Criterion validity of the genetic addiction risk severity (GARS) as a marker of reward deficiency in polydrug addiction: a multi-center study
Abstract
Aim: This study evaluated the Genetic Addiction Risk Severity (GARS) panel, which assesses genetic predisposition to addictive disorders by examining eleven polymorphisms in ten genes associated with dopaminergic reward system functioning.
Methods: The GARS registered mark instead panel includes six single-nucleotide polymorphisms [DRD1, DRD2, DRD3, DRD4, OPRM1, and catechol-O-methyltransferase (COMT)], four simple sequence repeats (5HTT, DAT1, DRD4, and MAOA), and one dinucleotide repeat (GABRA3). Criterion validity was tested in 393 polydrug abusers by correlating GARS scores with Addiction Severity Index-Multimedia Version (ASI-MV) alcohol and drug severity scores.
Results: We identified a significant correlation between GARS and the ASI-MV alcohol severity score. While individuals with elevated drug severity also exhibited increased GARS, the relationship did not follow a strictly linear pattern. Variations in multiple genes involved in dopaminergic signaling contributed to risk in an additive manner, with age serving as a significant covariate. A greater number (≥ 7) of reward gene polymorphisms associated with moderate reductions in dopamine signaling demonstrated a significant association with higher
Conclusion: Our findings align with previous research implicating dopaminergic pathways in the progression of alcoholism and substance abuse. Additionally, they build upon prior work by identifying a potential pre-existing polygenic risk factor, as defined by the GARS panel, that may be influenced by age-related physiological changes and environmental factors. Further research is warranted to explore associated endophenotypes, with particular emphasis on the role of Reward Deficiency Syndrome linked to dysfunction within the dopaminergic reward system.
Keywords
INTRODUCTION
The United States (US) is currently facing a significant public health crisis due to addiction and overdoses. According to the Centers for Disease Control and Prevention (CDC) in 2022, the US experienced a staggering 107,941 drug overdose deaths[1]. This represents a dramatic increase in the rate of drug overdose deaths, which has nearly quadrupled since 2002[1]. Additionally, approximately 70.3 million people over the age of 12 were found to have used illicit drugs in 2022, according to the Substance Abuse and Mental Health Services Administration (SAMHSA)[2]. The most commonly abused illicit drug was marijuana
While illicit drug use garners significant attention, the consumption of non-illicit substances, such as alcohol, tobacco, or vaping, poses equally pressing public health concerns. SAMHSA reported that
According to data from the National Institute on Drug Abuse (NIDA)[3], the annual cost of substance abuse in the US-including illicit drugs, alcohol, and tobacco exceeds $740 billion and continues to increase. The ongoing rise in addiction to chemical substances[4,5] underscores the necessity for innovative neurobiological insights to enhance prevention, diagnosis, and treatment strategies[6]. Studies in animals[7] and neuroimaging human research[8,9] attribute a pivotal role to the mesolimbic dopaminergic circuits subserving reward and motivation[10,11] at various stages of alcohol/drug addiction ranging from the acquisition of
Even though it is generally accepted that RDS is linked to mesolimbic circuitry, the debate about the roles of specific candidate genes is still ongoing. One extant panel[57] is comprised of comprehensive haplotype information for candidate genes in alcoholism, other addictions, and mood and anxiety disorders; it is comprised of 130 genes that were tagged and genotyped in 51 reference populations and 7 case/control populations using Illumina Golden Gate single-nucleotide polymorphism (SNP) genotyping technology. A comprehensive analysis[58] that synthesized data from 2,343 peer-reviewed studies, utilizing methodologies such as single-gene strategies, microarray analyses, proteomics, and genetic approaches to examine associations between genes or chromosomal regions and addiction, identified 1,500 human genes implicated in addictive behaviors. The resultant “Knowledgebase for Addiction Related Genes” (http://karg.cbi.pku.edu.cn) is the first molecular database for addiction-related genes with extensive annotations and a Web interface. The same authors also performed a meta-analysis of 396 genes supported by two or more independent items of evidence to define a putative common molecular network for addiction[58]. Nonetheless, it remains unclear what specific genes out of these 396 candidates subserve RDS and related neuropsychopathology.
As with complexities inherent in defining a single subtype of RDS, such as alcoholism or PTSD[17,47,59-61], the exigencies of an accurate control sample recruitment that is representative of the population of interest could confound genetic study results. According to the Epidemiological Catchment Area survey, close to one-third of the general population meets the criteria for common psychiatric disorders at some point in their lives. RDS is a “polygenic disorder” that involves multiple genes with various polymorphisms[62]. Expression of RDS requires reaching a threshold number of these polygenes and associated variants. Consequently, individuals may carry some of these polymorphic genes but do not exhibit RDS behaviors because the threshold has not been reached.
To that end, we balanced the recruiting efforts with pragmatics by removing confounding cases from the control group and by using stratified (weighting) samples to design the Genetic Addiction Risk Score (GARS) test, capturing distinct aspects of dopaminergic function that predicts liability for addiction and RDS[63,64]. Reward candidate genes were selected based on a thorough literature review to yield the rationale utilized for each listed risk allele. The unifying concept guiding the selection of specific alleles included in the panel was based on their significant contributions to diminished dopaminergic function, including genes encoding dopamine receptors (DRD1, DRD2, DRD3, DRD4), the dopamine transporter (DAT1), the serotonin transporter, catechol-O-methyltransferase (COMT), monoamine oxidase (MAO), gamma-aminobutyric acid (GABA) receptors, the µ-opioid receptor (OPRM1), and cytochrome P450. In addition, specific single nucleotide polymorphisms (SNPs) and point mutations that influence dopamine release in the brain's reward centers were incorporated. These genetic variants were selected to represent a hypodopaminergic phenotype, a choice substantiated by thousands of association studies that have provided compelling evidence linking these risk alleles to various addictive behaviors[65].
The ten genes (encompassing eleven polymorphisms) examined in this study encode proteins that are abundantly present in both cortical and subcortical neural structures. Dysregulation of these genes in the prefrontal cortex and limbic system may result in RDS[66-67]. A prior study[68] proposed a five dopamine-related gene panel including the DRD2/ANKKI, DRD3, and DAT to predict depression. Similarly, the Convergent Functional Genomics project[69] identified eleven candidate genes separating alcoholics from non-alcoholics. Even a single gene such as synuclein alpha (SNCA) regulating dopamine signaling showed a strong association with severe alcoholism (P = 0.0001). While it might be appealing to adopt such a parsimonious approach, it would be unreasonable to limit the testing to SNCA alone due to concerns regarding specificity and sensitivity[68]. Therefore, we have chosen to avoid an overly reductionistic approach to GARS by utilizing a small number of genes, e.g., DRD2/ANKKI, DRD1, and DAT1.
Although GARS’ construct validity can be ascertained via existing theoretical and empirical considerations[70-72], it is also important to juxtapose GARS against validated benchmarks, i.e., to assess its criterion validity.
The Addiction Severity Index (ASI) exemplifies a well-established tool for collecting comprehensive data, providing detailed information on the quantity, frequency, recency, and duration of alcohol and drug use[73]. This commonly utilized semi-structured interview also elicits information about life areas that are affected by alcohol/drug addiction. As both a psychodiagnostic and psychometric tool, ASI assesses addiction as a continuous measure of symptom severity c.f., categorical (present or absent) variables enabling the performance of more powerful correlational analyses.
The primary hypothesis pertained to an association between GARS and the ASI alcohol/drug scores. An a priori emphasis was given to the assumption that alcoholism, as well as addiction to other addictive substances, develops via negative reinforcement, i.e., alcohol/drugs are used to ameliorate the discomfort associated with the RDS[74]. However, an alternative explanation may be surmised in the form of the positive reinforcement idea - explicitly, that RDS, manifested via depressive symptomatology and related negative affective states, enhances alcohol/drug use by amplifying their rewarding and reinforcing properties[75]. This concept is reinforced by studies demonstrating a positive correlation between drug-induced euphoria and depressive symptoms, as reported by other research groups[76,77] and confirmed in our own investigations[78]. Furthermore, alcohol consumption may paradoxically offer a form of harm reduction in individuals with RDS by enhancing the sensitivity of previously under-responsive reward circuitry. This cross-sensitization effect not only augments responsiveness to drugs but also increases sensitivity to natural rewards, including those associated with social functioning[79]. Among varied drugs that are abused concurrently with alcohol, we could not a priori predict the net result. Therefore, exploratory analyses were employed to examine directionality and even the presence of potential interactions.
METHODS
Subjects
Study subjects were enrolled from nine addiction treatment centers geographically dispersed throughout the United States, namely Inflexxion, Addiction Recovery Resource, Catholic Charities of Maine, Center for Psychiatric Medicine, G & G Holistic Addiction Treatment Center, Integrative Life Center, Malibu Beach Recovery Center, Meadows Edge Recovery Center, and Tennessee Treatment Center. All study protocols were reviewed and approved by the PATH FOUNDATION IRB and received an exemption notification. The participants provided written informed consent after the study procedures were thoroughly explained to them. The genotyping data collection process conformed to the standards defined by the Health Insurance Portability and Accountability- and Genetic Information Non-Discrimination Acts.
Exclusion/Inclusion criteria
Since this paper primarily involved genotyping whereby the subjects volunteered to provide cheek cells for DNA analysis, whereby DNA is not altered by environmental factors including disease, the exclusion criteria included exposure to radiation or pollution toxicity. The second criterion involved English language comprehension, as each participant had to complete an online ASI Media V questionnaire.
Biopsychosocial assessments
Patients were interviewed and evaluated by Addiction Specialists using a standard battery of psychometric and -diagnostic questionnaires. Alcohol and drug use data were obtained using the ASI-Multi-Media
Sample collection and chain of custody
Participants were instructed to provide approximately 2 mL of saliva into a collection tube provided by the Institute for Behavioral Genetics (IBG) at the University of Colorado Boulder, Boulder, CO. Each sample was assigned a predetermined identification number and barcoded by Dominion Diagnostics. The saliva was stabilized using a buffer solution composed of Tris-EDTA, sodium dodecyl sulfate, and proteinase K at pH 8.0. Specimens were stored at room temperature at the collection sites and subsequently shipped to Dominion Diagnostics for transfer to Andrew Smollen at IBG for DNA extraction and isolation using standardized protocols[83].
Genotyping
An index of the genes included in the GARS panel and their associated polymorphisms are provided in Table 1. Each genetic variant or polymorphism was selected based on its well-established association with RDS, specifically its role in contribution to hypodopaminergic functioning within the brain’s reward circuitry. The allele and genotype frequencies observed for each variant align closely with those reported previously and in publicly available databases, suggesting that genotyping error (i.e., a ready source of bias that can reduce the power to detect a true effect) did not contribute to the observed frequencies.
GARS panel: genetic variants and risk alleles
Polymorphisms/Repeat | Gene | Variants | Risk allele |
Single nucleotide polymorphisms (SNPs) | |||
rs4532 | Dopamine D1 Receptor (DRD1) | A/G | G |
rs1800497 | Dopamine D2 Receptor (DRD2) | A1 (A)/A2 (G) | A1 |
rs6280 | Dopamine D3 Receptor (DRD3) | C/T | C |
rs1800955 | Dopamine D4 Receptor (DRD4) | C/T | C |
rs4680 | Catechol-O-Methyltransferase (COMT) | A (Met)/G (Val) | G |
rs1799971 | Mu-Opioid Receptor (OPRM1) | A (Asn40)/G (Asp40) | G |
Simple sequence repeats (variable number tandem repeats & insertion/deletions) | |||
3’ 40 base-pair repeat | Dopamine Transporter Receptor (DAT1) | 9 repeat (R) | 9R |
Intron 3, 48 base-pair repeat | Dopamine D4 Receptor (DRD4) | 7, 8, 9, 10, 11 repeats (R) | 7R |
3’ 30 base-pair Repeat | Monoamine Oxidase A | 3.5, 4, 5 repeats (R) | 4R |
43 base-pair 5’ insertion/deletion + rs25531 (5HTTLPR) | Serotonin Transporter Receptor (5HTT) | S (short) or Lg | S’ |
Dinucleotide repeats | |||
CA-repeat | Gamma-Aminobutyric Acid (GABA) A Receptor, beta 3 (GABRA3) | 171 - 201 | 181 |
Because we did not have RDS-free controls, we decided to count the number of alleles instead of developing a weighted power analysis (utilizing Odds Ratios). To further understand the relationship between ten genes and eleven polymorphisms employed in this study and the power of counting compared to weighing the power of each gene polymorphism, a subsequent test was statistically utilized, whereby each allele was provided with a higher power score (without actual ORs each allele was multiplied by a number above 1 to provide a differential power). In doing so, this test resulted in non-significance. Furthermore, switching an allele, for example, 10 R rather than 9R for DAT1, similarly canceled any statistical significance. Such manipulations suggest that utilizing the cluster of candidate genes and strict polymorphisms of the reward genes as represented by GARS provides discriminatory validity for addiction features defined via ASI clinical scores.
Assays for Amelogenin, MAOA-uVNTR, 5HTTLPR, DRD4, and DAT1[71] were performed using a multiplex polymerase chain reactiom (PCR) approach. In each 20 µL reaction, 2 µL of DNA (20 ng or less) was combined with 1.8 mM MgCl2, 180 µM of each deoxynucleotide triphosphate (dNTP, NEB), and 10% DMSO, with
Marker, primer, and resulting size ranges of characterized polymorphisms
Primer | Sequence (5’ → 3’) | Concentration (nM) | Size range (bp) |
Amelogenin-F | NED-CCC TGG GCT CTG TAA AGA ATA GTG | 300 | 103,109 |
Amelogenin-R | ATC AGA GCT TAA ACT GGG AAG CTG | 300 | (X, Y) |
MAO-uVNTR-F | 6FAM-ACA GCC TGA CCG TGG AGA AG | 200 | 291-381 |
MAO-uVNTR-R | GAA CGG ACG CTC CAT TCG GA | 200 | (2R-5R) |
DAT1-F | 6FAM-TGT GGT GTA GGG AAC GGC CTG AG | 300 | 200-600 |
DAT1-R | CTT CCT GGA GGT CAC GGC TCA AGG | 300 | (3R-13R) |
DRD4-F | VIC-GCT CAT GCT GCT GCT CTA CTG GGC | 600 | 279-711 |
DRD4-R | CTG CGG GTC TGC GGT GGA GTC TGG | 600 | (2R-11R) |
5HTTLPR-F | NED-ATG CCA GCA CCT AAC CCC TAA TGT | 600 | 376, 419-549 |
5HTTLPR-R | GGA CCG CAA GGT GGG CGG GA | 600 | (S, L-XL) |
5HTTLPR-Hu-F | 6FAM-GCA ACC TCC CAG CAA CTC CCT GT | 500 | 138, 181 |
5HTTLPR-Hu-R | GAG GTG CAG GGG GAT GCT GGA A | 500 | (S, L) |
GABRB3-F | 6FAM-CTC TTG TTC CTG TTG CTT TCA ATA CAC | 500 | 171 - 201 |
GABRB3-R | CAC TGT GCT AGT AGA TTC AGC TC | 500 |
The analysis of the dinucleotide repeat in GABRB3 was performed in a reaction containing 2 µL of DNA (20 ng or less), 200 µM of each deoxynucleotide triphosphate (dNTP, NEB), 2.5 mM MgCl2_22, fluorescently labeled forward and reverse primers (details provided in Table 2), 1 unit of AmpliTaq Gold® polymerase, and 1× PCR buffer II, resulting in a total reaction volume of 20 µL[84]. Amplifications utilized a modified touchdown PCR protocol[85,86].
The PCR cycling protocol commenced with an initial denaturation at 95 °C for 10 min. This was followed by two cycles consisting of 95 °C for 30 s, 72 °C for 60 s, and 65 °C for 30 s. Subsequently, the annealing temperature was decreased by 2 °C every two cycles from 65 °C to 57 °C across 10 cycles. This phase was then succeeded by 30 cycles of 95 °C for 30 s, 55 °C for 30 s, and 72 °C for 60 s. The reaction concluded with a final extension at 72 °C for 30 min, followed by a hold at 4 °C. To ensure assay accuracy, each 96-well plate incorporated non-template controls and DNA standards with established genotypes.
The assay for rs25531 (A/G), located within the long form of the 5HTTLPR region, has been described in detail elsewhere. This SNP facilitates the distinction between the LA and LG alleles. Amplification of the 5HTTLPR site containing the SNP was performed using a single PCR reaction composed as previously described, with primers referenced in prior studies. The thermal cycling conditions included an initial denaturation at 95 °C for 10 min, followed by two cycles of 95 °C for 30 s, 65 °C for 30 s, and 72 °C for 60 s. This was followed by two cycles at 95 °C for 30 s, 63 °C for 30 s, and 72 °C for 60 s, then 30 cycles at 95 °C for 30 s, 61 °C for 30 s, and 72 °C for 60 s. The process concluded with a final extension at 72 °C for 30 min and a hold at 4 °C.
Following PCR amplification, the products were incubated with five units of MspI (NEB, Ipswitch, MA) at 37 °C for 90 min[87]. The presence of a 97 bp restriction fragment indicated the LG allele. For the GARS panel, two combined alleles were reported: S’, which includes the S and LG alleles, and L’, which comprises the LA and extra-long alleles. These categories represent activity bins rather than individual alleles. Each
Genotyping of the SNPs TaqIA (rs1800497), COMT val158met (rs4680), DRD1 (rs4532), DRD3 (rs6280), DRD4-521C/T (rs1800955), and OPRM1 (rs1799971) was performed using the fluorogenic 5’ nuclease assay (TaqMan®, ABI, Foster City, CA)[88] on an ABI Prism® 7000 Sequence Detection System in allelic discrimination mode[89,90]. Each reaction was conducted in a total volume of 15 µL, containing 20 ng of genomic DNA, TaqMan® Universal PCR Master Mix, and primers and probes at final concentrations of
Probe and primer sequences for COMT val158met and DRD2 Taq1A polymorphisms
Gene | Probe/Primer | Sequence (5’ → 3’) |
COMT | T (met) probe | VIC-ACCTTGTCCTTCATGCCAGCGAAAT-NFGMGB |
C (Val) probe | FAM-CCTTGTCCTTCACGCCAGCGA-NFQMGB | |
Forward primer | TCGAGATCAACCCCGACTGT | |
Reverse primer | AACGGGTCAGGCATGCA | |
DRD2 | T (A1) probe | VIC-CCTGCCTTGACCAGC-NFQMGB |
C (A2) probe | FAM-CTGCCTCGACCAGC-NFQMGB | |
Forward primer | GTGCAGCTCACTCCATCCT | |
Reverse primer | GCAACACAGCCATCCTCAAAG |
Statistical analysis
Mean, standard deviations, regression tests, and chi-square were executed using SPSS (Version 21.0). Significant differences between dichotomized alcohol and drug severity scores were assessed using a Fischer Exact Test. Scores above and below the means of 4.65 for alcohol and 4.00 for drugs were respectively determined to be “high” (1) and “low” (0). Regression analyses were executed to determine the relationship between the severity risk score and genetic risk score, adjusting for age (continuous) and sex (dichotomous) variables. The distribution of scores on the alcohol and drug severity risk scales exhibited a slight skew toward lower values. To assess potential normalization, the effects of square-root and log-transformations were examined. In accordance with predefined criteria, each identified risk allele was assigned a value of 1. An individual's overall genetic risk score was then determined by summing the total number of risk alleles present within their genotype.
A dichotomous addiction risk scale was created by classifying those at or below the mean of 7.97 as having a “low” number of risk alleles and those above the mean as having a “high” number of addiction risk alleles. GARS severity scores were computed by dividing the summed GARS score by the total possible number of alleles (22 alleles from 11 genes). Scores below 0.30 were binned together in the “low” severity range, scores between 0.31 and 0.69 into the “moderate” severity range, and 0.70 to 0.99 into the “high” severity range. Hardy Weinberg equilibrium (HWE) was confirmed for each gene using chi-square tests.
RESULTS
Sample characteristics
The mean age of this sample was 35.3± standard deviation (SD) = 13.1 years; 57.8% (n = 160) of the sample were males and 88.1% (n = 244) reported their race as White [Table 4]. The “consented” subjects at seven different treatment centers were similar in terms of their demographics, except for younger males at the
Demographics by treatment center (N = 273)
Treatment center | N (%) | Gender | Age (years) mean (standard deviation, N) | Ethnicity (% white) |
Addiction recovery resource | 39 (14.1) | Males | 32.9 (12.7, 29) | 96.6 |
Females | 35.5 (15.1, 10) | 80.0 | ||
Catholic charities - maine | 41 (14.8) | Males | 33.7 (9.5, 34) | 88.2 |
Females | 40.4 (10.7, 1) | 85.7 | ||
Center for psychiatric medicine | 1 (0.4) | Males | 34.0 (0, 1) | 100 |
Females | -- | -- | ||
G & G holistic addiction treatment centers | 108 (39.0) | Males | 30.9 (12.0, 59) | 88.1 |
Females | 37.1 (14.2, 49) | 91.8 | ||
Integrative life center | 2 (0.9) | Males | 22 (0, 1) | 100 |
Females | 22 (0, 1) | 100 | ||
Malibu beach recovery center | 65 (23.5) | Males | 38.1 (13.8, 28) | 85.7 |
Females | 39.8 (14.3, 37) | 86.5 | ||
Meadows edge recovery center | 17 (6.1) | Males | 36.9 (15.4, 8) | 100 |
Females | 37.3 (11.3, 9) | 88.0 |
Allele and genotype frequencies
Among the discovery sample (n = 393), 17.6% scored in the “low severity” range, 80.7% in the “moderate” severity range, and 1.5% in the “high” severity range. No deviations from HWE were detected for any of the genotypes generated [Tables 5 and 6].
Allele and genotype frequencies of specific genes
Gene | N | Allele | Genotype | HWE (P-value) | Risk allele | |||
DRD1 | 368 | T | C | TT | CT | CC | 0.95 | C |
0.66 (488) | 0.34 (246) | 0.44 (162) | 0.45 (164) | 0.11 (42) | ||||
DRD2 (rs1800497, Taq1A) | 381 | A1 | A2 | A1A1 | A1A2 | A2A2 | 0.99 | A1 |
0.25 (188) | 0.75 (574) | 0.06 (23) | 0.37 (141) | 0.57 (216) | ||||
DRD3 (rs6280) | 383 | T | C | TT | CT | CC | 0.97 | C |
0.62 (472) | 0.38 (294) | 0.38 (145) | 0.47 (181) | 0.15 (56) | ||||
DRD4 (rs180095) | 350 | T | C | TT | CT | CC | 0.99 | C |
0.51 (360) | 0.49 (340) | 0.26 (92) | 0.50 (174) | 0.24 (82) | ||||
COMT (rs4680) | 380 | A | G | AA | AG | GG | 0.99 | G |
0.45 (345) | 0.55 (415) | 0.21 (78) | 0.50 (188) | 0.30 (113) | ||||
OPMR1 | 385 | A | G | AA | AG | GG | 0.86 | G |
0.87 (670) | 0.13 (100) | 0.76 (291) | 0.23 (87) | 0.02 (6) | ||||
DAT1 VNTR | 372 | 9R | 10R | 9R/9R | 9R/10R | 10R/10R | 0.97 | 9R |
0.25 (187) | 0.75 (557) | 0.06 (23) | 0.38 (139) | 0.56 (208) | ||||
DRD4 VNTR | 372 | 4R | 7R | 4R/4R | 4R/7R | 7R/7R | 0.98 | 9R |
0.78 (587) | 0.22 (165) | 0.61 (229) | 0.34 (128) | 0.05 (18) | ||||
MAOA-uVNTR VNTR* | 375 (males + females) | 3R | 4R | 3R/3R | 3R/4R | 4R/4R | 4R | |
0.37 (274) | 0.63 (476) | 0.13 (50) | 0.46 (173) | 0.40 (151) | ||||
MAOA-uVNTR VNTR | 169 (females only) | 3R | 4R | 3R/3R | 3R/4R | 4R/4R | 0.96 | 4R |
0.37 (124) | 0.63 (214) | 0.13 (22) | 0.46 (78) | 0.40 (68) | ||||
DRD1 (rs 4531) | 274 | T | C | TT | CT | CC | 0.96 | C |
0.66 (359) | 0.34 (189) | 0.43 (117) | 0.45 (123) | 0.12 (32) | ||||
DRD2 (rs1800497, Taq1A) | 278 | A1 | A2 | A1A1 | A1A2 | A2A2 | 0.97 | A1 |
0.24 (135) | 0.76 (421) | 0.06 (16) | 0.37 (102) | 0.57 (159) | ||||
DRD3 (rs6280) | 277 | T | C | TT | CT | CC | 0.96 | C |
0.62 (345) | 0.38 (209) | 0.39 (107) | 0.47 (130) | 0.14 (39) | ||||
DRD4 (rs180095) | 271 | T | C | TT | CT | CC | 0.99 | C |
0.51 (278) | 0.49 (264) | 0.26 (71) | 0.50 (135) | 0.24 (64) | ||||
COMT (rs4680) | 271 | A | G | AA | AG | GG | 0.99 | G |
0.49 (278) | 0.51 (264) | 0.24 (64) | 0.50 (135) | 0.36 (71) | ||||
OPMR1 (rs1799971) | 278 | A | G | AA | AG | GG | 0.97 | G |
0.88 (490) | 0.12 (66) | 0.78 (215) | 0.21 (58) | 0.01 (4) | ||||
DAT1 VNTR | 277 | 9R | 10R | 9R/9R | 9R/10R | 10R/10R | 0.93 | 9R |
0.24 (135) | 0.76 (419) | 0.06 (16) | 0.37 (102) | 0.57 (158) | ||||
DRD4 VNTR | 275 | 4R | 7R | 4R/4R | 4R/7R | 7R/7R | 0.95 | 9R |
0.76 (420) | 0.24 (130) | 0.58 (160) | 0.36 (99) | 0.06 (15) |
Repeat number and genotype frequencies of specific genes
Gene | N | Repeat number | Genotype | HWE (P-value) | Risk allele | |||
GABRA3 dinucleotide repeat | 362 | 179* | 0.71 (512) | 179/179 | 179/181 | 181/181 | 0.97 | 181 |
181 | 0.29 (212) | 0.50 (181) | 0.41 (149) | 0.09 (31) | ||||
5HTTLPR insertion/deletion and rs25531 SNP** | 371 | S’ | 0.51 (377) | S’/S’ | S’/L’ | L’/L’ | 0.96 | S’ |
L’ | 0.49 (365) | 0.26 (96) | 0.50 (185) | 0.24 (90) | ||||
GABRA3 dinucleotide repeat allele | 274 | 179* | 0.70 (382) | 179/179 | 179/181 | 181/181 | 0.98 | 181 |
181 | 0.30 (166) | 0.49 (133) | 0.42 (115) | 0.09 (25) | ||||
5HTTLPR insertion/deletion and rs25531 SNP** | 271 | S’ | 0.49 (264) | S’/S’ | S’/L’ | L’/L’ | 0.96 | S’ |
L’ | 0.51 (278) | 0.23 (64) | 0.50 (135) | 0.26 (71) |
Alcohol and drug severity scores
ASI alcohol and drug severity scores were slightly skewed to the left (Alcohol: skewness = -0.211, kurtosis = -1.473; Drugs: skewness = -0.922, kurtosis = -0.483), suggesting a mild-moderate range of addictive problems related to chemical substances. Patients with high alcohol severity ratings had increased psychiatric (χ2 = 10.26, P = 0.001), family- and medical- (χ2 = 8.20, P < 0.004), but not economic- or legal problems (χ2 > 0.1). Higher GARS was associated with higher alcohol severity scores (χ2 = 8.84, P = 0.004); this association survived gender and age adjustment. When dichotomized alcohol severity scores (low and high) were employed, there was a trend (χ2 = 3.37, P = 0.07) association. Patients with “high” drug severity scores had increased psychological- (χ2 = 8.26, P = 0.004) and family- (χ2 = 11.7, P = 0.001), but not medical-, economic-, or legal problems (χ2 > 0.1). Those with high drug severity had more addiction risk alleles, i.e., heightened GARS (P = 0.05); this did not seem to be a linear association evidenced in an insignificant result when continuous drug severity values were considered (χ2 = 1.43, P = 0.71); the contributions of age and gender were significant (See Figures 1-4 and Tables 7-9).
Figure 2. The allelic distribution of the cohort that completed the ASI questionnaire (n = 273). ASI: Addiction severity index.
Figure 3. Distribution of genetic addiction risk alleles as a function of “low” and “high” alcohol severity ratings scores.
Figure 4. Distribution of genetic addiction risk alleles as a function of ‘low” and “high” drug severity ratings scores.
Mean, standard deviation, sample size for alcohol & drug risk severity scores and genetic addiction risk scale by treatment center and gender
Treatment center | Gender | Alcohol risk severity score Mean (standard deviation, N) | Drugs risk severity score Mean (standard deviation, N) | GARS Mean (standard deviation, N) |
Addiction Recovery Resource | Males | 5.15 (2.65, 26) | 6.64 (1.85, 28) | 7.90 (1.88, 29) |
Females | 6.11 (2.52 9) | 6.00 (2.16, 7) | 9.80 (3.49, 10) | |
Catholic Charities - Maine | Males | 3.33 (2.23, 30) | 5.75 (2.14, 32) | 7.62 (2.39, 34) |
Females | 4.00 (2.94, 4) | 6.60 (1.52, 5) | 7.71 (2.87. 7) | |
Center for Psychiatric Medicine | Males | 2.00 (0, 1) | 4.00 (0, 1) | 7.00 (0, 1) |
Females | -- | -- | -- | |
G & G Holistic Addiction Treatment Centers | Males | 5.46 (2.22, 39) | 5.24 (2.73, 42) | 8.08 (2.18, 49) |
Females | 4.71 (2.74, 42) | 6.47 (1.95, 59) | 7.71 (2.40, 59) | |
Integrative Life Center | Males | 1.00 (0, 1) | 5.00 (0, 1) | 7.00 (0, 1) |
Females | 4.00 (0, 1) | 5.00 (0, 1) | 7.00 (0, 1) | |
Malibu Beach Recovery Center | Males | 4.65 (2.65, 23) | 5.34 (2.91, 26) | 7.96 (1.97, 28) |
Females | 4.44 (2.96, 34) | 5.33 (3.10, 27) | 8.38 (2.25, 37) | |
Meadows Edge Recovery Center | Males | 5.67 (2.94, 6) | 4.38 (2.50, 8) | 7.88 (2.14, 8) |
Females | 3.29 (2.63, 7) | 5.43, 3.04, 7) | 7.67 (3.32, 9) |
Parameter estimates and summary statistics for ASI alcohol risk severity score
Variable | B | S.E. | Wald Chi-Square | Df |
Intercept | -1.93 | 0.52 | 13.84 | 1 |
Sex | -0.19 | 0.29 | 0.42 | 1 |
Age | 0.05 | 0.11 | 15.29 | 1 |
GARS | 0.74 | 0.29 | 6.39 | 1 |
Parameter estimates and summary statistics for ASI drug risk severity score
Variable | Non-standardized Beta | Unstandardized S.E. | Standardized Beta | T | Significance |
Intercept | 0.92 | 0.09 | - | 10.50 | < 0.001 |
Sex | 0.07 | 0.04 | 0.12 | 1.87 | 0.06 |
Age | -0.01 | 0.01 | -0.21 | -3.43 | 0.001 |
GARS | -0.13 | 0.01 | -0.10 | -1.59 | 0.11 |
DISCUSSION
This study was carried out with a medium-sized cohort of addicted patients recruited from seven diverse chemical dependence programs. Besides gender at one center, there were no significant variations in any of the tested parameters across the cohort. Consequently, the dataset meets the Hardy-Weinberg Equilibrium criteria[91] for gene polymorphism distribution, thus enhancing our findings’ validity. An important aspect of the present multi-locus approach[92,93] study is that it captures the polymorphisms affecting the following fundamental processes of the dopaminergic system: (a) dopamine transport across the neuron; (b) synaptic metabolism of dopamine; (c) dopamine binding at the primary receptor subtypes; (d) endorphinergic sites determining the synaptic content of dopamine that are influenced by serotonin transporters, and e) GABA receptors that are inhibited via opiate receptor influencing the neuronal release of dopamine. A higher number (≥ 7) of reward-gene-polymorphisms linked to reduced dopamine signaling were significantly associated with increased ASI-MV alcohol severity scores. Similarly, a high number (≥ 4) of such polymorphisms were significantly correlated with higher ASI-MV drug severity scores.
Interestingly, the importance of a hypodopaminergic trait was demonstrated when significance was improved by exchanging specific SNPs of the DAT 10 allele with reduced dopaminergic function (9 alleles). Another key finding from this study is that these genetic influences are additive. We also found that age, but not gender, was a predictor of the ASI alcohol and drug severity scores. This finding may have molecular underpinnings given the well-described age-related D2 density reduction[94]. Since age is associated with hypodopaminergic state due to a reduced number of D2 receptors[95], it may drive substance-seeking behaviors[96]. In short, a combination of age and high GARS should have clinical relevance and may even serve as the basis for enhanced RDS spectrum behaviors[97], e.g., alcoholism in the elderly[98].
The present results extend our earlier report on the allelic association of the dopamine D2 receptor gene in alcoholism, in which we suggested that the presence of a specific allele predicted 77% of alcoholics whereas lack thereof predicted 72% of non-alcoholics[99]. Although there were conceptual similarities between the latter and present study (e.g., alcoholic subjects and genotyping techniques), there were also important differences, including the comprehensive panel measuring reward genes and polymorphisms, a larger sample size, as well as the focus on a clinical population rather than on post-mortem samples. Thus, the present data demonstrate a creation validity of GARS against the established criterion, that is to say, the ASI, which is the most commonly used psychometric tool in substance use disorders (https://www.sciencedirect.com/topics/medicine-and-dentistry/addiction-severity-index).
The observed pattern in ASI drug severity scores (i.e., higher GARS in patients with high vs. low drug severity) is consistent with prior observations[100], including the association of the DRD2/AAKKI haplotype with comorbid alcohol- and drug addiction[101]. However, a lack of linear relationship between GARS and ASI drug severity scores calls for further inquiry into the type of this relationship. Several hypotheses could explain this non-linear relationship. One possibility is that at a certain severity level, individuals might substitute drugs with alcohol consumption, which could temporarily improve some aspects of drug-related severity[102]. Alternatively, a more complex independent pattern may emerge particularly given the heterogeneous nature of addictive substances and their varying effects on the dopaminergic system[103]. It is also possible that the obtained results are a mere reflection of the underreporting of illegal drug consumption as compared to a legal substance, alcohol. Regardless of the underlying factors, it is critical for clinical assessments of addicted patients to account for all classes of addictive substances for comprehensive psychopathology characterization and for formulation of optimal treatment plans. Importantly, patients with “high” alcohol severity ratings also had increased psychological, family, and medical but not economic or legal problems. A similar pattern was recognized for those scoring in the “high” range of drug severity ratings, that is to say, increased psychological and familial but not medical, economic, or legal problems. In contrast, we did not detect a significant difference in the diagnosed psychiatric disorders. Taken together, these observations support further inquiry into additional GARS’ validity criteria along with disentanglement of inherited phenotypic traits vs. the ones that are acquired prior- or during addictive disorders.
Caveats
Overall, our results support the contribution of a hypodopaminergic trait to the pathogenesis of alcohol and drug use disorders, with potential implications for other non-substance-related RDS behaviors. Nonetheless, in addition to the aforementioned heterogeneous drug consumption patterns, several other caveats are usefully stated in advance, including a relatively modest sample size, lack of complementary psychodiagnostic assessments alongside the ASI, such as the Structured Clinical Interview for DSM-5, or the lack of additional psychometric assessments, such as the RDS Questionnaire or a uniform and validated RDS severity score index[104]. Additionally, while the results are robust, uncontrolled environmental factors could have affected dopamine and other monoamine metabolism and function, including storage, catabolism, synthesis, transport, cellular distribution and concentration, neuronal release, synaptic clearance, and catabolism, as well as other intracellular gene and environmental interactions.
As with the identification of substance-related diagnoses, patient characterization factors such as comorbidities, medications, demographics, personality traits, and overall health status could also potentially confound the outcomes of this sort of genetic study. For example, the presence of depression, even if it occurred long before the study, may alter the brain’s reward system[105], as might the concurrent use of psychotropic medicines[106,107].
Given the substantial prevalence of depression[108], however, implementing this as an exclusion factor would likely rule out such a high percentage of patient candidates as to make clinical genetic studies unfeasible. An alternative way for evaluating the origin of the hyporesponsive reward system in alcohol and drug addiction is through examination of monozygotic twins discordant for alcohol/drug exposure or via prospective studies.
The ideal technique for risk stratification is still being debated. The utility of a specific test in identifying a risk factor within a population is often conveyed through measures such as the odds ratio or relative risk for complex behaviors, such as RDS, in individuals with positive versus negative test results. However, the ability of current tests, including the GARS panel, to predict RDS on an individual level remains limited. In general, odds ratios exceeding 15 to 20 are typically required to meaningfully impact individual risk prediction[109]. Such high odds ratios are rarely observed for individual dichotomous predictors or continuous covariates, even when there are significant differences between affected and unaffected groups.
A comprehensive evaluation of a risk marker or risk score requires analyzing multiple parameters that reflect distinct performance characteristics. Essential metrics include sensitivity, specificity, area under the receiver operating characteristic (ROC) curve (AUC) or C-statistic, informativeness of the criteria, clinical likelihood ratios, model calibration, and reclassification. Although ROC curves and AUC were initially developed for assessing diagnostic tests, they serve as valuable tools for determining the discriminative power of a risk estimator. In terms of the GARS test, we will continue our research utilizing “super controls” to allow for appropriately weighted genes and apply logistic regression analysis to determine odds ratios and ROC. However, the use of trichomization is informative and has been standard in the field of medicine as utilized by pencil and paper questionnaires like the ASI.
Future directions
Our findings indicate that incorporating genetic models into routine clinical practice has the potential to enhance the personalization of preventive, diagnostic, and therapeutic interventions. However, a significant challenge lies in selecting appropriate tests, validating their clinical relevance, and identifying disorders that can be effectively applied to real-world patients. Since patient well-being cannot be adequately addressed through simplistic definitions, it is crucial to define suitable targets for RDS models. These targets should account for factors such as environmental, lifestyle, psychological, and neuroendocrine influences. Conversely, the use of inappropriate methodologies could lead to counterproductive outcomes, including inconclusive or irrelevant results. Furthermore, expanding the GARS panel to include additional candidate genes, such as those involved in the P450 enzyme system[110], FGF21[111], and APOE, could significantly enhance its clinical relevance and broaden its applicability.
Understanding genetic testing
If early diagnosis leads to better treatment outcomes, then at what point should the proposed paradigm shift using genotyping be adopted? Federal and state mandates already require gene testing at birth for some conditions. Unlike rare diseases with limited treatment options (e.g., sickle cell anemia, Huntington’s disease, congenital hypothyroidism, phenylketonuria, and galactosemia), RDS has effective therapeutic strategies[112]. Although RDS is not instantaneously life-threatening, early genetic testing could allow for timely diagnosis and non-pharmacologic interventions. It may transpire that exercise, diet, psychotherapy, behavioral interventions, or a safe and non-stimulant nutraceutical dopaminergic agonist therapy reduces the gene abnormality’s impact[113]. For instance, attention deficit hyperactivity disorder (ADHD), a subtype of RDS[114], is diagnosed in children and extends into adulthood. If not treated in a timely fashion, ADHD may constitute a risk factor for the development of substance use disorders[115]. Accordingly, even lay media (Bill Moyers of PBS) has suggested the heuristic value of early ADHD diagnosis for the prevention of the ensuing comorbid disorders. We propose that GARS be considered as a pre-diagnostic tool for early identification of RDS in our young people, including those with ADHD.
Limitations
A common in psychiatric genetics, including this article, is the inadequate or incomplete screening of controls. While our results are promising, we encourage future studies involving a larger population. If indeed others perform genome-wide association study (GWAS) studies directed at all addictive substance and non-substance behaviors (RDS), we caution that all controls are extensively screened for hidden RDS behaviors like gaming, overeating, hoarding, etc. The lack of employing highly screened controls free of RDS behaviors, in our opinion, may be a significant flaw in current and previous studies. Understanding this caveat could significantly reduce spurious results.
Statistical validation of GARS
The initial statistical evaluation concentrated on reward-related genes and their polymorphisms, predominantly associated with reduced dopamine activity within the mesolimbic brain reward circuitry. The GARS panel was assessed for its ability to predict risk for AUD using data from 74,566 case-control subjects[116]. Analysis of the identified risk alleles demonstrated significant odds ratios (OR) with 95% confidence intervals (CI), alongside an estimated population prevalence of alcoholism at 8%. Genes encoding dopamine receptors, including DRD1, DRD2, DRD3, and DRD4, as well as DAT1, COMT, OPRM1, and SLC6A4, exhibited significant associations with AUD risk compared to non-AUD controls, based on meta-analytic OR data. However, polymorphisms in GABRB3 and MAOA did not reach statistical significance, likely due to insufficient sample sizes.
Ongoing investigations into dopaminergic dysregulation underlying a hypothesized “pre-addiction” phenotype are currently under review. This research involves an extensive in silico analysis of GWAS data encompassing 88,788,381 samples from 1,373 studies, identifying 18 significant genes. Among these, APOE (P-value = 1.0E-126) was associated with pathways linked to opioid signaling, pain regulation, aging, and apoptosis. These findings are being integrated with the GARS test, highlighting key genes such as MAOA, COMT, APOE, and SLC4A6 as central components of these pathways, as identified through STRING-based interaction modeling. Additionally, the analysis revealed interactions with microRNAs hsa-miR-16-5p and hsa-miR-24-3p, while expanding the role of SLC6A4 to include interactions with 27 unique genes. Pharmacogenomic analyses identified 1,173 variant annotations for these genes, corroborated by enrichment and meta-analytic studies.
Collectively, these findings emphasize the critical role of dopaminergic pathways in linking addictive behaviors with depressive symptoms. They further propose RDS as a foundational “pre-addiction” phenotype, with pain, opioid dependency, aging, and apoptosis emerging as significant endophenotypic contributors.
Application of GARS in preaddiction screening
There is a critical need for the development of new therapies and reliable, safe, and effective screening methods for SUDs. Despite significant federal investment in research and treatment innovation over the past decades, treatment penetration rates remain below 25%[2]. A comparable situation occurred in the diabetes field, where early identification and intervention for prediabetes led to a substantial improvement in treatment penetration and outcomes[117,118]. The prediabetes model, which facilitates early detection and timely intervention, has proven successful in slowing the progression of the disease[117,118]. Similarly, the emerging concept of “preaddiction” has been proposed for inclusion in the DSM. Preaddiction may reflect a disruption in hedonistic homeostatic regulation, such as hypodopaminergia in the mesolimbic reward system, and is linked to a range of neurochemical imbalances involving opioidergic, serotonergic, cannabinergic, GABAergic, glutaminergic, and cholinergic pathways[60,116,119]. Therefore, Genetic testing, such as the GARS test, could be employed to screen for individuals at risk of preaddiction, as it identifies genetic predispositions to addictive behaviors and has been shown to predict the severity of alcohol and drug use.
Summary
Our study found a significant association between a ten-gene panel with eleven SNPs linked to a hypodopaminergic trait and ASI-MV alcohol and Drug severity scores. Seven or more reward-gene-polymorphisms were correlated with higher ASI-MV alcohol severity, while four or more were linked to higher ASI-MV drug severity scores. Replacing DAT 10 allele SNPs with DAT 9 allele SNPs, indicative of greater hypodopaminergic function, led to significant improvement. These genetic effects are additive and influenced by age, but gender was not a predictor of severity scores.
While the candidate gene approach may still be controversial[120], to our knowledge, this study is the first to correlate a panel of genes with polymorphisms reflecting the “Brain Reward Cascade”[121]. Future research should extend the GARS test to include more genes and polymorphisms linked to hypodopaminergic traits. The GARS test can be used to improve clinical interactions and decision making by reducing denial, validating family genetic patterns, and guiding assessment of addiction/relapse risk and treatment plans. Larger studies are needed for confirmation, and the inclusion of RDS “free controls” would undoubtedly strengthen the results and assist in determining accurate odds ratios for each allele.
Since our 1990 JAMA report linking the DRD2 Taq A1 allele to severe alcoholism, genetic candidate association studies, including GWAS, have proliferated. To identify individuals at risk for at least AUD, Blum’s group developed a GARS test, which includes ten genes and eleven associated risk alleles, as described herein. We validated these risk alleles by analyzing studies from 1990 until 2021 involving 74,566 case-controls related to AUD. This analysis calculated the Hardy-Weinberg Equilibrium of each polymorphism in cases and controls and used Pearson’s χ2or Fisher’s exact tests for gender, genotype, and allele distribution. The analyses found the OR, 95%CI for OR, and a post-risk estimation of 8% for the population’s alcoholism prevalence revealed a significant detection. The OR results showed significance for DAT1, DRD2, DRD3, DRD4, COMT, OPRM1, and 5HTT at 5%. While most GARS research is derived from our laboratory, we encourage more independent research to confirm our findings[71,120-144].
Conclusion
This study identified a significant association between the GARS panel and the ASI-MV alcohol severity score, reinforcing previous findings that link dopaminergic function to alcoholism and substance abuse. The results also suggest that polygenic risk factors defined by GARS may be modulated by age-related pathophysiological and environmental factors. Future research, including GWAS, is warranted to explore the endophenotypes associated with RDS, which arise from a hypofunctional dopaminergic system.
DECLARATIONS
Acknowledgments
The authors appreciate the staff at Western University Health Sciences, Pomona, CA., USA and a number of individuals that contributed to this investigation: Carolee Lindsey, Joan Borsten, Elizabeth Winchel, Holly Cook, Adi Jaffe, Colin Hanna, Luis Llanos Gomez, Ashim Gupta, Stephanie Bickley, Aryeh R Pollack, Albert Villapiano, Lee McCormick, Edward Chapman, Marjorie C. Gondre-Lewis, and John Femino.
Authors’ contributions
This work was accomplished at Colorado University Bouler -Institute of Behavioral Genetics, under the direction of: Smolen A, Haberstick BC, Blum K
The first draft was written: Blum K, Smolen A, Haberstick BC
The statistics were accomplished: Haberstick BC, Han D
The entire manuscript was initially edited: Elman I, Pinhasov A
All data were collected and housed at Dominion: Hauser M
The ASI Data were produced at INFEXXION: Bowirrat A
The figures were developed: Gilley G
Each author edited and approved the final manuscript: Blum K, Haberstick BC, Smolen A, Han D, Elman I, Dennen CA,Oscar-Berman M, Smith DE, Simpatico T, Giordano J, Braverman ER, Hauser M, Butler S, Roy lll AK, Thanos PK, Inaba D, Fried L, Ceccanti M, Zeine F, Jafari N, Sunder K, Bowirrat A, Pinhasov A, Lewandrowski KU, Sharafshah A, Murphy KT, Makale M, Khalsa J, Baron DD, Badgaiyan R, Modestino EJ, Gilley E, McLaughlin T, Gold MS
Following a number of draft revisions, each author contributed comments and edits as well.
Availability of data and materials
The data involved in the study have been included in the main text. Additional raw data will be made available by the corresponding author upon reasonable request.
Financial support and sponsorship
Dr. Blum K is one of NIH recipients of R41 MD012318/MD/NIMHD NIH HHS/United States.
Conflicts of interest
The primary conflict of interest is that of the lead author Blum K. It is to be noted that Blum K is credited with an issued USA-GARS Patent and other patents on genetic testing for pre-addiction domestically and worldwide. Blum K is also credited with 22 GARS-issued European patents. Blum K has assigned these patents toTranspliceGen Holdings LLC and licensed them to TranspliceGen Therapeutics Inc. There are no other conflicts to report. Blum K is an Editorial Board member of Journal of Translational Genetics and Genomics. Blum K was not involved in any steps of the editorial process, including reviewer selection, manuscript handling, and decision making. The other authors declared that there are no conflicts of interest.
Ethical approval and consent to participate
Since the requested procedure does not involve invasive techniques on the patients in the investigation but only consists of a simple cheek cell swabbing and a pencil-paper questionnaire, it is considered exempt. All study protocols were reviewed and approved by the PATH FOUNDATION IRB, which issued an exemption notification. The participants provided written informed consent after the study procedures were thoroughly explained to them.
Consent for publication
Not applicable.
Copyright
© The Author(s) 2025.
REFERENCES
1. Spencer MR, Garnett MF, Miniño AM. Drug overdose deaths in the United States, 2002-2022. NCHS Data Brief No. 491, March 2024. Available from: https://www.cdc.gov/nchs/products/databriefs/db491.htm. [Last accessed on 11 Mar 2025].
2. Key substance use and mental health indicators in the United States: results from the 2022 national survey on drug use and health. Available from: https://www.samhsa.gov/data/sites/default/files/reports/rpt42731/2022-nsduh-nnr.pdf. [Last accessed on 11 Mar 2025].
4. Derefinko KJ, Brown R, Danzo A, et al. Addiction medicine training fellowships in north america: a recent assessment of progress and needs. J Addict Med. 2020;14:e103-9.
5. Grucza RA, Sher KJ, Kerr WC, et al. Trends in adult alcohol use and binge drinking in the early 21st-century United States: a meta-analysis of 6 national survey series. Alcohol Clin Exp Res. 2018;42:1939-50.
6. Ray LA, Grodin EN, Leggio L, et al. The future of translational research on alcohol use disorder. Addict Biol. 2021;26:e12903.
8. Tanabe J, Regner M, Sakai J, Martinez D, Gowin J. Neuroimaging reward, craving, learning, and cognitive control in substance use disorders: review and implications for treatment. Br J Radiol. 2019;92:20180942.
9. Hillmer AT, Mason GF, Fucito LM, O’Malley SS, Cosgrove KP. How imaging glutamate, γ-aminobutyric acid, and dopamine can inform the clinical treatment of alcohol dependence and withdrawal. Alcohol Clin Exp Res. 2015;39:2268-82.
10. Feltenstein MW, See RE. The neurocircuitry of addiction: an overview. Br J Pharmacol. 2008;154:261-74.
11. Buck SA, Torregrossa MM, Logan RW, Freyberg Z. Roles of dopamine and glutamate co-release in the nucleus accumbens in mediating the actions of drugs of abuse. FEBS J. 2021;288:1462-74.
12. Chiara G, Imperato A. Ethanol preferentially stimulates dopamine release in the nucleus accumbens of freely moving rats. Eur J Pharmacol. 1985;115:131-2.
13. Engleman EA, Ingraham CM, Rodd ZA, Murphy JM, McBride WJ, Ding ZM. The reinforcing effects of ethanol within the prelimbic cortex and ethanol drinking: involvement of local dopamine D2 receptor-mediated neurotransmission. Drug Alcohol Depend. 2020;214:108165.
14. Dahchour A, Ward RJ. Changes in brain dopamine extracellular concentration after ethanol administration; rat microdialysis studies. Alcohol Alcohol. 2022;57:165-75.
15. Domi E, Domi A, Adermark L, Heilig M, Augier E. Neurobiology of alcohol seeking behavior. J Neurochem. 2021;157:1585-614.
17. Elman I, Borsook D. The failing cascade: comorbid post traumatic stress- and opioid use disorders. Neurosci Biobehav Rev. 2019;103:374-83.
18. Volkow ND, Tomasi D, Wang GJ, et al. Predominance of D2 receptors in mediating dopamine’s effects in brain metabolism: effects of alcoholism. J Neurosci. 2013;33:4527-35.
19. Jayaram-lindström N, Ericson M, Steensland P, Jerlhag E. Dopamine and alcohol dependence: from bench to clinic. In: Meil WM, Ruby CL, editors. Recent advances in drug addiction research and clinical applications.2016. Available from: http://dx.doi.org/10.5772/63144. [Last accessed on 11 Mar 2025].
21. Vitali M, Napolitano C, Berman MO, et al. Neurophysiological measures and alcohol use disorder (AUD): hypothesizing links between clinical severity index and molecular neurobiological patterns. J Addict Res Ther. 2016;5:182.
22. Comings DE, Blum K. Reward deficiency syndrome: genetic aspects of behavioral disorders. Prog Brain Res. 2000;126:325-41.
23. Blum K, Mclaughlin T, Gold MS, et al. Are we getting high cause the thrill is gone? J Addict Psychiatry. ;2023:7:5-516.
24. Voon V, Grodin E, Mandali A, et al. Addictions neuroImaging assessment (ANIA): towards an integrative framework for alcohol use disorder. Neurosci Biobehav Rev. 2020;113:492-506.
25. Blum K, Bowirrat A, Baron D. , et al. Identification of stress-induced epigenetic methylation onto dopamine D2 gene and neurological and behavioral consequences. Gene Protein Dis. 2024;3:10.36922/gpd.1966.
27. Gondré-Lewis MC, Bassey R, Blum K. Pre-clinical models of reward deficiency syndrome: a behavioral octopus. Neurosci Biobehav Rev. 2020;115:164-88.
28. Büchel C, Peters J, Banaschewski T, et al. IMAGEN consortium. Blunted ventral striatal responses to anticipated rewards foreshadow problematic drug use in novelty-seeking adolescents. Nat Commun. 2017;8:14140.
29. Wingo T, Nesil T, Choi JS, Li MD. Novelty seeking and drug addiction in humans and animals: from behavior to molecules. J Neuroimmune Pharmacol. 2016;11:456-70.
30. Elman I, Lowen S, Frederick BB, Chi W, Becerra L, Pitman RK. Functional neuroimaging of reward circuitry responsivity to monetary gains and losses in posttraumatic stress disorder. Biol Psychiatry. 2009;66:1083-90.
31. Elman I, Ariely D, Mazar N, et al. Probing reward function in post-traumatic stress disorder with beautiful facial images. Psychiatry Res. 2005;135:179-83.
32. Lee JS, Jung S, Park IH, Kim JJ. Neural basis of anhedonia and amotivation in patients with schizophrenia: the role of reward system. Curr Neuropharmacol. 2015;13:750-9.
33. Lambert C, Da Silva S, Ceniti AK, Rizvi SJ, Foussias G, Kennedy SH. Anhedonia in depression and schizophrenia: a transdiagnostic challenge. CNS Neurosci Ther. 2018;24:615-23.
34. Cooper JA, Arulpragasam AR, Treadway MT. Anhedonia in depression: biological mechanisms and computational models. Curr Opin Behav Sci. 2018;22:128-35.
35. Elman I, Borsook D, Lukas SE. Food intake and reward mechanisms in patients with schizophrenia: implications for metabolic disturbances and treatment with second-generation antipsychotic agents. Neuropsychopharmacology. 2006;31:2091-120.
36. Blum K, Thanos PK, Gold MS. Dopamine and glucose, obesity, and reward deficiency syndrome. Front Psychol. 2014;5:919.
37. Thanos PK, Zhuo J, Robison L, et al. Suboptimal maternal diets alter mu opioid receptor and dopamine type 1 receptor binding but exert no effect on dopamine transporters in the offspring brain. Int J Dev Neurosci. 2018;64:21-8.
38. Gyollai A, Griffiths MD, Barta C, et al. The genetics of problem and pathological gambling: a systematic review. Curr Pharm Des. 2014;20:3993-9.
39. Schmidt C, Morris LS, Kvamme TL, Hall P, Birchard T, Voon V. Compulsive sexual behavior: prefrontal and limbic volume and interactions. Hum Brain Mapp. 2017;38:1182-90.
40. Modestino EJ, Blum K, Dennen CA, et al. Theorizing the role of dopaminergic polymorphic risk alleles with intermittent explosive disorder (IED), violent/aggressive behavior and addiction: justification of genetic addiction risk severity (GARS) testing. J Pers Med. 2022;12:1946.
41. Blum K, Braverman ER, Wu S, et al. Association of polymorphisms of dopamine D2 receptor (DRD2), and dopamine transporter (DAT1) genes with schizoid/avoidant behaviors (SAB). Mol Psychiatry. 1997;2:239-46.
42. Chester DS, DeWall CN, Derefinko KJ, et al. Looking for reward in all the wrong places: dopamine receptor gene polymorphisms indirectly affect aggression through sensation-seeking. Soc Neurosci. 2016;11:487-94.
43. Qayyum A, Zai CC, Hirata Y, et al. The role of the catechol-o-methyltransferase (COMT) geneVal158Met in aggressive behavior, a review of genetic studies. Curr Neuropharmacol. 2015;13:802-14.
44. Elman I, Borsook D, Volkow N. Pain and suicidality: insights from reward and addiction neuroscience. Prog Neurobiol. 2013;109:1-27.
45. Belujon P, Grace AA. Dopamine system dysregulation in major depressive disorders. Int J Neuropsychopharmacol. 2017;20:1036-46.
46. Williams CL, Milanak ME, Judah MR, Berenbaum H. The association between PTSD and facial affect recognition. Psychiatry Res. 2018;265:298-302.
47. Blum K, Bowirrat A, Braverman ER, et al. Reward deficiency syndrome (RDS): a cytoarchitectural common neurobiological trait of all addictions. Int J Environ Res Public Health. 2021;18:11529.
48. Volkow ND, Fowler JS, Wang GJ, Goldstein RZ. Role of dopamine, the frontal cortex and memory circuits in drug addiction: insight from imaging studies. Neurobiol Learn Mem. 2002;78:610-24.
49. Enman NM, Arthur K, Ward SJ, Perrine SA, Unterwald EM. Anhedonia, reduced cocaine reward, and dopamine dysfunction in a rat model of posttraumatic stress disorder. Biol Psychiatry. 2015;78:871-9.
50. Felger JC, Mun J, Kimmel HL, et al. Chronic interferon-α decreases dopamine 2 receptor binding and striatal dopamine release in association with anhedonia-like behavior in nonhuman primates. Neuropsychopharmacology. 2013;38:2179-87.
51. Comings DE, Wu S, Chiu C, et al. Polygenic inheritance of tourette syndrome, stuttering, attention deficit hyperactivity, conduct, and oppositional defiant disorder: The additive and subtractive effect of the three dopaminergic genes-DRD2, DβH, and DAT1. Am J Med Genet. 1996;67:264-88.
52. Gjedde A, Kumakura Y, Cumming P, Linnet J, Møller A. Inverted-U-shaped correlation between dopamine receptor availability in striatum and sensation seeking. Proc Natl Acad Sci U S A. 2010;107:3870-5.
53. Noble EP, Blum K, Ritchie T, Montgomery A, Sheridan PJ. Allelic association of the D2 dopamine receptor gene with receptor-binding characteristics in alcoholism. Arch Gen Psychiatry. 1991;48:648-54.
54. Kuchel O, Racz K, Debinski W, Falardeau P, Buu NT. Contrasting dopaminergic patterns in two forms of genetic hypertension. Clin Exp Hypertens A. 1987;9:987-1008.
55. Camarini R, Marcourakis T, Teodorov E, Yonamine M, Calil HM. Ethanol-induced sensitization depends preferentially on D1 rather than D2 dopamine receptors. Pharmacol Biochem Behav. 2011;98:173-80.
56. Nelson AM, Larson GA, Zahniser NR. Low or high cocaine responding rats differ in striatal extracellular dopamine levels and dopamine transporter number. J Pharmacol Exp Ther. 2009;331:985-97.
57. Hodgkinson CA, Yuan Q, Xu K, et al. Addictions biology: haplotype-based analysis for 130 candidate genes on a single array. Alcohol Alcohol. 2008;43:505-15.
58. Li CY, Mao X, Wei L. Genes and (common) pathways underlying drug addiction. PLoS Comput Biol. 2008;4:e2.
59. Blum K, McLaughlin T, Bowirrat A, et al. Reward deficiency syndrome (RDS) surprisingly is evolutionary and found everywhere: is it “blowin’ in the wind”? J Pers Med. 2022;12:321.
60. Blum K, Han D, Bowirrat A, et al. Genetic addiction risk and psychological profiling analyses for “preaddiction” severity index. J Pers Med. 2022;12:1772.
61. Blum K, Cadet JL, Thanos PK, et al. Neurogenetics of alcohol use disorder a subset of reward deficiency syndrome: candidate genes to be or not to be? In: Tsermpini EE, Alda M, Patrinos GP, editors. Psychiatric genomics. Elsevier Inc.; 2022. p. 105-60.
62. Blum K, Badgaiyan RD, Agan G, et al. Molecular genetic testing in reward deficiency syndrome (RDS): facts and fiction. J Reward Defic Syndr. 2015;1:65-8.
63. Oberlin BG, Dzemidzic M, Bragulat V, et al. Limbic responses to reward cues correlate with antisocial trait density in heavy drinkers. Neuroimage. 2012;60:644-52.
64. Hu G, Jain K, Hurle M. Revealing transforming growth factor-beta signaling transduction in human kidney by gene expression data mining. OMICS. 2005;9:266-80.
65. Stice E, Yokum S, Burger K, Epstein L, Smolen A. Multilocus genetic composite reflecting dopamine signaling capacity predicts reward circuitry responsivity. J Neurosci. 2012;32:10093-100.
66. Blum K, Hauser M, Fratantonio J, Badgaiyan RD. Molecular genetic testing in pain and addiction: facts, fiction and clinical utility. Addict Genet. 2015;2:1-5.
67. Blum K, Oscar-Berman M, Jacobs W, McLaughlin T, Gold MS. Buprenorphine response as a function of neurogenetic polymorphic antecedents: can dopamine genes affect clinical outcomes in reward deficiency syndrome (RDS)? J Addict Res Ther. 2014;5:1000185.
68. Levey DF, Le-Niculescu H, Frank J, et al. GESGA Consortium. Genetic risk prediction and neurobiological understanding of alcoholism. Transl Psychiatry. 2014;4:e391.
69. Pearson-Fuhrhop KM, Dunn EC, Mortero S, et al. Dopamine genetic risk score predicts depressive symptoms in healthy adults and adults with depression. PLoS One. 2014;9:e93772.
70. Gilley ED, Bowirrat A, Gupta A, et al. The future is now for precision genomic addiction medicine as a frontline modality for inducing “dopamine homeostasis” in reward deficiency syndrome (RDS). Curr Pharm Biotechnol. 2024;25:42-57. Available from: https://doi.org/10.2174/1389201024666230427111117. [Last accessed on 17 Mar 2025]
71. Blum K, Bowirrat A, Baron D, et al. Biotechnical development of genetic addiction risk score (GARS) and selective evidence for inclusion of polymorphic allelic risk in substance use disorder (SUD). J Syst Integr Neurosci. 2020;6:10.15761/JSIN.1000221.
72. Gold MS, Blum K, Febo M, et al. Molecular role of dopamine in anhedonia linked to reward deficiency syndrome (RDS) and anti- reward systems. Front Biosci (Schol Ed). 2018;10:309-25.
73. Elman I, D’Ambra N, Krause S, et al. Ultrarapid opioid detoxification: effects on cardiopulmonary physiology, stress hormones and clinical outcomes. Drug Alcohol Depend. 2001;61:163-72.
74. Khantzian EJ. The self-medication hypothesis of substance use disorders: a reconsideration and recent applications. Harv Rev Psychiatry. 1997;4:231-44.
75. Uslaner J, Kalechstein A, Richter T, Ling W, Newton T. Association of depressive symptoms during abstinence with the subjective high produced by cocaine. Am J Psychiatry. 1999;156:1444-6.
76. Tremblay LK, Naranjo CA, Graham SJ, et al. Functional neuroanatomical substrates of altered reward processing in major depressive disorder revealed by a dopaminergic probe. Arch Gen Psychiatry. 2005;62:1228-36.
77. Tremblay LK, Naranjo CA, Cardenas L, Herrmann N, Busto UE. Probing brain reward system function in major depressive disorder: altered response to dextroamphetamine. Arch Gen Psychiatry. 2002;59:409-16.
78. Elman I, Karlsgodt KH, Gastfriend DR, Chabris CF, Breiter HC. Cocaine-primed craving and its relationship to depressive symptomatology in individuals with cocaine dependence. Psychopharmacol. 2002;16:163-7.
79. Berridge KC. The debate over dopamine’s role in reward: the case for incentive salience. Psychopharmacology. 2007;191:391-431.
80. Butler SF, Redondo JP, Fernandez KC, Villapiano A. Validation of the spanish addiction severity index multimedia version (S-ASI-MV). Drug Alcohol Depend. 2009;99:18-27.
81. Sobell LC, Kwan E, Sobell MB. Reliability of a drug history questionnaire (DHQ). Addict Behav. 1995;20:233-41.
82. Strantz IH, Welch SP. Postpartum women in outpatient drug abuse treatment: correlates of retention/completion. J Psychoactive Drugs. 1995;27:357-73.
83. Haberstick BC, Smolen A, Stetler GL, et al. Simple sequence repeats in the national longitudinal study of adolescent health: an ethnically diverse resource for genetic analysis of health and behavior. Behav Genet. 2014;44:487-97.
84. Mutirangura A, Kuwano A, Ledbetter SA, Chinault AC, Ledbetter DH. Dinucleotide repeat polymorphism at the D15S11 locus in the angelman/prader-willi region (AS/PWS) of chromosome 15. Hum Mol Genet. 1992;1:139.
85. Anchordoquy HC, McGeary C, Liu L, Krauter KS, Smolen A. Genotyping of three candidate genes after whole-genome preamplification of DNA collected from buccal cells. Behav Genet. 2003;33:73-8.
86. Don J, Wolgemuth DJ. Identification and characterization of the regulated pattern of expression of a novel mouse gene, meg1, during the meiotic cell cycle. Cell Growth Differ. 1992;3:495-505.
87. Wendland JR, Martin BJ, Kruse MR, Lesch KP, Murphy DL. Simultaneous genotyping of four functional loci of human SLC6A4, with a reappraisal of 5-HTTLPR and rs25531. Mol Psychiatry. 2006;11:224-6.
88. Haberstick BC, Smolen A. Genotyping of three single nucleotide polymorphisms following whole genome preamplification of DNA collected from buccal cells. Behav Genet. 2004;34:541-7.
89. Lee LG, Livak KJ, Mullah B, Graham RJ, Vinayak RS, Woudenberg TM. Seven-color, homogeneous detection of six PCR products. Biotechniques. 1999;27:342-9.
90. Livák F, Tourigny M, Schatz DG, Petrie HT. Characterization of TCR gene rearrangements during adult murine T cell development. J Immunol. 1999;162:2575-80.
91. Chen B, Cole JW, Grond-Ginsbach C. Departure from hardy weinberg equilibrium and genotyping error. Front Genet. 2017;8:167.
92. Yokum S, Marti CN, Smolen A, Stice E. Relation of the multilocus genetic composite reflecting high dopamine signaling capacity to future increases in BMI. Appetite. 2015;87:38-45.
93. Conner BT, Hellemann GS, Ritchie TL, Noble EP. Genetic, personality, and environmental predictors of drug use in adolescents. J Subst Abuse Treat. 2010;38:178-90.
94. Ishibashi K, Ishii K, Oda K, Kawasaki K, Mizusawa H, Ishiwata K. Regional analysis of age-related decline in dopamine transporters and dopamine D2-like receptors in human striatum. Synapse. 2009;63:282-90.
95. Bäckman L, Nyberg L, Lindenberger U, Li SC, Farde L. The correlative triad among aging, dopamine, and cognition: current status and future prospects. Neurosci Biobehav Rev. 2006;30:791-807.
96. Trifilieff P, Martinez D. Imaging addiction: D2 receptors and dopamine signaling in the striatum as biomarkers for impulsivity. Neuropharmacology. 2014;76:498-509.
97. Blum K, Braverman ER, Holder JM, et al. The reward deficiency syndrome: a biogenetic model for the diagnosis and treatment of impulsive, addictive and compulsive behaviors. J Psychoactive Drugs. 2000;32:1-112.
98. Li SC, Papenberg G, Nagel IE, et al. Aging magnifies the effects of dopamine transporter and D2 receptor genes on backward serial memory. Neurobiol Aging. 2013;34:358.e1-10.
99. Blum K, Noble EP, Sheridan PJ, et al. Allelic association of human dopamine D2 receptor gene in alcoholism. JAMA. 1990;263:2055-60.
100. Blum K, Modestino EJ, Badgaiyan RD, et al. Analysis of evidence for the combination of pro-dopamine regulator (KB220PAM) and naltrexone to prevent opioid use disorder relapse. EC Psychol Psychiatry. 2018;7:564-79.
101. Xu K, Lichtermann D, Lipsky RH, et al. Association of specific haplotypes of D2 dopamine receptor gene with vulnerability to heroin dependence in 2 distinct populations. Arch Gen Psychiatry. 2004;61:597-606.
102. Shapira B, Rosca P, Berkovitz R, Gorjaltsan I, Neumark Y. The switch from one substance-of-abuse to another: illicit drug substitution behaviors in a sample of high-risk drug users. PeerJ. 2020;8:e9461.
103. Elman I, Pustilnik A, Borsook D. Beating pain with psychedelics: matter over mind? Neurosci Biobehav Rev. 2022;134:104482.
104. Kótyuk E, Urbán R, Hende B, et al. Development and validation of the reward deficiency syndrome questionnaire (RDSQ-29). J Psychopharmacol. 2022;36:409-22.
105. Admon R, Holsen LM, Aizley H, et al. Striatal hypersensitivity during stress in remitted individuals with recurrent depression. Biol Psychiatry. 2015;78:67-76.
106. Elman I, Upadhyay J, Langleben DD, Albanese M, Becerra L, Borsook D. Reward and aversion processing in patients with post-traumatic stress disorder: functional neuroimaging with visual and thermal stimuli. Transl Psychiatry. 2018;8:240.
107. Kim YJ, Kook WA, Ma SX. , et al. The novel psychoactive substance 25E-NBOMe induces reward-related behaviors via dopamine D1 receptor signaling in male rodents. Arch Pharm Res. 2024;47:360-76.
108. Weissman MM, Wickramaratne P, Greenwald S. The changing rate of major depression. JAMA. 1992;268:3098-105.
109. Black RA, Trudeau KJ, Cassidy TA, Budman SH, Butler SF. Associations between public health indicators and injecting prescription opioids by prescription opioid abusers in substance abuse treatment. J Opioid Manag. 2013;9:5-17.
110. Bondon-Guitton E, Despas F, Becquemont L. The contribution of pharmacogenetics to pharmacovigilance. Therapie. 2016;71:223-8.
111. Talukdar S, Owen BM, Song P, et al. FGF21 regulates sweet and alcohol preference. Cell Metab. 2016;23:344-9.
112. Blum K, Soni D, Badgaiyan RD, Baron D. Overcoming reward deficiency syndrome by the induction of “dopamine homeostasis” instead of opioids for addiction: illusion or reality? J Osteopath Med. 2022;122:333-7.
113. Blum K, Gondré-Lewis MC, Baron D, et al. Introducing precision addiction management of reward deficiency syndrome, the construct that underpins all addictive behaviors. Front Psychiatry. 2018;9:548.
114. Blum K, Chen AL, Braverman ER, et al. Attention-deficit-hyperactivity disorder and reward deficiency syndrome. Neuropsychiatr Dis Treat. 2008;4:893-918.
115. Ivanov I, Bjork JM, Blair J, Newcorn JH. Sensitization-based risk for substance abuse in vulnerable individuals with ADHD: review and re-examination of evidence. Neurosci Biobehav Rev. 2022;135:104575.
116. Blum K, Bowirrat A, Thanos PK, et al. Evidence based clinical analytics supporting the genetic addiction risk severity (GARS) assessment to early identify probands in preaddiction. EC Psychol Psychiatry. 2024;13:1-3.
117. An X, Zhang Y, Sun W, et al. Early effective intervention can significantly reduce all-cause mortality in prediabetic patients: a systematic review and meta-analysis based on high-quality clinical studies. Front Endocrinol. 2024;15:1294819.
118. Prevention Program Research Group. 10-year follow-up of diabetes incidence and weight loss in the diabetes prevention program outcomes study. Lancet. 2009;374:1677-86.
119. Blum K, Elman I, Dennen CA, et al. “Preaddiction” construct and reward deficiency syndrome: genetic link via dopaminergic dysregulation. Ann Transl Med. 2022;10:1181.
120. Samek DR, Bailey J, Hill KG, et al. A test-replicate approach to candidate gene research on addiction and externalizing disorders: a collaboration across five longitudinal studies. Behav Genet. 2016;46:608-26.
121. Bjork JM, Smith AR, Chen G, Hommer DW. Mesolimbic recruitment by nondrug rewards in detoxified alcoholics: effort anticipation, reward anticipation, and reward delivery. Hum Brain Mapp. 2012;33:2174-88.
122. Blum K, Han D, Gupta A, et al. Statistical validation of risk alleles in genetic addiction risk severity (GARS) test: early identification of risk for alcohol use disorder (AUD) in 74,566 case-control subjects. J Pers Med. 2022;12:1385.
123. Blum K, Baron D, Lott L, et al. In search of reward deficiency syndrome (RDS)-free controls: the “holy grail” in genetic addiction risk testing. Curr Psychopharmacol. 2020;9:7-21.
124. Blum K, Brodie MS, Pandey SC, et al. Researching mitigation of alcohol binge drinking in polydrug abuse: KCNK13 and RASGRF2 gene(s) risk polymorphisms coupled with genetic addiction risk severity (GARS) guiding precision pro-dopamine regulation. J Pers Med. 2022;12:1009.
125. Gupta A, Bowirrat A, Gomez LL, et al. Hypothesizing in the face of the opioid crisis coupling genetic addiction risk severity (GARS) testing with electrotherapeutic nonopioid modalities such as H-Wave could attenuate both pain and hedonic addictive behaviors. Int J Environ Res Public Health. 2022;19:552.
126. Blum K, Chen ALC, Thanos PK, et al. Genetic addiction risk score (GARS)TM, a predictor of vulnerability to opioid dependence. Front Biosci (Elite Ed). 2018;10:175-96.
127. Moran M, Blum K, Ponce JV, et al. High genetic addiction risk score (GARS) in chronically prescribed severe chronic opioid probands attending multi-pain clinics: an open clinical pilot trial. Mol Neurobiol. 2021;58:3335-46.
128. Vereczkei A, Barta C, Magi A, et al. FOXN3 and GDNF polymorphisms as common genetic factors of substance use and addictive behaviors. J Pers Med. 2022;12:690.
129. Blum K, Steinberg B, Gondre-Lewis MC, et al. A review of DNA risk alleles to determine epigenetic repair of mRNA expression to prove therapeutic effectiveness in reward deficiency syndrome (RDS): embracing “precision behavioral management”. Psychol Res Behav Manag. 2021;14:2115-34.
130. Fried L, Modestino EJ, Siwicki D, et al. Hypodopaminergia and “precision behavioral management” (PBM): it is a generational family affair. Curr Pharm Biotechnol. 2020;21:528-41.
131. Thanos PK, Hanna C, Mihalkovic A, et al. Genetic correlates as a predictor of bariatric surgery outcomes after 1 year. Biomedicines. 2023;11:2644.
132. Blum K, Bowirrat A, Gondre Lewis MC, et al. Exploration of epigenetic state hyperdopaminergia (surfeit) and genetic trait hypodopaminergia (deficit) during adolescent brain development. Curr Psychopharmacol. 2021;10:181-96.
133. Dennen CA, Blum K, Bowirrat A, et al. Genetic addiction risk severity assessment identifies polymorphic reward genes as antecedents to reward deficiency syndrome (RDS) hypodopaminergia’s effect on addictive and non-addictive behaviors in a nuclear family. J Pers Med. 2022;12:1864.
134. Blum K, Baron D, McLaughlin T, et al. Summary document research on RDS anti-addiction modeling: annotated bibliography. J Addict Psychiatry. 2024;8:1-33.
135. Bajaj A, Blum K, Bowirrat A, et al. DNA directed pro-dopamine regulation coupling subluxation repair, H-Wave® and other neurobiologically based modalities to address complexities of chronic pain in a female diagnosed with reward deficiency syndrome (RDS): emergence of induction of “dopamine homeostasis” in the face of the opioid crisis. J Pers Med. 2022;12:1416.
136. Thanos PK, Hanna C, Mihalkovic A, et al. The first exploratory personalized medicine approach to improve bariatric surgery outcomes utilizing psychosocial and genetic risk assessments: encouraging clinical research. J Pers Med. 2023;13:1164.
137. Gondré-Lewis MC, Elman I, Alim T, et al. Frequency of the dopamine receptor D3 (rs6280) vs. opioid receptor µ1 (rs1799971) polymorphic risk alleles in patients with opioid use disorder: a preponderance of dopaminergic mechanisms? Biomedicines. 2022;10:870.
138. Blum K, Oscar-Berman M, Femino J, et al. Withdrawal from buprenorphine/naloxone and maintenance with a natural dopaminergic agonist: a cautionary note. J Addict Res Ther. 2013:4.
139. Blum K, Gold MS, Cadet JL, et al. Invited expert opinion- Bio-informatic and limitation Di-rectives to help adopt Ge-netic addiction risk screening and identify preaddictive reward dysregulation: Re-quired analytic evidence to induce dopamine homeostatsis. Med Res Arch. 2023:11.
140. Blum K, Braverman ER, Gold MS, et al. Addressing cortex dysregulation in youth through brain health check coaching and prophylactic brain development. INNOSC Theranostics Pharmacol Sci. 2024;7:1472.
141. Blum K, Badgaiyan RD, Agan G, et al. Response to the SAMHSA clinical advisory: considerations for genetic testing in the assessment of substance use disorder risk. Subst Abuse Rehabil. 2025;16:23-6.
142. Lewandrowski KU, Sharafshah A, Elfar J, Schmidt SL, Blum K, Wetzel FT. A pharmacogenomics-based in silico investigation of opioid prescribing in post-operative spine pain management and personalized therapy. Cell Mol Neurobiol. 2024;44:47.
143. Blum K, Green R, Mullen P, et al. Reward deficiency syndrome solution system (RDSSS) a genetically driven putative inducer of “dopamine homeostasis” as a futuristic alternative to enhance rehabilitation instead of incarceration. Asian J Complement Altern Med. 2023;11:11-4.
Cite This Article

How to Cite
Download Citation
Export Citation File:
Type of Import
Tips on Downloading Citation
Citation Manager File Format
Type of Import
Direct Import: When the Direct Import option is selected (the default state), a dialogue box will give you the option to Save or Open the downloaded citation data. Choosing Open will either launch your citation manager or give you a choice of applications with which to use the metadata. The Save option saves the file locally for later use.
Indirect Import: When the Indirect Import option is selected, the metadata is displayed and may be copied and pasted as needed.
About This Article
Copyright
Data & Comments
Data
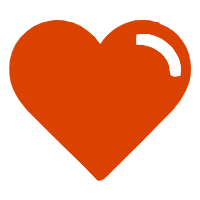
Comments
Comments must be written in English. Spam, offensive content, impersonation, and private information will not be permitted. If any comment is reported and identified as inappropriate content by OAE staff, the comment will be removed without notice. If you have any queries or need any help, please contact us at [email protected].