The polygenic risk score in the breast cancer treatment
Abstract
Breast cancer (BC) remains the most common cause of death in women worldwide, but advances in science have allowed earlier diagnosis and more comprehensive treatment. This review highlights the impact of extensive molecular genetic testing in assessing the risk of BC susceptibility, as well as possible responses to chemotherapy and radiotherapy. Studies in the literature show that several Single Nucleotide Polymorphisms (SNPs) of genes involved in molecular pathways may become predictors of the risk of developing BC. For example, SNPs in genes such as RAD51 and XRCC3, already known to be linked with high BC susceptibility, were also correlated with a different response to radiotherapy and related adverse effects. In addition, the SNP ESR1 PvuII (rs2234693), on the ESR1 gene, has been associated with a poor prognosis in advanced BC, but can be a good predictor of the therapeutic effect of hormonal treatment. Therefore, SNPs can be considered as possible new biomarkers for BC risk and prognosis. In this view, it is important to evaluate Polygenic Risk Score, an essential component for accurate BC risk prediction, which may potentially improve screening and prevention strategies. Bioinformatics tools are available to calculate polygenic risk by assessing the presence of SNPs and patients’ family and personal history. This represents an important step forward in the era of personalized medicine for BC.
Keywords
INTRODUCTION
Breast Cancer (BC) is one of the most common cancers affecting women worldwide, although it can also occur in men. BC can affect any woman in her postpubertal years, with the prevalence increasing with age, and it comprises 18% of all cancers in women. Between 2015 and 2020, 7.8 million women were diagnosed with BC globally[1]. Currently, it is estimated that there are more than one million new diagnoses each year[2]. According to the World Health Organization (WHO), early diagnosis and treatment are key to improving outcomes and survival rates, so most developed countries have scheduled regular mammography screening for the general population. Improved awareness of risk factors can contribute significantly to early diagnosis and better management of the disease. The pathogenesis of BC is the result of a complex interaction of several factors, including genetic, hormonal, and environmental factors. A small percentage of all women with BC (5%-10%) have a genetic predisposition that gives them an increased risk of developing the disease. This predisposition is due to the presence of pathogenic mutations in high- and moderate-risk BC susceptibility genes, such as BRCA1/BRCA2 and other genes such as PALB2, CDH1, PTEN, STK11, TP53 (higher relative risk of cancer or highly actionable), and ATM, BARD1, CHEK2, RAD51C, RAD51D, NF1 (moderate relative risk of cancer or potential impact for therapy/change in medical management)[3-5], or the presence of BC-associated common SNPs, each weighted according to its association with BC risk[6]. Non-genetic risk factors include environmental and lifestyle factors such as alcohol consumption, obesity, lack of physical activity, exposure to radiation, use of exogenous female hormones [e.g., menopausal hormone therapy (MHT) and hormonal contraceptives], and reproductive factors (early menarche and late menopause) [Figure 1][7,8].
In particular, hormonal factors play a crucial role in the development of BC; in fact, prolonged exposure to estrogen can promote the growth of some BC cells. BC typically begins in the cells lining the ducts (ductal carcinoma) or lobules (lobular carcinoma) of the breast. Therefore, BC is classified into invasive ductal carcinoma (IDC), ductal carcinoma in situ (DCIS), invasive lobular carcinoma (ILC), mucinous adenocarcinoma, and other rare types based on histological parameters[2,9]. Also important in tumor development is the tumor microenvironment, including surrounding cells, blood vessels, and immune components, which can influence tumor growth and progression. The immune system typically works to detect and eliminate cancerous cells and those with DNA damage. BC could arise from a malfunction of this essential immune defense and surveillance mechanism[10]. Understanding these factors is crucial for implementing preventive measures, such as regular screenings, maintaining a healthy lifestyle, and discussing risks with healthcare providers. Genome-wide Association Studies (GWAS) have identified multiple common BC susceptibility variants that individually confer a moderate risk, but their combined effect can be substantial and can be subsumed in a polygenic risk score (PRS)[11,12]. PRS is a tool used to quantify the genetic risk of an individual developing a particular disease, including various forms of cancer. Large cohorts of individuals are analyzed to identify common genetic variants that occur more frequently in people with a specific cancer than in those without it[13]. The PRS calculation represents the sum of the effects of m SNPs, based on the estimated SNP effect sizes
These scores include hundreds to thousands of SNPs, motivated by theory and data showing that many diseases are polygenic[14]. Individuals can be stratified into different risk categories (e.g., low, moderate, high) based on their PRS. This helps identify those at higher genetic risk who may benefit from increased surveillance or preventive measures. The usefulness of PRS in addition to existing risk calculation models such as the Gail Model[15-17], Breast and Ovarian Analysis of Disease Incidence and Carrier Estimation Algorithm (BOADICEA)[18], and the BC Surveillance Consortium (BCSC)[19] has been demonstrated in several studies. In particular, in a study by Li et al., it was shown that the addition of PRS to the BOADICEA model caused 16% of the women enrolled in the study to exceed the risk threshold, enabling them to access mammography screening with magnetic resonance imaging (MRI)[20]. The use of these risk models, which incorporate factors such as family history and environmental background, provides an opportunity to more accurately discriminate risk and introduce true population-based risk stratification[13]. PRS offers a promising approach to understanding and managing BC risk, allowing for personalized medicine, tailored prevention, early detection, and treatment strategies. This review, resulting from an in-depth study of the most recent and significant literature on PRS and its use in clinical practice, aims to highlight that the integration of bioinformatics tools into the diagnostic process would suggest a decisive role in increasingly personalized surveillance programs.
POLYGENIC RISK SCORE ERA IN CANCER RISK STRATIFICATION
As a complex and polygenic trait, the onset of cancer is determined by deep and intricate relationships between a multitude of genes and the environment.
According to the cancer genes census carried out by Catalogue of Somatic Mutations in Cancer (COSMIC), there are about 580 genes with well-documented effects on cancer if mutated[21].
When the “common variant-common disease” hypothesis was formulated, its proponents envisioned that GWAS could identify the profile of common variant sets for each type of cancer in order to profile the heritable component of the risk. Indeed, large-scale GWAS have highlighted hundreds of associations between common SNPs and the increased risk of developing cancer[22]. For each specific cancer type, a single genetic variant presents just a modest impact on the risk of developing the disease. However, the cumulative effect of these common variants on the genome is significant for many cancers and, more generally, for many complex and polygenic traits[23]. Genomics has revolutionized the field of epidemiology and public health through the implementation of genetic data in order to stratify populations based on risk and divide them into groups targeted for specific screening and prevention programs[22].
Currently, the number of SNPs strongly associated with BC risk has been further expanded, reaching 313 loci. The BOADICEA algorithm (Breast and Ovarian Analysis of Disease Incidence and Carrier Estimation Algorithm) calculates the cumulative risk of BC occurrence based on data from family history, mammographic imaging examinations, and several risk factors, both genetic and lifestyle-related. The genetic data on which BOADICEA is based include rare pathogenic variants in high- and moderate-risk genes for BC (BRCA1, BRCA2, PALB2, CHEK2 and ATM) and a PRS calculated from 313 BC-associated variants (PRS313)[6]. Risk stratification impacts cancer screening programs, such as test modality, screening interval, or eligibility criteria based on individual risk as determined by genetic and non-genetic patient features[24]. Recent international studies involving hundreds to thousands of women reveal that the implementation of PRS enables more precise risk stratification[6,25,26]. Every risk stratification tool has its own reliability and effectiveness, but may leave out some categories. Hence, the implementation of different stratification tools could be useful to improve the risk stratification process and implement appropriate surveillance and prevention programs. Based on the data from Ho et al.’s study, family history and genetic risk are effective risk stratification tools for women younger than 50 years of age[27]. This finding is of major importance since, in many countries, the eligibility age for mammography screening is 50 years. Genetic risk profiles make it possible to guide younger women to make informed decisions about whether to start screening at an earlier age[27]. The use of PRS could exert a key role in women with a higher risk of BC through increased preventive screening frequency. For example, PRS represents a good tool for women who are most likely to benefit from mammography screening [Figure 2][28].
These data suggest that implementation of the PRS with other risk stratification tools could prove extremely useful (i) to identify all categories of women at risk, which through the use of a single tool may not be identified; (ii) to build personalized surveillance programs on the patient based on genetic, family, and medical history data, making the patient aware of and participating in the prevention process; and (iii) to allow early risk stratification as the PRS data are potentially identifiable from birth.
BC RISK PREDICTION TOOLS IN COMBINATION WITH POLYGENIC RISK SCORE
BC risk assessment has traditionally relied on standardized screening recommendations, largely based on age. However, BRCA1 and BRCA2 mutations account for only 10% of all BC cases[29]. Therefore, there is a strong need to identify additional biomarkers to improve BC risk stratification and enhance screening programs.
Various clinical risk assessment models (such as BCRAT, IBIS, Gail, and BCSC) are currently employed to estimate a patient’s BC risk. The two most commonly utilized models are the Breast Cancer Risk Assessment Tool (BCRAT) and the International Breast Cancer Intervention Study (IBIS) risk prediction model[30].
The National Cancer Institute (NCI) BCRAT, developed by Gail et al., is based on a case-control study of 5,998 American women. Initially validated on a primarily Caucasian cohort, it was subsequently adjusted for women of diverse ethnic backgrounds (African American, Hispanic, Asian, and Pacific Islander)[31,32]. The latest version incorporates six clinical risk factors: current age, age at menarche, age at first live birth, number of first-degree relatives with BC, history of breast biopsies, and ethnicity. However, it is not applicable for women with BRCA1 or BRCA2 mutations.
In contrast, the IBIS model (also known as the Tyrer-Cuzick model) accounts for multigenerational family history and BRCA1/BRCA2 mutation data. Developed using data from daughters of BC patients, it assesses lifetime BC risk by considering family history, reproductive and hormonal factors, and individual characteristics. Unlike BCRAT, it evaluates both genetic and non-genetic risk factors such as age, menopause status, body mass index, history of hyperplasia, and hormone replacement therapy. Additionally, IBIS can be applied to women without known BRCA mutations or BC family history. A recent study found that IBIS provides better overall risk estimates than BCRAT, although both models perform similarly for women without BRCA mutations[33].
Advances in genetics have introduced more specific approaches to BC risk assessment, personalized to each patient’s unique genetic profile. Several risk prediction tools now integrate PRS, which calculates risk based on the cumulative effect of multiple common genetic variants, primarily SNPs. PRS models, which incorporate SNPs identified through GWAS, explain over 30% of BC inheritance.
GWAS have analyzed hundreds of thousands to millions of SNPs to identify those more prevalent in BC patients than in the general population. These studies calculate odds ratios (ORs) to measure the association between certain variants and BC risk. To date, GWAS have identified over 190 likely target genes and more than 170 genomic regions linked to BC susceptibility, with more than 300 SNPs associated with BC development.
PRS provides an aggregated risk estimate by combining the effects of numerous susceptibility variants identified in GWAS. While each variant confers only a small individual risk, their cumulative impact can substantially affect BC risk prediction. PRS is typically calculated by multiplying the published ORs for each risk SNP, enhancing BC risk stratification at the population level [Figure 3].
The Expected Allele Frequency (EAF) and OR for each SNP are used to calculate a risk score for each genotype, following the equation p2 + 2pq + q2 = 1, where p is the risk allele frequency and q is the non-risk allele frequency. Here, p2 represents the frequency of homozygous risk alleles, 2pq is the frequency of heterozygous risk alleles, and q2 is the frequency of homozygous non-risk alleles[1].
The discovery of new SNPs associated with BC in large-scale GWAS has expanded the number of SNPs integrated into PRS models, thus improving the accuracy and precision of BC risk stratification.
THE CORRELATION BETWEEN BREAST CANCER PRS AND THE TREATMENT RESPONSE
BC remains the leading cause of death among women. This type of cancer is highly heterogeneous, varying in molecular subtypes, epidemiological risk, and response to treatment. Approximately two-thirds of BC express the estrogen receptor (ER), encoded by the estrogen receptor alpha gene (ESR1) gene, which plays a crucial role in both BC tumorigenesis and the selection of targeted therapies[34]. The ER is essential for the normal development of the mammary gland, but it also plays a significant role in driving the growth of ER-positive BC[35]. The ER is mainly a nuclear protein that acts as a transcription factor, depending on the presence of a ligand. ER's function is driven by two main activation domains: one at the N-terminal, which operates without a ligand, and the other at the C-terminal, which requires a ligand to function. The ligand binding domain (LBD) is found at the C-terminal end, while the DNA-binding and hinge regions are centrally located within the protein. When estrogen binds to ER, it triggers activation and structural changes in the LBD, enabling the estrogen-ER complex to attach to specific DNA sequences known as estrogen response elements (ERE). This interaction also involves co-repressor and co-activator proteins, which together regulate the transcription of genes that respond to estrogen, playing critical roles in both normal physiological functions and the development and progression of cancer[36]. Clinically, ER is a recognized predictor of how effective endocrine therapies will be in treating BC. Tumors that are ER-positive rely on ER-related pathways for their survival and continuous growth. Amplification of the ESR1 can impact the function of the intricate ER signaling pathways within BC cells. In addition to that, variations in genes responsible for Tamoxifen metabolism, as well as polymorphisms within the ESR1 gene itself, can affect how patients respond to Tamoxifen treatment[35]. Specifically, the polymorphism in intron 1 of the ESR1 gene (rs2234693), known as ESR1 PvuII, has been linked to a higher risk of developing BC and reduced ER expression[34]. However, several recent studies are investigating the potential role of PvuII polymorphism as a predictive marker and a guide for clinicians in choosing the appropriate therapy. For example, in their retrospective study involving 104 patients with advanced-stage BC, Karsono et al. sequenced the ESR1 polymorphism and divided the population into wild-type (TT) patients and those with TC and CC genotypes[34]. Their study demonstrated that TT patients had a longer survival rate compared to those with TC and CC genotypes. However, they found a statistically significant difference in survival among the
Recent research has explored the impact of polymorphisms in the XRCC3 and RAD51 genes on BC treatment outcomes. RAD51 is crucial for the homologous recombination repair (HRR) of DNA double-strand breaks (DSBs), with the RAD51 gene located on chromosome 15q15.1 being a key player in this repair pathway and linked to BC development. Additionally, other RAD51-like genes, such as RAD51B, RAD51C, RAD51D, XRCC2, and XRCC3, are believed to function as central proteins in the HRR process[37]. Polymorphisms in the XRCC3 gene, such as XRCC3 Thr241Met, have been associated with varying responses to radiotherapy and chemotherapy. For example, specific genotypes of XRCC3 have been linked to increased sensitivity to anthracycline-based chemotherapy, which is commonly used in treating BC. These genetic variations may reduce the DNA repair capacity of cancer cells, leading to better treatment outcomes due to increased DNA damage in these cells[38]. Similarly, RAD51 polymorphisms have been shown to influence treatment responses, particularly in the context of radiotherapy. Variants like RAD51 (rs1801321) have been associated with altered expression levels of RAD51, which can affect how effectively BC cells repair DNA damage after treatment. High expression of RAD51 has also been linked to more aggressive tumor characteristics, such as increased metastasis and poorer responses to therapy. If validated in larger studies, genetic factors could potentially be used in the future to identify patients who are at a higher risk for acute or delayed adverse reactions to radiotherapy[39].
THE POTENTIAL FUTURE OF BREAST CANCER PRS WITHIN ROUTINE CLINICAL PRACTICE: BENEFITS AND CHALLENGES
Recent research has demonstrated the potential of PRS in estimating BC risk. However, PRS has not yet been fully integrated into clinical practice. To implement PRS in a healthcare setting, it is critical to evaluate its impact on patients' everyday lives. For instance, women identified as being at higher genetic risk of developing BC through PRS might use this information to make informed decisions about preventive options, including chemopreventive medications, prophylactic mastectomy, or changes in lifestyle. Introducing PRS testing earlier in life could be beneficial, although there are concerns about the risk of stigma or discrimination, and how parents might react to learning about the hereditary risks passed on to their children. Care must be taken to ensure that patients are not unduly alarmed by their genetic risk, nor subjected to unnecessary radiation through excessive mammography screening. Effective communication of PRS results and their implications is key to ensuring its appropriate use[1]. Despite the promising applications of PRS, several challenges remain.
First, robust data from GWAS and large-scale genetic epidemiological research are essential for constructing reliable PRS, particularly for rarer cancers[40], which suffer from a scarcity of epidemiological studies. Additionally, PRS models must be developed using diverse population cohorts to ensure broader applicability. Up to now, many large studies have predominantly focused on individuals of European descent. While PRS models derived from European ancestry cohorts have shown moderate applicability to Latinas and Asian populations, they have demonstrated weaker associations in women of African ancestry, likely due to differences in genetic backgrounds[23,25,41-43]. The most comprehensive GWAS on BC in African ancestry populations, conducted by Jia et al. identified a PRS that demonstrated improved predictive power, similar to those observed in other populations[44]. This suggests that PRS could serve as a valuable tool for identifying high-risk individuals, aiding in the earlier implementation of screening in such groups.
In terms of reproductive aging, the study by Coignet et al. examined the genetic factors contributing to reproductive aging in African American (AA) and European American (EA) women[45]. Their findings suggest a limited overlap in the genetic determinants of these phenotypes between the two groups. Specifically, no genome-wide significant variants were identified in AA women regarding age at menarche (AM) and age at natural menopause (ANM). This discrepancy may be attributed to both the limited availability of large-scale epidemiological cohorts and the distinct genetic architecture seen in African populations. The absence of genome-wide significant results in AA populations highlights the need for future studies with larger sample sizes, particularly for ANM. This study emphasizes the importance of cross-ethnic validation of GWAS findings and the inclusion of reproductive lifespan in analyses, which may be more relevant to breast cancer risk than focusing solely on AM or ANM.
To enhance the accuracy of PRS and address health disparities in breast cancer risk, particularly for underrepresented ethnic groups, future studies with larger sample sizes and more comprehensive genotyping platforms are crucial. Minority populations have been historically underrepresented in genetic studies of cancer susceptibility, which contributes to the current limitations in PRS accuracy for these groups.
On the economic front, Dixon et al. highlight the scarcity of research into the cost-effectiveness of integrating PRS into cancer screening programs[46]. More data are needed to assess the financial implications of using PRS, including the costs of genetic data acquisition, risk stratification, and its overall integration into routine clinical care[46]. The use of PRS is also unlikely to become a priority in low- and middle-income countries (LMICs), despite the decreasing cost of genetic testing and the acceptance of polygenic cancer risk assessment tools in high-income countries. Most LMICs struggle to maintain effective, sustainable cancer screening programs due to multiple barriers, such as socio-cultural challenges to implementation[47].
Clear guidelines are urgently needed to help healthcare professionals and the public understand PRS, how to interpret its results, and how to involve patients in discussions regarding their BC risk and appropriate preventive strategies.
DISCUSSION
PRS is emerging as a promising tool for genetic risk stratification in BC and represents a significant leap forward in the field of genomics and personalized medicine. The ability to integrate detailed genetic information derived from the analysis of multiple common genetic variants offers the potential to significantly improve risk prediction and inform clinical decisions and prevention strategies.
One of the main strengths of PRS lies in their ability to provide a more nuanced understanding of BC risk than traditional models that focus on family history or single high-penetrance mutations like those in the BRCA1/2 genes. For the vast majority of individuals who do not carry such mutations, PRS can still offer valuable risk information by capturing the polygenic nature of BC. Studies have demonstrated that individuals in the highest percentiles of PRS have a significantly elevated risk of BC compared to those with average or lower scores.
One of the most relevant aspects of PRS is their ability to correlate genetic risk with specific tumor characteristics. As highlighted in the study by Lopes Cardozo et al., PRS not only predicts the likelihood of developing BC but is also associated with specific tumor features, such as subtype and cancer aggressiveness[48]. This association can be particularly useful for personalizing treatment, enabling clinicians to adopt more targeted strategies based on each patient's genetic profile and contributing a small amount to an individual's overall risk of developing BC. However, the predictive accuracy of PRS, while promising, must be approached with caution. The inclusion of only common genetic markers may not be sufficient to capture the complexity of genetic predisposition, suggesting the need for more comprehensive models that also incorporate rare genetic factors and environmental influences.
Furthermore, as discussed in the study of Yiangou et al., variations in polygenic scores among different European populations underscore the importance of tailoring PRS based on genetic ancestry[49]. This study highlights how using a PRS that is not properly calibrated for specific populations can lead to inaccurate risk estimates. In particular, PRS developed on populations of European origin may not be directly transferable to non-European populations without a significant loss of precision. This aspect represents a crucial challenge for the global implementation of PRS in clinical practice, requiring further research to develop scores adapted to different ethnicities.
The integration of PRS into clinical practice has been explored in the article by Padrik et al.[50]. This study demonstrates the feasibility of using PRS to guide clinical decisions, highlighting how PRS can be employed to enhance screening and prevention strategies. The application of PRS can enable more accurate risk stratification, which, in turn, can reduce the need for invasive tests or unnecessary interventions for women with a lower genetic risk, while focusing resources on those with a higher risk. However, the implementation of these tests in clinical practice is not without challenges. Widespread adoption requires standardization of the tests, education of both physicians and patients on the benefits and limitations of PRS, and further validation studies to confirm the clinical utility of these tools.
In summary, while PRS represents a significant advance in understanding and managing BC risk, there are still several barriers to overcome before they can be adopted as a universal standard of care. Predictive accuracy, generalizability across different populations, and practical integration into clinical routines are key areas that require further research and refinement. Despite these challenges, the evolution of PRS could revolutionize the approach to BC prevention and treatment, leading to increasingly personalized and evidence-based medicine.
CONCLUSION
PRS offers significant potential for personalized BC risk assessment and management. However, challenges remain, particularly regarding generalizability across different populations, integration into clinical practice, and the need for comprehensive approaches that include environmental and other genetic factors. The current research indicates that while PRS can enhance current screening and prevention strategies, further development and validation are necessary to maximize its effectiveness.
DECLARATIONS
Authors’ contributions
Made substantial contributions to the conception and design of the study: Anaclerio F, Pilenzi L, Minelli M, Antonucci I, Stuppia L
Performed data acquisition and material support: Anaclerio F, Pilenzi L, Minelli M, Giansante R, Cicirelli M, Scorrano V, Antonucci I, Stuppia L
Availability of data and materials
Not applicable.
Financial support and sponsorship
None.
Conflicts of interest
All authors declared that there are no conflicts of interest.
Ethical approval and consent to participate
Not applicable.
Consent for publication
Not applicable.
Copyright
© The Author(s) 2024.
REFERENCES
1. Roberts E, Howell S, Evans DG. Polygenic risk scores and breast cancer risk prediction. Breast 2023;67:71-7.
2. Purrahman D, Mahmoudian-Sani MR, Saki N, Wojdasiewicz P, Kurkowska-Jastrzębska I, Poniatowski ŁA. Involvement of progranulin (PGRN) in the pathogenesis and prognosis of breast cancer. Cytokine 2022;151:155803.
3. Tung N, Ricker C, Messersmith H, et al. Selection of germline genetic testing panels in patients with cancer: ASCO guideline. J Clin Oncol 2024;42:2599-615.
4. Di Rado S, Giansante R, Cicirelli M, et al. Detection of germline mutations in a cohort of 250 relatives of mutation carriers in multigene panel: impact of pathogenic variants in other genes beyond BRCA1/2. Cancers 2023;15:5730.
5. Anaclerio F, Pilenzi L, Dell’Elice A, et al. Clinical usefulness of NGS multi-gene panel testing in hereditary cancer analysis. Front Genet 2023;14:1060504.
6. Mavaddat N, Michailidou K, Dennis J, et al. Polygenic risk scores for prediction of breast cancer and breast cancer subtypes. Am J Hum Genet 2019;104:21-34.
7. Britt KL, Cuzick J, Phillips KA. Key steps for effective breast cancer prevention. Nat Rev Cancer 2020;20:417-36.
8. Evans DGR, van Veen EM, Harkness EF, et al. Breast cancer risk stratification in women of screening age: incremental effects of adding mammographic density, polygenic risk, and a gene panel. Genet Med 2022;24:1485-94.
9. Sharma GN, Dave R, Sanadya J, Sharma P, Sharma KK. Various types and management of breast cancer: an overview. J Adv Pharm Technol Res 2010;1:109-26.
10. Akram M, Iqbal M, Daniyal M, Khan AU. Awareness and current knowledge of breast cancer. Biol Res 2017;50:33.
11. Michailidou K, Lindström S, Dennis J, et al. Association analysis identifies 65 new breast cancer risk loci. Nature 2017;551:92-4.
12. Milne RL, Kuchenbaecker KB, Michailidou K, et al. Identification of ten variants associated with risk of estrogen-receptor-negative breast cancer. Nat Genet 2017;49:1767-78.
13. Lambert SA, Abraham G, Inouye M. Towards clinical utility of polygenic risk scores. Hum Mol Genet 2019;28:R133-42.
14. Visscher PM, Wray NR, Zhang Q. et al. 10 years of GWAS discovery: biology, function, and translation. Am J Hum Genet 2017;101:5-22.
15. Allman R, Dite GS, Hopper JL, et al. SNPs and breast cancer risk prediction for African American and Hispanic women. Breast Cancer Res Treat 2015;154:583-9.
16. Dite GS, MacInnis RJ, Bickerstaffe A, et al. Breast cancer risk prediction using clinical models and 77 independent risk-associated snps for women aged under 50 years: Australian breast cancer family registry. Cancer Epidemiol Biomarkers Prev 2016;25:359-65.
17. Dite GS, Mahmoodi M, Bickerstaffe A, et al. Using SNP genotypes to improve the discrimination of a simple breast cancer risk prediction model. Breast Cancer Res Treat 2013;139:887-96.
18. Lee A, Mavaddat N, Wilcox AN, et al. BOADICEA: a comprehensive breast cancer risk prediction model incorporating genetic and nongenetic risk factors. Genet Med 2019;21:1708-18.
19. Shieh Y, Hu D, Ma L, et al. Breast cancer risk prediction using a clinical risk model and polygenic risk score. Breast Cancer Res Treat 2016;159:513-25.
20. Li H, Feng B, Miron A, et al. Breast cancer risk prediction using a polygenic risk score in the familial setting: a prospective study from the Breast Cancer Family Registry and kConFab. Genet Med 2017;19:30-5.
21. Laderian B, Zhou M, Fojo T. Distribution of cancer genes in human chromosomes. Semin Oncol 2020;47:409-13.
22. Sud A, Turnbull C, Houlston R. Will polygenic risk scores for cancer ever be clinically useful? NPJ Precis Oncol 2021;5:40.
23. Wang Y, Zhu M, Ma H, Shen H. Polygenic risk scores: the future of cancer risk prediction, screening, and precision prevention. Med Rev 2021;1:129-49.
24. Taylor LC, Law K, Hutchinson A, Dennison RA, Usher-Smith JA. Acceptability of risk stratification within population-based cancer screening from the perspective of healthcare professionals: a mixed methods systematic review and recommendations to support implementation. PLoS One 2023;18:e0279201.
25. Ho WK, Tan MM, Mavaddat N, et al. European polygenic risk score for prediction of breast cancer shows similar performance in Asian women. Nat Commun 2020;11:3833.
26. Mavaddat N, Pharoah PDP, Michailidou K, et al. Prediction of breast cancer risk based on profiling with common genetic variants. J Natl Cancer Inst 2015;107:djv036.
27. Ho PJ, Ho WK, Khng AJ, et al. Overlap of high-risk individuals predicted by family history, and genetic and non-genetic breast cancer risk prediction models: implications for risk stratification. BMC Med 2022;20:150.
28. Li J, Ugalde-Morales E, Wen WX, et al. Differential burden of rare and common variants on tumor characteristics, survival, and mode of detection in breast cancer. Cancer Res 2018;78:6329-38.
29. Sun YS, Zhao Z, Yang ZN, et al. Risk factors and preventions of breast cancer. Int J Biol Sci 2017;13:1387-97.
30. Jantzen R, Payette Y, de Malliard T, Labbé C, Noisel N, Broët P. Validation of breast cancer risk assessment tools on a French-Canadian population-based cohort. BMJ Open 2021;11:e045078.
31. Gail MH, Brinton LA, Byar DP, et al. Projecting individualized probabilities of developing breast cancer for white females who are being examined annually. J Natl Cancer Inst 1989;81:1879-86.
32. Farkas A, Vanderberg R, Merriam S, DiNardo D. Breast cancer chemoprevention: a practical guide for the primary care provider. J Womens Health 2020;29:46-56.
33. Tyrer J, Duffy SW, Cuzick J. A breast cancer prediction model incorporating familial and personal risk factors. Stat Med 2004;23:1111-30.
34. Karsono R, Haryono SJ, Karsono B, Harahap WA, Pratiwi Y, Aryandono T. ESR1 PvuII polymorphism: from risk factor to prognostic and predictive factor of the success of primary systemic therapy in advanced breast cancer. BMC Cancer 2021;21:1348.
35. Markiewicz A, Wełnicka-Jaśkiewicz M, Skokowski J, et al. Prognostic significance of ESR1 amplification and ESR1 PvuII, CYP2C19*2, UGT2B15*2 polymorphisms in breast cancer patients. PLoS One 2013;8:e72219.
36. Reinert T, Saad ED, Barrios CH, Bines J. Clinical implications of ESR1 mutations in hormone receptor-positive advanced breast cancer. Front Oncol 2017;7:26.
37. Yu J, Wang CG. Relationship between polymorphisms in homologous recombination repair genes RAD51 G172T, XRCC2 & XRCC3 and risk of breast cancer: a meta-analysis. Front Oncol 2023;13:1047336.
38. Castro E, Olmos D, Garcia A, Cruz JJ, González-Sarmiento R. Role of XRCC3, XRCC1 and XPD single-nucleotide polymorphisms in survival outcomes following adjuvant chemotherapy in early stage breast cancer patients. Clin Transl Oncol 2014;16:158-65.
39. Goričar K, Dugar F, Dolžan V, Marinko T. NBN, RAD51 and XRCC3 polymorphisms as potential predictive biomarkers of adjuvant radiotherapy toxicity in early HER2-positive breast cancer. Cancers 2022;14:4365.
40. Klein RJ, Gümüş ZH. Are polygenic risk scores ready for the cancer clinic? - a perspective. Transl Lung Cancer Res 2022;11:910-9.
41. Junior HLR, Novaes LAC, Datorre JG, Moreno DA, Reis RM. Role of polygenic risk score in cancer precision medicine of non-European populations: a systematic review. Curr Oncol 2022;29:5517-30.
42. Evans DG, van Veen EM, Byers H, et al. The importance of ethnicity: are breast cancer polygenic risk scores ready for women who are not of white European origin? Int J Cancer 2022;150:73-9.
43. Liu C, Zeinomar N, Chung WK, et al. Generalizability of polygenic risk scores for breast cancer among women with European, African, and Latinx Ancestry. JAMA Netw Open 2021;4:e2119084.
44. Jia G, Ping J, Guo X, et al. Genome-wide association analyses of breast cancer in women of African ancestry identify new susceptibility loci and improve risk prediction. Nat Genet 2024;56:819-26.
45. Coignet MV, Zirpoli GR, Roberts MR, et al. Genetic variations, reproductive aging, and breast cancer risk in African American and European American women: the women’s circle of health study. PLoS One 2017;12:e0187205.
46. Dixon P, Keeney E, Taylor JC, Wordsworth S, Martin RM. Can polygenic risk scores contribute to cost-effective cancer screening? A systematic review. Genet Med 2022;24:1604-17.
47. Dannhauser FC, Taylor LC, Tung JSL, Usher-Smith JA. The acceptability and clinical impact of using polygenic scores for risk-estimation of common cancers in primary care: a systematic review. J Commun Genet 2024;15:217-34.
48. Lopes Cardozo JMN, Andrulis IL, Bojesen SE, et al. Associations of a breast cancer polygenic risk score with tumor characteristics and survival. J Clin Oncol 2023;41:1849-63.
49. Yiangou K, Mavaddat N, Dennis J, et al. Differences in polygenic score distributions in European ancestry populations: implications for breast cancer risk prediction. medRxiv 2024.
Cite This Article

How to Cite
Anaclerio, F.; Pilenzi, L.; Minelli, M.; Giansante, R.; Cicirelli, M.; Scorrano, V.; Antonucci, I.; Stuppia, L. The polygenic risk score in the breast cancer treatment. J. Transl. Genet. Genom. 2024, 8, 328-39. http://dx.doi.org/10.20517/jtgg.2024.60
Download Citation
Export Citation File:
Type of Import
Tips on Downloading Citation
Citation Manager File Format
Type of Import
Direct Import: When the Direct Import option is selected (the default state), a dialogue box will give you the option to Save or Open the downloaded citation data. Choosing Open will either launch your citation manager or give you a choice of applications with which to use the metadata. The Save option saves the file locally for later use.
Indirect Import: When the Indirect Import option is selected, the metadata is displayed and may be copied and pasted as needed.
About This Article
Special Issue
Copyright
Data & Comments
Data
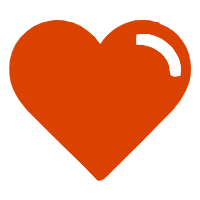
Comments
Comments must be written in English. Spam, offensive content, impersonation, and private information will not be permitted. If any comment is reported and identified as inappropriate content by OAE staff, the comment will be removed without notice. If you have any queries or need any help, please contact us at support@oaepublish.com.