State of the art in determining the optimal reverse logistics network for your product
Abstract
The primary focus of this study is to explore and answer the following research question: “In what ways can organizations enhance the efficiency and responsiveness of their reverse logistics network design?” The study presents a conceptual framework that the authors have built, incorporating several essential variables to guide the process of network design. The conceptual framework for reverse logistics networks has been developed by conducting an analysis of the fundamental factors that influence network design decisions. This paradigm facilitates the decision-making process for firms when considering the centralization or decentralization of their reverse logistics operations and the outsourcing or insourcing of specific operational aspects. Moreover, the authors have discovered and highlighted relevant literature that exhibits strong alignment with our proposed framework, hence offering useful insights for decision-making in the domain of reverse logistics network design. Within our framework, two crucial dimensions are considered at each stage: the choice between internal processing vs. outsourcing and the decision to centralize or decentralize operations. With consideration of the factors noted above, this paper proposes a mixed integer linear programming model for the design of a reverse logistics network. This model aims to minimize the total cost at each stage of reverse logistics operations.
Keywords
INTRODUCTION
Reverse logistics is the process of planning, implementing, and controlling the efficient, cost-effective flow of raw materials, in-process inventory, finished goods, and related information from the point of consumption to the point of origin for the purpose of recapturing value or proper disposal. It includes the management of return, repair, refurbishment, and recycling activities [Council of Supply Chain Management Professionals (CSCMP)]. Recalls, commercial returns, wrong deliveries, warranties, repairs, and refurbishment or end-of-life returns are some of the examples of reverse logistics that companies need to manage. It has historically been a neglected part of supply chain management but is currently gaining much more attention due to its direct impact on profit margins, companies’ environmental image, and corporate social responsibility. A typical reverse logistics chain process is shown in Figure 1 below.
Much of the reverse logistics work has been focusing on products or industries such as electronics, transportation containers, auto parts, carpet recycling, papers, and computer components. This is mainly due to the efficiency to be gained from product-specific knowledge in recycling or remanufacturing processes[1].
While extensive studies have focused on a particular product or industry, some research has been conducted to tackle the general case for reverse logistics across all products and industries. Fleischmann et al. identified characteristics of product recovery networks by dividing them into three types (bulk recycling, assembly product remanufacturing, and re-usable items) and then classifying the network characteristics within each group such as dedicated facilities, reuse in the original market, and mandatory recovery[1,2]. Solutions have concentrated on mixed-integer linear programming (LP) models for network design, largely deterministic facility location allocation models. Recently, models have begun to incorporate stochastic programming and robust optimization approaches to address uncertainties in the quality and quantity of return products[3,4].
There are many trade-offs that must be considered for efficient reverse logistics networks. Among these trade-offs are centralized vs. decentralized sorting and testing, dedicated disassembly plants vs. in-plant remanufacturing, and company-specific vs. industry-wide collection systems. He et al. investigate how competitive collection and channel convenience drive the remanufacturing and recycling to be more sustainable[5]. Previously, researchers have used case studies to develop reverse production classifications but have not addressed specific trade-offs that must be considered for network design[6,7].
Nowadays, under globalization’s implications, designing or building operating networks is a must for the companies to expand their business globally. These networks enable a company to sell its products to customers around the world while providing the firm access to worldwide resources. Thus, combining forward logistics and reverse logistics within network design becomes essential for achieving better performance. Four critical areas - compatibility, configuration, coordination, and control - should be evaluated to make sure that each network design decision yields a more competitive network.
In this research, the authors will address decisions in the design of reverse logistics networks and propose a framework for evaluating the necessary trade-offs in network design. It will also include factors that have impacts on network design decisions in reverse logistics.
Significance of the research
This research compiles numerous of publications from top journals, case studies, and seminar reports in Reverse Logistics and Network Design. It gives us the opportunity to put together a list of key decision factors on Reverse Logistics Network Design so that we can propose a mathematical model for reverse logistics networks to help companies make better decisions in designing their reverse logistics operations.
Research questions and objectives
The ultimate aim of the research project is to provide a framework to recommend to organizations or companies on how they could design an appropriate network for their reverse logistics to achieve their organizational objectives. In order to make these recommendations, the pros and cons of each network configuration will be evaluated to align with the business objective.
The objectives of this research are to:
• Identify key factors related to Network Design Decisions in Reverse Logistics for all supply chains;
• Propose a framework to recommend a proper Network Design Decision in related industries and situations;
• Propose a mathematical model to optimize its reverse logistics network.
In short, this research attempts to answer the following questions:
• What are the key factors in deciding on Reverse Logistics networks?
• How many types of Reverse Logistics Network Designs are there? What are the advantages and disadvantages of each type?
• Which situations or industries will be suitable for each Reverse Logistics Network Design?
Research methodology
The research methodology employed in this study involves the utilization of secondary data to identify the factors influencing reverse logistics network design. Furthermore, examples are employed to evaluate the efficacy of the conceptual framework, following these steps:
1. Identification of key activities or processes for Reverse Logistics operations based on past research: this includes the examination of activities such as collection, sorting and testing, processing of returns, and inventory storage.
2. Evaluation of pros and cons for each network configuration in various scenarios: the study assesses the advantages and disadvantages associated with different network configurations that are suitable for specific circumstances.
3. Propose of a conceptual framework for designing reverse logistics networks: a conceptual framework is developed based on the identified factors and considerations.
4. Develop a mathematical model based on the conceptual framework using mixed integer LP.
By employing secondary data analysis, conducting scenario-based evaluations, and proposing a mathematical model, this research aims to provide valuable insights into the design of reverse logistics networks.
LITERATURE REVIEW
Reverse logistics, the process of managing the flow of products from end-users back to the point of origin for recovery, recycling, or proper disposal, has garnered increasing attention in recent years owing to its potential economic and environmental benefits. To design efficient and sustainable reverse logistics networks, researchers and practitioners have delved into various quantitative models, optimization techniques, and case studies. This literature review provides an overview of key research in the field, drawing insights from prominent references.
Quantitative models and optimization techniques for reverse logistics
Fleischmann et al. conducted a comprehensive review of quantitative models for reverse logistics, addressing complexities such as multiple returns channels, uncertain product conditions, and heterogeneous product flows[2]. This study emphasized the need for analytical tools to optimize reverse logistics processes effectively. Srivastava focused on network design optimization for reverse logistics, including facility locations, transportation routes, and inventory management[8]. The approach sought to minimize costs and environmental impact while maximizing service levels in reverse logistics networks.
Sabri et al. presented a multi-objective approach for simultaneous strategic and operational planning in supply chain design, encompassing both forward and reverse logistics activities[9]. Their model aimed to strike a balance between minimizing costs and maximizing customer service levels in reverse logistics. Jayaraman et al. proposed a simulated annealing methodology for distribution network design, offering a robust optimization approach to tackle the complexities of reverse logistics and effectively manage reverse flows in supply chains[10].
Amin et al. developed a multi-objective facility location model for closed-loop supply chain networks under uncertain demand and return scenarios, providing valuable insights into managing uncertainties in reverse logistics networks[11].
Wang et al. investigate the relationship between enterprises’ green growth model and value chain reconstruction[12]. Agrawal et al. addressed critical aspects related to circular supply chains in a systematic literature review[1]. Their research shed light on adoption and implementation, forecasting product returns, outsourcing, reverse logistics networks from a secondary market standpoint, and disposition decisions, offering insights into the conceptualization and examination of circular supply chain challenges.
Advanced optimization approaches for reverse logistics
Pishvaee et al. proposed a robust possibilistic programming approach for socially responsible supply chain network design, emphasizing the importance of sustainability in reverse logistics network design, and employed an accelerated Benders decomposition algorithm for sustainable supply chain network design under uncertainty, with a case study in the pharmaceutical industry highlighting the significance of considering uncertainties in reverse logistics[13].
Van Engeland et al. provided a comprehensive examination of reverse logistics and waste management by analyzing relevant literature on network design within the waste reverse supply chain domain[4]. Their research highlighted the significance of considering factors such as multi-objective and multi-level uncertainty when designing networks for reverse supply chains.
In summary, the reviewed literature showcases the evolving field of reverse logistics network design, covering quantitative models, optimization techniques, case studies, sustainability considerations, and uncertainty management. The insights from these references contribute to enhancing the efficiency and sustainability of reverse logistics practices, leading to improved economic and environmental outcomes. Further research in this area is crucial to continuously advance the field of reverse logistics network design and its impact on supply chain management and sustainability.
Key processes of reverse logistics
Reverse logistics encompasses a wide range of items and activities, such as:
• Movement of capital items and equipment to the next emergency response.
• Removal of containers and packaging from the response area.
• Destruction of spoiled food commodities and expired pharmaceuticals.
• Return of rejected goods to suppliers.
• Movement of excess or over-supplied goods to other programs or organizations.
Considering the following questions before designing any logistics network is crucial:
1. What logistics activities and recovery processes are involved?
2. Which parties are responsible for performing the logistics activities?
3. Where should the logistics activities be carried out?
Tan et al. conducted a study on a US-based computer company’s Asia-Pacific operations and identified inefficiencies and high costs in their reverse logistics programs[14]. As a result, they developed a decision model to assist practitioners in cost control and profit maximization in potential reverse logistics activities. The costs discussed by them include transportation, customs duty, acquisition, handling, repair, reuse, scrap, storage, and freight costs.
Furthermore, Guide et al. framework addresses expected costs such as remanufacturing costs, logistics costs, machine and labor costs, the value of time (e.g., opportunity costs), costs of lost sales, and inspection costs[7]. These are just a few examples of the key costs evaluated when determining the appropriate reverse logistics disposition. Various costs associated with reverse logistics must be taken into account when deciding on a disposition option, and these costs may influence a firm’s choice of alternatives.
In the context of reverse logistics, Barker emphasizes four fundamental stages of flow[15]: collection, sort-test, processing, and storage[2,16-18] highlights the decisions companies face regarding the collection of recoverable products from former users, the inspection of collected products to separate recoverable resources from worthless scrap, the processing of collected products to make them marketable, and the distribution of recovered products to future customers.
Barker proposes a product recovery flow diagram depicting the four stages [Figure 2][15,17]. After the collection and sorting/testing stages, the product undergoes processing, which may involve finished product reuse, remanufacturing and spare parts recovery, reprocessed raw materials, and waste disposal. The products are then either centrally stored or returned to the original source.
Stage 1 (Collection): In the collection stage of reverse logistics, companies must decide whether to use a proprietary or industry-wide collection system. A proprietary system involves collecting a company’s own products for recovery, which can strengthen customer relationships but may have higher transportation costs. Companies can use their own transportation or outsource to third-party logistics providers. On the other hand, industry-wide collection systems involve multiple producers’ products and are more cost-effective due to economies of scale but offer less control to individual companies. Proprietary systems are common in remanufacturing, while industry-wide systems are used for commodity-type products.
Stage 2 (Sort and test): Sorting and testing are essential in managing and profiting from the reverse flow. Companies often encounter issues with lenient return policies and customer errors. Sorting and testing can be done centrally or in distributed locations. Central sorting is efficient for high-volume and costly testing procedures but may result in higher transportation costs. Distributed sites are used for low-cost testing procedures but require consistent and reliable testing methods and can complicate the network due to separate scrap and return product streams.
Stage 3 (Processing): After sorting and testing, the key decision in the processing stage of reverse logistics is whether to reprocess at the original facility or at a secondary facility. Reprocessing at the original facility is efficient and suitable for machine remanufacturing or spare parts recovery but may require increased processing capacity. In zero return programs, products do not come back through the return channel, which can negatively affect customer satisfaction and brand. Processing at a secondary facility or outsourcing to a third party offers economies of scale for bulk commodity-type products but requires establishing separate facilities with potential efficiency loss.
Stage 4 (Storage): Once products have been processed, they are stored either centrally or closer to the source of returns. Slow-moving products are stored centrally when there is no market demand, while high-demand products are distributed nearer to customers. Effective storage management is crucial in reverse logistics to ensure that products are available when needed.
In summary, these four stages (Collection, Sort and Test, Processing, and Storage) represent key components of the reverse logistics process, which involves managing the flow of products and materials from the end consumer back through the supply chain for recovery and reuse. Figure 3 describes the flow activities in Reverse Logistics[19].
Key factors to consider in reverse logistics network design
Centralized vs. decentralized processing
Centralized processing, a long-standing practice in reverse logistics, has recently gained greater attention from manufacturing companies. In this system, all products within the reverse logistics pipeline are brought to a central facility for sorting, processing, and subsequent shipment to their next destinations. Centralization offers the advantage of efficiently managing the reverse logistics flow, potentially leading to increased revenues from returned items. Moreover, it aids in determining the most appropriate reverse channel for handling returns.
On the contrary, a decentralized system is more suitable when products are returned directly from consumers to retailers. In this scenario, individual sales outlets serve as gatekeepers for processing returns.
The choice between centralized and decentralized approaches in reverse logistics network design hinges on industry-specific and geographic factors. Considerations encompass the product lifecycle, product value and cost, return volume, and the geographic distribution of returns. Ultimately, the decision must be based on a comprehensive evaluation of these factors, allowing manufacturers to determine the most effective processing approach based on their unique circumstances and objectives [Figure 4].
In-house vs. third-party logistics providers
The decision between insourcing and outsourcing in reverse logistics network design is pivotal. In insourcing, a company handles the entire reverse logistics process, including material reuse. In contrast, outsourcing involves third-party providers taking on various aspects of reverse logistics. Companies may choose outsourcing due to resource constraints or lack of expertise, with the extent of outsourcing varying based on factors such as personnel skills and cost considerations. Successful third-party logistics providers (3PLs) in reverse logistics, such as FedEx, Genco, and ASTAR, offer outsourcing options. The choice to outsource also depends on the type of product and industry; more specialized activities, such as remanufacturing, tend to remain in-house, while less specialized tasks, such as recycling, can be outsourced. Partner selection is critical in outsourcing, focusing on capabilities, track record, and alignment with the company’s goals. As we examine 3PLs, they face the challenge of designing adaptable networks to meet diverse client needs, but efficiency can lead to economies of scale and scope[1,20,21].
Summary of literature review
The literature review highlights key considerations in designing effective reverse logistics operations. Companies should assess whether to centralize or decentralize processing, decide on outsourcing or insourcing, and consider consolidating shipments or direct shipping between stages. These choices influence costs, delivery time, product shelf life, and return value. This is a research gap that the authors would like to investigate in this study. Furthermore, aligning the reverse logistics network design with the overall business strategy is essential for success[7].
METHODS
Introduction and justification
The aim of this research is to address the critical need for designing efficient reverse logistics networks that align with organizational objectives. Reverse logistics has gained prominence due to its environmental and economic implications, making network design decisions pivotal for companies. This research aims to develop a comprehensive framework to guide organizations in making informed network design decisions.
Research objectives and hypotheses
Our primary research objectives are:
1. To identify and analyze key factors influencing network design decisions in the context of reverse logistics.
2. To propose a robust conceptual framework that assists organizations in making appropriate network design decisions.
3. To develop a mathematical model based on the framework to optimize reverse logistics network design.
Key factors identification
The authors have employed a mixed-methods approach to identify the key factors affecting network design decisions in reverse logistics. This approach involves an in-depth literature review and expert interviews. The literature review will delve into works related to reverse logistics, network design, and their interplay. Expert interviews with professionals in the field will provide insights into real-world challenges and factors that influence network design.
Conceptual framework development
Building on insights gathered from the literature review and expert interviews, the authors have developed a comprehensive conceptual framework. This framework will capture the multifaceted considerations involved in network design decisions, including economic, environmental, and operational factors. It will also account for the dynamic nature of industries and situations.
Mathematical model and optimization
Based on the conceptual framework, the authors have constructed a mathematical model that optimizes reverse logistics network design decisions. The model will consider key factors identified in the framework, such as transportation costs, facility capacities, and lead times. The authors have employed mixed LP to find the optimal solutions that minimize costs while meeting organizational objectives.
Validity and reliability
To ensure the validity and reliability of our findings, the authors employ triangulation by using multiple data sources, employing different methods of data collection, and involving experts in validating the proposed framework and model. Peer reviews and feedback from advisors will further contribute to enhancing the credibility of our research.
Ethical considerations
Ethical considerations will be prioritized throughout the research process. Informed consent will be obtained from participants involved in interviews and case studies. Confidentiality of sensitive data will be maintained, and ethical guidelines set by relevant institutions will be strictly adhered to.
Limitations
The authors acknowledge potential limitations such as the availability of accurate data from industry partners and the inherent complexities of real-world logistics scenarios. These limitations will be transparently communicated in the research findings.
In conclusion, our research methodology adopts a comprehensive approach, encompassing literature review, expert insights, conceptual framework development, mathematical modeling, case studies, and rigorous analysis. This holistic approach ensures the reliability and relevance of our research outcomes, contributing to the advancement of reverse logistics network design practices.
DISCUSSION
In this section, a conceptual framework for designing a reverse logistics network is presented. The framework, illustrated in Figure 5, has been developed through a combination of insights from the literature review and information gathered from company websites. This framework encompasses four key stages in the selection of reverse logistics operations modes: (1) Collection; (2) Sorting and testing; (3) Processing; and (4) Storage.
There are two dimensions to consider at each stage in designing the network, namely, Internal processing vs. outsourcing and centralizing vs. decentralizing of operations.
Table 1 shows some of the previous research works on reverse logistics with different network designs from stage 1 to 4.
Summary of case studies on reverse logistics processes
Case study | Stage 1: Collection | Stage 2: Sort/Test | Stage 3: Processing | Stage 4: Storage |
Brandão et al.[22] | Waste removal from relatively few construction sites | Sorted at the central sorting facility into clean, half-clean, and polluted sand | Polluted sand cleaned at a central facility, and final products are clean and half-clean sand | Centralized storage due to its bulkiness |
Fleischmann et al.[18] | Collection from business customers on expiration of lease contracts | Sorting and testing at central disassembly centers | Repair at disassembly centers; final products are refurbished machines and spare parts | Centralized storage of spare parts to reduce inventory costs |
Hong et al.[23] | Collection at municipal and non-profit sites from residential customers | Sorting at distributed collection sites | Commercial processing sites process for recycling. Scrap sent to commercial processing sites | Centralized storage due to its bulkiness |
Islam et al.[24] | Collection of e-waste after the end of life | Sorting at the collection sites | Electronic parts are recycled or disposed of | Centralized storage of electronic wastes |
Mahmoodi et al.[20] | Collection of solar panels after the end of life | Sorting at the collection sites | Usable parts are recycled or disposed of | Centralized storage of recycled parts |
MATHEMATICAL MODEL FOR THE PROPOSED FRAMEWORK
The mathematical model for the proposed framework
This model can be used to determine the optimal quantities to be collected, sorted, processed, and stored at each station while making outsourcing/insourcing decisions and satisfying the responsiveness requirements for n number of stations in the manufacturing process. The specific values of the parameters (e.g., costs, capacities, and time thresholds) and constraints will depend on the company’s resources and business requirements.
Notation:
• Ii: total number of stations at stage i, i = 1, 2, 3, 4.
• T0: maximum turnaround time requirement/quota from the beginning of stage 1 to the end of stage 4.
• C0: penalty cost if the total turnaround time exceeds the maximum time requirement T0.
Decision variables:
• Xi1: Quantity of components collected at collection station i (in units), where i ∈ I1
• Xi2: Quantity of components sent to sorting and testing station i (in units), where i ∈ I2
• Xi3: Quantity of components processed at processing station i (in units), where i ∈ I3
• Xi4: Quantity of components stored at storage station i (in units), where i ∈ I4
• Yi1: Binary decision variable for outsourcing at collection station i, where Yi1 = 1 represents outsourcing, and Yi1 = 0 represents insourcing for i ∈ I1
• Yi2: Binary decision variable for outsourcing at sorting and testing station i, where Yi2 = 1 represents outsourcing, and Yi2 = 0 represents insourcing for i ∈ I2
• Yi3: Binary decision variable for outsourcing at processing station i, where Yi3 = 1 represents outsourcing, and Yi3 = 0 represents insourcing for i ∈ I3
• Yi4: Binary decision variable for outsourcing at storage station i, where Yi4 = 1 represents outsourcing, and Yi4 = 0 represents insourcing for i ∈ I4
• Ti1: Time taken to collect the items at collection station i (in some time unit), where i ∈ I1
• Ti2: Time taken to sort and test the items at sorting and testing station i (in some time unit), where i ∈ I2
• Ti3: Time taken to process the items at processing station i (in some time unit), where i ∈ I3
• Ti4: Time taken to store the items at storage station i (in some time unit), where i ∈ I4
Objective Function (Minimize Cost and Penalty):
Here, [·]+ is the plus function defined by [t]+ = max{t,0} for any t ∈ R. In the objective function above, the summation of the first four items denotes the total operation cost. The last part denotes the penalty cost when the total time taken over the four stages exceeds the required time. For convenience in description and without loss of generality, in what follows, we assume that |I1| = |I2| = |I3| = |I4| = n. That is, there are n stations at each stage.
Constraints:
• Number of Collection Stations Constraint:
• Number of Sorting and Testing Stations Constraint:
• Number of Processing Stations Constraint:
• Number of Storage Stations Constraint:
• Storage Capacity Constraint:
Responsiveness Constraints:
•
•
•
•
Outsourcing Constraints:
• Yi1 ∈ {0,1} for i = 1, ..., n
• Yi2 ∈ {0,1} for i = 1, ..., n
• Yi3 ∈ {0,1} for i = 1, ..., n
• Yi4 ∈ {0,1} for i = 1, ..., n
Non-negativity Constraints:
Xi1, Xi2, Xi3, Xi4, Ti1, Ti2, Xi3, Ti4 ≥ 0 for i = 1, ..., n
This model can be used to determine the optimal quantities to be collected, sorted, processed, and stored at each station while making outsourcing/insourcing decisions and satisfying the responsiveness requirements for the underlying stations in the manufacturing process. The specific values of the parameters (e.g., costs, capacities, and time thresholds) and constraints will depend on the company’s resources and business requirements.
Note also that the objective function is nonlinear due to the involvement of the plus function [·]+. Then, the proposed model is a nonlinear optimization model with linear constraints, non-negativity constraints, and outsourcing constraints (i.e., 0-1 binary) concerning decision variables. To deal with the nonlinearity of the objective function and ease numerical computation, we introduce an auxiliary variable ϒ. Specifically, we reformulate the objective function Z as:
where
Clearly, the inequality constraint above is linear in Ti1, Ti2, Ti3, Ti4, and ϒ. Also, the objective function Ẑ is a linear function. Incorporating the above two constraints into the constraints discussed previously, the new model transforms into a linear optimization problem subject to the underlying linear inequality constraints, 0-1 binary, and nonnegativity constraints as discussed above. This model can be solved effectively using professional software such as Matlab and Gurobi, for instance.
Numerical example
Let us assume there are three stations available for each type: collection, sorting and testing, processing, and storage (i.e., n = 3).
Given data:
• Ci1 = 10, Ci2 = 15, Ci3 = 8, Ci4 = 5 (Cost coefficients for each type of station at station i)
• Capacity_Storage = 50 (Storage capacity at each storage station)
• Tmax_Collection = 15, Tmax_SortingTesting = 20, Tmax_Processing = 10, Tmax_Storage = 25 (Maximum time allowed for each type of station)
Solution: Let us assume the LP model provides the following solution:
•
•
•
•
•
•
•
•
Objective function value:
Substituting these values into the objective function:
Z =
In this example, the value of the objective function Z represents the total cost and time required based on the given solution. The values of decision variables and constraints reflect the decisions made for each station and the satisfaction of various requirements (e.g., time limits and capacities). The goal of the LP model is to find such values of decision variables that minimize the objective function while satisfying the given constraints.
Sensitivity analysis involves exploring how changes in the coefficients of the objective function or the right-hand sides of the constraints affect the optimal solution. Here, we will demonstrate a simple sensitivity analysis by adjusting the costs Ci1, Ci2, Ci3 and Ci4 in the objective function and keep the other values unchanged from the previous example for the sake of simplicity.
Let us consider the original costs:
• Ci1 = 10
• Ci2 = 15
• Ci3 = 8
• Ci4 = 5
Now, let us examine the impact of a change in the costs by increasing them:
New costs:
• Ci1 = 12
• Ci2 = 18
• Ci3 = 10
• Ci4 = 8
Let us recalculate the objective function value using these new costs:
Z = (12·20·0) + (18·10·1) + (10·25·0) + (8·30·1) + 10 + 8 + 6 + 20 + (18·20·1) + (10·15·0) + (8·40·1) + 12 + 10 + 5 + 18 + (12·25·0) + (18·15·1) + (10·10·1) + (8·25·0) + 9 + 7 + 4 + 15 = 590
Comparing this result with the original objective function value of 508, we can observe that increasing the costs has led to an increase in the total cost and time. This demonstrates the sensitivity of the optimal solution to changes in the costs associated with different stations. This sensitivity analysis highlights how changes in the costs can influence the overall cost and time of the network design. It is important to consider these results in the context of your business goals and constraints when making decisions based on the model.
CONCLUSION
In this study, we have examined and analyzed in depth each of the most important aspects of reverse logistics network design. On the basis of our findings, we propose a comprehensive conceptual framework that can help companies make informed decisions regarding the centralization or decentralization, outsourcing or insourcing, and use of open or closed-loop supply chains for their reverse logistics networks.
The conceptual framework that has been created encompasses four distinct processes within reverse logistics activities, namely collection, sorting and testing, processing, and storing. Within our framework, two crucial dimensions are considered at each stage: the choice between internal processing vs. outsourcing and the decision to centralize or decentralize operations. With consideration of the factors noted above, this paper proposes a mixed integer LP model for the design of a reverse logistics network, which considers the minimization of the total cost at each stage in reverse logistics operations.
Future research
Our current research only makes use of data from a company to study its fitness. Thus, the framework has not been tested widely or become generalized. Future research could study more industries to verify and further improve the model to incorporate stochastic programming and robust optimization approaches to address uncertainties in the quality and quantity of return products.
DECLARATIONS
Acknowledgments
The authors express their sincere gratitude to Dr. Meng Fanwen, their former colleague at The Logistics Institute of Asia Pacific, for the invaluable feedback and insightful suggestions that substantially enhanced the mixed-integer programming model in the research. Dr. Fanwen’s expertise and contributions have significantly enriched the quality of the work. The mathematical model is an extension of the working paper published in MIT-DSpace by Dr. Albert Tan and Dr. Janya.
Authors’ contributions
Developed the mathematical model and test the model using an example: Tan AWK
Proposed a conceptual framework for reverse logistics networks: Chanchaichujit J
Conducted a literature review and summarized the findings of this research: Kwa PTH
Availability of data and materials
Data are generated to test the model.
Financial support and sponsorship
None.
Conflicts of interest
All authors declared that there are no conflicts of interest.
Ethical approval and consent to participate
Not applicable.
Consent for publication
Not applicable.
Copyright
© The Author(s) 2023.
REFERENCES
1. Agrawal S, Singh RK, Murtaza Q. A literature review and perspectives in reverse logistics. Resour Conserv Recycl 2015;97:76-92.
2. Fleischmann M, Krikke HR, Dekker R, Flapper SDP. A characterisation of logistics networks for product recovery. Omega 2000;28:653-66.
3. Hsuan J, Skjøtt-Larsen T, Kinra A, Kotzab H. Managing the global supply chain. 4th ed. Copenhagen Business School Press; 2015. Available from: https://research.cbs.dk/en/publications/managing-the-global-supply-chain. [Last accessed on 12 Dec 2023].
4. Van Engeland J, Beliën J, De Boeck L, De Jaeger S. Literature review: strategic network optimization models in waste reverse supply chains. Omega 2020;91:102012.
5. He Q, Wang N, Browning TR, Jiang B. Competitive collection with convenience-perceived customers. Eur J Oper Res 2022;303:239-54.
6. Govindan K, Soleimani H, Kannan D. Reverse logistics and closed-loop supply chain: a comprehensive review to explore the future. Eur J Oper Res 2015;240:603-26.
7. Guide VD Jr, Pentico D. A hierarchical decision model for re-manufacturing and re-use. Int J Logist Res Appl 2003;6:29-35.
9. Sabri EH, Beamon BM. A multi-objective approach to simultaneous strategic and operational planning in supply chain design. Omega 2000;28:581-98.
10. Jayaraman V, Ross A. A simulated annealing methodology to distribution network design and management. Eur J Oper Res 2003;144:629-45.
11. Amin SH, Zhang G. A multi-objective facility location model for closed-loop supply chain network under uncertain demand and return. Appl Math Model 2013;37:4165-76.
12. Wang N, He Q, Jiang B. An overview of enterprises’ green growth model and value chain reconstruction. In: Enterprises’ green growth model and value chain reconstruction. Singapore: Springer; 2022. pp. 1-27.
13. Pishvaee MS, Razmi J, Torabi SA. Robust possibilistic programming for socially responsible supply chain network design: a new approach. Fuzzy Sets Syst 2012;206:1-20.
14. Tan AWK, Kumar A. A decision-making model for reverse logistics in the computer industry. Int J Logis Manag 2006;17:331-54.
15. Barker TJ. Reverse logistics: a multicriteria decision model with uncertainty. 2010. Available from: https://www.proquest.com/openview/45057320471a11ae55ead6109334af44/1?pq-origsite=gscholar&cbl=18750. [Last accessed on 12 Dec 2023].
16. de Brito MP, Dekker R, Flapper SDP. Reverse logistics: a review of case studies. In: Fleischmann B, Klose A, editors. Distribution logistics. Lecture notes in economics and mathematical systems. Berlin, Heidelberg: Springer; 2005. pp. 243-81.
17. Zhang X, Zou B, Feng Z, Wang Y, Yan W. A review on remanufacturing reverse logistics network design and model optimization. Processes 2022;10:84.
18. Fleischmann M, Bloemhof-Ruwaard JM, Dekker R, van der Laan E, van Nunen JAEE, Van Wassenhove LN. Quantitative models for reverse logistics: a review. Eur J Oper Res 1997;103:1-17.
19. Barker TJ, Zabinsky ZB. Reverse logistics network design: a conceptual framework for decision making. Int J Sustain Eng 2008;1:250-60.
20. Mahmoudi S, Huda N, Alavi Z, Islam MT, Behnia M. End-of-life photovoltaic modules: a systematic quantitative literature review. Resour Conserv Recycl 2019;146:1-16.
21. Cheong M. New models in logistics network design and implications for 3PL companies. 2005. Available from: https://www.semanticscholar.org/paper/New-Models-in-Logistics-Network-Design-and-for-3PL-Cheong/ff4b1323ac69d73d75468135c909855c4cc6254b. [Last accessed on 12 Dec 2023].
22. Brandão R, Edwards DJ, Hosseini MR, Silva Melo AC, Macêdo AN. Reverse supply chain conceptual model for construction and demolition waste. Waste Manag Res 2021;39:1341-55.
23. Hong IH, Assavapokee T, Ammons J, et al. Planning the e-scrap reverse production system under uncertainty in the state of Georgia: a case study. IEEE Trans Electron Packag Manufact 2006;29:150-62.
Cite This Article
Export citation file: BibTeX | RIS
OAE Style
Tan AWK, Kwa PTH, Chanchaichujit J. State of the art in determining the optimal reverse logistics network for your product. Green Manuf Open 2024;2:1. http://dx.doi.org/10.20517/gmo.2023.080501
AMA Style
Tan AWK, Kwa PTH, Chanchaichujit J. State of the art in determining the optimal reverse logistics network for your product. Green Manufacturing Open. 2024; 2(1): 1. http://dx.doi.org/10.20517/gmo.2023.080501
Chicago/Turabian Style
Tan, Albert Wee Kwan, Philip Teow Huat Kwa, Janya Chanchaichujit. 2024. "State of the art in determining the optimal reverse logistics network for your product" Green Manufacturing Open. 2, no.1: 1. http://dx.doi.org/10.20517/gmo.2023.080501
ACS Style
Tan, AWK.; Kwa PTH.; Chanchaichujit J. State of the art in determining the optimal reverse logistics network for your product. Green. Manuf. Open. 2024, 2, 1. http://dx.doi.org/10.20517/gmo.2023.080501
About This Article
Special Issue
Copyright
Data & Comments
Data
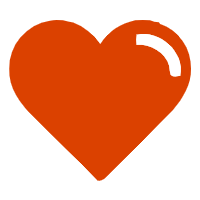

Comments
Comments must be written in English. Spam, offensive content, impersonation, and private information will not be permitted. If any comment is reported and identified as inappropriate content by OAE staff, the comment will be removed without notice. If you have any queries or need any help, please contact us at support@oaepublish.com.