Special Issue
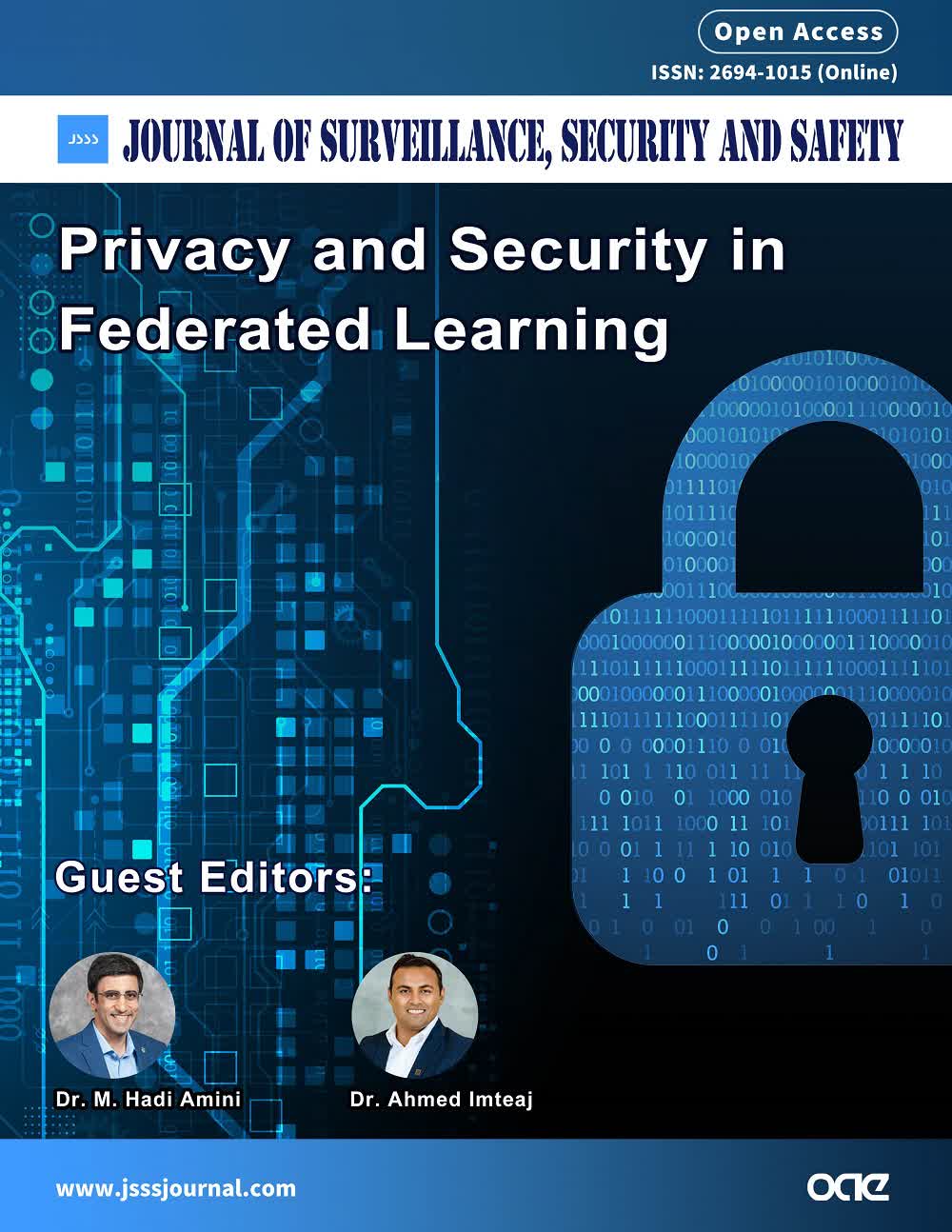
Topic: Privacy and Security in Federated Learning
A Special Issue of Journal of Surveillance, Security and Safety
ISSN 2694-1015 (Online)
Submission deadline: 28 Feb 2025
Guest Editors
Dr. M. Hadi Amini
Knight Foundation School of Computing and Information Sciences, Florida International University, Miami, FL, USA.
Special Issue Introduction
Recent smart devices such as smartphones, smartwatches, and smart glasses have access to an unprecedented amount of data, most of which is private and therefore often unutilized due to privacy concerns. Although some industries try to capture their users' data in different ways to provide privacy-preserving services, it is not sufficient for improving learning models while ensuring the privacy of users. Today's revolution in machine learning models is fueled by data, and better-quality data can help achieve superior performance. In addition, modern smart devices have become computationally efficient, which facilitates the concept of performing computation at the edge devices. This data is stored to secure local storage and only the local model parameters can be used for global model training. Due to the concern about revealing personally identifiable information, the sensitive data are not utilized to their full potential to build a robust artificial intelligence system. Hence, there is an emerging need to develop private and secure distributed learning algorithms. Rather than transferring the local data to a centralized storage system, we can perform computation at the user end in a distributed fashion using Federated Learning algorithms. Though federated learning has the potential to make distributed decision-making more effective, it faces several challenges in terms of security and privacy. This Special Issue calls for original work on innovative machine learning algorithms that are robust against privacy and security threads and can ensure the privacy of users.
Topics include but are not limited to:
● Architecture and privacy-preserving learning protocols;
● Federated learning and distributed privacy-preserving algorithms;
● Effectiveness and robustness of privacy-preserving techniques in the face of adversarial attacks;
● Investigating the use of privacy-enhancing methods, such as differential privacy, homomorphic encryption, secret sharing techniques, homomorphic encryption and secure multi-party computation, in the context of federated learning;
● Human-in-the-loop privacy-aware machine learning;
● Incentive mechanism and game theory;
● Privacy-aware knowledge-driven federated learning;
● Exploring the trade-offs between privacy, security, and performance in federated learning;
● Privacy Attack and defense models for FL environments;
● Safety and security assessment of distributed AI solutions;
● Relations of privacy with fairness, transparency, and adversarial robustness;
● Privacy and secure learning in computer vision and natural language processing tasks;
● Privacy and security mechanisms for Online social networks;
● Applications of secure/privacy-preserving machine learning in homeland security, critical infrastructure resilience and security.
Topics include but are not limited to:
● Architecture and privacy-preserving learning protocols;
● Federated learning and distributed privacy-preserving algorithms;
● Effectiveness and robustness of privacy-preserving techniques in the face of adversarial attacks;
● Investigating the use of privacy-enhancing methods, such as differential privacy, homomorphic encryption, secret sharing techniques, homomorphic encryption and secure multi-party computation, in the context of federated learning;
● Human-in-the-loop privacy-aware machine learning;
● Incentive mechanism and game theory;
● Privacy-aware knowledge-driven federated learning;
● Exploring the trade-offs between privacy, security, and performance in federated learning;
● Privacy Attack and defense models for FL environments;
● Safety and security assessment of distributed AI solutions;
● Relations of privacy with fairness, transparency, and adversarial robustness;
● Privacy and secure learning in computer vision and natural language processing tasks;
● Privacy and security mechanisms for Online social networks;
● Applications of secure/privacy-preserving machine learning in homeland security, critical infrastructure resilience and security.
Keywords
Federated learning, security and privacy of machine learning, distributed learning
Submission Deadline
28 Feb 2025
Submission Information
For Author Instructions, please refer to https://www.oaepublish.com/jsss/author_instructions
For Online Submission, please login at https://www.oaecenter.com/login?JournalId=jsss&IssueId=jsss2502281378
Submission Deadline: 28 Feb 2025
Contacts: Stella Qian, Assistant Editor, [email protected]
Published Articles
Coming soon