A life cycle decision framework of China offshore wind turbines with ANP-Intuitionistic fuzzy TOPSIS method
Abstract
In China, offshore wind energy is a popular source of green energy. Selecting offshore wind turbine design schemes is a decision problem based on multiple criteria. However, the selection is hardly made from the point of view of the life cycle due to the complex association of evaluation criteria, especially in the conceptual design stage. To solve this problem, a new multi-criteria decision-making framework for selecting the life cycle design scheme of offshore wind turbines is designed. The design information of the life cycle process of these turbines is expressed using Function-Structure-Material-Process-Transportation model in this framework. The life cycle evaluation index system of offshore wind turbines was established and mapped with this model to carry out rapid evaluation of various schemes. In light of this, an intuitive fuzzy Technique for Order of Preference by Similarity to Ideal Solution (TOPSIS) method based on analytic network process (ANP) was proposed to process fuzzy decision information and establish criteria correlation. Finally, a case study was conducted, and sensitivity and comparative analyses were carried out. The proposed method can effectively select the optimal scheme from six different design schemes for the life cycle of offshore wind turbines.
Keywords
INTRODUCTION
Energy consumption is rising daily as a result of the quick development of the world economy, and all nations are deeply concerned about the greenhouse gas emissions and environmental damage that the old energy production method causes. Nowadays, the new energy has entered a stage of rapid development, and many researchers are paying increasing attention to wind energy resources. The primary way wind energy is used is through wind power, which, compared with other new energy sources, offers low cost, cleanliness and significant benefits. The global wind power business has been flourishing in recent years, particularly with the quick advancement of offshore wind turbines. These turbines have several advantages over onshore wind turbines, including not occupying land, high wind speed, long utilization time and stable wind energy resources. In addition, they are usually located near the power load center, and wind power is more easily connected to the grid. In the context of low-carbon energy transition, factors such as the reduction of offshore wind power costs and large-scale wind turbines will drive the installed capacity to continue to increase, and offshore wind power will usher in a period of rapid growth. According to the data collated by the National Energy Administration of China and China Business Industry Research Institute, the cumulative installed capacity of offshore wind power reached 3,051 million kilowatts by the end of 2022, and it is expected to reach 5,841 million kilowatts by 2025 [Figure 1]. Nowadays, offshore wind farms have become one of the key directions of the future wind energy industry.
However, due to the complexity of the offshore environment compared to the onshore environment, including high salt spray, lightning strikes, and offshore typhoons, offshore wind turbines are significantly more complex than onshore structures, and the technical difficulty and cost of installation, operation and maintenance are high. As a result, there are higher requirements for selecting offshore wind turbines[1,2]. Although offshore wind power generation helps to reduce environmental pollution and greenhouse gas emissions, the manufacturing, transportation and decommissioning produce a lot of greenhouse gas emissions and consume energy[3]. Therefore, during the life cycle of offshore wind turbines, the influence on the environment should be considered. In this context, an effective way to solve the above problems is Life Cycle Design to integrate green characteristics into the whole process of the product life cycle[4,5].
Because of the importance of wind energy, a great deal of research has been done on it. In the past few years, an increasing number of academics have researched the evaluation and decision-making of offshore wind turbines. The conceptual design of products has been supported by a significant number of design schema representation models. Meanwhile, to address the selection and design optimization issues of offshore wind turbines, various evaluation criteria systems and evaluation decision-making methods for these turbines are proposed. Studies related to this research can be categorized into three categories: design scheme expression models, evaluation criteria systems, and design scheme decision-making methods for offshore wind turbines. Comparisons of relevant investigations for each group are provided below.
The life cycle design is widely regarded as the most critical stage to achieve product greenness[6]. Conceptual design is a key factor affecting product performance, cost and environment, and the program decisions made at this stage also have an important effect on the environment. Gero and Kannengiesser took the lead in proposing the conceptual design model of function-behavior-structure (FBS) and generated design schemes by establishing functional decomposition and association mapping[7,8]. In order to adapt to complex and variable product design requirements, many improvements have been made to FBS models, such as functional decomposition and modeling[9], requirement-FBS models[10], function-behavior-state models[11], demand-function-Principle-system models[12], etc. However, most of these models only meet the functional and design requirements of products and lack the consideration of product environment information. In order to support life cycle design modeling effectively, numerous scholars have introduced environmental information into FBS models. Deng proposed a function-environment-behavior-structure conceptual design approach for environmental protection[13]. Li et al. used FBS model to establish an energy consumption model and linked design aspects with energy use in order to take the environmental impact into account[14]. In order to solve environmental problems, especially to reduce resource consumption and waste, Umeda et al. proposed an upgradable product design method based on FBS model[15]. The above research based on the improved FBS model can support the green design of products, but the life cycle design takes into account the green characteristic of the product life cycle, and there are problems such as excessive design information, wide range and difficult access. The above studies rarely consider material, process, transportation and other information and cannot effectively generate and express product life cycle design solutions, which makes it hard to sustain the life cycle design of offshore wind turbines.
Selecting suitable design schemes for the life cycle of offshore wind turbines can not only boost power generation profits and lower operation and maintenance costs[16] but also help these turbines perform more sustainably by addressing potential environmental issues at the outset of the design process. Most studies only take into account the influencing factors when determining the best offshore wind turbine, including technical performance[17], economic performance[18], environmental problems[19], wind resources[20], etc. What is more, the impact on society for those who gain from it must be considered. As a result, Abdel-Basset et al. established a site selection evaluation system for offshore wind turbines by comprehensively considering six indicators: wind resources, construction, economy, environment, society, and risk, along with related secondary indicators[21]. Yu et al. put forward a standard system for evaluating offshore wind turbines, including technical reliability, resources, economy, environmental impact and supplier performance[22]. Offshore wind turbines have a very complex operating environment, which makes maintenance time-consuming and expensive. Based on the initial criteria, Ma et al. incorporated technology for intelligent monitoring, anti-corrosion, lightning protection, and anti-typhoon[23]. Rotor diameter was one of the selection factors for offshore wind turbines that Wang et al. accounted for with the ongoing improvement of the decision index[24]. To sum up, although the existing offshore wind turbine evaluation index system provides a variety of evaluation indicators, the evaluation index is single and imperfect (only several major economic, environmental, social, risk and other indicators are considered), and the influencing factors of the design scheme are not systematically considered from the perspective of the whole life cycle. Consequently, the final evaluation result lacks comprehensiveness and objectivity. From the actual situation, for the special operating environment of offshore wind turbines, some indicators such as ease of maintenance, ecological impact, noise and visual impact cannot be ignored.
Generally, the choice of offshore wind turbines is thought of as a multi-criteria decision methods (MCDM) problem[25], and one of the most commonly used models is analytic hierarchy process (AHP). Huang et al. come up with a decision-making method based on AHP to study the selection of wind turbines by evaluating the effective performance of three representative bidding wind turbines for an offshore Fujian wind farm[26]. Guner et al. conducted a study on the installation of offshore wind power plants in Turkey and used the AHP, one of the techniques for MCDM analysis, to conduct appropriate site selection[27]. Wind turbines incorporate the AHP and other MCDM approaches while making decisions. Through the Technique for Order of Preference by Similarity to Ideal Solution (TOPSIS), Lozano-Minguez et al. suggest a strategy for assessing the selection of support structures for offshore wind turbines in various designs[28]. Bagočius et al. used AHP to ascertain criteria weights and Weighted Aggregates Sum Product Assessment (WASPAS) to evaluate offshore wind turbines[29]. However, considering the fuzziness and subjectivity of expert judgment, the MCDM method, in conjunction with fuzzy set theory (FST), is applied. Using fuzzy Bayesian networks and expert domain knowledge, Xue et al. offer a new offshore wind turbine selection method[30]. Deveci et al. used interval rough numbers (IRN) in conjunction with the best-worst method (BWM) to select the greatest offshore wind farm sites in the Turkish coastal region[31]. Spreafico et al. introduced prospective life cycle assessment (LCA) to evaluate the environmental sustainability of eco-design solutions (ideas, immature products, etc.), all processes to gather product life cycle specific functions from patent literature, used to build a prospective inventory of LCA to ensure data quality; it is applied to the case study of titanium powder production through innovative processes[32]. Even though the above decision-making methods are widely employed in offshore wind turbine selection, the evaluation criteria are independent of each other and do not consider their correlation, which reduces the precision of the decision-making results. In particular, the assessment of offshore wind turbines involves numerous and interrelated criteria.
Therefore, in view of the above issues, this study not only accounts for the technical, economic, social and environmental impacts but also further considers the indicators of the whole life cycle stage of offshore wind turbines. Given the relationship between these indicators, a new selection method for offshore wind turbines is proposed in this paper. It can be seen from previous studies that TOPSIS can be used in the field of MCDM, and the cons and pros of each scheme can be quantitatively evaluated on the same scale, which is suitable for assessing the design of offshore wind turbines[33]. Therefore, TOPSIS is used as the main method of alternative ranking in this study. Additionally, the intuitionistic fuzzy numbers (IFNs) exhibit better performance in displaying the uncertainty of decision information[34]. To this end, in order to make full use of the information from the experts, indicators and design schemes, this paper adopts IFNs to overcome the problem of fuzzy information. In the evaluation criteria system, there are mutual relations among indicators. The importance of each criterion was analyzed layer by layer in AHP. However, AHP defaults that the standards of the same layer are independent of each other. Relative to AHP, the weight determination method of analytic network process (ANP) eliminates the strict hierarchical relationship of AHP in the network layer and considers the dependency and feedback of criteria in decision-making problems[35]. To sum up, on the basis of the above supplements and improvements, the results of offshore wind turbine selection will be more reasonable.
Based on the above analysis, this paper aims to address the problems existing in the evaluation and decision of offshore wind turbine design schemes and proposes a multi-criteria decision-making framework for the life cycle design scheme of offshore wind turbines based on ANP-Intuitionistic fuzzy TOPSIS method for enterprise designers to make the optimal decision in offshore wind turbine design. The main contributions are as follows:
(1) The Function-Structure-Material-Process-Transportation (FSMPT) model of the life cycle design scheme of offshore wind turbines is constructed. In the conceptual design stage, the design information of the product, such as function, structure, material, process and transportation, is considered. This model can fully express and integrate the life cycle information of the offshore wind turbine design scheme and support the generation and decision of the life cycle design scheme.
(2) Based on the existing research experience, the evaluation criteria system of the life cycle of offshore wind turbines is established, which can cover all stages of the life cycle of wind turbines, and can be mapped and correlated with the FSMPT model to quickly evaluate the design scheme. The integration and effective correlation of offshore wind turbine design information with life cycle stages is realized.
(3) A decision-making method for the life cycle design of offshore wind turbines based on the ANP-Intuitionistic fuzzy TOPSIS is proposed, which quantifies the interaction and feedback between the two indicators according to the contact information, solves the decision-making problem of multi-indicator interaction, and deals with the uncertain information effectively. This approach can improve the integrity of decision information and the accuracy of decision results and sort out the optimal scheme.
The remainder of this work is structured as follows. Section “METHODS” focuses on the FSMPT conceptual model and the evaluation index system for the offshore wind turbine life cycle design; the decision-making framework for the offshore wind turbine life cycle design based on ANP-Intuitionistic fuzzy TOPSIS is introduced. Section “RESULTS” provides examples of offshore wind turbines to demonstrate the implementation process and efficacy of this framework. Section “DISCUSSION” analyzes and discusses the result of the case. The conclusions are presented in the final section.
METHODS
FSMPT model for the life cycle design expression of offshore wind turbines
The conceptual design model of most products only considers the function and product design requirements, and lacks the product life cycle information, which cannot effectively generate and express the product life cycle design scheme. Therefore, based on FBS model[8] and product structure tree, a life cycle design scheme expression model of offshore wind turbines, namely FSMPT model, was built [Figure 2]. Through the direct mapping of function to structure, the model is integrated into the interdomain (vertical) and intra-domain (horizontal) to realize the interaction of the five aspects of offshore wind turbine information so as to provide support for the life cycle design of offshore wind turbines.
Figure 2. Function-Structure-Material-Process-Transportation (FSMPT) model of offshore wind turbines.
In the FSMPT model, F represents function domain: function is generally determined according to user and design requirements; S stands for structure domain: structure is the carrier to realize the function, that is, the design unit, which refers to the relationship between various parts of the offshore wind turbine, so as to achieve certain functions; M denotes material domain: the main raw materials used to produce various offshore wind turbine structures, including auxiliary materials, etc.; P signifies process domain: during the production process of offshore wind turbine, depending on the structural characteristics of raw materials and offshore wind turbine, appropriate processing methods have been chosen to process raw materials to obtain the structure required by the offshore wind turbine; T symbolizes transportation domain: transportation of components and raw materials for offshore wind turbines.
Evaluation criteria system for the life cycle design of offshore wind turbines
The life cycle process of offshore wind turbines
The life cycle process of offshore wind turbines is divided into five stages: raw material acquisition, production and manufacturing, transportation and installation, operation and maintenance, and disassembly and disposal[36] [Figure 3]. Each phase involves a number of processes. The raw material acquisition phase is mainly raw material steel smelting. During the production and manufacturing phase, components of the foundation, tower, engine room, rotor and transmission grid are manufactured in the offshore wind turbine factory. The parts will then be transported from the plant to the offshore wind farm and assembled and installed through crane work and other on-site construction work during the transportation and installation phase, including the hoisting method, the type of installation ship, and the transportation organization of materials in the yard. The operation and maintenance phase includes oil and lubricant changes, gear and generator refurbishment, and transportation to and from the wind turbine for regular inspections[37], taking into account the capacity of wind farms, location away from the shore or port, marine climate and hydrological conditions. Finally, in the disassembly and disposal phase, the main processes include the dismantling of the offshore equipment, the transportation from the installation location to the disposal location, and the disposal of materials[38]. These five stages describe the entire life cycle of an offshore wind generator from birth to death, with various properties at different stages influencing each other.
Evaluation criteria system for the life cycle design
A literature survey was conducted to preliminarily determine the criteria to be used in decision studies for offshore wind turbines. Then, by referring to the evaluation criteria system of green mechanical and electrical products of some scholars and following the principle of establishing the evaluation criteria system of green products[39,40], the multi-attribute green evaluation index system of offshore wind turbines is established in accordance with the current research progress on selection of offshore wind turbines and product attributes. There are six main criteria (environment, technology, resources, energy, economic and social attributes) with 25 sub-criteria.
The above attributes cover all stages of the life cycle for offshore wind turbines. Due to the varying emphases of each stage, some attributes or sub-criteria of the multi-attribute green evaluation criteria system should be selectively ignored at distinct stages in practical application. In addition, so as to facilitate the subsequent calculation during the calculation process, the attribute layer will be crossed, the criteria layer will be directly modeled, and the specific sub-criteria value of the criteria layer will be calculated separately[41]. Therefore, based on the multi-attribute green evaluation index system of the design decision and life cycle process of offshore wind turbines, the evaluation criteria system of the life cycle phase is constructed. As shown in Figure 4, five main criteria include 33 sub-criteria. Table 1 lists these criteria and their explanations.
Explanations of the life cycle evaluation criteria system of offshore wind turbines
Sub-criteria | Explanation |
(S1 Raw material acquisition, S6 Production and manufacturing, S12 Transportation and installation, S19 Operation and maintenance, S29 Disassembly and disposal phase) energy consumption | Energy is consumed in manufacturing, logistics and decommissioning of offshore wind turbines[3]. Roughly more than 84% of the energy is used to produce wind turbines, with the rest used for transportation, foundation, maintenance and demolition |
(S2 Raw material acquisition, S7 Production and manufacturing, S13 Transportation and installation, S20 Operation and maintenance, S30 Disassembly and disposal phase) resource consumption | Resources mainly refer to machinery and equipment, materials, water and humans[42] |
S3 Material acquisition technology level | The raw material of offshore wind turbines is mainly steel, and the smelting process of steel is very important. Therefore, the technical level of raw material acquisition should be considered |
S4 Raw material cost | The cost of purchasing and acquiring raw materials for offshore wind turbine components |
(S5 Raw material acquisition, S11 Production and manufacturing, S18 Transportation and installation, S33 Disassembly and disposal phase) greenhouse gas emissions | The manufacturing, logistics and decommissioning of offshore wind turbines will generate greenhouse gas emissions[3]. The steel in the tower, the engine room and the concrete in the foundation are the main causes of environmental impact |
S8 Manufacturing process complexity | Offshore wind turbines to adapt to various materials and processes, parameters matching to ensure the production of high-quality wind turbines |
S9 Manufacturing technology level | Including structural reliability, assembly technology level, etc. Specifically refers to the physical strength, safety and dependability of offshore wind turbine systems |
S10 Manufacturing process cost | The process cost of producing and manufacturing the offshore wind turbine parts |
S14 The technical complexity of transportation and installation | Some measures taken during transportation and installation of offshore wind turbines include cable laying, foundation and installation of wind turbines, etc.[43] |
S15 Transportation and installation cost | The cost of installing and purchasing offshore wind turbines[24] |
S16 Degree of social need | It is composed of opinions of residents and has an important impact on future development[18]; On the other hand, it is the dual carbon policy, green environmental protection needs |
(S17 Transportation and installation, S27 Operation and maintenance phase) ecological impact | Offshore wind turbines will produce noise pollution during construction and operation, which will have a negative impact on Marine animals. Influence the flight of birds[19] |
S21 Turbine output power | The efficiency of wind turbines capturing wind energy is related to wind speed and rotational speed |
S22 Ease of maintenance | Maximum generating power, low start-up wind speed, and the conversion rate of wind energy to electricity are desirable |
S23 System conversion rate | Due to the complexity of the offshore wind farm environment, maintenance time and expense are high in case of failure, so the maintenance simplicity is very important[24] |
S24 Operational safety | Offshore wind farms, affected by the hostile environment, have high requirements for wind turbines, and their operation safety deserves high attention[24] |
S25 Operating cost | Labor, maintenance, and energy consumption costs are all included in the total cost of an offshore wind turbine in operation[17] |
S26 Sales profit | Annual funds generated by offshore wind turbines[44] |
S28 Noise and visual impact | Noise from offshore wind turbine operations causes sleep disorders and hearing loss among residents. The blades interrupt sunlight and produce shadow flickering[19] |
S31 Recovery technology level | Offshore wind turbine recovery measures taken when the reinforced concrete foundation is abandoned; Waste iron and steel are raw materials for metal smelting and processing |
S32 Recovery cost | Cost of offshore wind turbine recovery, landfill, incineration, etc.[17] |
Some of these wind turbine base indicators combine the product attributes of offshore wind turbines, such as:
(1) The transportation and installation phase of offshore wind turbines is considered more over onshore, including transport distance, transport mode, cable laying, foundation installation and wind turbine installation; 81% of the total cost is due to the installation of towers, nacelles, blades and foundations. Offshore wind turbines can often be installed entirely at once or in sections. Although there are few hoisting times over the entire hoisting procedure and little time is spent building at sea, the assembly yard must be set up on land. Meanwhile, transportation concerns and very high requirements exist for the loading capacity of the dock, the loading capacity of the barge, and the capability for lifting and hoisting. The hoisting period is lengthy, and numerous hoisting times occur during the segmented hoisting procedure. To sum up, the technical complexity and transportation installation cost need to be considered in the index system.
(2) All stages of the life cycle of offshore wind turbines will affect Marine life and birds, resulting in greenhouse gas emissions and resource energy consumption. Indicators such as ecological impact, greenhouse gas emissions and resource energy consumption are thus taken into account in these stages.
(3) In addition to rain, snow, fog, wind and other bad weather conditions, onshore wind farms can generally be quick and timely on-site maintenance. However, due to the changing climate of the offshore environment and the complex situation of the sea wave ditch, the operation environment of offshore wind turbines is harsh, and maintenance personnel can only reach the designated place through the work boat or helicopter to repair or replace the equipment. This adds to the difficulty and high cost of repair and maintenance, which influences the cost of wind power. Therefore, high requirements are put forward for the operation safety and maintenance simplicity in the operation and maintenance phase.
(4) As offshore wind turbines must withstand strong wind loads at sea, seawater corrosion and wave impact, etc., they are far more complicated than those on land, with high technical difficulty and high construction costs. Challenges include optimizing the design of the wind turbine structure to effectively withstand strong wind load, fatigue load, and unbalanced wind turbine load during the life cycle of the unit. Additionally, there is a need to optimize the tower structure and mechanical support structure design to meet the requirements of offshore wind turbine load as much as possible to reduce the weight of the whole machine. Designing the wind turbine transmission chain and the system layout to improve the reliability and maintainability of the wind turbine is also crucial. Therefore, the manufacturing process and cost in the production and manufacturing phase are very important.
Association between FSMPT model and evaluation criteria system
To address issues such as low integration degree and lack of effective correlation between FSMPT model and life cycle stage, the correlation mapping between FSMPT model and the evaluation criteria system of the offshore wind turbines life cycle is constructed, and the mapping correlation between the criteria of the life cycle phase and the model domain is used to accurately and rapidly evaluate the design scheme of the offshore wind turbines life cycle.
In the FSMPT model, the material domain represents the raw materials needed to produce offshore wind turbines and is associated with the raw material acquisition phase; The function domain refers to the functions of wind turbines and is connected with the operation and maintenance phase; The process domain pertains to the production and processing mode of wind turbine and is correlated with production and manufacturing phase; The structure domain is concerned with disassembly and disposal phase; The transportation domain relates to the transportation and installation phase.
The correlation between the FSMPT model and the life cycle evaluation criteria system is shown in Figure 5. The FSMPT model is on the left, while the five main criteria of the criteria system are on the right. The two sides are collectively called the correlation mapping between the FSMPT model and the life cycle evaluation criteria system of the offshore wind turbine.
Decision-making framework for the life cycle design of offshore wind turbines
The intuitionistic fuzzy set (IFS) is Bulgarian scholar Atanassov’s extension of Zadeh’s classic FST. It also considers three characteristics: membership, non-membership and hesitation[45], and it has strong flexibility and applicability in solving fuzzy problems. TOPSIS is a commonly used multiple index decision analysis method. The relative distance between each scheme and the ideal scheme is computed by creating “positive ideal solution” and “negative ideal solution” of assessment issue to rank the pros and cons. Bilgili et al. introduced intuitive fuzzy TOPSIS. After determining the weights of evaluation criteria, they constructed an intuitive fuzzy evaluation matrix for design schemes and then used TOPSIS method to evaluate and rank design schemes[46].
Weight determination is the basis of design scheme evaluation. In the evaluation criteria system, there often exists a mutual relationship between the criteria, but AHP fails to reflect this internal relationship. To solve the structure of decision problems with dependency and feedback, Professor Thomas L. Saaty of the University of Pittsburgh devised the weight determination method (ANP)[47] based on AHP[48].
The criteria correlation is not considered in the selection process of the life cycle design schemes of offshore wind turbines, and the uncertainty information will be generated when the expert decision is made. As a result, this study provides the Intuitionistic fuzzy TOPSIS framework based on ANP theory to analyze offshore wind turbine design schemes and assist firms in finding the optimal design scheme. As shown in Figure 6, the proposed framework can be separated into four components: scheme expression, criteria calculation, expert evaluation, and result ranking. First, experts select and express alternative life cycle design schemes of offshore wind turbines based on experience and FSMPT model. Secondly, ANP is used to establish criteria relation and calculate its weight. Thirdly, the expert weights are determined and the schemes are scored with IFNs according to the correlation between criteria and model. Finally, to rate the design schemes and find the ideal option, TOPSIS is employed.
Suppose X = {X1, X2, …, Xq}, Y = {Y1, Y2, …, Yz}, and A = {A1, A2, …, Ap} represent a set of indices, subindices, and alternative design options, respectively. The implementation of ANP-Intuitionistic fuzzy TOPSIS method requires the following steps.
Step 1: Identify alternative life cycle design schemes of offshore wind turbines. The FSMPT model, including material, transportation, process, use and recycling, is adopted to express the design schemes.
Step 2: Use ANP to establish the criteria network and determine the criteria weight. Many factors influence the selection of design schemes of offshore wind turbines. Different criteria and correlations exert distinct influences on the selection of a design scheme. These are the precise steps:
1. Build the network structure.
Assemble the thoughts of various experts, determine the evaluation criteria, ignore the factors that have less impact on the overall, group the factors, form the factor group, and determine the interaction between levels, between and within the factor group. The ANP structure is then built based on the hierarchy in the control layer and the influence relationship between criteria in the network layer [Figure 7].
2. Construct a judgment matrix.
Experts are consulted to determine the relative weights of the two evaluation criteria, and values are then assigned using the “1-9 scale method” in Table 2 in order to create the decision matrix. First, the criteria
1-9 scale method
Scale | Description |
1 | The i factor is as significant as the j factor |
3 | The i factor is slightly more significant over the j factor |
5 | The i factor is obviously significant over the j factor |
7 | The i factor is strongly significant over the j factor |
9 | The i factor is critically significant over the j factor |
2, 4, 6, 8 | Somewhere in between |
3. Construct the unauthorized super matrix.
Taking N factor groups as the main norm, the internal and external relations among factors of each factor group were compared in turn, and the weighting matrix Ws was obtained. This matrix does not display the priority, and pairs of factor groups need to be compared to make the non-weighted matrix become the weighted matrix.
4. Create the weight super matrix.
Taking the factor group Cj as the norm, the paired comparison of the factor group is carried out, and the matrix aj is constructed. The normalization is carried out to obtain the normalized eigenvector (a1j, a2j, …, aNj)T. Then, taking N factor groups as the norm, the normalized feature vectors of each matrix aj are summarized into a weight matrix As to reflect the relationship between factor groups.
With the weight matrix As, the non-weighted super matrix Ws can be transformed into the weighted super matrix W:
5. Obtain the ultimate super matrix.
Finally, the ultimate super matrix Wsl is employed to display the correlation between factors. When the limit converges and is unique, the weight of each factor in the matrix is obtained.
When ANP method involves a high number of criteria, calculating the matrix becomes difficult and complicated, which makes it easy to produce errors. Without the aid of software, it is challenging to apply ANP to resolve real-world decision issues. Therefore, to calculate complex matrices in this study, Super Decision (SD) software is utilized[49]. This software, created by Rozann W. Satty and William Adams in accordance with the principles of AHP and ANP, makes it easier to apply the ANP approach practically, which is particularly helpful for solving the MCDM problem. The steps of the software to implement the ANP method are shown in Figure 8.
Step 3: Linguistic terms and values for the significance of experts and design schemes.
Ambiguity and uncertainty arise when experts evaluate design proposals based on their own experience and subjective consciousness, and each expert has a different weight. As a result, fuzzy language can be utilized to solve the uncertainty of expert and design scheme evaluation attribute value. The fuzzy language is divided into several levels and the evaluation semantics are obtained by experts on the basis of a comprehensive understanding of the actual situation of offshore wind turbines by referring to the relevant standards. In order to process the data more conveniently and intuitively, we can employ mathematical methods to convert the fuzzy language of qualitative index into numbers. This work introduces IFS, which quantifies the fuzziness of expert evaluations.
In a finite set X, let M be the IFS[50]. The following is the definition of IFS M:
Where αM (x): X → [0, 1] represents the membership function, and βM (x): X → [0, 1] indicates the non-membership function.
In comparison to traditional FST, IFS adds a third component called hesitancy. If ηM (x) indicates the uncertainty degree about whether x belongs to M, ηM (x) can be represented as follows:
When ηM (x) is low, x is more certain that it belongs to M. When ηM (x) is large, it is even more uncertain that x belongs to M. When αM (x) = 1 - βM (x), IFS changes to FST. M is the IFS in set X; λ is a positive integer, and then the formula is[45]:
Therefore, the linguistic terms in Table 3 are employed and quantified by IFNs to calculate the weight of decision makers (DMs). To evaluate alternative design options, the linguistic terms in Table 4 are employed and quantified with IFNs.
The significance of linguistic terms for decision makers (DMs)
Linguistic terms | Intuitionistic fuzzy numbers (IFNs) |
Very Significant (VS) | (0.90, 0.10) |
Significant (S) | (0.75, 0.20) |
Medium (M) | (0.50, 0.45) |
Insignificance (I) | (0.35, 0.60) |
Very Insignificance (VI) | (0.10, 0.90) |
The significance of linguistic terms of alternative design options
Linguistic terms | Intuitionistic fuzzy numbers (IFNs) |
Extremely high (EH) | [1.00, 0.00] |
Very very high (VVH) | [0.90, 0.10] |
Very high (VH) | [0.80, 0.10] |
High (H) | [0.70, 0.20] |
Medium high (MH) | [0.60, 0.30] |
Medium (M) | [0.50, 0.40] |
Medium low (ML) | [0.40, 0.50] |
Low (L) | [0.25, 0.60] |
Very low (VL) | [0.10, 0.75] |
Very very low (VVL) | [0.10, 0.90] |
Step 4: Determine expert weights.
The experts have diverse academic backgrounds and individual abilities, which means that decisions made by each expert can exert varying effects on the final result. To this end, offshore wind turbine design companies determine the importance of an expert by considering three factors: (1) relevant experience in wind turbine design schemes; (2) relevant educational background in wind turbine design schemes; (3) the position of the expert.
Assuming there are l DMs, Dk = [αk, βk, ηk] can be used to indicate the IFN of kth DM, and the weight of kth DM can be calculated as:
Step 5: Gather expert opinions to evaluate alternative design proposals.
According to the mapping association between the life cycle criteria and the FSMPT model and Table 4, experts estimate alternative offshore wind turbine life cycle design schemes under various life cycle criteria and convert these evaluation results into corresponding IFNs.
Assume that T(k) = (tij(k))p*q is each DM intuitionistic fuzzy decision matrix, and λ = {λ1, λ2, λ3, …, λk, …, λl} is the weight of DM; according to the Intuitionistic Fuzzy Weighted Averaging (IFWA) operator[51], an aggregate intuitionistic fuzzy decision matrix is established after combining expert opinions as follows:
Where tij = [αAi (xj), βAi (xj), ηAi (xj)] (i = 1, 2, …, p; j = 1, 2, …, q).
Step 6: Establish the aggregate weighted intuitionistic fuzzy decision matrix.
By calculating the weight W of the index and constructing the matrix T, the aggregate weighted intuitionistic fuzzy decision matrix T’ can be generated using Equation (8) for intuitionistic fuzzy multiplication.
Step 7: Get intuitionistic fuzzy ideal solutions.
Suppose that Q1 and Q2 are, respectively, benefit and cost indicators, and U+ and U-, respectively, represent positive and negative intuitionistic fuzzy ideal solutions. Equation (11) determines U+ and U-:
Among them,
Step 8: Determine the distance measures.
According to Equations (16) and (17), the normalized Euclidean distance Ei+ and Ei- related to intuitionistic fuzzy positive and negative ideal solutions for each alternative design scheme are calculated[52].
Step 9: Determine the relative proximity.
Equation (18) is used to define the relative proximity for alternative design scheme Ai in relation to the intuitionistic fuzzy ideal solution:
Step 10: Determine the ranking of alternative design options.
According to the calculation results of relative proximity, the alternative design schemes are arranged in descending order, and the life cycle design scheme of green offshore wind turbines is selected.
RESULTS
Case description
The life cycle design schemes of the offshore wind turbine manufactured by China’s CRRC Shandong Wind Power Co., Ltd. are evaluated and decided in this study. Table 5 displays the primary technical characteristics of the offshore wind turbine.
Main technical parameters of offshore wind turbine
Model number | Rated power (MW) | Rated speed (m/s) | Incoming/outgoing wind speed (m/s) | Service life (years) |
CWT2500-D143 | 10 | 10 | 3/22 | 20 |
Decision on the life cycle design of offshore wind turbines
Identify alternative life cycle design schemes
Through enterprise research, the present development trend of offshore wind turbines, comprehensive consideration of wind resources, climate, geography and other considerations, experts preliminarily selected six design schemes for offshore wind farms (labeled as A1, A2, A3, A4, A5 and A6). The life cycle design model of FSMPT was established to express six design schemes. The life cycle design scheme of offshore wind turbines is mainly divided into five functions: energy conversion, variable pitch, transmission, yaw and support. Each function contains several sub-functions, structure, materials, process and transportation information. The relevant information is presented in Supplementary Tables 1 and 2. Among them, FSMPT model of A1 is shown in Figure 9.
Determination of the correlation and weights of criteria
The evaluation index of the life cycle for offshore wind turbines has a complex mutual influence relation. Four researchers who specialize in offshore wind turbines construct the criteria correlation questionnaire according to the actual situation.
After establishing the correlation of criteria, the particular steps to compute the weight of criteria are as follows: SD software is used to build the network structure of the life cycle evaluation criteria system for offshore wind turbines. Design scheme evaluation is set as the target layer; criteria serve as the norm layer, and sub-criteria as factors are added to the network layer [Figure 10]. Four experts and technicians in the field of offshore wind turbines were invited to discuss the questionnaire to fill in the judgment matrix. After the judgment matrix is substituted into ANP network, the weights of criteria and sub-criteria are obtained. The relevant data and calculation results are presented in Supplementary Tables 3 and 4.
Determination for the life cycle design scheme of green offshore wind turbine
Step 1: Importance and weight of DMs.
The importance of the three experts (DM1, DM2, DM3) was determined by the offshore wind turbine Company, and the linguistic terms and weights of the importance of DMs were obtained according to Table 3 and Equation (9).
Step 2: Establish the aggregate intuitionistic fuzzy decision matrix.
On the basis of Table 4, according to the grade of the qualitative index and the numerical value of the quantitative index (obtained by enterprise research), the scores of DMs on the design schemes are obtained, and these scores are converted into IFNs. The relevant data and calculation results are presented in Supplementary Tables 5-7.
In order to aggregate the opinions of DMs, the aggregate matrix T is obtained according to Equation (10), as given below:
Step 3: Establish the aggregate weighted intuitionistic fuzzy decision matrix.
According to ANP method, the weights of aggregate criteria and sub-criteria (ACS) are below:
According to Equations (8) and (10), the weighted matrix T’ is constructed as follows:
Step 4: Get the intuitionistic fuzzy ideal solution.
The operation and maintenance phase, disassembly and disposal phase are the benefit criteria Q1 = {C4, C5}; the raw material acquisition phase, production and manufacturing phase, transportation and installation phase are the cost criteria Q2 = {C1, C2, C3}; the intuitionistic fuzzy ideal solutions are as follows:
Step 5: Determine the distance measures.
According to Equations (16) and (17), euclidean distance for each alternative design scheme is obtained [Table 6].
Ranking of alternative design schemes
Alternative design schemes | Ei+ | Ei- | Ci | Rank |
A1 | 0.3550 | 1.1061 | 0.7570 | 1 |
A2 | 0.9803 | 0.4741 | 0.3260 | 3 |
A3 | 1.0296 | 0.4714 | 0.3141 | 4 |
A4 | 0.9739 | 0.4210 | 0.3018 | 6 |
A5 | 1.0654 | 0.4873 | 0.3138 | 5 |
A6 | 0.3742 | 1.0524 | 0.7377 | 2 |
Step 6: Rank solutions.
The alternative design schemes were ranked in Table 6 in descending order of relative proximity: A1 > A6 > A2 > A3 > A5 > A4; A1 was chosen as the finest life cycle design scheme for offshore wind turbines.
DISCUSSION
The weight results indicate that summing the weights of the sub-criteria will obtain the weight of the index. C1 and C2 have the highest weights, which are 0.2914 and 0.4147 separately. C3 and C4 have the least weight of 0.1099 and 0.0468, separately. The results indicate that when it comes to choosing the life cycle design schemes of offshore wind turbines, the experts pay much attention to the C2 production and manufacturing phase. The environmental impact of this phase accounts for a bigger share of the total life cycle, with over 84% of the energy used for production. This is mainly because the equipment and foundation consume a lot of steel materials, coupled with the need to deal with the complex climate and dangerous operating environment, complex structure and technology, difficult maintenance, and higher requirements for this stage. Offshore wind farms are relatively expensive, with material costs typically accounting for about 40% to 50% of total investment. Therefore, it is clearly reasonable to use the C1 raw material acquisition phase to evaluate the competitiveness of wind turbines. When dealing with the discarded wind turbine, the metal in the foundation, blades, engine room and tower barrel can be recycled, and the remaining chemical materials can be landfill. Therefore, the importance of the C5 disassembly and disposal phase is obvious. Although the weight of the C3 transportation and installation phase does not dominate this decision, it remains important. Marine fuel is needed for the transportation of wind turbine equipment and building materials, installation and construction of equipment, and transportation of operations and maintenance personnel and consumables. There is a small amount of self-consumption power in the commissioning phase of operation and maintenance of wind turbines, which consumes almost no power in operation and has fewer maintenance times, so C4 operation and maintenance phase has the least impact. TOPSIS means that the best design scheme is closest to the ideal solution, and A1 was chosen as the greenest life cycle design for offshore wind turbines.
Comparison of different methods
We compared the ANP-Intuitionistic fuzzy TOPSIS method with classical Intuitionistic fuzzy TOPSIS and AHP-Intuitionistic fuzzy TOPSIS methods and conducted comparative analysis on the outcomes of the same case to confirm the effectiveness and viability of the method proposed in this paper [Table 7].
Comparison of the proposed method with other methods
Evaluation methods | Calculation result | Rank | |||||
A1 | A2 | A3 | A4 | A5 | A6 | ||
Intuitionistic fuzzy Technique for Order of Preference by Similarity to Ideal Solution (TOPSIS) | 0.9450 | 0.2393 | 0.1287 | 0.1973 | 0.5189 | 0.3612 | A1 >A5> A6 > A2 > A4 > A3 |
Analytic hierarchy process (AHP)-Intuitionistic fuzzy TOPSIS | 0.7205 | 0.2939 | 0.2847 | 0.2529 | 0.3445 | 0.6960 | A1 > A6 > A5 > A2 > A3 > A4 |
Analytic network process (ANP)-Intuitionistic fuzzy TOPSIS | 0.7570 | 0.3260 | 0.3141 | 0.3018 | 0.3138 | 0.7377 | A1 > A6 > A2 > A3 > A5 > A4 |
To visually compare the results of different approaches, Figure 11 shows the ranking of their alternative design options. Figure 11 shows that the curve trends of the three methods are very similar; A1 is obviously the best choice of the three methods, and the optimal results are the same, which indicates that the proposed method is credible and acceptable. Additionally, the difference between the predictive ranking of the proposed method and AHP-Intuitionistic fuzzy TOPSIS lies in the position of A5, and the variance between the proposed method and Intuitionistic fuzzy TOPSIS is reflected in the ranking positions of A3 and A5. The relationship between criteria will obviously lead to the difference in ranking results. Through comparative analysis, we can conclude that the ANP-Intuitionistic fuzzy TOPSIS method does have its advantages in terms of stability and feasibility. Therefore, the evaluation method suggested in this paper can be applied as the core approach in the life cycle design scheme selection for offshore wind turbines.
Sensitivity analysis of index association
Sensitivity analysis was performed to confirm the stability of the ANP-Intuitionistic fuzzy TOPSIS framework. The outcomes of the three scenarios were analyzed in accordance with changes in the associations between criteria [Table 8 and Figure 12].
Ranking of alternative design schemes for three scenarios
Alternative design schemes | Original | 1 | 2 | 3 |
Ci (Rank) | Ci (Rank) | Ci (Rank) | Ci (Rank) | |
A1 | 0.7570 (1) | 0.7298 (1) | 0.8619 (1) | 0.6240 (1) |
A2 | 0.3260 (3) | 0.4777 (3) | 0.4821 (3) | 0.1706 (4) |
A3 | 0.3141 (4) | 0.4536 (4) | 0.4528 (5) | 0.1699 (5) |
A4 | 0.3018 (6) | 0.4374 (5) | 0.4666 (4) | 0.1046 (6) |
A5 | 0.3138 (5) | 0.3874 (6) | 0.2692 (6) | 0.4283 (3) |
A6 | 0.7377 (2) | 0.7112 (2) | 0.8473 (2) | 0.5924 (2) |
The purpose of the sensitivity analysis is to determine the stability of the proposed ANP-Intuitionistic fuzzy TOPSIS framework in the decision-making process. A small change in the relationship between criteria has little impact on the ranking of alternative offshore wind turbine life cycle designs. Table 8 and Figure 12 show that A1 has the highest score of the three experiments; Ranking sequence (A1 > A6 > A2) makes up a large proportion of the three scenarios, with only scenario 3 being different from the others. However, scene 3 and the original scene maintain the same bottom state of A4. Therefore, based on the evaluation obtained, the decision process of this paper was correspondingly robust in relation to the index, and in most cases, alternative design A1 emerged as the winner. In conclusion, the results suggest that the proposed framework is practicable, effective, and robust.
CONCLUSIONS
This work proposes a new multi-criteria hybrid decision-making framework for offshore wind turbines. Firstly, on the basis of the FBS model and product structure tree theory, the system integrates the product design information, such as function, structure, material, process and transportation, and establishes the FSMPT model to realize the life cycle information representation of the design scheme for offshore wind turbines. Then, combined with the peculiarities of the actual offshore wind turbines and the literature research and referring to the opinions of related industries and experts, the life cycle evaluation criteria system is established. The index system consists of five criteria and 33 sub-criteria, which can cover all phases of the wind turbine life cycle. Meanwhile, it can be mapped and correlated with the FSMPT model to quickly evaluate the design scheme. Finally, the ANP-Intuitionistic fuzzy TOPSIS evaluation method is constructed and used in the case study of evaluating and selecting the offshore wind turbine design scheme. The results show that the decision method put forth in this study effectively addresses the issue of reciprocal feedback of the life cycle criteria for offshore wind turbines, fully accounts for evaluation uncertainty, enhances the objectivity and precision of the evaluation outcomes, and can provide a reference for enterprises to design green offshore wind turbines.
Some limitations still persist in the follow-up research on the decision of the life cycle design for offshore wind turbines. First, it is necessary to further study the life cycle information of more specific material energy consumption and environmental impact in each stage of the life cycle of offshore wind turbines and optimize the life cycle evaluation criteria system. Second, the weight assigned by experts is static. Further consideration should be given to the volatile environment of offshore wind farms so that the decision criteria are weighted to account for the possibility of future variations. According to the particular circumstances, the weights of the various decision indices should be updated and modified.
DECLARATIONS
Acknowledgements
We would express our gratitude to CRRC Wind Power Co., Ltd, China for providing the data for the life cycle design of the wind turbine and thank all anonymous reviewers for their helpful suggestions to improve this paper.
Authors’ contributions
Conceptualization, writing-original draft: Zhou J
Formal analysis, visualization: Zhou J, Wang Y, Kong L, Guo J
Methodology: Zhou J, Wang L
Investigation: Zhou J, Ge C, Wang Y, Kong L, Guo J
Data curation: Wang L, Ge C
Supervision: Wang L, Li J, Li F
Funding acquisition: Li J, Li F, Li L
Availability of data and materials
All data and materials used or analyzed during the current study are included in this manuscript.
Financial support and sponsorship
This research was supported by the National Key R&D Program of China (Grant No. 2020YFB1711603) and the National Natural Science Foundation of China (Grant No. 52175473).
Conflicts of interest
All authors declared that there are no conflicts of interest. Ge C is affiliated with CRRC Wind Power Co., Ltd, China. Li F is an Editorial Board Member of the journal Green Manufacturing Open.
Ethical approval and consent to participate
Not applicable.
Consent for publication
Not applicable.
Copyright
© The Author(s) 2024.
Supplementary Materials
REFERENCES
1. Lubing X, Xiaoming R, Shuai L, Xin H. An opportunistic maintenance strategy for offshore wind turbine based on accessibility evaluation. Wind Eng 2020;44:455-68.
2. Mohammad Shafinejad M, Abedi M. Selection of suitable sites for offshore wind farms in the Caspian Sea and choosing the most suitable wind turbine in each area. Wind Eng 2021;45:294-313.
3. Yang J, Chang Y, Zhang L, Hao Y, Yan Q, Wang C. The life-cycle energy and environmental emissions of a typical offshore wind farm in China. J Clean Prod 2018;180:316-24.
4. Kong L, Wang L, Li F, et al. A life-cycle integrated model for product eco-design in the conceptual design phase. J Clean Prod 2022;363:132516.
5. Zhang X, Zhang L, Fung KY, Bakshi BR, Ng KM. Sustainable product design: a life-cycle approach. Chem Eng Sci 2020;217:115508.
6. Kuo TC, Wang CJ. Integrating robust design criteria and axiomatic design principles to support sustainable product development. Int J Pr Eng Man GT 2019;6:549-57.
7. Gero JS, Kannengiesser U. The situated function-behaviour-structure framework. Design Stud 2004;25:373-91.
8. Deng YM, Zhu YW. Function to structure/material mappings for conceptual design synthesis and their supportive strategies. Int J Adv Manuf Technol 2009;44:1063-72.
9. Yuan L, Liu Y, Sun Z, Cao Y, Qamar A. A hybrid approach for the automation of functional decomposition in conceptual design. J Eng Design 2016;27:333-60.
10. Christophe F, Bernard A, Coatanéa É. RFBS: a model for knowledge representation of conceptual design. CIRP Ann 2010;59:155-8.
11. Habib T, Komoto H. Comparative analysis of design concepts of mechatronics systems with a CAD tool for system architecting. Mechatronics 2014;24:788-804.
12. Chen Y, Zhao M, Xie Y, Zhang Z. A new model of conceptual design based on Scientific Ontology and intentionality theory. Part II: the process model. Design Stud 2015;38:139-60.
13. Deng YM. Function and behavior representation in conceptual mechanical design. AI EDAM 2002;16:343-62.
14. Li L, Yu S, Tao J, Li L. A FBS-based energy modelling method for energy efficiency-oriented design. J Clean Prod 2018;172:1-13.
15. Umeda Y, Kondoh S, Shimomura Y, Tomiyama T. Development of design methodology for upgradable products based on function-behavior-state modeling. AI EDAM 2005;19:161-82.
16. Wang Y, Qin Y, Wang K, et al. Where is the most feasible, economical, and green wind energy? Evidence from high-resolution potential mapping in China. J Clean Prod 2022;376:134287.
17. Supciller AA, Toprak F. Selection of wind turbines with multi-criteria decision making techniques involving neutrosophic numbers: a case from Turkey. Energy 2020;207:118237.
18. Deveci M, Cali U, Kucuksari S, Erdogan N. Interval type-2 fuzzy sets based multi-criteria decision-making model for offshore wind farm development in Ireland. Energy 2020;198:117317.
19. Peri E, Tal A. A sustainable way forward for wind power: assessing turbines’ environmental impacts using a holistic GIS analysis. Appl Energy 2020;279:115829.
20. Dhiman HS, Deb D. Fuzzy TOPSIS and fuzzy COPRAS based multi-criteria decision making for hybrid wind farms. Energy 2020;202:117755.
21. Abdel-basset M, Gamal A, Chakrabortty RK, Ryan M. A new hybrid multi-criteria decision-making approach for location selection of sustainable offshore wind energy stations: a case study. J Clean Prod 2021;280:124462.
22. Yu Y, Wu S, Yu J, Xu Y, Song L, Xu W. A hybrid multi-criteria decision-making framework for offshore wind turbine selection: a case study in China. Appl Energy 2022;328:120173.
23. Ma Y, Xu L, Cai J, Cao J, Zhao F, Zhang J. A novel hybrid multi-criteria decision-making approach for offshore wind turbine selection. Wind Eng 2021;45:1273-95.
24. Wang J, Xu L, Cai J, Fu Y, Bian X. Offshore wind turbine selection with a novel multi-criteria decision-making method based on Dempster-Shafer evidence theory. Sustain Energy Techn 2022;51:101951.
25. Gao J, Guo F, Ma Z, Huang X, Li X. Multi-criteria group decision-making framework for offshore wind farm site selection based on the intuitionistic linguistic aggregation operators. Energy 2020;204:117899.
26. Huang R, Zhang M, Guo M, et al. Selection of offshore wind turbine based on analytic hierarchy process. In: 2020 5th Asia Conference on Power and Electrical Engineering (ACPEE); 2020 Jun 04-07; Chengdu, China. IEEE; 2020. pp. 341-5.
27. Güner F, Başer V, Zenk H. Evaluation of offshore wind power plant sustainability: a case study of Sinop/Gerze, Turkey. IJGW 2021;23:370.
28. Lozano-minguez E, Kolios AJ, Brennan FP. Multi-criteria assessment of offshore wind turbine support structures. Renew Energy 2011;36:2831-7.
29. Bagočius V, Zavadskas EK, Turskis Z. Multi-person selection of the best wind turbine based on the multi-criteria integrated additive-multiplicative utility function. J Civ Eng Manag 2014;20:590-9. Available from: https://www.tandfonline.com/doi/abs/10.3846/13923730.2014.932836. [Last accessed on 25 Oct 2023]
30. Xue J, Yip TL, Wu B, Wu C, van Gelder PHAJM. A novel fuzzy Bayesian network-based MADM model for offshore wind turbine selection in busy waterways: an application to a case in China. Renew Energy 2021;172:897-917.
31. Deveci M, Özcan E, John R, Pamucar D, Karaman H. Offshore wind farm site selection using interval rough numbers based Best-Worst Method and MARCOS. Appl Soft Comput 2021;109:107532.
32. Spreafico C, Landi D, Russo D. A new method of patent analysis to support prospective life cycle assessment of eco-design solutions. Sustain Prod Consump 2023;38:241-51.
33. Zhu GN, Hu J, Ren H. A fuzzy rough number-based AHP-TOPSIS for design concept evaluation under uncertain environments. Appl Soft Comput 2020;91:106228.
34. Wu X, Zhu Z, Chen C, Chen G, Liu P. A monotonous intuitionistic fuzzy TOPSIS method under general linear orders via admissible distance measures. IEEE Trans Fuzzy Syst 2023;31:1552-65.
35. Bringas EN, Bowles G, Walker GH. Supporting complex decision making in learning space design: WDA-ANP, a novel sociotechnical systems approach. Facilities 2022;40:435-51.
36. Wang S, Wang S, Liu J. Life-cycle green-house gas emissions of onshore and offshore wind turbines. J Clean Prod 2019;210:804-10.
37. Schreiber A, Marx J, Zapp P. Comparative life cycle assessment of electricity generation by different wind turbine types. J Clean Prod 2019;233:561-72.
38. Gao C, Na H, Song K, et al. Environmental impact analysis of power generation from biomass and wind farms in different locations. Renew Sustain Energy Rev 2019;102:307-17.
39. Yang W, Zhang J. Assessing the performance of gray and green strategies for sustainable urban drainage system development: a multi-criteria decision-making analysis. J Clean Prod 2021;293:126191.
40. Yang Z, Shang WL, Zhang H, Garg H, Han C. Assessing the green distribution transformer manufacturing process using a cloud-based q-rung orthopair fuzzy multi-criteria framework. Appl Energy 2022;311:118687.
41. Cui Y, Yang L, Shi L, Liu G, Wang Y. Cleaner production indicator system of petroleum refining industry: from life cycle perspective. J Clean Prod 2022;355:131392.
42. Yadegaridehkordi E, Hourmand M, Nilashi M, et al. Assessment of sustainability indicators for green building manufacturing using fuzzy multi-criteria decision making approach. J Clean Prod 2020;277:122905.
43. van Hagen L, Petrick K, Wilhelm S, Schmehl R. Life-cycle assessment of a multi-megawatt airborne wind energy system. Energies 2023;16:1750.
44. Wu Y, Tao Y, Zhang B, Wang S, Xu C, Zhou J. A decision framework of offshore wind power station site selection using a PROMETHEE method under intuitionistic fuzzy environment: a case in China. Ocean Coast Manage 2020;184:105016.
45. Chunhua F, Shi H, Guozhen B. A group decision making method for sustainable design using intuitionistic fuzzy preference relations in the conceptual design stage. J Clean Prod 2020;243:118640.
46. Bilgili F, Zarali F, Ilgün MF, Dumrul C, Dumrul Y. The evaluation of renewable energy alternatives for sustainable development in Turkey using intuitionistic fuzzy-TOPSIS method. Renew Energy 2022;189:1443-58.
47. Chen CH. A hybrid multi-criteria decision-making approach based on ANP-entropy TOPSIS for building materials supplier selection. Entropy 2021;23:1597.
48. Saaty TL. Decision making - the analytic hierarchy and network processes (AHP/ANP). J Syst Sci Syst Eng 2004;13:1-35.
49. Li TC, Zhang HQ, Du JG, Qian J. Evaluating the ability of new ship maintenance based on super decision software. In: 2020 International Conference on Wireless Communications and Smart Grid (ICWCSG); 2020 Jun 12-14; Qingdao, China. IEEE; 2020. pp. 484-7.
50. Memari A, Dargi A, Akbari Jokar MR, Ahmad R, Abdul Rahim AR. Sustainable supplier selection: a multi-criteria intuitionistic fuzzy TOPSIS method. J Manuf Syst 2019;50:9-24.
51. Chen L, Yu H. Emergency alternative selection based on an E-IFWA approach. IEEE Access 2019;7:44431-40.
Cite This Article
Export citation file: BibTeX | RIS
OAE Style
Zhou J, Wang L, Li F, Ge C, Li J, Wang Y, Kong L, Guo J. A life cycle decision framework of China offshore wind turbines with ANP-Intuitionistic fuzzy TOPSIS method. Green Manuf Open 2024;2:3. http://dx.doi.org/10.20517/gmo.2023.081802
AMA Style
Zhou J, Wang L, Li F, Ge C, Li J, Wang Y, Kong L, Guo J. A life cycle decision framework of China offshore wind turbines with ANP-Intuitionistic fuzzy TOPSIS method. Green Manufacturing Open. 2024; 2(1): 3. http://dx.doi.org/10.20517/gmo.2023.081802
Chicago/Turabian Style
Zhou, Jiaxuan, Liming Wang, Fangyi Li, Chunli Ge, Jianfeng Li, Yitong Wang, Lin Kong, Jing Guo. 2024. "A life cycle decision framework of China offshore wind turbines with ANP-Intuitionistic fuzzy TOPSIS method" Green Manufacturing Open. 2, no.1: 3. http://dx.doi.org/10.20517/gmo.2023.081802
ACS Style
Zhou, J.; Wang L.; Li F.; Ge C.; Li J.; Wang Y.; Kong L.; Guo J. A life cycle decision framework of China offshore wind turbines with ANP-Intuitionistic fuzzy TOPSIS method. Green. Manuf. Open. 2024, 2, 3. http://dx.doi.org/10.20517/gmo.2023.081802
About This Article
Copyright
Data & Comments
Data
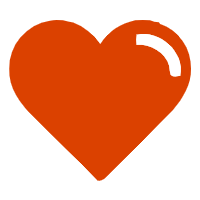

Comments
Comments must be written in English. Spam, offensive content, impersonation, and private information will not be permitted. If any comment is reported and identified as inappropriate content by OAE staff, the comment will be removed without notice. If you have any queries or need any help, please contact us at support@oaepublish.com.