Review and challenges for the remanufacturing assembly quality with uncertainty
Abstract
Remanufacturing businesses have difficulty in competing and expanding due to the unpredictable quality and performance of remanufactured products. It has turned into a problem area and a bottleneck for the growth of the remanufacturing sector. The control and management of remanufactured assembly quality (RAQ) is considered one of the key factors affecting the quality of remanufactured products. By reviewing existing literature, we have found that in remanufacturing assembly systems, the uncertainty of remanufacturing components, the volatility of remanufacturing assembly processes, the complexity of assembly control, and the diversity of assembly schemes are the main reasons for the difficulty in ensuring assembly quality. However, existing literature lacks research on data management, evolutionary mechanisms of RAQ, and multivariate control. To improve the stability of RAQ and improve the quality of remanufactured products, we propose the construction of an intelligent reasoning mode for precise RAQ control and a tailored, intelligent control method for individual components, providing support for the intelligent control of remanufactured assembly functional modules. This study provides a basis for implementing high-performance assembly technology for remanufactured products. Our findings should encourage the industry’s modernization for remanufacturing.
Keywords
INTRODUCTION
To achieve carbon neutrality strategic goals, the manufacturing industry must carry out carbon reduction and decarbonization throughout the industrial chain; thus, it must construct a sustainable industrial green development model[1]. Remanufacturing is widely considered indispensable and important across multiple industries. It involves the revitalization of old machinery and equipment by using them as raw materials and adopting specialized processes and technologies. This process essentially entails carrying out new manufacturing procedures on the basis of the original production methods[2]. Moreover, the remanufactured products are expected to have the same performance or quality as the original new counterparts[3,4]. As a result, remanufacturing can bring energy conservation, emission reduction, and material recycling[5].
Practical data statistics show that the failures caused by the assembly process account for about 46% of the total failures of complex electromechanical products. Unlike the standardization and consistency of original manufacturing components, the control and management of remanufactured assembly quality (RAQ) faces challenges such as uncertainty in the quality of remanufactured components, high-dimensional uncertainty in production data, complex and ever-changing evolution mechanisms, and diversified remanufacturing assembly control[6,7]. These situations result in discrepancies in the quality of remanufactured products. Consumers worry about the reliability of remanufactured products[8,9]. To address the above challenges, ensure high-performance assembly in remanufacturing, and improve the quality of remanufactured products, this article takes remanufacturing assembly systems as the research object, constructs a remanufacturing assembly quality data chain, establishes a quantitative evaluation model for remanufacturing assembly quality, explores the evolution mechanism of remanufacturing assembly quality, proposes a personalized intelligent control method for remanufacturing assembly quality, and designs and develops an intelligent control platform for remanufacturing assembly quality. The aim is to form a theoretical and methodological system for quantitative evaluation, evolution mechanism, and intelligent control to ensure the quality of remanufacturing assembly, providing support for the upgrading and high-quality development of China’s remanufacturing industry.
LITERATURE REVIEW
In recent years, the quality problem of remanufacturing assembly systems has gradually attracted the attention of experts and scholars. Search in the Web of Science database with the keywords Remanufacturing Assembly, Quality, Methods of Remanufacturing Assembly, and Remanufacturing Components, the number of research papers on RAQ has increased year by year [Figure 1]; the average citation frequency of each paper is 9.47, and the h-index is 7.
We have searched and reviewed papers on remanufacturing assembly in high-quality and high-level journals recognized by experts and scholars in SCI, SSCI, EI, IEEE, Xplore, Scopus, and Google Scholar databases as follows:
The uncertainty of remanufacturing assembly systems
The uncertainty of remanufacturing assembly systems poses challenges for quality control. It is highlighted by the randomness of recycled materials and remanufactured parts (quantity, time, and quality), the dynamics of remanufacturing production processes, and the complexity of its testing and evaluation[10]. Regarding research methods, the primary approach is to describe uncertainty qualitatively or quantitatively through models and construct optimization models targeting remanufacturing costs, quality, time, carbon emissions, and customer satisfaction. Mukhopadhyay and Ma used a two-stage random analysis to study optimal procurement strategies and production decision-making of remanufactured systems[11]. Based on defining the uncertainty connotation of remanufactured parts, Liu et al. established an information entropy measurement model for the uncertainty of remanufactured assembly dimensions to improve the accuracy of remanufactured assembly[12]. Kim et al. studied the efficiency of RAQ using priority scheduling methods[13].
Furthermore, Ge et al. constructed an uncertainty measurement model for remanufacturing cylinder and cylinder heads and proposed a gas tightness quality control method based on a BP neural network[14]. To reduce the impact of uncertainty on a remanufacturing production system, Zahraei and Teo applied the balanced production method to provide a new way to optimize recycling and inventory processes of the remanufacturing system[15]. Huang et al. proposed a method of characterizing recovery quality uncertainty based on modal intervals to guide production activities[16]. Liao et al. assessed environmental benefits of remanufacturing under uncertainty and discussed the factors affecting environmental benefits, providing the methodology for assessing the environmental benefits of remanufacturing production systems under uncertainty[17]. To address the uncertainty and high personalization issues in remanufacturing, Huang et al. proposed a method for designing remanufacturing solutions based on incomplete information about used parts[18]. Wang et al. proposed a component-oriented reassembly model to manage uncertainty[19]. Considering revenue, quality, and demand uncertainty, Reddy et al. proposed a scenario-based two-stage stochastic programming for hybrid manufacturing-manufacturing systems[20]. These authors also presented a two-stage stochastic linear model for a make-to-order hybrid manufacturing-remanufacturing production system by integrating capacity and inventory decisions[21]. Information technology, big data technology, and artificial intelligence (AI) technology will enhance opportunities and ideas in research and practice to relieve uncertainty of remanufacturing assembly systems.
The process methods of a remanufacturing assembly system
Process methods are associated with challenges to the ever-changing and dynamic remanufacturing assembly. To enhance the utilization rate of remanufactured parts and remanufacturing assembly accuracy, Muruganantham et al. used experimental design and variance analysis methods to determine the factors that affect the quality of remanufactured components[22]. Liu et al. proposed a quality optimization method for complex mechanical product remanufacturing processes with the assembly deviation degree[23]. They studied the online optimization method for quality control point tolerance zones in the remanufactured assembly process[24]. Shen et al. proposed an RAQ control method with three-dimensional tolerances and verified its effectiveness with an example[25]. Hu et al. proposed a state space model-based optimization control strategy for automotive engine remanufacturing assembly accuracy, improving the efficiency and quality of the assembly[26]. Liu et al. studied an integrated optimization strategy for quality control of remanufactured products, achieving online quality control in the remanufactured assembly process[27]. Luh et al. utilized Lagrange relaxation, stochastic dynamic programming, and heuristic solving methods to minimize total delay, lead time, and inventory holding costs, thereby improving the efficiency of remanufacturing systems[28].
Taking remanufacturing stage cost, quality loss, and process capability as optimization objectives, Chen
Multi-objective optimization of a remanufacturing assembly system
The optimization and merging of remanufacturing assembly groups have been a focus of remanufacturing assembly production management to increase the effectiveness and quality of remanufacturing assembly[36]. For example, Liu et al. proposed a tolerance grading selection procedure for remanufactured parts to increase the quality of remanufactured products[37]. Su et al. established a comprehensive selection model for remanufactured parts and used improved ant colony optimization algorithms to realize the optimal selection and assembly of remanufactured parts to increase the efficiency of remanufactured parts[38]. Considering quality, cost, and resource utilization, Jiang et al. studied an optimization method for remanufacturing machine tools[39]. Xue et al. established a multi-objective optimization model for remanufacturing parts with optimization objectives such as life balance and cost[40]. Chen et al. proposed a research method to restructure waste mechanical equipment components based on non-cooperative games[41]. Guiras et al. studied the optimization problem of remanufacturing assembly systems under changes in the ordering cost and quality of waste products[42].
For multi-objective collaborative optimization of cost and quality in remanufacturing products, Xing et al. constructed a remanufacturing matching optimization model under dimensional accuracy constraints[43]. Considering the selection principles of cost, remanufacturing resource utilization, and carbon emissions, Yin et al. established a selection model based on the NSGA-III algorithm using assembly dimension chain tolerance as a constraint function[44]. Lean remanufacturing has been proven to be an effective tool for improving the performance of remanufactured assembly systems[45]; it can accelerate the remanufactured product delivery process and enhance the quality of remanufactured goods[46]. Fu et al. explored how to reduce projected completion time and overall delay and addressed a stochastic multi-objective integrated disassembly-reprocessing-reassembly scheduling problem[47]. Ahn et al. proposed a mixed integer programming model that combines the remanufacturing decomposition process with the assembly system to optimize the disassembly, remanufacturing, and assembly processes[48]. Reddy and Kumar proposed a two-stage stochastic linear model to optimize remanufacturing assembly systems[49]. To depict the level of uncertainty present in the actual production environment, Guo et al. proposed a mathematical model of the stochastic hybrid production line balancing problem with several objectives[50]. These studies improved the resource efficiency and quality assurance capability of remanufacturing assembly systems.
Intelligent control of quality for remanufacturing assembly
With the rapid progress of the new generation of information technology, big data technology and AI technology have been applied in remanufacturing[51]. The requirement for the remanufacturing business to grow in a high-quality manner underscores the need for the intelligence of the entire system[52].
Some investigators explored the intelligent control of RAQ. For example, Ketzenberg et al. studied the impact of yield information on remanufactured parts[53]. Liu et al. applied the J2EE architecture to develop a dynamic process quality system for the engine remanufacturing assembly process and verified its applicability[54]. To achieve the traceability of remanufacturing processes and quality information, Liu et al. explored the future automotive remanufacturing operation mode based on digital twins[55]. Song et al. studied the re-planning of the remanufacturing workshop production line and introduced modules such as residual life evaluation, additive manufacturing, quality monitoring, and RAQ control[56]. The effects of these innovations on the enterprise were demonstrated; nevertheless, remanufacturing continues to face numerous challenges for its future expansion in China.
Facing uncertainty, complexity, and dynamics is a challenge for intelligent quality control for remanufacturing assembly. Researching and breaking through the disconnectedness between intelligence and remanufacturing assembly is a critical issue; it is one of the impasses that urgently needs to be breached to enhance the core competitiveness of remanufacturing enterprises in China. Compared with existing literature, our study has certain novelty, as shown in Table 1.
Research gap and contribution research
Author(s) | Uncertainty | Process methods | Multi-objective optimization | Intelligent control | Data-driven multivariate control |
Zahraei et al. (2018)[15] | √ | √ | |||
Huang et al. (2020)[18] | √ | √ | √ | ||
Reddy et al. (2021)[20] | √ | √ | |||
Liu et al. (2019)[27] | √ | √ | √ | ||
Chen et al. (2023)[29] | √ | √ | |||
Liu et al. (2022)[34] | √ | √ | √ | ||
Guiras et al. (2018)[42] | √ | √ | |||
Kurilova-Palisaitiene et al. (2018)[46] | √ | √ | |||
Reddy and Kumar (2021)[49] | √ | √ | |||
Song et al. (2023)[56] | √ | ||||
Wurster et al. (2022)[57] | √ | √ | |||
Andersen et al. (2022)[58] | √ | √ | |||
This paper | √ | √ | √ | √ | √ |
RESEARCH GAPS
An in-depth study is required in the following areas to guarantee high-performance assembly in remanufacturing and enhance the quality of remanufactured products:
The data management of RAQ
At present, most scholars have explored the uncertainty of remanufacturing assembly systems from aspects such as remanufacturing assembly component information and remanufacturing quality information. However, they rarely pay attention to remanufacturing assembly quality data. The primary sources of quality data uncertainty for remanufacturing assembly include inconsistent standards, missing data, and higher dimensions. It is necessary to establish a data management system that conforms to the characteristics of quality data for remanufacturing assembly to integrate and proceed with the data and knowledge of a remanufacturing assembly system and unify management and analysis.
The evolution mechanism of RAQ
Relevant scholars have analyzed the quality optimization methods of the remanufacturing assembly process from the aspects of cost, quality, and process capability optimization in the remanufacturing stage, remanufacturing assembly group optimization, and multi-objective collaborative optimization of remanufacturing product cost and quality. Research has shown that the factors affecting the quality of remanufactured assembly are numerous and highly uncertain, resulting in an intricate process for initial assembly for the generation, transmission, and coupling of mistakes. This phenomenon complicates the process of traditional assembly quality models, accurately characterizing the evolution mechanism of the quality for remanufactured assembly. Therefore, it is necessary to mine production data of remanufacturing assembly, enhance the process by which the quality for remanufacturing assembly evolves, and offer theoretical backing for accurate control of that process.
The multivariate control of RAQ
In the context of the information age, the demand for intelligence in remanufacturing and assembly business has increased. Some scholars have explored intelligent control of remanufacturing assembly quality, achieving information plasticity in the remanufacturing process. Compared to traditional manufacturing assembly quality control, the quality control of remanufacturing assembly has more influencing factors, more complex processes, and higher customized requirements. It requires more data and knowledge to meet the diversified needs for adapting to different parts, achieve precise operation of quality control for remanufacturing assembly, support quality measurement, evaluation, and traceability of remanufacturing assembly, ensuring that the quality is stable and reliable for the remanufacturing assembly, and reducing or eliminating doubts and concerns of consumers about quality of remanufactured products.
It must be acknowledged that crucial enabling technology support for attaining high-quality development in the remanufacturing business assures high-performance assembly and enhances the quality of remanufactured products.
FUTURE RESEARCH DIRECTIONS
Different from the consistency, standardization, and scale of an original assembly system, the remanufacturing assembly process with multiple uncertain remanufactured parts combinations requires absolute precise control [Figure 2]. It can ensure that the quality of remanufactured products is not worse than that of new products[57,58].
Based on the literature review and discussion above, we put forward potential research directions.
Multi-source data-driven quality control theory of remanufacturing assembly
To assure high-performance assembly in remanufacturing, increase remanufactured product quality, and address the high-dimensional uncertainty of remanufacturing assembly systems, future research should carry out comprehensive multi-source data fusion and analysis research. By using methods such as deep learning, feature extraction, and machine learning, key influencing factors and quality control flaws can be identified, which can optimize the quality control strategy for remanufacturing assembly, construct the data-driven quality evolution mechanism of remanufacturing assembly, and develop intelligent control processes for RAQ. These moves would create a theoretical and methodological framework for the quantitative assessment, the evolution mechanism, and intelligent management of RAQ, which supports the development of the remanufacturing industry at a high level of quality.
The research content of the quality control theory of remanufacturing assembly driven by multi-source data covers the following aspects:
Data perception and semantic representation of remanufacturing assembly quality: Construct a data perception interaction framework for remanufacturing assembly systems that integrates physical space and virtual space and study data interaction protocols for remanufacturing assembly quality. Digitally characterize the influencing factors, processes, and controls of remanufacturing assembly quality, construct a multi-source data conversion mechanism for remanufacturing assembly systems, and study unified semantic modeling and representation techniques for remanufacturing assembly quality data objects.
Remanufacturing assembly quality data chain: Propose a multidimensional, heterogeneous, missing, and dynamic method for processing and integrating remanufacturing assembly quality data. The quality data of remanufacturing assembly is associated with multiple sources according to temporal and process logic. Based on the remanufacturing assembly process data, the formation and evolution of remanufacturing assembly quality are described, and a remanufacturing assembly quality data chain is constructed to provide support for the evaluation and control of remanufacturing assembly quality, including expression, understanding, encapsulation, transmission, and sharing.
Remanufacturing assembly quality evaluation model: Starting from the logical relationship of “state fluctuation data change quality change”, this study investigates the functional boundaries of remanufacturing assembly quality elements and constructs a remanufacturing assembly quality loss index model based on Taguchi’s quality concept. In response to the problem of data fragmentation caused by cross stages in the remanufacturing assembly system, a multi-stage parameter estimation method based on deep neural networks for remanufacturing assembly processes is studied, and a data-driven quantitative evaluation model for remanufacturing assembly quality is established.
Studying the quality control theory of remanufacturing assembly driven by multiple data sources and information technology means achieving online monitoring and control of RAQ, which can help improve the quality level and production efficiency of remanufacturing assembly products and further promote the development of the remanufacturing industry.
Research on the evolution mechanism of RAQ
A quality information-sharing platform and collaborative mechanism should be established to promote the exchange and sharing of quality information among relevant stakeholders in the supply chain. Through cooperation, coordination, and joint efforts, a more comprehensive quality control system for remanufacturing and assembly should be constructed. Furthermore, a complete quality control standard system should be established to address the issue of inconsistent and incomplete quality control standards for remanufactured components[59]. The standard system should include inspection, evaluation, analysis, improvement, and other quality aspects for remanufacturing assembly. By strengthening the formulation of national and industry standards and establishing unified quality standards for remanufacturing assembly, it is possible to standardize how the remanufacturing sector develops.
Research on the Evolution Mechanism of RAQ includes the following specific contents.
Method for monitoring the quality elements of remanufacturing assembly: Establish a database of remanufacturing assembly quality elements, construct a remanufacturing assembly quality function house, convert customer requirements, quality element characteristics, and evolution process parameters into intuitive mathematical matrices, and identify key quality elements in the remanufacturing assembly system. Furthermore, a monitoring method for the fluctuation (random and abnormal) of remanufacturing assembly quality elements based on deep neural networks and Lean Six Sigma theory is proposed, providing support for remanufacturing assembly quality monitoring and traceability.
Modeling the Evolution Mechanism of Remanufacturing Assembly Quality: Given the many factors and complex mechanisms that affect the quality of remanufacturing assembly, methods such as neural networks, Bayesian networks, and support vector machines are proposed to predict and model the quality of remanufacturing assembly. Fuzzy association rule mining and recursive process mining algorithms are applied to analyze the historical data of remanufacturing assembly quality and the research results of remanufacturing experts from multiple perspectives, Construct a correlation model between quality control points and quality element indicators in the remanufacturing assembly process, study the evolution mechanism of remanufacturing assembly quality, and reveal the quality status, fluctuation trend, and cumulative effects of the remanufacturing assembly process.
A three-dimensional model of “problem data knowledge” for remanufacturing assembly quality: Based on database construction and mechanism research, a three-dimensional model of “problem data knowledge” for remanufacturing assembly quality is proposed, forming a closed-loop cycle of problems, data, and knowledge. A knowledge graph of remanufacturing assembly quality evolution based on multi-task graph neural networks is constructed, and the formation, maintenance, and decline laws of remanufacturing assembly performance are studied, realize data reuse and knowledge inheritance of remanufacturing assembly quality, and promote spiral upgrading and improvement of remanufacturing assembly quality.
Intelligent control and control platform for RAQ
Future remanufactured product quality control technology will typically be intelligent[60], including intelligent identification, intelligent detection, intelligent optimization, and intelligent monitoring of remanufactured assembly. It is necessary to research and develop intelligent quality control and management systems by using AI, Big data analysis, Internet of Things and other emerging technologies. Such a system can monitor the quality indicators during the remanufacturing assembly process in real-time. Through data analysis and prediction models[61], it can provide early warning and solve potential quality problems, thereby improving assembly quality and production efficiency. The goals of the system are to improve the production efficiency and quality control level of remanufacturing assembly, increase the comfort and value of remanufacturing production work, and attract more talent to participate in the growth of the remanufacturing industry. In the end, intelligence, humanization, and efficiency in the remanufacturing assembly can be achieved.
The following are some specific contents that may be included in this research field.
Abnormal feature recognition and control strategy in the remanufacturing assembly process: With the support of the remanufacturing assembly quality evolution mechanism and knowledge graph, explore the recognition method of remanufacturing assembly control curves and abnormal feature curves based on machine vision, identify and track parameter deviations in the remanufacturing assembly process, and predict the trend of abnormal features in the remanufacturing assembly process. Develop corresponding process operations and intelligent control strategies based on the knowledge graph and construct an abnormal feature library and control strategy library in the remanufacturing assembly process.
Intelligent control method for remanufacturing assembly quality based on component adaptation: Simulate and simulate the data of remanufacturing assembly systems based on the evolutionary mechanism, explore real-time monitoring, efficient analysis, and deep mining technology for dynamic data of remanufacturing assembly processes. Based on the remanufacturing assembly quality data chain, a multi-parameter model and solution method for the remanufacturing assembly process state space are studied. Based on deep reinforcement learning and quality evolution knowledge graph, an intelligent inference mode for precise control of remanufacturing assembly quality is constructed. A component-based intelligent control method for remanufacturing assembly quality is proposed, providing support for the multi-operation and precise control of remanufacturing assembly functional modules.
Design and development of an intelligent control platform for remanufacturing assembly quality: Based on virtual/physical systems, construct a heterogeneous network environment for remanufacturing assembly systems, explore seamless connection and integration technologies between the physical environment and virtual space of remanufacturing assembly systems, and study key technologies such as software and hardware topology structures, data knowledge fusion systems, and augmented reality control for remanufacturing assembly quality intelligent control; Taking a remanufacturing assembly workshop for a certain electromechanical product as the application object, based on its product, process, business, and production models and rules, the above theories, methods, and technologies are integrated and applied to the remanufacturing assembly quality intelligent control platform.
Promoting environmental friendliness and sustainable development
Future research should focus more on environmental protection and resource utilization issues in the remanufacturing assembly process, which can reduce energy consumption and emissions and promote the sustainable development of remanufacturing assembly. Considering economic, environmental, and social benefits, the remanufacturing assembly of mechanical and electrical products can be promoted towards a more environmentally friendly and sustainable direction. The following are some specific contents that may be included in this research field.
Resource recycling: Research methods for recycling, dismantling, and reusing waste materials and parts during the remanufacturing assembly process. This includes developing efficient recycling technologies, designing detachable and reusable product structures, and implementing effective resource recycling strategies to reduce resource waste and environmental pollution.
Green material selection and design: Research the selection and design methods of green materials in the remanufacturing assembly process, prioritizing the selection of environmentally friendly materials and technologies. Considering factors such as sustainability, renewability, and environmental impact during the life cycle of materials, develop corresponding material selection principles and design guidelines to reduce negative impacts on the environment.
Energy efficiency and carbon emission reduction: Research on energy management and carbon emission reduction methods for remanufacturing assembly systems. Improve energy efficiency and reduce carbon emissions by optimizing energy utilization and production processes. Energy-saving equipment, intelligent control, and energy management technologies can be used to reduce energy consumption and carbon emissions during remanufacturing and assembly.
Environmental risk assessment and management: Research on environmental risk assessment and management methods in the remanufacturing assembly process. By evaluating the remanufacturing assembly process and related environmental impacts, identify and manage potential environmental risks, take corresponding control and protective measures, and ensure the environmental safety and sustainability of the remanufacturing assembly system.
Environmental policy and economic incentives: Research, formulate, and implement environmental policies and economic incentives that adapt to remanufacturing. By means of taxation, subsidies, standards, and regulation, guide enterprises and individuals to take environmental actions, encourage the development and application of remanufacturing, and promote environmentally friendly and sustainable development.
The research content on promoting environmental friendliness and sustainable development of remanufacturing assembly systems involves resource recycling, green material selection and design, energy efficiency and carbon emission reduction, environmental risk assessment and management, as well as environmental policies and economic incentives. These contents aim to reduce resource waste and environmental pollution and promote the development of remanufacturing assembly systems in a more environmentally friendly and sustainable direction.
Strengthening the cultivation of remanufacturing talents
With the growth of the remanufacturing industry[62], the development of the remanufacturing business is hampered by the scarcity of remanufacturing skills. Therefore, it is urgent to establish a mechanism for cultivating remanufacturing talents. The research on strengthening the training of remanufacturing talents involves curriculum system design, teaching method innovation, practice base construction, teacher team construction, resource sharing and cooperation, as well as career development and Lifelong learning. These contents aim to cultivate talents with professional knowledge and practical skills in remanufacturing, meet the needs of the remanufacturing industry development, and promote the sustainable development of the remanufacturing industry. More importantly, it is necessary to establish incentive mechanisms to attract outstanding teachers and students to participate in cultivating remanufacturing talents. The industry should establish a mechanism for cultivating remanufacturing talents; doing so will also provide employment opportunities.
This article will be based on the theories of data mining and system modeling optimization, study the quantitative evaluation method of remanufacturing assembly quality driven by data, deeply explore the evolution mechanism and laws of remanufacturing assembly quality, and design and develop an intelligent control platform for remanufacturing assembly quality. On the one hand, it helps to promote resource recycling, reduce carbon emissions in the remanufacturing assembly process, and meet the development needs of sustainable industrial green development models in various countries. On the other hand, the stability of remanufacturing assembly systems will promote the improvement of remanufacturing product quality, satisfy the demands of consumers, improve the competitiveness of the remanufacturing industry, and promote the sustainable development of remanufacturing enterprises.
CONCLUSION
Remanufacturing is a crucial component of green manufacturing and a successful strategy for creating a society that conserves resources and respects the environment. Assembly is one of the crucial yet weak elements in ensuring the quality of remanufactured electromechanical goods. The current research of domestic and foreign scholars on the data management of RAQ and its evolutionary mechanism and multivariate mechanism needs to be explored in depth. Intelligent manufacturing is currently causing significant and profound changes in the development concept, manufacturing modes, and other aspects of the manufacturing industry, changing the technological infrastructure of the manufacturing sector as it becomes the foundation for the next industrial revolution. With the development of AI, intelligent detection, and other technologies in the future, remanufacturing assembly technology must achieve a transformation of intelligence, humanization, precision, and efficiency and achieve controllable, measurable, visible, and traceable scientific assembly, offering assurances regarding the safety, dependability, and quality of remanufactured items.
This small review paper is a further exploration and refinement based on the paper[10]. In the context of the rapid development of big data and AI technologies, remanufacturing assembly quality control faces greater challenges. In order to enhance the stability of remanufacturing assembly systems, improve the quality of remanufactured products, and realize the high-quality development of the remanufacturing industry, we propose potential research directions. These include the development of a multi-source data-driven quality control theory of remanufacturing assembly, research on the evolution mechanism of RAQ, the creation of intelligent control and control platforms for RAQ, promotion of environmental friendliness and sustainable development, and the reinforcement of efforts to cultivate remanufacturing talents. We will conduct in-depth explorations in the field of intelligent remanufacturing assembly to provide theoretical and methodological support for the high-quality development of China’s remanufacturing industry.
DECLARATIONS
Acknowledgments
The authors sincerely thank the editor and the anonymous reviewers for their constructive comments and suggestions that have greatly improved the manuscript.
Authors’ contributions
Conceptualization, writing-original draft preparation: Liu C
Resources: Zhu Q
Writing-review and editing: Mao H
All authors read and approved the final manuscript.
Availability of data and materials
All data generated or analyzed during this study are included in this published article.
Financial support and sponsorship
The first author is supported by the General Program of Anhui Natural Science Foundation (No. 2008085ME150), the Anhui Social Science Innovation and Development Research Project (2021CX069), Anhui Province University Innovation Group(2023AH010055), and Anhui Province Teaching and Research Project (2021jyxm1502, 2022kcsz287). The second author is supported by the Major Program (72192833/72192830) and Science Fund for Creative Research Groups (72088101) of National Natural Science Foundation of China.
Conflict of interest
All authors declared that there are no conflicts of interest.
Ethical approval and consent to participate
Not applicable.
Consent for publication
Not applicable.
Copyright
© The Author(s) 2023.
REFERENCES
1. Wang L, Guo Y, Cao J, Zhang Z, Xia X, Zhao H. Remanufacturing service combination and optimization for generalized growth of retired mechanical products. J Mech Eng 2023;59:339-54. Available from: http://www.cjmenet.com.cn/CN/10.3901/JME.2023.07.339.[Last accessed on 29 Nov 2023]
2. Li F, Li Z, Wang L, et al. Review on ICE remanufacture with additive repair technology. Chin Mech Eng 2019;30:1119-27,33. Available from: http://qikan.cmes.org/zgjxgc/EN/10.3969/j.issn.1004-132X.2019.09.015.[Last accessed on 29 Nov 2023]
3. Xu B, Dong S, Zhu S, Shi P. Prospects and developing of remanufacture forming technology. J Mech Eng 2012;48:96-105. Available from: http://www.cjmenet.com.cn/CN/abstract/abstract3990.shtml.[Last accessed on 29 Nov 2023]
4. Wang J, Gao S, Yang Y, Bao Y, Zhang Y. Review of the remanufacturability evaluation of mechanical equipment. J Mech Eng 2022;58:221-34.
5. Cao H, Du Y. Connotation and technology system framework of in-service remanufacturing of machine tools. Chin Mech Eng 2018;29:2357-63.
6. Zhang H, Li M, Liu W, Yuan Y. A review of key fundamental scientific problems for mechanical equipment remanufacturing. Chin Mech Eng 2018;29:2581-9.
7. Liu C, Zhu Q, Wei F, et al. A review on remanufacturing assembly management and technology. Int J Adv Manuf Technol 2019;105:4797-808.
8. Shi J, Zhu Q. A research into the mechanism of how multi-source quality information influences online sales of remanufactured products. Manage Rev 2021;33:199-208. Available from: https://journal05.magtech.org.cn/jweb_glpl/EN/Y2021/V33/I11/199. [Last accessed on 27 Nov 2023]
9. Zhao C, Wang D, Younas A, Zhang B. Coordination of closed-loop supply chain considering loss-aversion and remanufactured products quality control. Ann Oper Res 2022:1-24.
10. Guide VDR Jr, Jayaraman V, Linton JD. Building contingency planning for closed-loop supply chains with product recovery. J Oper Manag 2003;21:259-79.
11. Mukhopadhyay SK, Ma H. Joint procurement and production decisions in remanufacturing under quality and demand uncertainty. Int J Prod Econ 2009;120:5-17.
12. Liu M, Liu C, Xing L, Mei F, Zhang X. Study on a tolerance grading allocation method under uncertainty and quality oriented for remanufactured parts. Int J Adv Manuf Technol 2016;87:1265-72.
13. Kim JM, Zhou YD, Lee DH. Priority scheduling to minimize the total tardiness for remanufacturing systems with flow-shop-type reprocessing lines. Int J Adv Manuf Technol 2017;91:3697-708.
14. Ge M, Liu C, Liu M. The online quality control methods for the assembling of remanufactured engines’ cylinder block and cover under uncertainty. Int J Adv Manuf Technol 2014;74:225-33.
15. Zahraei SM, Teo CC. Optimizing a recover-and-assemble remanufacturing system with production smoothing. Int J Prod Econ 2018;197:330-41.
16. Huang M, Yi P, Shi T, Guo L. A modal interval based method for dynamic decision model considering uncertain quality of used products in remanufacturing. J Intell Manuf 2018;29:925-35.
17. Liao H, Deng Q, Wang Y, Guo S, Ren Q. An environmental benefits and costs assessment model for remanufacturing process under quality uncertainty. J Clean Prod 2018;178:45-58.
18. Huang W, Jiang Z, Wang T, Wang Y, Hu X. Remanufacturing scheme design for used parts based on incomplete information reconstruction. Chin J Mech Eng 2020;33:41.
19. Wang Y, Mendis GP, Peng S, Sutherland JW. Component-oriented reassembly in remanufacturing systems: managing uncertainty and satisfying customer needs. J Manuf Sci Eng 2019;141:021005.
20. Reddy KN, Kumar A, Velaga NR. Scenario-based two-stage stochastic programming for a hybrid manufacturing-remanufacturing system with the uncertainty of returns, quality and demand. Sādhanā 2021;46:59.
21. Cai X, Lai M, Li X, Li Y, Wu X. Optimal acquisition and production policy in a hybrid manufacturing/remanufacturing system with core acquisition at different quality levels. Eur J Oper Res 2014;233:374-82.
22. Muruganantham R, Annamalaisundaram S, Sangeetha D, Boopathy SR. Process optimization and remanufacturability analysis of fuel cell - Membrane electrode assembly with process simulation. J Fuel Cell Sci Technol 2012;9:061002.
23. Liu M, Sun Z, Ge M, Wang X, Wang Q. Remanufacturing quality optimization method of complex mechanical products based on assembly deviation degree. Chin Mech Eng 2014;25:1473-7,97. Available from: http://www.cmemo.org.cn/EN/Y2014/V25/I11/1473. [Last accessed on 27 Nov 2023]
24. Liu M, Xing L, Liu C, Zhang M. Online tolerance optimization for remanufactured complex mechanical product assembly processes. Chin Mech Eng 2015;26:55-62. Available from: http://www.cmemo.org.cn/EN/Y2015/V26/I1/55. [Last accessed on 27 Nov 2023]
25. Shen W, Pang K, Liu C, Ge M, Zhang Y, Wang X. The quality control method for remanufacturing assembly based on the Jacobian-torsor model. Int J Adv Manuf Technol 2015;81:253-61.
26. Hu J, Zhang Y, Ge M, Liu M, Liu C, Wang X. Optimal control method on assembly precision for a remanufactured car engine based on state space model. Assem Autom 2016;36:460-72.
27. Liu C, Zhu Q, Wei F, et al. An integrated optimization control method for remanufacturing assembly system. J Clean Prod 2019;248:119261.
28. Luh PB, Yu D, Soorapanth S, Khibnik AI, Rajamani R. A Lagrangian relaxation based approach to schedule asset overhaul and repair services. IEEE Trans Autom Sci Eng 2005;2:145-57.
29. Chen Y, Jiang Z, Zhu S, Zhang H. Optimization method for remanufacturing tolerance of electromechanical products with process condition constraint. Comput Integr Manuf Syst 2023;29:1327-35.
30. Geng Z, Bidanda B. Tolerance estimation and metrology for reverse engineering based remanufacturing systems. Int J Prod Res 2022;60:2802-15.
31. Wang Z, Jiang X, Liu W, Shi M, Yang S, Yang G. Precision prediction and error propagation model of remanufacturing machine tool assembly process. Comput Integr Manuf Syst 2021;27:1300-8.
32. Sun H. Key technology of main drive assembly and inspection technology of meshing quality of gear pair during TBM remanufacturing. Tunnel Construction 2020;40:890-7.
33. Li W, Zhang C, Liu C, Liu X. Error propagation model and optimal control method for the remanufacturing assembly. J Intell Fuzzy Syst 2022;42:2533-47.
34. Liu Q, Song J, Lv J, Tang O, Zhang Z. Mixed-flow assembly line balancing with uncertain assembly times in remanufacturing. IFAC-PapersOnLine 2022;55:97-102.
35. Kim MG, Yu JM, Lee DH. Scheduling algorithms for remanufacturing systems with parallel flow-shop-type reprocessing lines. Int J Prod Res 2015;53:1819-31.
36. Liu M, Liu C, Xing L, Zhang X, Wang Q, Wang X. Quality oriented assembly grouping optimal allocation method for remanufactured complex mechanical products. Chin J Mech Eng 2014;50:150-5.
37. Liu M, Liu C, Zhu Q. Optional classification for reassembly methods with different precision remanufactured parts. Assem Autom 2014;34:315-22.
38. Su B, Huang X, Ren Y, Wang F, Xiao H, Zheng B. Research on selective assembly method optimization for construction machinery remanufacturing based on ant colony algorithm. Chin J Mech Eng 2017;53:60-8.
39. Jiang X, Wang W, Zhang H, Zhang K, Li L. Optimal selective assembly method for remanufacturing product considering quality, cost and resource utilization. Chin J Mech Eng 2019;55:180-8.
40. Xue C, Jiang Z, Zhang X, Wang H. Multi-objective optimization model and application of components reuse combination for used mechanical equipment. Chin J Mech Eng 2017;53:76-85.
41. Chen Y, Jiang Z, Zhu S, Zhang H. Research on reassembly strategy of used mechanical equipment components based on non-cooperative game. Chin J Mech Eng 2021;57:203-12.
42. Guiras Z, Turki S, Rezg N, Dolgui A. Optimization of two-level disassembly/remanufacturing/assembly system with an integrated maintenance strategy. Appl Sci 2018;8:666.
43. Xing S, Jiang Z, Zhu S, Zhang H. Research on optimization method for parts selection of remanufactured products under dimensional accuracy constraints. Chin J Mech Eng 2022;58:221-8.
44. Yin L, Yang F, Zhu F, Fang L. Study on selective assembly of remanufacturing parts to be assembled based on NSGA-III. J Mach Design 2022;39:53-60.
45. Vasanthakumar C, Vinodh S, Ramesh K. Application of interpretive structural modelling for analysis of factors influencing lean remanufacturing practices. Int J Prod Res 2016;54:7439-52.
46. Kurilova-Palisaitiene J, Sundin E, Poksinska B. Remanufacturing challenges and possible lean improvements. J Clean Prod 2018;172:3225-36.
47. Fu Y, Zhou MC, Guo X, Qi L. Stochastic multi-objective integrated disassembly-reprocessing-reassembly scheduling via fruit fly optimization algorithm. J Clean Prod 2021;278:123364.
48. Ahn HD, Lee DH, Kim HJ. Solution algorithms for dynamic lot-sizing in remanufacturing systems. Int J Prod Res 2011;49:6729-48.
49. Reddy KN, Kumar A. Capacity investment and inventory planning for a hybrid manufacturing-remanufacturing system in the circular economy. Int J Prod Res 2021;59:2450-78.
50. Guo J, Pu Z, Du B, Li Y. Multi-objective optimisation of stochastic hybrid production line balancing including assembly and disassembly tasks. Int J Prod Res 2022;60:2884-900.
51. Wang T, Liao B, Yang C. Research on big-data-driven green intelligent manufacturing mode and the implementation design. JCQU 2020;43:64-73.
52. Xu B, Xia D, Tan J, Dong S. Status and development of intelligent remanufacturing in China. Chin Surf Eng 2018;31:1-13.
53. Ketzenberg ME, Souza GC, Guide Jr VDR. Mixed assembly and disassembly operations for remanufacturing. Prod Oper Manag 2003;12:320-35.
54. Liu M, Wang Q, Zhao Z, Ling L. Dynamic assembly process quality control system for mechanical products remanufacturing. Comput Integr Manuf Syst 2014;20:817-24.
55. Liu D, Huang H, Wang B, Zhou T, Luo S. Operation paradigm for remanufacturing shop-floor based on digital twin. Comput Integr Manuf Syst 2019;25:1515-27.
56. Song B, Jiang X, Wang S, Liu Q, Yu T. The promotion and application of green remanufacturing: a case study in a machine tool plant. Environ Sci Pollut Res 2023;30:40870-85.
57. Wurster M, Michel M, May MC, Kuhnle A, Stricker N, Lanza G. Modelling and condition-based control of a flexible and hybrid disassembly system with manual and autonomous workstations using reinforcement learning. J Intell Manuf 2022;33:575-91.
58. Andersen AL, Brunoe TD, Bockholt MT, et al. Changeable closed-loop manufacturing systems: challenges in product take-back and evaluation of reconfigurable solutions. Int J Prod Res 2022;61:839-58.
59. Liu C, Chen J, Wang X. Quantitative evaluation model of the quality of remanufactured product. IEEE Trans Eng Manag 2023:1-12.
60. Liu C, Cai W, Zhang C, Wei F. Data-driven intelligent control system in remanufacturing assembly for production and resource efficiency. Int J Adv Manuf Technol 2023;128:3531-44.
61. Liu C, Chen J, Cai W. Data-driven remanufacturability evaluation method of waste parts. IEEE Trans Ind Inform 2021;18:4587-95.
Cite This Article
Export citation file: BibTeX | RIS
OAE Style
Liu C, Zhu Q, Mao H. Review and challenges for the remanufacturing assembly quality with uncertainty. Green Manuf Open 2023;1:15. http://dx.doi.org/10.20517/gmo.2023.072701
AMA Style
Liu C, Zhu Q, Mao H. Review and challenges for the remanufacturing assembly quality with uncertainty. Green Manufacturing Open. 2023; 1(3): 15. http://dx.doi.org/10.20517/gmo.2023.072701
Chicago/Turabian Style
Liu, Conghu, Qinghua Zhu, Huiying Mao. 2023. "Review and challenges for the remanufacturing assembly quality with uncertainty" Green Manufacturing Open. 1, no.3: 15. http://dx.doi.org/10.20517/gmo.2023.072701
ACS Style
Liu, C.; Zhu Q.; Mao H. Review and challenges for the remanufacturing assembly quality with uncertainty. Green. Manuf. Open. 2023, 1, 15. http://dx.doi.org/10.20517/gmo.2023.072701
About This Article
Special Issue
Copyright
Data & Comments
Data
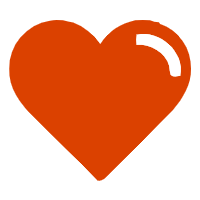

Comments
Comments must be written in English. Spam, offensive content, impersonation, and private information will not be permitted. If any comment is reported and identified as inappropriate content by OAE staff, the comment will be removed without notice. If you have any queries or need any help, please contact us at support@oaepublish.com.