons. Conclusions: ChatGPT has the potential to address patient queries; however, further development is needed to address current limitations - namely dependability. Future research should assess chatbot performance across other plastic surgical procedures. |
Can AI answer my questions? Utilizing artificial intelligence in the perioperative assessment for abdominoplasty patients | Comparative evaluation study | Chatbot/NLP | Outcomes assessed: LLM response readability was assessed using the Flesch-Kincaid, flesch reading ease score, and Coleman-Liau index. The DISCERN score and a Likert scale were utilized to evaluate the quality of LLM responses by 2 plastic surgery residents. A consensus was reached by 5 consultant plastic surgeons. Conclusions: There were differences in readability between LLMs. LLMs require careful selection and weaknesses must be addressed to ensure optimal patient education. |
Generating informed consent documents Related to blepharoplasty using ChatGPT | Validation study design | Chatbot/NLP | Outcomes assessed: 4 board-certified surgeons and 4 non-medical staff compared AI-generated informed consent documents with existing informed consent documents for: accuracy, informativeness, and accessibility. Conclusions: ChatGPT cannot be used as a standalone patient resource, but it can assist in re-writing or updating medical documents. |
Online patient education in body contouring: A comparison between Google and ChatGPT | Validation study | Chatbot/NLP | Outcomes assessed: ChatGPT responses were assessed by four consultant plastic surgeons using a Global Quality Assessment Scale. Conclusions: ChatGPT demonstrated weakness in providing references but was deemed to provide good-quality responses that were useful to patients. AI was thought to optimize patient queries regarding body contouring. |
Artificial intelligence in postoperative care: Assessing large language models for patient recommendations in plastic surgery | Comparative evaluation study | Chatbot/NLP | Outcomes assessed: three independent authors assessed the accuracy, readability, understandability, and actionability of LLM responses. Conclusions: ChatGPT4 was the most effective LLM; however, Google Gemini provided more readable and actionable responses. All LLMs demonstrated potential as adjunctive tools in postoperative care but required additional refinement before use as standalone resources. |
Easing the burden on caregivers- applications of artificial intelligence for physicians and caregivers of children with cleft lip and palate | Validation study with parallel review | Chatbot/NLP | Outcomes assessed: The ability of ChatGPT to respond to common postoperative queries as assessed by two pediatric plastic surgeons. The ability of ChatGPT to develop a model for family-centric perioperative care was also evaluated. Conclusions: ChatGPT responses were found to be verbose with some notable incongruencies but generally accurate. AI could supplement caregiver-centered perioperative care through a modified model of care. |
Comparative analysis of artificial intelligence virtual assistant and large language models in post-operative care | Comparative analysis | AIVA, LLM, NLP | Outcomes assessed: 242 questions were presented “over the phone” to AIVA (AI virtual assistant) and responses were analyzed in terms of accuracy, knowledge gap, and overall appropriateness. Scores were then compared with ChatGPT4 and Google Bard (Google Gemini). Further assessments were made for readability. A qualified plastic surgeon provided definitive answers for each question and four healthcare professionals assessed AI responses using a three-point Likert scale. Conclusions: A specialized AIVA was found to be more efficacious than LLMs ChatGPT and Google Bard - demonstrating superior accuracy, a smaller knowledge gap, and higher appropriateness in response to LLMs. However, the study concluded that AI tools are supplementary at best and should only aid traditional patient education methods as opposed to replacing them. |
An artificial intelligence language model improves readability of burns first aid information | Quasi-experimental study design | Chatbot/NLP | Outcomes assessed: Readability assessment of AI re-written burns first aid information aimed at the level of an 11-year-old. Five different readability metrics were utilized. Conclusions: AI models can substantially improve the readability of existing information and may aid clinicians in updating and enhancing such resources. AI may be used to make health information more accessible for those without advanced health literacy. However, such models are not without biases and inaccuracies in content. |
Evaluation of the artificial intelligence chatbot on breast reconstruction and its efficacy in surgical research: a case study | Observational case study | Chatbot/NLP | Outcomes assessed: ChatGPT responses to six questions were assessed using Likert scales. A panel of two specialist plastic surgeons evaluated responses. Conclusions: ChatGPT can provide sufficiently accurate, jargon-free information to a layperson - but poses significant challenges to academic integrity and often provides superficial responses. LLMs should be trained on specialized data sets and outputs should be examined by experts. |
AI in hand surgery: assessing large language models in the classification and management of hand injuries | Case study | Chatbot/NLP | Outcomes assessed: Correctness of LLM classification of hand injuries as verified by a board-certified hand surgeon. Conclusion: both ChatGPT and Google Gemini show potential but are not suitable for current use. ChatGPT has a slight bias toward surgical treatment, whereas Google Gemini leans toward conservative management. LLMs have the potential to enhance diagnostic accuracy. |
Exploring the potential of ChatGPT-4 in responding to common questions about abdominoplasty: an AI-Based case study of a plastic surgery consultation | Comparative study | Chatbot/NLP | Outcomes assessed: ChatGPT4 responses to commonly asked abdominoplasty questions were analyzed by a qualified plastic surgeon for informational depth, response articulation, and competency. Conclusions: ChatGPT4 showed promise, but rigorous checks and continuous improvement regarding personalization of advice and correct referencing are needed before implementation into healthcare settings. |
Evaluating AI’s efficacy in enhancing patient education and answering FAQs in plastic surgery: a focused case Study on breast reconstruction | Case study | Chatbot/NLP | Outcomes assessed: ChatGPT4 responses were assessed for proficiency, depth, and precision. Response content was assessed for accuracy, depth, and user-friendliness by content experts (four experienced plastic surgeons). Conclusions: ChatGPT4 showed strengths in simplifying complex medical topics; it often provided generalized responses. While promising, ChatGPT4 cannot supplement traditional doctor-patient advice. Further development is needed to complement the personalized care provided during doctor-patient consultations. |
Integrating artificial intelligence in orthognathic surgery: A case study of ChatGPT’s role in enhancing physician-patient consultations for dentofacial deformities | Case study | Chatbot/NLP | Outcomes assessed: ChatGPT4 responses to nine sequential questions were assessed by a panel of four qualified plastic surgeons for adequacy, accuracy, and clarity. Conclusions: ChatGPT4 could provide detailed and systematic information with simple explanations. However, it lacks the esoteric qualities, individualized advice, and emotional intelligence needed to replace doctor-patient consultations. ChatGPT4 could serve as an assistant to physicians. |
Artificial intelligence knowledge of evidence-based recommendations in gender affirmation surgery and gender identity: is ChatGPT aware of WPATH recommendations? | Validation study | Chatbot/NLP | Outcomes assessed: ChatGPT4 was prompted with 31 frequently asked questions from Google and 95 questions adapted from WPATH guidelines. Two independent reviewers and 2 adjudicators categorized responses as agree, neutral, and disagree in accordance with WPATH guidelines. Conclusions: ChatGPT responses were largely accurate, comprehensive and successfully defined a range of concepts. However, ChatGPT provided some exclusionary and biased responses. Chatbots must provide fact-based and inclusive outputs. With proper regulation, ChatGPT has the potential to be a trusted education source for patients and providers. |
Using generative artificial intelligence tools in cosmetic surgery: A study on rhinoplasty, facelifts, and blepharoplasty procedures | Observational study | GANs | Outcomes assessed: GANs were used to generate realistic images of noses, faces, and eyelids. The resulting images were assessed by three qualified plastic surgeons for comprehensibility, accuracy to real life, and discernability. Conclusions: Notable limitations in GAN-generated images were noted. These included pseudorealistic and artistic renderings that diverge from real life. Images also demonstrated bias toward particular skin tones and lacked multiple angles of view. While AI is a potent tool, it must not overshadow or replace the intrinsic value of hands-on experience and direct patient interaction. |
The role of an open artificial intelligence platform in modern neurosurgical education: a preliminary study | Single arm study | ChatGPT/NLP | Outcomes assessed: Step 1 assessed whether Chat GPT could provide the correct answers to questions from the Turkish Neurosurgical Society Board Exam (TNSBE). Step 2 asked ChatGPT to generate questions from answers with associated explanations for each question. In Step 3, Chat GPT was asked to create case studies and assessed for consistency and accuracy in terms of medical history, examination findings, radiological results, diagnostic tests, and treatment processes. Lastly, Chat GPT was asked to comment on its own academic writing capabilities. Conclusions: Although ChatGPT has the potential to revolutionize learning for medical students, residents and neurosurgeons, it is not a reliable source. It lacks the ability to accurately cite sources and creates contradictions. Currently, it is best as a language tool to summarize, interpret, and simplify data - however, it may achieve better results with high-level prompts. |
GENERATING INTERACTIVE EDUCATIONAL MATERIALS AND LEARNING RESOURCES
With ongoing advancements in surgery and the increasing volume of knowledge to grasp, LLMs may be adopted to enhance learning efficiency. By combining rapid response times with advanced natural language capabilities, these tools can serve as dynamic resources capable of answering surgical questions and creating customized learning materials[23]. Brennan et al. investigated using ChatGPT to optimize otolaryngology education by guiding trainees through procedures[24]. Although the LLM provided procedural steps for a tonsillectomy, reviewers noted that the response was more suitable for junior trainees, as ChatGPT struggled with the more nuanced details of the procedure. Similarly, Mohapatra et al. observed that AI-generated surgical protocols missed crucial information, often leading to confusion among residents[25]. This issue was further highlighted in a study by Lebhar et al., revealing Plastics and Reconstructive Surgery residents were able to identify multiple inaccuracies in ChatGPT-generated procedural steps for a Fisher cleft repair, showing a preference for protocols written by experienced craniomaxillofacial surgeons[26]. These findings underscore the importance of integrating AI tools with expert oversight to ensure the accuracy and reliability of surgical education materials.
However, LLMs were more successful in generating interactive case studies to supplement surgical teaching and consolidate key concepts. ChatGPT was used to create a case study consisting of hypothetical patient data, clinical examination results, differential diagnoses, and a treatment plan, achieving a score of 100% from reviewers for its usefulness and accuracy[25]. However, a less specific prompt received a score of 43.33%. These results suggest that ChatGPT can generate relevant case scenarios for study, though only under conditions where a prompt was well-engineered[27]. Sevgi et al. determined that simulated case reports generated by ChatGPT were realistic in terms of their examination findings, investigations, and management[28]. Collectively, these studies emphasize that LLMs are better suited for trainees and medical students requiring a simplified but high-yield overview of a topic, given responses from ChatGPT are often concise and logical. However, the levels of detail and precision may be inadequate for more advanced trainees who may already have an extensive knowledge base.
In addition to outputting text, images from text prompts can also be produced via GANs. GANs hold the potential to produce images of anatomical structures and pathological features for learning, overcoming issues of privacy and confidentiality involved with using real patient images[10]. In an experimental study, Seth et al. investigated using AI models to artificially create images of skin ulcers, comparing the performance of DALL-E2 (Open AI, San Francisco USA), Midjourney (Midjourney, San Francisco USA), and Blue Willow (LimeWire, San Francisco USA) in performing this task[10]. Out of these three GANs, DALL-E2 was the most successful, avoiding issues such as overly stylized or completely irrelevant images. Although capable of mimicking realistic human skin, the images produced still lacked crucial details such as depth and the color of ulcers. Hence, in its current state, GAN technology cannot accurately create and depict medical images.
Integrating generative AI into surgical education holds great promise, yet significant challenges must be addressed before it can be widely adopted. From a technical perspective, generative AI models face a commonly recognized phenomenon known as “hallucination”, which refers to the models generating nonsensical or fictitious information, including false references to non-existent literature[29]. Multiple studies uncovered incorrect answers that were supported by seemingly logical justifications, which were later found to be false[25,30,31]. This phenomenon poses major risks to surgical education, especially in studies where junior trainees have difficulty identifying mistakes in AI-generated responses and may even prefer them for their clarity[26]. There are further concerns surrounding the inherent quality of the data that these models are trained on, which could lead to biases, inaccuracies and errors in the output[29]. When combined with the failure of AI models to verify information or cite sources consistently, there are significant risks in propagating misinformation. Finally, AI models face the “black box problem” where there is insufficient understanding surrounding the model’s inner workings, leading to a lack of transparency around the mechanisms in which responses are generated and, thus, mistrust toward the system[32]. Given these ongoing challenges, it is paramount that medical professionals thoroughly assess and fact check AI-generated resources before implementing them in educational settings.
USE OF LLMS IN SURGICAL EXAMINATIONS
LLM technology has potential applications in surgical curriculums and can be utilized by educators to develop exam questions, evaluate the clarity of questions, and mark examinations[24,28]. These roles can reduce the burden of work on educators, allowing more time to provide feedback and practical supervision to trainees. Sevgi et al. elucidated the ability of ChatGPT to design sample questions suitable for the level of a neurosurgery board exam, along with answers and relevant explanations[28]. While the LLM developed two appropriate sample questions, a third question was deemed unsuitable as it included two correct answers, underscoring the need to review AI-generated content before implementation in surgical examinations.
Several studies explored the accuracy of LLMs in answering questions. In one study, ChatGPT-4 achieved an 83.0% accuracy level on questions from a bariatric surgery textbook, with the highest success on definition and evaluation questions[33]. Another study assessing performance on the Korean Surgical Society and the Korean Academy of Medical Science (KAMS) board certification exam, found that GPT-4 achieved a consistently high level of accuracy across several subspecialties, with an overall rate of 76.4%[34]. Along with answers, ChatGPT provided justifications for each question, although some justifications were factually incorrect despite appearing logically sound. While LLMs are not yet suitable for marking surgical examinations in their current state, they can still be utilized to assess and improve the clarity of questions to optimize the writing of surgical exams.
Beyond their applications in developing and evaluating exam questions, LLMs hold potential as tools for exam preparation. An RCT by Wu et al. leveraged ChatGPT as an interactive exam preparation tool for hepatobiliary surgery[35]. Traditionally, interns received handouts, textbook readings, lectures, and clinical skills teaching. In the experimental group, these materials were supplemented with ChatGPT. Instead of passive reading, ChatGPT offered an interactive platform for developing questions, participating in simulated dialogues, summarizing literature reviews, and clarifying surgical steps for various hepatobiliary procedures. A subsequent theoretical exam and clinical skills assessment showed that interns in the experimental group performed significantly higher than those with traditional teaching, highlighting the advantages of interactive learning for surgical knowledge.
In contrast, a crossover study by Araji and Brooks provided a different perspective on the efficacy of ChatGPT for surgical exam preparation[31]. Participants completed two standardized assessments on general surgery topics, first using either a Google search or ChatGPT, and then switching to the other resource for the second assessment. Interestingly, no difference in scores was observed between the two resources. A post-assessment survey revealed that only 26% of the 19 medical students were likely to use ChatGPT in their surgical rotations, citing issues such as fabricated references, lack of images and diagrams, and inaccurate information. Conversely, a Google search allowed for the comparison of multiple resources and the screening of reliable sources. While preparation with ChatGPT yielded results comparable to Google, factors such as accuracy and the lack of graphics, such as concept maps, should be considered.
FEEDBACK GENERATION IN SIMULATED AND CLINICAL SCENARIOS
Acquiring feedback from experienced surgeons is not always possible, and as such, alternative methods for receiving feedback have been explored, with AI offering promising results. One such system is the Virtual Operative Assistant (VOA), an “AI tutoring system”[36]. The VOA adopts a supervised ML algorithm that classifies learner performance based on pre-defined metrics representative of surgical performance. The VOA then integrates with NeuroVR (NeuroVR, Netherlands), a tumor resection virtual simulator that provides a realistic visual and tactile experience while simultaneously recording user metrics such as tool positioning, forces applied, and acceleration when manipulating simulated instruments[36]. Its generative AI component lies in its ability to create detailed audiovisual feedback after evaluating user metrics, outlining the user’s performance as a percentage score, generating a graph comparing user performance to that of an expert, and outputting a written statement on actionable steps to improve. Feedback is further enhanced by the delivery of a 60-second video showcasing an expert demonstration[36].
In a randomized clinical trial by Fazlollahi et al., 70 medical students performed multiple simulated subpial resections on the NeuroVR[17]. Those who received feedback from the VOA in between sessions demonstrated significantly higher performance scores assessed by a deep learning algorithm, compared to those who received traditional instructor feedback or no feedback at all[17]. A subsequent retrospective cohort study by Fazlollahi et al. following up on the RCT further emphasized the utility of AI-generated feedback[37]. Participants in the VOA group displayed significant improvements from baseline across 32 metrics by the conclusion of their fifth simulated tumor resection compared to controls who received no feedback between attempts. The most pertinent metrics included a reduced rate of healthy tissue removal and improved instrument control, as evidenced by a reduced divergence of instruments that matched expert benchmarks[37]. However, these improvements also led to inadvertent effects of a significant decrease in dominant hand velocity and acceleration in addition to the rate of tumor removal, highlighting the need for a balanced approach that integrates AI-generated feedback with human guidance to minimize unwanted consequences.
A similar generative AI feedback system was explored by Ma et al. in the context of assessing needle handling and needle-driving skills while performing a simulated vesicourethral anastomosis on a da Vinci surgical robot[38]. Rather than obtaining live user metrics as with the VOA, a video of the simulated session was instead recorded and processed by an AI algorithm. Feedback was then delivered via an interface displaying selected video clips from the user side by side with an expert reference video, with a textual teaching point statement appearing below, e.g., “use a smooth, continuous motion”. Significant improvements in needle handling skills were observed from users compared to controls, although improvements in needle driving skills failed to reach statistical significance, possibly because needle driving inherently requires more practice[38]. Though still a prototype, this AI-feedback system could perhaps shorten learning curves and provide further opportunities to practice surgical skills outside the operating room. All generative AI applications in surgical education can be seen in Figure 2.
Figure 2. Flowchart of generative AI applications in surgical education settings. AI: Artificial intelligence.
The findings from Yang and Shulruf also corroborate those of Fazlollahi et al. and Ma et al.[17,37-39]. Medical interns were tasked with practicing suturing and ligature skills and assigned to the WKS-2RII system that utilizes an AI algorithm to analyze data collected from embedded sensors within a simulated silicon skin suturing pad and a webcam. Parameters such as the forces applied to the tissue, tension, distance between sutures, and wound dehiscence were assessed, allowing real-time live feedback in the form of visual data, images, and reference parameters to be generated. Students who undertook feedback from the WKS-2RII demonstrated higher performance at their surgical Objective Structured Clinical Examination (OSCE) than those led by conventional tutoring, with higher self-reported confidence in suturing and ligature skills[39].
Although a less sophisticated method with limited assessable metrics, feedback can be formulated by providing LLMs with postoperative details and outcomes of a procedure. A study by Jarry Trujillo et al. evaluated ChatGPT’s ability to identify errors and provide feedback using this approach[40]. Surgical residents assessed the usefulness and quality of ChatGPT’s responses in identifying and explaining errors in laparoscopic cholecystectomy scenarios. ChatGPT correctly identified the errors, with residents finding the AI responses useful 96.43% of the time and comparable in quality to those of experienced surgeons. However, it is essential to note that laparoscopic cholecystectomy is a highly standardized procedure with abundant literature available for the LLM to access. Furthermore, prompts were carefully crafted through multiple rounds of experimentation in a process known as “prompt engineering”[27]. Unlike systems such as the VOA, procedural details had to be manually translated into narrative text, a time-consuming process that may introduce bias[40]. Since the effectiveness of LLMs relies heavily on prompts, surgical trainees could benefit from guidelines on using tools such as ChatGPT effectively.
PERFORMANCE COMPARISON OF LLMS
In recent years, several LLMs have gained prominence, from OpenAI’s ChatGPT to Microsoft’s Bing. ChatGPT offers two available models: ChatGPT-3.5 and ChatGPT-4. While ChatGPT-3.5, released in 2022, is publicly accessible and available at no cost, ChatGPT-4, released the following year, is available at a monthly subscription cost and boasts improvements in functionality, memory, and performance[41]. On the KAMS board certification exam, ChatGPT-3.5 managed an accuracy of 46.8%, while ChatGPT-4 scored 76.4%[34]. The significant improvement in accuracy from ChatGPT-3.5 to ChatGPT-4 showcases the rapid advancement of generative AI and its potential for even greater performance in the future.
Interestingly, significant performance discrepancies were noted when comparing ChatGPT-4 with Google’s Bard (Google, California) and Microsoft’s Bing (Microsoft, Washington). In a study by Lee et al., ChatGPT-4 demonstrated improvements over its predecessor and other models, achieving an accuracy of 83% on textbook questions related to bariatric surgery, compared to Bard’s 76% and Bing’s 65%[33,34]. These results establish ChatGPT-4 as the most accurate and reliable LLM currently available for surgical education. Guthrie et al. evaluated the efficacy of their specialty-specific LLM, the Operating and Anaesthetic Reference Assistant (OARA, Texas USA), which was trained using a comprehensive dataset of peer-reviewed articles on surgery and anesthetics[42]. In their study, experts rated responses from OARA as 65.3% accurate, 14.7% inaccurate, and 20.0% precise partially across 150 prompts in the surgery and anesthesia domains. These findings demonstrate the capabilities of specialty-specific LLMs, which can be regularly updated with current medical research and guidelines to enhance their accuracy and relevance.
FUTURE DIRECTION
Future research could benefit from incorporating larger sample sizes to enhance the validity and generalisability of the findings. Exploring other AI models is also crucial for developing a more comprehensive understanding of their capabilities, particularly since different models may be better suited for specific surgical education applications based on their training data. While most studies have focused on ChatGPT, it is important to consider other advanced models such as Llama 3.1 (Meta, California, USA) and Claude 3.5 Sonnet (Anthropic, San Francisco, USA)[43]. Current research has also primarily focused on a limited number of surgical subspecialties, emphasizing the generation of text for learning resources and feedback. Broadening the integration of LLMs across a wider range of specialties could identify where these models are most effective and reveal other applications in surgical education.
Additionally, there is no standardized evaluation framework or tool specific for AI-generated outputs in surgical education. While organizations such as the National Institute of Standards and Technology are developing metrics and methodologies for assessing AI technologies, such as accuracy and robustness, individual studies often rely on custom criteria and tools to evaluate content quality[44]. Given the increasing role of AI in surgical education, establishing a standardized protocol for assessing the validity and accuracy of AI-generated outputs should be a priority for future research.
While the potential benefits of integrating generative AI into surgical education are promising, it is crucial to consider the associated risks, particularly the potential negative consequences of over-reliance on AI systems [44]. One significant concern is the possibility of diminished clinical judgment and decision-making skills among trainees. As generative AI becomes more advanced and accessible, there is a risk that surgical trainees may begin to rely excessively on AI-generated guidance and feedback, potentially leading to a decline in the development of critical thinking and problem-solving skills that are essential in the operating room[43]. The nuances of surgical decision-making often require an understanding of context, patient-specific factors, and the ability to adapt to unexpected challenges - skills that may not be fully nurtured if AI tools are overly dependent upon.
Furthermore, over-reliance on AI could result in the erosion of traditional mentorship and the apprenticeship model of surgical training, which has long been the cornerstone of surgical education. The interpersonal exchange between trainee and mentor, where experiential knowledge and tacit understanding are passed down, is irreplaceable by AI. There is also the concern that the use of AI might lead to the standardization of training experiences, where trainees are exposed to a narrower set of scenarios generated by AI, rather than the broad spectrum of real-world cases that can only be experienced through hands-on practice and observation. Additionally, the “black box” nature of many AI models raises transparency and trust issues[44]. Suppose trainees cannot fully understand the underlying mechanisms of AI decision-making. In that case, they may struggle to critically evaluate AI-generated recommendations, potentially leading to the acceptance of incorrect or suboptimal guidance. This lack of transparency could also foster a false sense of security, where the authority of AI is trusted implicitly without the necessary scrutiny, thereby increasing the risk of medical errors.
Finally, ethical and legal implications must be considered, particularly in the context of accountability. In cases where AI-generated recommendations lead to adverse outcomes, the delineation of responsibility between the AI system, the trainee, and the supervising surgeon becomes blurred. This ambiguity could complicate legal proceedings and raise concerns about the appropriate level of human oversight required when integrating AI into surgical practice. Given these risks, it is imperative that the integration of AI in surgical training is approached with caution. There must be a deliberate effort to balance AI-assisted learning and traditional training methods, ensuring that AI serves as an adjunct to, rather than a replacement for, the essential components of surgical education. Ongoing research and the development of comprehensive guidelines will be crucial in mitigating these risks and ensuring that AI enhances, rather than detracts from, the quality of surgical training.
CONCLUSION
Generative AI tools offer the potential to generate tailored interactive learning resources and exam preparation material, along with feedback post virtual simulations. However, with the technical challenges of AI models, further development of the technology may be required before more widespread adoption. In its current state, the integration of generative AI should be approached with caution and balanced with traditional supervision from experienced surgeons, utilizing a blended learning approach. Furthermore, their application should be focused on clearly defined and well-documented topics, guided by high-quality prompts to ensure accuracy and relevance. Nonetheless, ongoing research is still necessary to determine the feasibility of generative AI use across surgical subspecialties and explore other potential uses.
DECLARATIONS
Authors’ contributions
Methodology, literature search, data extraction, manuscript writing and editing: Yang E, Rao L, Dissanayake S
Manuscript writing and editing, and supervision: Seth I, Cuomo R, Rozen WM
All authors have made substantial contributions to the study and agree with the final version of the manuscript.
Availability of data and materials
All materials utilized in this review are accessible through PubMed, Scopus, Clarivate Web of Sciences, Scopus, and Cochrane Library.
Financial support and sponsorship
None.
Conflicts of interest
Rozen WM and Cuomo R are on the Editorial Board of the Plastic Aesthetic Research Journal, while the other authors declare that there are no conflicts of interest. Rozen WM and Cuomo R are guest editors for Artificial Intelligence in Plastic Surgery. Ishith Seth is the Guest Editor Assistant for Artificial Intelligence in Plastic Surgery.
Ethical approval and consent to participate
Not applicable.
Consent for publication
Not applicable.
Copyright
© The Author(s) 2024.
REFERENCES
1. Reddy S. Generative AI in healthcare: an implementation science informed translational path on application, integration and governance. Implement Sci 2024;19:27.
3. Memarian B, Doleck T. ChatGPT in education: methods, potentials, and limitations. Comput Hum Behav Artif Hum 2023;1:100022.
4. Barreto F, Moharkar L, Shirodkar M, Sarode V, Gonsalves S, Johns A. Generative artificial intelligence: opportunities and challenges of large language models. In: Balas VE, Semwal VB, Khandare A, editors. Intelligent Computing and Networking. Singapore: Springer Nature; 2023. pp. 545-53. Available from: https://scholar.google.com/scholar?q=Generative+artificial+intelligence:+opportunities+and+challenges+of+large+language+models&hl=zh-CN&as_sdt=0&as_vis=1&oi=scholart. [Last accessed on 14 Nov 2024].
5. De Angelis L, Baglivo F, Arzilli G, et al. ChatGPT and the rise of large language models: the new AI-driven infodemic threat in public health. Front Public Health 2023;11:1166120.
6. Shen Y, Heacock L, Elias J, et al. ChatGPT and other large language models are double-edged swords. Radiology 2023;307:e230163.
7. Raiaan MAK, Mukta MSH, Fatema K, et al. A review on large language models: architectures, applications, taxonomies, open issues and challenges. IEEE Access 2024;12:26839-74.
8. Meyer JG, Urbanowicz RJ, Martin PCN, et al. ChatGPT and large language models in academia: opportunities and challenges. BioData Min 2023;16:20.
9. Hong Y, Hwang U, Yoo J, Yoon S. How generative adversarial networks and their variants work: an overview. ACM Comput Surv 2020;52:1-43.
10. Seth I, Lim B, Cevik J, et al. Utilizing GPT-4 and generative artificial intelligence platforms for surgical education: an experimental study on skin ulcers. Eur J Plast Surg 2024;47:2162.
11. Xie Y, Seth I, Hunter-Smith DJ, Rozen WM, Seifman MA. Investigating the impact of innovative AI chatbot on post-pandemic medical education and clinical assistance: a comprehensive analysis. ANZ J Surg 2024;94:68-77.
12. Skjold-Ødegaard B, Søreide K. Competency-based surgical training and entrusted professional activities - perfect match or a procrustean bed? Ann Surg 2021;273:e173-5.
13. Kotsis SV, Chung KC. Application of the “see one, do one, teach one” concept in surgical training. Plast Reconstr Surg 2013;131:1194-201.
15. Scott MT, Rehman SU, NeMoyer RE, Patel NM. Optimizing surgical education through the implementation of a feedback curriculum. Am J Surg 2022;224:893-9.
16. Park SH. Use of generative artificial intelligence, including large language models such as ChatGPT, in scientific publications: policies of KJR and prominent authorities. Korean J Radiol 2023;24:715-8.
17. Fazlollahi AM, Bakhaidar M, Alsayegh A, et al. Effect of artificial intelligence tutoring vs expert instruction on learning simulated surgical skills among medical students: a randomized clinical trial. JAMA Netw Open 2022;5:e2149008.
18. Singh NK, Raza K. Medical image generation using generative adversarial networks: a review. In: Patgiri R, Biswas A, Roy P, editors. Health informatics: a computational perspective in healthcare. Singapore: Springer; 2021. pp. 77-96.
19. Atkinson CJ, Seth I, Xie Y, et al. Artificial intelligence language model performance for rapid intraoperative queries in plastic surgery: ChatGPT and the deep inferior epigastric perforator flap. J Clin Med 2024;13:900.
20. Seth I, Lim B, Joseph K, et al. Use of artificial intelligence in breast surgery: a narrative review. Gland Surg 2024;13:395-411.
21. Kirubarajan A, Young D, Khan S, Crasto N, Sobel M, Sussman D. Artificial intelligence and surgical education: a systematic scoping review of interventions. J Surg Educ 2022;79:500-15.
22. Guerrero DT, Asaad M, Rajesh A, Hassan A, Butler CE. Advancing surgical education: the use of artificial intelligence in surgical training. Am Surg 2023;89:49-54.
23. Locke S, Bashall A, Al-adely S, Moore J, Wilson A, Kitchen GB. Natural language processing in medicine: a review. Trends Anaesth Crit Care 2021;38:4-9.
24. Brennan L, Balakumar R, Bennett W. The role of ChatGPT in enhancing ENT surgical training - a trainees’ perspective. J Laryngol Otol 2024;138:480-6.
25. Mohapatra DP, Thiruvoth FM, Tripathy S, et al. Leveraging large language models (LLM) for the plastic surgery resident training: do they have a role? Indian J Plast Surg 2023;56:413-20.
26. Lebhar MS, Velazquez A, Goza S, Hoppe IC. Dr. ChatGPT: utilizing artificial intelligence in surgical education. Cleft Palate Craniofac J 2024;61:2067-73.
27. Meskó B. Prompt engineering as an important emerging skill for medical professionals: tutorial. J Med Internet Res 2023;25:e50638.
28. Sevgi UT, Erol G, Doğruel Y, Sönmez OF, Tubbs RS, Güngor A. The role of an open artificial intelligence platform in modern neurosurgical education: a preliminary study. Neurosurgical Review 2023;46:86.
29. Nah F, Zheng R, Cai J, Siau K, Chen L. Generative AI and ChatGPT: applications, challenges, and AI-human collaboration. J Inf Technol Case Appl Res 2023;25:277-304.
30. Shah A, Mavrommatis S, Wildenauer L, Bohn D, Vasconcellos A. Performance of ChatGPT on hand surgery board-style examination questions. J Orthop Exp Innov 2024;5.
31. Araji T, Brooks AD. Evaluating the role of ChatGPT as a study aid in medical education in surgery. J Surg Educ 2024;81:753-7.
32. Eschenbach WJ. Transparency and the black box problem: why we do not trust AI. Philos Technol 2021;34:1607-22.
33. Lee Y, Tessier L, Brar K, et al; ASMBS Artificial Intelligence and Digital Surgery Taskforce. Performance of artificial intelligence in bariatric surgery: comparative analysis of ChatGPT-4, Bing, and Bard in the American society for metabolic and bariatric surgery textbook of bariatric surgery questions. Surg Obes Relat Dis 2024;20:609-13.
34. Oh N, Choi GS, Lee WY. ChatGPT goes to the operating room: evaluating GPT-4 performance and its potential in surgical education and training in the era of large language models. Ann Surg Treat Res 2023;104:269-73.
35. Wu C, Chen L, Han M, Li Z, Yang N, Yu C. Application of ChatGPT-based blended medical teaching in clinical education of hepatobiliary surgery. Med Teach 2024;Online ahead of print.
36. Mirchi N, Bissonnette V, Yilmaz R, Ledwos N, Winkler-Schwartz A, Del Maestro RF. The virtual operative assistant: an explainable artificial intelligence tool for simulation-based training in surgery and medicine. PLoS One 2020;15:e0229596.
37. Fazlollahi AM, Yilmaz R, Winkler-Schwartz A, et al. AI in surgical curriculum design and unintended outcomes for technical competencies in simulation training. JAMA Netw Open 2023;6:e2334658.
38. Ma R, Kiyasseh D, Laca JA, et al. Artificial intelligence-based video feedback to improve novice performance on robotic suturing skills: a pilot study. J Endourol 2024;38:884-91.
39. Yang YY, Shulruf B. Expert-led and artificial intelligence (AI) system-assisted tutoring course increase confidence of Chinese medical interns on suturing and ligature skills: prospective pilot study. J Educ Eval Health Prof 2019;16:7.
40. Jarry Trujillo C, Vela Ulloa J, Escalona Vivas G, et al. Surgeons vs ChatGPT: assessment and feedback performance based on real surgical scenarios. J Surg Educ 2024;81:960-6.
41. Seth I, Lim B, Xie Y, et al. Comparing the efficacy of large language models ChatGPT, BARD, and Bing AI in providing information on rhinoplasty: an observational study. Aesthet Surg J Open Forum 2023;5:ojad084.
42. Guthrie E, Levy D, Del Carmen G. The Operating and Anesthetic Reference Assistant (OARA): a fine-tuned large language model for resident teaching. Am J Surg 2024;234:28-34.
43. Artificial Analysis. Comparison of models: quality, performance & price analysis. Available from: https://artificialanalysis.ai/models. [Last accessed on 14 Nov 2024].
44. Team AP. Artificial intelligence measurement and evaluation at the national institute of standards and technology. Available from: https://www.nist.gov/system/files/documents/2021/06/16/AIME_at_NIST-DRAFT-20210614.pdf. [Last accessed on 14 Nov 2024].
Cite This Article
How to Cite
Download Citation
Export Citation File:
Type of Import
Tips on Downloading Citation
Citation Manager File Format
Type of Import
Direct Import: When the Direct Import option is selected (the default state), a dialogue box will give you the option to Save or Open the downloaded citation data. Choosing Open will either launch your citation manager or give you a choice of applications with which to use the metadata. The Save option saves the file locally for later use.
Indirect Import: When the Indirect Import option is selected, the metadata is displayed and may be copied and pasted as needed.
About This Article
Special Issue
Copyright
Data & Comments
Data
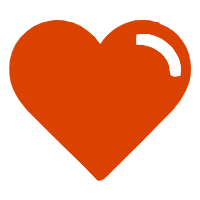
Comments
Comments must be written in English. Spam, offensive content, impersonation, and private information will not be permitted. If any comment is reported and identified as inappropriate content by OAE staff, the comment will be removed without notice. If you have any queries or need any help, please contact us at [email protected].