An entropy - based fuzzy QFD model on used product remanufacturing design
Abstract
Compared with new products, consumers put forward higher requirements on remanufactured products. Aimed at effectively transforming customer requirements (CRs) to technical characteristics (TCs) of remanufactured product design, an entropy-based fuzzy quality function deployment (QFD) model is proposed. In this model, triangular fuzzy numbers are suggested to express the vagueness information of CRs first, and then the importance of CRs is determined by an entropy-based algorithm, and the relationship between CRs and TCs is well explained by fuzzy QFD; finally, the importance of TCs is determined to clarify the designing emphasis. A remanufacturing engine is conducted to illuminate the practicability of this model. The results show that “Restorability” and “Reliability” will be treated as the key TCs to control, with the importance degree of 0.16 and 0.15. The entropy-based fuzzy QFD model can also be applied to similar product designs.
Keywords
INTRODUCTION
Used product remanufacturing can help reduce energy consumption, resource consumption, and environmental pollution[1,2]. Nowadays, the remanufacturing industry processes many types of products, such as automobile engines, construction machinery, and industrial machinery[3-6]. However, because returned products are used as blanks in remanufacturing, the various failure modes and degrees of failure of such products may lead to dynamic uncertainties and variations in quality levels[7,8]. Unlike traditional manufacturing, a general remanufacturing process comprises the following steps: disassembly, inspection, cleaning, testing, repair, and assembly[9]. Moreover, owing to the distinct remanufacturing processes with new manufacturing, diverse technical characteristics (TCs) may exist[10,11]. Research has indicated that design decisions may greatly influence the efficiency and effectiveness of product remanufacturing, and technical design characteristics need to be considered in remanufacturing used products[12].
Many studies have explored used product remanufacturing design. Ke et al. proposed an integrated design method for remanufacturing scheme based on performance demand[13]; Shahbazi et al. investigated how circular product design can facilitate automated remanufacturing processes by conducting multiple case studies[14]; Boorsma et al. explored how design facilitates the implementation of remanufacturing[15]. Considering the problem that many components of remanufactured products will have been subjected to regular stresses in their first cycle of use, Tant et al. pointed out that the integrity of individual components should be assessed before consigning them to storage[16]; Wang et al. applied stylized models to a monopoly original equipment manufacturer (OEM) and suggested four legislative scenarios to analyze the fundamental conflicts of interest associated with remanufacturing and product design[17]; Ke et al. proposed an integrated design method for remanufacturing by considering carbon emissions[18]. Similarly, Dey et al. used analytical approaches to derive closed-loop solutions for complex nonlinear profit functions while accounting for the effects of carbon policies and multi-period delays in payments in the context of product remanufacturing[19]. The research contributions for remanufacturing design are shown in Table 1.
Contributions for remanufacturing design
No. | Methods or viewpoint | Authors |
1 | Design decisions may greatly influence the efficiency and effectiveness of product remanufacturing | Zhang et al. (2019)[12] |
2 | Integrated design method for remanufacturing scheme | Ke et al. (2022)[13] |
3 | How circular product design can facilitate automated remanufacturing processes | Shahbazi et al. (2022)[14] |
4 | How design facilitates remanufacturing implementation | Boorsma et al. (2020)[15] |
5 | Integrity of individual components should be assessed | Tant et al. (2019)[16] |
6 | Fundamental conflicts of remanufacturing and product design | Wang et al. (2022)[17] |
7 | Remanufacturing design method considering carbon emissions | Ke et al. (2023)[18] |
10 | Carbon policies and multi-period delays in payments in the context of product remanufacturing | Dey et al. (2023)[19] |
The above studies investigated different aspects of remanufacturing design; however, there remains a research gap: (1) they did not consider customer requirements (CRs) for remanufactured products and the role of TCs of remanufacturing in satisfying these CRs; (2) Remanufacturing design priorities cannot be determined to develop remanufacturing process planning.
With elevated customer expectations regarding the security, reliability, and quality of remanufactured products, some complex relationships must exist between the CRs and TCs[20]. In this regard, accurately defining, decomposing, and converting the CRs to TCs is a new challenge in used product remanufacturing design. However, to date, no study has focused on solving this issue.
Quality function deployment (QFD) is a quality assurance method that involves carefully considering CRs and striving to meet them, and it is widely used in product design and development[21-23]. In recent years, the fuzzy set theory has been applied extensively to multi-criteria decision-making (MCDM)[24-26], and fuzzy QFD has been widely employed as a quality tool to satisfy CRs in product design and development[27-30]. The key step in implementing QFD is converting CRs to product TCs. For remanufactured products, the CRs are often more elevated than those for new products, and customers focus more on product performance, safety, and environmental protection. However, because these requirements are based mostly on artificial judgments and evaluations, they are not expressed exactly, and a fuzzy evaluation is required to incorporate them into the QFD method.
Entropy is an objective method that finds widespread use in MCDM as a weighting approach to ensure that weights are objective[31-34]. The technique considers the relationship between indicators and evaluates their importance, thereby eliminating the need for subjective judgment or expert experience to determine weights[35,36]. The CRs associated with remanufacturing design are complex, and it is difficult to determine their degrees of importance objectively. In this case, the entropy weighting method is suitable.
This study incorporates the concept of entropy into fuzzy QFD to determine the importance of CRs and transform them into TCs of remanufactured products to accurately determine the importance of the TCs. This process is expected to help clarify the design emphasis of product remanufacturing. Although the entropy and fuzzy QFD methods have been widely applied, this study presents an innovation as it is the first exploration of their use in product remanufacturing design to consider CRs and TCs by the methods. The proposed model does not involve complex operations or rely on huge theoretical foundations, but the resulting process is complete and universal, and general technical personnel can quickly understand and apply it, which may be an advantage of the proposed approach.
METHODS
House of quality
House of quality (HoQ), as the important tool of QFD, formally articulates how a company sees the relationship between the CRs and the product design characteristic[37] [Figure 1].
The general principle of HoQ is to identify “whats” and then relate “whats” to “hows”. Its details may vary between its variants. As suggested by Hauser and Clausing[38], the HoQ can be built through the following process.
Step 1: Identifying ‘‘whats”. ‘‘whats” are CRs expressed by the customer’s own words.
Step 2: Identifying ‘‘hows”. ‘‘hows” are TCs of product and affect one or more ‘‘whats”, which are listed in the columns of HoQ.
Step 3: Weighting each ‘‘what”. ‘‘what” is listed in a column in the matrix.
Step 4: Determining the relationship matrix of ‘‘whats” and ‘‘hows”. This is the core element of the matrix designated.
Step 5: Determining the importance of the ‘‘Hows’’. The weights of the ‘‘hows” are placed at the bottom of HoQ.
Abbreviations and notations
The abbreviations and notations used in this study are listed in Table 2.
Abbreviations and notations
No. | Notations | Implication | No. | Notations | Implication |
1 | QFD | Quality function deployment | 11 | cml | Ability to achieve the CRs for the lth company |
2 | HoQ | House of quality | 12 | bmx | Importance of the mth CR for the xth customer |
3 | CRs | Customer requirements | 13 | km | Importance of the mth CR |
4 | TCs | Technical characteristics | 14 | bm | Average relative importance of the mth CR |
5 | TFN | Triangular fuzzy number | 15 | cm | Achievement ability for the mth CR |
6 | AHP | Analytic hierarchy process | 16 | am | Target capability to meet the mth CRs |
7 | Xi | The ith customers | 17 | em | Priority level |
8 | Km | The mth types of CRs | 18 | um | Improvement ability |
9 | Hi | The ith types of TCs | 19 | rmn | TFN relations between mth CR and nth TC |
10 | Ci | The ith remanufacturing company | 20 | hn | Importance of the nth TC |
Determining the importance of customer requirements
Assuming X customers and M types of requirements exist, expressed as Km (m = 1, 2, ..., M), the first step in this study is to determine the importance of CRs. Assuming L companies are involved in remanufacturing a product, and one of them is our remanufacturing company identified as CI, and the L-1 competitors are denoted as C1, C2, …, CL-1. Then, the steps for determining the importance of the CRs are as follows:
(1) Assume that bmx is the importance of the mth CR for the xth customer, expressed as
Then, the average relative importance bm of the mth CR is given as
(2) The degree of satisfaction of the CRs for the L companies can be expressed as follows:
(3) Assuming cml is the ability of the lth company to achieve the CRs, the ability of all the competing companies to achieve the mth CR cm is expressed as
(4) We introduce the entropy weight theory, where the intermediate variables gml and E(KM) are expressed in Equations (5) and (6), respectively, and the priority level of achievement em of km is expressed in Equation (7).
(5) Let us assume that am is the target capability of company CI to meet the CRs. Then, the ability of remanufacturing company CI to improve these CRs is expressed as
(6) Based on the average relative importance bm, priority level em, and improvement ability um, the importance of the mth CR can be determined using
Relationship between customer requirements and technical characteristics
Assume that the products to be remanufactured have N TCs. Then, the correlation matrix between the CRs and TCs is as
The value of rmn can be referenced from Table 1, and the importance of the nth TC is given as
Determining triangular fuzzy number
In this paper, the triangular fuzzy number (TFN) is used to quantify linguistic data for reducing the influence of subjectivity and uncertainty and improving the reliability of decision-making[39]. The relevant linguistic scales are presented in Table 3.
Crisp and fuzzy AHP scales
AHP scale | TFN | Linguistic scales |
1 | (1,1,1) If diagonal | Equal importance |
(1,1,2) Otherwise | ||
2 | (1,2,3) | Important |
3 | (2,3,4) | Moderately important |
4 | (3,4,5) | Intermediate |
5 | (4,5,6) | Strongly important |
6 | (5,6,7) | Intermediate |
7 | (6,7,8) | Very strongly important |
8 | (7,8,9) | Intermediate |
9 | (8,9,10) | Extremely important |
In this study, the non-fuzzy method presented by Xiao and Li is used for TFN defuzzification[40]. The associated calculation is given in
where φ(x) is the clear number (CN) of xi; l, m, and n comprise the TFN; l and u represent the lower and upper bounds of the TFN, respectively; m is the median value of the TFN. M and N are determined using l, m, and u, which indicates that the possibility of m may be M times of u and N times of l.
RESULTS AND DISCUSSION
Case study
The WD615 Steyr engine is extensively employed in long-distance transportation vehicles and heavy-duty trucks in China, and approximately 80% of its components can be reused and remanufactured for functional recovery[41]. However, in the process of remanufacturing design, CRs are often ignored and are not translated into remanufacturing TCs. Therefore, a method that fully considers the CRs and emphasizes the design of the engine remanufacturing process is needed.
Importance of customer requirements
There are four types of WD615 engine customers: X1 denotes heavy-duty truck customers, X2 represents engineering machinery customers, X3 symbolizes mining machinery customers, and X4 stands for long-distance transportation machinery customers. The CRs are divided into eight categories, as summarized in Table 4.
Customer requirements description
K1 | K2 | K3 | K4 | K5 | K6 | K7 | K8 |
Reliable use | Perfect function | Energy conservation | Environmental protection | Low failure rate | Cheap price | Long life | Low noise |
By conducting a long-term follow-up survey of these WD615 engine customers and using Equations (1) and (2), the TFNs of the relative importance of CRs were determined, as listed in Table 5.
Relative importance of CRs by TFNs
CR | bm1 | bm2 | bm3 | bm4 | TFN of bm |
K1 | (6,7,8) | (4,5,6) | (4,5,6) | (5,6,7) | (4.75,5.25,6.75) |
K2 | (3,4,5) | (4,5,6) | (5,6,7) | (7,8,9) | (4.75,5.75, 6.75) |
K3 | (4,5,6) | (2,3,4) | (1,2,3) | (5,6,7) | (3,4,5) |
K4 | (6,7,8) | (1,1,2) | (4,5,6) | (4,5,6) | (3.75,4.5,5.5) |
K5 | (4,5,6) | (7,8,9) | (8,9,10) | (6,7,8) | (6.25,7.25,8.25) |
K6 | (5,6,7) | (3,4,5) | (6,7,8) | (3,4,5) | (4.25,5.25,6.25) |
K7 | (2,3,4) | (6,7,8) | (1,2,3) | (5,6,7) | (3.25,4.5,5.5) |
K8 | (1,2,3) | (3,4,5) | (6,7,8) | (4,5,6) | (3.5,4.75,6) |
The corresponding clear numbers bm of CRs importance were obtained using Equation (12), and the results are presented in Table 6.
Clear number bm of relative importance for CRs
CR | K1 | K2 | K3 | K4 | K5 | K6 | K7 | K8 |
bm | 5.55 | 5.79 | 4.06 | 4.60 | 7.28 | 5.30 | 4.51 | 4.83 |
Currently, competition in the engine remanufacturing industry in China is extremely fierce, and it is important to learn about products of competitors during the design and development of engine remanufacturing processes. Let us assume that the competitors of company C1 are companies C2 and C3. Then, using Equation (3), the degrees to which different companies satisfy the relevant CRs were computed, and the results are listed in Table 7.
Satisfied degrees of companies for CRs
CR | X1 | X2 | X3 | X4 | ||||||||
C1 | C2 | C3 | C1 | C2 | C3 | C1 | C2 | C3 | C1 | C2 | C3 | |
K1 | (4,5,6) | (2,3,4) | (4,5,6) | (2,3,4) | (4,5,6) | (4,5,6) | (4,5,6) | (2,3,4) | (6,7,8) | (4,5,6) | (4,5,6) | (6,7,8) |
K2 | (8,9,10) | (4,5,6) | (6,7,8) | (4,5,6) | (2,3,4) | (6,7,8) | (6,7,8) | (4,5,6) | (4,5,6) | (6,7,8) | (2,3,4) | (2,3,4) |
K3 | (6,7,8) | (8,9,10) | (4,5,6) | (6,7,8) | (6,7,8) | (2,3,4) | (4,5,6) | (6,7,8) | (2,3,4) | (4,5,6) | (8,9,10) | (4,5,6) |
K4 | (2,3,4) | (2,3,4) | (4,5,6) | (2,3,4) | (2,3,4) | (1,1,2) | (4,5,6) | (2,3,4) | (2,3,4) | (2,3,4) | (1,1,2) | (4,5,6) |
K5 | (6,7,8) | (1,1,1) | (2,3,4) | (2,3,4) | (4,5,6) | (2,3,4) | (1,1,1) | (1,1,1) | (6,7,8) | (4,5,6) | (2,3,4) | (2,3,4) |
K6 | (1,1,2) | (1,1,1) | (2,3,4) | (6,7,8) | (2,3,4) | (6,7,8) | (2,3,4) | (2,3,4) | (1,1,2) | (1,1,2) | (4,5,6) | (4,5,6) |
K7 | (6,7,8) | (8,9,10) | (2,3,4) | (4,5,6) | (8,9,10) | (4,5,6) | (4,5,6) | (4,5,6) | (2,3,4) | (4,5,6) | (6,7,8) | (4,5,6) |
K8 | (4,5,6) | (4,5,6) | (6,7,8) | (4,5,6) | (2,3,4) | (4,5,6) | (6,7,8) | (1,1,1) | (2,3,4) | (6,7,8) | (4,5,6) | (4,5,6) |
The corresponding clear numbers of competitive ability for each company were computed using Equation (12), and the results are presented in Table 8. Using Equations (4)-(8), the priority level em and the improvement ability um of company C1 were obtained, and the results are listed in Table 8. The em and um of companies C2 and C3 were determined similarly.
Competitive ability, priority level and improvement ability
CR | Clear number of competitive ability | em | bm | um | ||
C1 | C2 | C3 | ||||
K1 | 4.56 | 4.06 | 6.04 | 0.1259 | 7 | 1.53 |
K2 | 7.04 | 4.06 | 5.55 | 0.1247 | 9 | 1.27 |
K3 | 6.04 | 8.03 | 4.06 | 0.1232 | 9 | 1.49 |
K4 | 3.49 | 2.42 | 3.62 | 0.1258 | 5 | 1.43 |
K5 | 4.06 | 2.53 | 4.06 | 0.125 | 7 | 1.72 |
K6 | 3.17 | 3.05 | 4.06 | 0.1265 | 5 | 1.57 |
K7 | 5.55 | 7.53 | 4.06 | 0.1239 | 9 | 1.62 |
K8 | 6.04 | 3.54 | 4.96 | 0.1249 | 7 | 1.16 |
The ultimate relative importance values km of the CRs were obtained using Equation (9), and they are listed in Table 9, along with the corresponding normalized importance values k’m.
Ultimate relative importance of CRs
TC | K1 | K2 | K3 | K4 | K5 | K6 | K7 | K8 |
km | 1.07 | 0.92 | 0.75 | 0.83 | 1.57 | 1.05 | 0.9 | 0.7 |
k’m | 0.14 | 0.12 | 0.09 | 0.11 | 0.2 | 0.13 | 0.12 | 0.09 |
Relationship between CRs and TCs
The key factor in QFD implementation is converting CRs to specific TCs. Based on an investigation conducted by an expert team, the WD615.65 engine has eight TCs, H1-H8, listed in Table 10.
Technical characteristic description of remanufacturing engine
H1 | H2 | H3 | H4 | H5 | H6 | H7 | H8 |
Fuel economy | Fuel Applicability | Restorability | Cleavability | Dynamic performance | Reliability | Replaceability | Assimilability |
The relationship between CRs and TCs are identified by a multidisciplinary team, expressed by rmn (m = 1, 2, ..., 8; n = 1, 2, ..., 8). Using Equation (10), the relationship matrix is listed in Table 11.
Relation matrix of CRs and TCs
![]() | H1 | H2 | H3 | H4 | H5 | H6 | H7 | H8 |
r11-r18 | r21-r28 | r31-r38 | r41-r48 | r51-r58 | r61-r68 | r71-r78 | r81-r88 | |
K1 | (2,3,4) | (2,3,4) | (4,5,6) | (6,7,8) | (4,5,6) | (2,3,4) | (5,6,7) | (4,5,6) |
K2 | (3,4,5) | (4,5,6) | (8,9,10) | (5,6,7) | (2,3,4) | (4,5,6) | (6,7,8) | (4,5,6) |
K3 | (4,5,6) | (4,5,6) | (8,9,10) | (6,7,8) | (2,3,4) | (6,7,8) | (4,5,6) | (2,3,4) |
K4 | (4,5,6) | (2,3,4) | (1,2,3) | (1,1,1) | (2,3,4) | (3,4,5) | (2,3,4) | (3,4,5) |
K5 | (2,3,4) | (1,2,3) | (6,7,8) | (2,3,4) | (4,5,6) | (2,3,4) | (6,7,8) | (1,1,1) |
K6 | (2,3,4) | (1,1,2) | (1,1,1) | (6,7,8) | (2,3,4) | (6,7,8) | (1,1,2) | (2,3,4) |
K7 | (4,5,6) | (5,6,7) | (8,9,10) | (4,5,6) | (4,5,6) | (8,9,10) | (4,5,6) | (2,3,4) |
K8 | (6,7,8) | (4,5,6) | (4,5,6) | (3,4,5) | (2,3,4) | (4,5,6) | (2,3,4) | (1,1,1) |
Importance of technical characteristics
The relative importance of the TCs and the clear number of rij can be determined using Equations (11) and (12), respectively, and the results are listed in the HoQ [Figure 2]. According to these results, the importance order of the TCs is as follows: H3(0.16) >H6(0.15) > H4(0.14) > H7(0.13) > H1(0.12) > H5(0.11) >
The result illustrated that “Restorability” and “Reliability” will be treated as the key TCs for engine remanufacturing design to control, and “Fuel Applicability” and “Assimilability” are the least important TCs; nevertheless, in the remanufacturing designing, these two TCs should not be neglected. In fact, through the model process, we can see that, the importance order of TCs highly relies on the relationship expressed by TFNs between CRs and TCs; therefore, accurately determining the TFNs is important. Additionally, the weighs of CRs should be considered.
Discussion and conclusion
This study presented an entropy-based fuzzy QFD model for the remanufacturing design by fully considering the product CRs and TCs. With this model, the CRs were identified, and their importance values were determined using the entropy weighting method. Then, TFNs were used to express the relationships between the CRs and TCs to help designers fully consider the CRs in product remanufacturing. Finally, the importance of the TCs was determined using the fuzzy QFD method. This strategy can connect CRs with remanufacturing design naturally and helps strengthen effective communication and collaboration among remanufacturing implementers.
The WD 615.65 engine was considered as a case study to illustrate the practical applicability of the proposed model. The results showed that the entropy-based fuzzy QFD model was well applicable. The case study considered herein may be simple and seem insufficient to verify the effectiveness and advantages of the method. However, it only demonstrates the process of the proposed model to provide an understanding of how to apply the model. In the future, we will develop a more rigorous case study involving complex products to verify the proposed model. Moreover, the results of the method rely on the data largely; therefore, the credibility of the survey data is important. To ensure the credibility of the survey data of the engine customers, it is recommended that more customers be surveyed and efforts be made to strive for the maximum possible samples.
Compared with similar research, the contribution of this study is summarized as four points: (1) It provided an effective method that can transform CRs to TCs in the remanufactured product design by fully considering the factors of achievement ability of CRs, the average importance of CRs, and the improvement ability of the companies; (2) It offered a good communication tool among the participants of remanufacturing engineering and helps to realize the collaborative management of remanufacturing objectives and design processes; (3) Through the guidelines of the importance of TCs, engineers can clarify the design focus for the used engine remanufacturing, such as developing optimal process routes and procedures for the high “restorability” and “reliability”, applying more appropriate repairing materials for the high “Cleavability”, and so on; (4) This method can not only be used in designing product remanufacturing processes but also applied to the design of new products. In the latter case, the CRs and TCs should be considered from the perspective of a new product.
While this study clarifies the design emphasis by analyzing the relationships between CRs and TCs, it does not describe remanufacturing process planning, which is reserved for a future study. On the other hand, future work can focus on developing computation software procedures and compiling pseudocode of the algorithm by which users can freely define the CRs and TCs and conveniently determine the designing emphasis.
DECLARATIONS
Acknowledgments
The authors gratefully acknowledge the support of Jinan Fuqiang Power Co., LTD and the 2023 Liaoning Province Education Department Project (No. JYTMS20230430). They would also like to thank the editor and reviewers for their constructive suggestions.
Authors’ contributions
Made substantial contributions to the conception and design of the study and performed data analysis and interpretation: Shi J, Yu Z, Li M
Performed data acquisition and provided administrative, technical, and material support: Pan Y, Zhu H
Availability of data and materials
Not applicable.
Financial support and sponsorship
None.
Conflicts of interest
All authors declared that there are no conflicts of interest.
Ethical approval and consent to participate
Not applicable.
Consent for publication
Not applicable.
Copyright
© The Author(s) 2024.
REFERENCES
1. Zhang X, Ao X, Cai W, Jiang Z, Zhang H. A sustainability evaluation method integrating the energy, economic and environment in remanufacturing systems. J Clean Prod 2019;239:118100.
2. Sarkar B, Ullah M, Sarkar M. Environmental and economic sustainability through innovative green products by remanufacturing. J Clean Prod 2022;332:129813.
3. Kernbaum S, Heyer S, Chiotellis S, Seliger G. Process planning for IT-equipment remanufacturing. CIRP J Manuf Sci Technol 2009;2:13-20.
4. Li J, Xie X, Chen G, et al. Analysis and application on technical evaluation model of remanufacturing for auto parts. Mach Design Res 2016;32:52-4. Available from: https://caod.oriprobe.com/articles/47641719/Analysis_and_Application_on_Technical_Evaluation_M.htm. [Last accessed on 23 Apr 2024]
5. Ramírez FJ, Castellani M, Xu W. Autonomous remanufacturing. Int J Adv Manuf Technol 2023;124:2971-2.
6. Wu CH, Wu HH. Competitive remanufacturing strategy and take-back decision with OEM remanufacturing. Comput Ind Eng 2016;98:149-63.
7. Yanıkoğlu İ, Denizel M. The value of quality grading in remanufacturing under quality level uncertainty. Int J Prod Res 2021;59:839-59.
8. Du Y, Zheng Y, Wu G, Tang Y. Decision-making method of heavy-duty machine tool remanufacturing based on AHP-entropy weight and extension theory. J Clean Prod 2020;252:119607.
9. Shi J, Li T, Liu Z. A three-dimensional method for evaluating the remanufacturability of used engines. Int J Sustain Manuf 2015;3:363-88.
10. Li C, Li C, Cao H, Yi Q. Uncertain remanufacturing process routings model for used components based on GERT network. Comput Integr Manuf Syst 2012;18:298-305. (in Chinese).
11. Peng S, Li T, Li M, et al. An integrated decision model of restoring technologies selection for engine remanufacturing practice. J Clean Prod 2019;206:598-610.
12. Zhang X, Zhang S, Zhang L, Xue J, Sa R, Liu H. Identification of product’s design characteristics for remanufacturing using failure modes feedback and quality function deployment. J Clean Prod 2019;239:117967.
13. Ke C, Jiang Z, Zhu S, Wang Y. An integrated design method for remanufacturing process based on performance demand. Int J Adv Manuf Technol 2022;118:849-63.
14. Shahbazi S, Johansen K, Sundin E. Product design for automated remanufacturing - a case study of electric and electronic equipment in sweden. Sustainability 2021;13:9039.
15. Boorsma N, Balkenende R, Bakker C, Tsui T, Peck D. Incorporating design for remanufacturing in the early design stage: a design management perspective. Jnl Remanufactur 2021;11:25-48.
16. Tant KMM, Mulholland AJ, Curtis A, Ijomah WL. Design-for-testing for improved remanufacturability. J Remanuf 2019;9:61-72.
17. Wang Q, Li B, Chen B, Wang Z, Liu W, Cheng Y. Impact of product design on remanufacturing under environmental legislation. Comput Ind Eng 2022;165:107889.
18. Ke C, Pan X, Wan P, Jiang Z, Zhao J. An integrated design method for used product remanufacturing scheme considering carbon emission. Sustain Prod Consump 2023;41:348-61.
19. Dey BK, Datta A, Sarkar B. Effectiveness of carbon policies and multi-period delay in payments in a global supply chain under remanufacturing consideration. J Clean Prod 2023;402:136539.
20. Yang X, Cao H, Liu H, Deng Q. Construct method of predicting satisfaction model of customer requirements based on product technical characteristics. J Manuf Technol Manag 2012;25:201-12.
21. Johnson CN. Best of back to basics: QFD explained. Qual Prog 2016;49:40. Available from: https://asq.org/quality-progress/articles/best-of-back-to-basics-qfd-explained?id=5f52b777dc954361a63b37e5f1790447. [Last accessed on 23 Apr 2024].
22. Kirgizov UA, Kwak C. Quantification and integration of Kano’s model into QFD for customer-focused product design. Qual Technol Quant Manag 2022;19:95-112.
23. Wu Y, Ho CC. Integration of green quality function deployment and fuzzy theory: a case study on green mobile phone design. J Clean Prod 2015;108:271-80.
24. Zhou J, Liu L, Sun L, Xiao F. A multi-criteria decision-making method for hesitant fuzzy linguistic term set based on the cloud model and evidence theory. J Intell Fuzzy Syst 2019;36:1797-808.
25. Wang L, Huang H, Chai Y. Choosing multi-issue negotiating object based on trust and K-armed bandit problem. J Softw 2006;17:2537-46 (in Chinese). Available from: https://jos.org.cn/josen/article/pdf/20061213.[Last accessed on 8 May 2024]
26. Al-shami TM, Mhemdi A. Generalized frame for orthopair fuzzy sets: (m,n)-fuzzy sets and their applications to multi-criteria decision-making methods. Information 2023;14:56.
27. Maputi ES, Arora R, Pham D. Gear concept selection procedure using fuzzy QFD, AHP and tacit knowledge. Cogent Eng 2020;7:1802816.
28. Yazdani M, Wang ZX, Chan FTS. A decision support model based on the combined structure of DEMATEL, QFD and fuzzy values. Soft Comput 2020;24:12449-68.
29. Wang H, Fang Z, Wang D, Liu S. An integrated fuzzy QFD and grey decision-making approach for supply chain collaborative quality design of large complex products. Comput Ind Eng 2020;140:106212.
30. Yazdani M, Kahraman C, Zarate P, Onar SC. A fuzzy multi attribute decision framework with integration of QFD and grey relational analysis. Expert Syst Appl 2019;115:474-85.
31. Zafar S, Alamgir Z, Rehman MH. An effective blockchain evaluation system based on entropy-CRITIC weight method and MCDM techniques. Peer Peer Netw Appl 2021;14:3110-23.
32. Wang C, Le TQ, Chang K, Dang T. Measuring road transport sustainability using MCDM-based entropy objective weighting method. Symmetry 2022;14:1033.
33. Midrar T, Khan S, Abdullah S, Botmart T. Entropy based extended TOPOSIS method for MCDM problem with fuzzy credibility numbers. AIMS Math 2022;7:17286-312.
34. Rogulj K, Kilić Pamuković J, Ivić M. Hybrid MCDM based on VIKOR and cross entropy under rough neutrosophic set theory. Mathematics 2021;9:1334.
36. Deschênes L, Lyklema J. Entropy studies in interface science: an ageless tool. Curr Opin Colloid Interface Sci 2019;44:220-4.
39. Vapnik VN. Statistical learning theory. New York: Wiley; 1998. Available from: https://www.wiley.com/en-us/Statistical+Learning+Theory-p-9780471030034. [Last accessed on 23 Apr 2024].
40. Xiao Y, Li H. Improvement on judgement matrix based on triangle fuzzy number. Fuzzy Syst Math 2003;17:59-64. (in Chinese) Available from: http://en.cnki.com.cn/Article_en/CJFDTOTAL-MUTE200302011.htm.[Last accessed on 26 Apr 2024].
Cite This Article
Export citation file: BibTeX | EndNote | RIS
OAE Style
Shi J, Yu Z, Li M, Pan Y, Zhu H. An entropy - based fuzzy QFD model on used product remanufacturing design. Green Manuf Open 2024;2:8. http://dx.doi.org/10.20517/gmo.2023.103101
AMA Style
Shi J, Yu Z, Li M, Pan Y, Zhu H. An entropy - based fuzzy QFD model on used product remanufacturing design. Green Manufacturing Open. 2024; 2(2): 8. http://dx.doi.org/10.20517/gmo.2023.103101
Chicago/Turabian Style
Junli Shi, Zihui Yu, Mingying Li, Yuxiang Pan, Huawei Zhu. 2024. "An entropy - based fuzzy QFD model on used product remanufacturing design" Green Manufacturing Open. 2, no.2: 8. http://dx.doi.org/10.20517/gmo.2023.103101
ACS Style
Shi, J.; Yu Z.; Li M.; Pan Y.; Zhu H. An entropy - based fuzzy QFD model on used product remanufacturing design. Green. Manuf. Open. 2024, 2, 8. http://dx.doi.org/10.20517/gmo.2023.103101
About This Article
Special Issue
Copyright
Data & Comments
Data
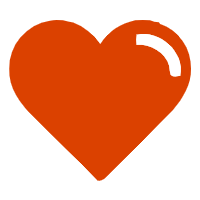
Comments
Comments must be written in English. Spam, offensive content, impersonation, and private information will not be permitted. If any comment is reported and identified as inappropriate content by OAE staff, the comment will be removed without notice. If you have any queries or need any help, please contact us at support@oaepublish.com.