Are there possible issues that we overlook when selecting green infrastructure?
Abstract
Promoting green infrastructure (GN) as a tool for reducing natural disaster risk is promising, particularly in depopulated areas worldwide. Images of GN as being multifunctional and environment friendly seem to enhance citizens’ positive attitudes toward it. While conventional types of disaster prevention infrastructure, such as concrete levees, can completely prevent damage, GN can relieve the effects of natural hazards. By switching from conventional infrastructure to GN, societies could reduce the costs of disaster prevention infrastructure. However, the risk of disaster damage may increase in some areas. This study aimed to better understand citizens’ preference for GN. It was hypothesized that those who prefer GN are those who do not take the initiative to learn about it and that some citizens are not fully aware of the heterogeneous risks when selecting GN. To test the hypotheses, structural and measurement models, including latent variables, were developed, and structural equation modeling was performed. Data for constructing the measurement and structural equations were obtained using a web questionnaire administered by a professional research company in 2022 with 1,200 participants across Japan. Data from the portal site for the official statistics of Japan (e-Stat) were also used. The results confirm the hypothesis that individuals who favor GN are those who do not proactively seek to acquire knowledge concerning it. The findings highlight the importance of considering, in future policymaking, the possibility that citizens who prefer GN without knowledge about it may face high risks as a result of its uninformed adoption and use.
Keywords
INTRODUCTION
The severe and frequent occurrence of natural disasters is one of the biggest global issues in current times. This affects many aspects of citizens’ lives, including finances[1,2], markets[3], and food supply[4,5], among others. People expect former safety levels to be maintained and do not want to suffer further damage. However, it is not realistic to expect some societies to maintain such safety levels because this incurs additional initial and maintenance costs for disaster prevention infrastructure, such as conventionally used concrete levees (hereafter referred to as gray infrastructure [GY])[6,7].
One such example is regions in developed countries that face depopulation[8]. Japan has witnessed depopulation throughout the country, particularly in local areas where nature remains abundant[9]. A reasonable approach would be to utilize ecosystem goods and services for disaster prevention and reduction, that is, ecosystem-based disaster risk reduction (eco-DRR), especially if such areas face both depopulation and disaster risk increments. This is because eco-DRR will not incur initial costs, and maintenance costs may not be a burden because the ecosystem has self-restoration functions[10]. Enhancement of the eco-DRR function of green infrastructure (GN) may also decrease the natural disaster risk level of other areas; eco-DRR activities in upstream regions are not only a natural buffer in the region itself but may also relieve natural disasters in populous downstream areas[11].
Existing studies have stimulated the application of nature-based infrastructure, such as forests, wetlands, coral reefs, and oyster reefs (hereafter, GN also includes elements such as the latter two that are sometimes referred to as blue infrastructure), because they are abundant in depopulating rural areas. GN can present an appealing image to citizens because of its multifunctionality, including with respect to the mitigation of climate change, the development of beautiful scenery, and the maintenance of a sound ecosystem[12,13]. Evidence suggests that the application of GY can adversely affect the environment[14]. By contrast, the selection of GN may resemble that for other products and activities, such as eco-labeled products, which may, in turn, facilitate citizens’ acceptance of GN.
Some factors could enhance citizens’ preference for GN over GY; recent studies have pointed to factors that may be related to ecosystem conservation and interest in natural disaster prevention. Some recent studies are particularly valuable to understand the different aspects related to the preference for GN. Falco and Corbi[15] investigated how environmental preference is shaped in people and found that natural disaster experience in early adulthood induces pro-environmental attitudes. Risk-aversion activities may differ according to people’s experiences of major natural disasters[16]. However, to the best of the author’s knowledge, no existing studies have considered the key factor that differentiates citizens’ preferences for GN and GY.
This study sheds light on the heterogeneity that presents in risk-aversion activities (Other existing studies on GN have also included risk in their examination. However, the author could not find studies considering heterogeneous risks associated with adopting GN. For a comprehensive literature review, see, for example, Venkataramanan et al.[17]). In this regard, Chen et al. point out that financial firms’ responses in the capital market vary according to the type of firm and the natural disaster experienced[18]. In the case of citizens, the application of GN may incur heterogeneous risks not only due to variations in the vulnerability of each citizen (e.g., age and physical conditions) but also because of differences in the vulnerability of their living place. This is one of the issues we overlook when selecting GN.
For example, the risk of river flooding is serious for those living alongside the river but may not affect those living at a distance from it. This is the crucial difference between the selection of GN and other environmentally friendly activities, such as choosing eco-labeled products. This is because the latter does not involve heterogeneous risks related to living places. This study investigates whether citizens select GN proper recognition of the existence of heterogeneous risks. Considering the example of river flooding, although GY almost completely prevents it as long as the river water level is below the upper limit of concrete levees, GN relieves flooding events by reducing the volume of discharged water. In this study, it is posited that some citizens have not paid enough attention to heterogeneous risks owing to the lack of knowledge on the subject and a conventionally positive image of environmentally sound activities.
PROCEDURE OVERVIEW
Factors that may relate to citizens’ disaster prevention and reduction activities
The factors directly or indirectly associated with citizens’ disaster prevention and reduction activities vary widely. Based on the main factors of this study, namely, risk and knowledge, four types of citizens were identified; the details are presented in Table 1. Types 1 and 3 citizens are at low risk and may choose GN. Type 2 citizens face high risk but can cope with possible disasters because they know their high risk. Type 4 citizens also face high risk but are unaware of it. In actual policies, Type 4 citizens should be the main target because they face unrecognized high risks. Additionally, the related factors should be considered when promoting risk management.
Types of citizens
Knowledge: high | Knowledge: low | |
Risk: low | Type 1 They may select GN because the risk is low | Type 3 They may select GN because the risk is low |
Risk: high | Type 2 They may decide not to select GN because the risk is high | Type 4 They lack knowledge and may select GN without recognizing the high risk |
This study examines natural disasters and GN in a general context; that is, different types of disasters, such as typhoons, tsunamis, and earthquakes, and different types of GN, such as coral reefs, wetlands, and forests, are considered. Generally, GN has no clear threshold level at which damage happens. For example, wetlands and forests may absorb heavy rainfall, but some amount of surface runoff can occur (e.g., similar to how sponges do not completely absorb water). By contrast, GY has a clear threshold level. For example, river water will not leak until the river water level exceeds the height of the embankment. Thus, in the case of GN, damages may occur in the earlier stage of natural events that accompany associated risks. The same can be said for other GN and GY (e.g., coast forests may not completely protect against tsunamis, while concrete seawalls provide some protection unless the upper limits are breached). Because GY is part of conventional infrastructure, it can be assumed that citizens understand the above characteristics of GY. However, the application of GN infrastructure is more recent, and it is often the case that hazards (e.g., surface runoff) in earlier stages of disasters are not explained to citizens clearly. Venkataramanan et al. pointed out that people’s overall knowledge and awareness of GN is low[17]. Therefore, it is reasonable to suppose that some citizens do not perceive heterogeneous risks in the case of GN, as shown in Table 1.
Structural equation modeling
Structural equation modeling (SEM) allows the creation of latent variables based on observed data, making it possible to develop multiple-equation models to reveal the relationship between observable and latent variables. Because some variables, such as the preference for GN and GY, are not observable, this study applied SEM to create these factors as latent variables and investigated whether citizens who prefer GN are those who do not take the initiative to learn about it.
Many studies have used SEM to investigate disaster-related issues[19]. Some studies have tested theoretical models using this technique. For example, Cai et al. examined disaster risk reduction based on the social cognitive theory[20-23], and Shi et al. used the theory of planned behavior[24-27] to identify the factors that influence stakeholders’ intentions to participate in environmental governance in the Yangtze River Delta Region. Other studies have applied SEM in an exploratory manner. Dargin et al. adopted an approach to combine the disaster risk theory and food-energy-water nexus systems thinking and tested the proposed relationship using SEM[28]. He et al. used SEM to determine self-protection factors against COVID-19[29]. Similarly, the current study employed exploratory procedures in selecting variables to expand on extant knowledge in this domain.
LITERATURE REVIEW AND HYPOTHESES DEVELOPMENT
Preparation: soft measures
Preparation for natural disasters is one of the factors that may relate to risk awareness and knowledge and influence the preference for GN and GY. Both soft and hard measures are used to mitigate the hazards of natural disasters[30]. These measures are perceivable real actions[31]. To the best of the author’s knowledge, no existing studies have investigated the relationship between preparation for disaster and preference for GN. However, such a relationship is considered plausible based on the following literature, which is tested using hypotheses developed in this study.
Adelman et al. distinguish “hardship” as an experience of the present and “vulnerability” as the risk of experiencing hardship in the future[32]; “hardship” is uncontrollable as it has already happened and “vulnerability” is controllable through citizens’ behavior. People will engage in disaster reduction behavior, especially when they perceive high risks[33,34]: they prepare because they experience the present hardship
H1. Those who are keen on natural disaster prevention and engage in risk reduction activities (soft measures) do not prefer GN.
Preparation: hard measures
The same prediction may hold for hard measure preparations. Rufat et al. suggest that the physical environment may influence citizen behavior[38]. People may also prepare for disasters through the selection of a living environment. It is assumed that those who take hard measures do not prefer GN while those who do not prepare may show a neutral preference for GN.
H2. Those who are keen on natural disaster prevention and engage in risk reduction activities (hard measures) do not prefer GN.
Garbage disposal
Existing studies have pointed out that citizens do not fully recognize the importance of participating in disaster mitigation activities[39]. The importance of preparing for disasters has gradually been acknowledged by local governments and communities following severe natural events worldwide, and mutual communication and cooperation with relatives, friends, and neighbors have been emphasized because they can help compensate for citizens’ insufficient knowledge and skills[40,41]. The conditions in towns/cities where citizens live and their relationships with other residents may relate to their preference for GN. Therefore, some sociodemographic variables related to the preference for GN may be included as explanatory variables. Prior review articles have pointed out that the same sociodemographic variable can be either significant or not significant depending on each study. Wachinger et al. stated that some personal factors, such as age and gender, influenced natural disaster risk perception in some studies but not in others[31]. Further, Venkataramanan et al. pointed out that few studies have systematically assessed the role of demographic, socioeconomic, and/or geographic characteristics that are tied to knowledge and awareness toward GI, which the current study aimed to address by including diverse variables and investigating them in an exploratory manner[17].
While the accumulation of potential explanatory variables’ roles has still not been enough, the sign condition of garbage disposal is decidable a priori. GN is an ecosystem-based infrastructure, and people’s activities related to garbage dumping have had a negative impact on its condition. Based on this context, the following hypothesis was developed:
H3. Those with less knowledge about matters related to garbage disposal prefer GN.
GY
As explained above, GN has been introduced in local areas under financial constraints. While GN is an ecosystem-based infrastructure, GY is an artificial concrete infrastructure; people may prefer one over the other. Therefore, the following hypothesis was developed:
H4. Those who prefer GY do not prefer GN.
Preference for GN/GY and knowledge
GN and GY are different types of infrastructure used for preventing and reducing natural disasters. Suppose a river runs through the middle of a city’s residential area. As explained before, GY, such as concrete levees, fully protects against floods in rivers until the water level reaches the edge of the levee. Once the river water level exceeds the height of the levee, excess water flows into the residential area; however, this study treated such events as less common. GN, such as wetlands, may absorb increased water and relieve the impact of excess water.
Let us further suppose that the initial and maintenance costs of GY are high while almost no initial cost is required for GN. Existing studies also point out that associated costs of GN are much lesser than those of GY[14,42]. For example, Wang et al. showed that the economic and other costs of GN are lower than those of GY in the case of combined sewer systems[42]. However, it is important to note that because GN is an ecosystem-based infrastructure, citizens, especially those living in watersheds, are expected to conserve their river basin.
For simplicity, let us consider the costs and risks of GY as a baseline. In the above setting, the cost of GN is lower than that of GY. If citizens select GN instead of GY, they enjoy a cost reduction. The disaster risk in GN is higher than that in GY, and the risk increment differs among citizens. For example, those living alongside the river may face higher risks, whereas the risk to those living far from the river may not change; that is, heterogeneous risks exist among citizens. While promoting GN, cost savings are often emphasized, and the existence of heterogeneous risks may not be acknowledged. Certain citizens prefer GN. This is because, owing to insufficient information, they associate environmentally friendly ideas and images (e.g., as with eco-labeled products) with GN.
This study posits that some citizens prefer GN without recognizing the existence of heterogeneous risks among them. Cautious citizens, especially those considering the existence of associated risks of GN, would be more hesitant about adopting GN in their region. Indeed, an existing study suggests that lack of knowledge is an obstacle to disaster avoidance behaviors[43]. Accordingly, the following hypotheses were formulated:
H5. Those who prefer GN are those who do not take the initiative to learn about it.
H6. Those who prefer GY are those who take the initiative to learn about it.
METHODS
Questionnaire
Two questionnaires were administered to gather data to test the hypotheses using SEM. The first was a pre-survey of 100 participants conducted between October 18 and October 20 2022. The pre-survey was conducted by a professional web research company, and participants were randomly selected from registered candidates throughout Japan. The questionnaire used in the pre-survey was modified based on both the responses and the estimation results of the SEM, and the main survey was conducted between November 29 and December 2 2022, by the same research company. The number of participants was 1200. In both the pre-survey and the main survey, those younger than 18 were excluded as candidates.
The questionnaire comprised two parts. The first section contained questions on respondents’ demographic attributes, and the second included inquiries related to natural disasters. The responses of participants to the main survey were used as variables in the SEM analyses. Postal code information of the respondents was also included in the questionnaire, and municipal-level data from e-Stat (the official website for government statistics of Japan)[44] were linked to each respondent’s data based on their postal code.
Data and variable description
Data used in SEM analyses were either obtained via the questionnaire survey or gathered from e-Stat[44]. The latent and other variables created using the above data are explained in Tables 2 and 3, respectively.
Latent variables
Latent variable | Code | Questionnaire items |
GREEN | KL_GN | (The number of selected options between (1) and (7) was used; if option (8) was selected, 0 was assigned) Which of the following statements about green infrastructure did you know about or have heard before participating in this questionnaire survey? (1) Coastal forests block sea breeze and reduce salt damage to inland fields and houses (2) Coastal forests weaken the power of tsunamis and storm surges, reducing damage to coastal areas (3) Coral reefs weaken the power of tsunamis and storm surges, reducing damage to coastal areas (4) Wetlands along rivers serve as retarding basins during heavy rains, reducing flood damage (5) An ecosystem that exerts disaster prevention and mitigation functions is sometimes called green infrastructure (6) Maintenance costs of green infrastructure are cheaper than those of conventional disaster prevention and mitigation infrastructure, such as concrete seawalls (7) Green infrastructure is a nature-based infrastructure. Thus, it is maintained and regenerated without human intervention (8) I didn’t know about any of the above options |
GRAY | KL_GY | (The number of selected options between (1) and (7) was used; if option (8) was selected, 0 was assigned) Which of the following statements about gray infrastructure did you know about or have heard before participating in this questionnaire survey? (1) Concrete retaining walls prevent landslides from collapsing on slopes and increase the safety of building sites (2) Wave-dissipating blocks (tetrapods) weaken the power of waves and suppress coastal erosion (3) Concrete tide barriers constructed along the coast prevent high tides from invading the land (4) Concrete breakwaters built underwater prevent high waves and tsunamis from invading land (5) Man-made structures that perform disaster prevention and mitigation functions are sometimes called gray infrastructure (6) Green infrastructure such as coastal forests can only "weaken" the power of high waves and tsunamis, but gray infrastructure can "prevent" high waves and tsunamis from invading the land as long as the level of water is lower than such infrastructure (7) Gray infrastructure is man-made. Thus, it is necessary to regularly repair and reconstruct such infrastructure (8) I didn’t know about any of the above options |
GREEN, GRAY | Living_area | Which of the following images is closest to your favorite living area (your home and the surrounding land area)? You can choose an image that is different from your current place of residence (1) Although there are trees along the roadside, most of the land is covered with concrete and asphalt (2) Although there are many trees along the roadside, in parks, and in sites of facilities and residences, approximately 60%-70% of the land is covered with concrete or asphalt (3) Although the center of the area is covered with concrete and asphalt, approximately 60%-70% of the town’s land is covered with fields, grasslands, and mountain forests (4) It is a natural environment: houses are scattered, and most of the land is covered with fields, grasslands, and forests, except for a few asphalt roads |
Avoidance | Have you ever traversed a road that was flooded with water due to heavy rain (the level of water was so high that water got into your shoes when you stepped on it)? (1) I have walked on roads that were flooded with water due to heavy rain many times (2) I have walked on a road flooded with water due to heavy rain once or several times (3) I have never walked on a road that was flooded due to heavy rain, but I have taken a detour before (4) I have never walked on a road that was flooded due to heavy rain or taken a detour | |
Risk_1 | (If the respondent selected Caterer A store, 0 was assigned, and if Caterer B store was selected, 1 was assigned) Suppose you order a bento box from one of the following caterers. Which one would you like to order from? Caterer store A: • Evaluations from people who ate their food are often consistent • There is a 90% probability that 10 people will say it is delicious, but there is also a 10% probability that no one will say it is delicious • Cost is 9,000 JPY for 10 people Caterer store B: • People who have eaten their food often have mixed reviews • There is a 40% probability that all 10 people will say it is delicious, but there is also a 10% probability that no one will say it is delicious. Additionally, there is a 30% probability that only six people will say it is delicious and a 20% probability that only three people will say it is delicious • Cost is 6,400 JPY for 10 people | |
Risk_2 | Suppose you are driving a car on a road carved out of a mountain slope. Do you feel safer if the cut slope is covered with concrete or trees? Further, suppose that scientists suggest that concrete and tree cover function equally and make no difference to safety. Which of the following represents your opinion? (1) Concrete is safer (2) If anything, concrete is safer (3) If anything, trees are safer (4) Trees are safer | |
PRE_1 | Are there any places near your residence where you or your home may be in danger if an earthquake, heavy rain, storm, tsunami, or storm surge occurs? | |
Pre1_1 | Mountain(s), cliff(s), etc. (1) There is no such place nearby (2) I don’t feel the danger of disasters (3) I sometimes feel the danger of a disaster (4) A disaster has happened nearby (5) I have experienced a disaster (disasters) | |
Pre1_2 | Lake(s), pond(s), etc. (1) There is no such place nearby (2) I don’t feel the danger of disasters (3) I sometimes feel the danger of a disaster (4) A disaster has happened nearby (5) I have experienced a disaster (disasters) | |
Pre1_3 | River(s) (1) There is no such place nearby (2) I don’t feel the danger of disasters (3) I sometimes feel the danger of a disaster (4) A disaster has happened nearby (5) I have experienced a disaster (disasters) | |
Pre1_4 | Ocean (1) There is no such place nearby (2) I don’t feel the danger of disasters (3) I sometimes feel the danger of a disaster (4) A disaster has happened nearby (5) I have experienced a disaster (disasters) | |
PRE_2 | How much do you prepare for natural disasters? Please rate yourself and choose the option that you think most accurately reflects your position | |
Pre2_1 | Ensuring secure furniture and preventing dishes from falling off shelves (1) 0% (2) 25% (3) 50% (4) 75% (5) 100% | |
Pre2_2 | You and your family have decided how to confirm the safety of each other and the place to gather (1) 0% (2) 25% (3) 50% (4) 75% (5) 100% | |
Pre2_3 | You select a place with solid ground or a place that is less susceptible to tsunamis and storm surges in a residential area (1) 0% (2) 25% (3) 50% (4) 75% (5) 100% | |
Pre2_4 | You have stored water and food at home to prepare for a disaster (1) 0% (2) 25% (3) 50% (4) 75% (5) 100% | |
Pre2_5 | You prepare fire insurance and/or earthquake insurance contracts (1) 0% (2) 25% (3) 50% (4) 75% (5) 100% | |
Pre2_6 | You have developed relationships with neighbors that enable cooperation in times of disaster (1) 0% (2) 25% (3) 50% (4) 75% (5) 100% |
Other variables
Variable (code) | Questionnaire items |
Men | What is your gender? (0) Woman (1) Man |
Married | What is your marital status? (0) Married (1) Not married |
Education | Which of the following is your final educational level? If you graduated from two or more schools, please select the highest educational level (1) Junior high school or less (including elementary school graduates, dropouts, etc.) (2) High school (regardless of full-time, part-time, correspondence, etc.) (3) Vocational school/specialized training college (diploma, etc.) (4) College of technology (technical college, associate degree) (5) Junior college (junior college degree) (6) University (bachelor’s degree) (7) Graduate school (master’s/doctoral degree) |
Garbage | (The number of selected options between (1) and (7) was used; if option (8) was selected, 0 was assigned) (1) In the past, people in Japan used to wash their clothes in rivers and throw some of their garbage into rivers (2) Plastic trash has been found at the bottom of the Mariana Trench, the deepest oceanic trench on Earth (3) If marine debris such as shopping bags that wash ashore covers the coast, mangrove buds cannot grow (4) If drifted wood and metal products damage mangrove trunks, respiratory roots, and support roots, the mangroves will die (5) When materials discarded as garbage contaminate the water and soil of mangrove habitats, mangroves also suffer damage (6) When mangroves die, sand flows away, riverbanks and coasts recede, and sea breeze tends to enter inland, making salt damage and wind damage more likely to occur (7) In some Southeast Asian countries, rivers become clogged with garbage, which intensifies flooding (8) I didn’t know about any of the above |
Descriptions | |
Age_mean | The median age of a city in 2015 from e-Stat[44] |
Income_mean | Taxable income (per taxpayer) [thousand JPY] in 2020 from e-Stat[44] |
Forest | “Forest area [ha] in 2014” divided by “Habitable land area [ha] in 2020” from e-Stat[44] |
Life_exp_m | Average life expectancy (at the age of 0 years old, male) in 2020 from e-Stat[44] |
Habitable | Habitable area ratio [%] in 2020 from e-Stat[44] |
Pop_per | Population density per 1 km2 of habitable area in 2015 from e-Stat[44] |
In this study, four latent variables were proposed: the preference for GN (GREEN); the preference for GY (GRAY); prevention and reduction activities for natural disasters reflected in hard measures (physical safety level of living place, PRE_1); and prevention and reduction activities for natural disasters reflected in soft measures (activities in living place, PRE_2). These latent variables were constructed in the measurement models, and the preference for GN was explained by other latent variables in the structural model using SEM. The construction of the four latent variables is explained later in this section.
Because GN is a new concept for most citizens, and both GN and GY exert similar functions of disaster prevention and reduction, it was reasonable to consider commonly observed variables for both infrastructures. In this study, living area (Living_area), avoidance behavior of natural disasters in daily life (Avoidance) and risk perception (Risk_1, Risk_2) were included as observed variables [Table 2]. Here, Living_area reflected the respondents’ homes and the surrounding land area in which they led their daily lives (natural and urban landscapes), and Avoidance indicated how keenly respondents avoided getting their shoes soaked due to submerged roads. Risk_1 was the risk preference in a general context with two options (risk-seeking and risk-aversion), and Risk_2 was the risk preference of GN and GY in a more general context.
Theoretical and conceptual framework
The theoretical framework of this study was based on the perception formation model proposed by Litterer[45], which has been applied to study many topics such as environmentally safe products[46], life insurance[47], and financial investment[48]. This model consists of three components: one perception variable, one formation variable, and some explanatory variables. In this study, PRE_1, PRE_2, sociodemographic variables, and GRAY were explanatory variables; GREEN corresponded to the perception variable; and KL_GN was equivalent to the formation variable.
Analytical framework
Measurement models
The first two latent variables were the preference for GN (GREEN) and GY (GRAY). The associated measurement models were as follows:
Here, the sign conditions of KL_GN and KL_GY were negative and positive, reflecting H5 and H6, respectively. Typically, those who prefer the objects have more knowledge and the sign condition should be positive. However, in the case of GN, because it is an ecosystem-based infrastructure, it may not prevent damages even if a hazard is moderate; thus, people who prefer GN may not have a lot of knowledge about it (One of the reviewers pointed out the possibility of applying a moderated mediation model. Because this study treated green infrastructure in general and considered different types of natural disasters, it was impossible to create a risk variable effectively).
The remaining two latent variables were PRE_1 and PRE_2, that is, prevention and reduction activities against natural disasters. PRE_1 included four observed variables and reflected each respondent’s choice of living place based on its physical safety level. PRE_2 included six observed variables and reflected each respondent’s activity level in daily disaster prevention and/or reduction. The measurement models are given below:
Structural model
The following structural model was developed:
The variableQ and variablee-Stat in Eq. (5) were prepared based on data from the questionnaire survey of this study and e-Stat[44]. Both variableQ and variablee-Stat were included as control variables because they represent respondents’ internal factors (e.g., Age_mean) and external factors (e.g., Education)[49].
Estimation procedure
This study used confirmatory factor analysis (CFA) to prepare the latent variables PRE_1 and PRE_2. This is because the first five factors (Pre2_1–Pre2_5) and the last factor (Pre2_6) focused on slightly different aspects of daily life activities of citizens. While Pre2_6 asked about cooperation in their places of residence, the other items asked about their activities at home. Based on the CFA results, Pre_1-1–Pre 1-4 were included in PRE_1. Pre2_6, in addition to Pre2_1–Pre2_5, was included in PRE_2. The CFA was conducted using the R environment[50] and its packages “psych” and “GPArotation”.
The R packages “lavaan”[51], “tidyverse”, and “semPlot” were used for SEM analyses. SEM enables us to test cause-and-effect interactions among latent variables[24]. It was conducted through the following steps. First, the estimation performances of the four latent variables in the measurement models and the related hypotheses (H5 and H6) were assessed. Subsequently, the four latent variables and other observed variables were used to develop a structural model. Similar variables, such as the “age” of a respondent from the questionnaire survey and the “average age” of the respondent’s living city from e-Stat[44], were available for some explanatory variables in the structural model. In such cases, the more suitable ones were selected based on the estimation results when constructing the structural model.
Several fit indices were used to check the goodness-of-fit of the models. As a rule of thumb, > 0.8 is usually recommended as the threshold for the comparative fit index (CFI), goodness-of-fit index (GFI), adjusted GFI (AGFI), and incremental fit index (IFI), and values of the root mean squared error of approximation (RMSEA) are judged as close fit for < 0.05, fair fit for 0.05-0.08, and moderate fit for 0.08-0.1[52,53].
RESULTS
Respondent characteristics
There were no invalid answers because the professional web research company restricted respondents from submitting answer forms without answering all the questions. However, 17 participants were excluded from the analyses because they provided postal codes that did not exist, making it impossible to assign e-Stat[44] data to their answers. Thus, the sample size was 1,183 (i.e., 1200-17). The demographic characteristics of the respondents are presented in Table 4.
Respondent demographics (n = 1,183)
Categories | n | % | |
Gender | Man Woman | 611 572 | 51.6 48.4 |
Marital status | Single Married | 460 723 | 38.9 61.1 |
Age (years) | 18-20 21-30 31-40 41-50 51-60 61-70 71-80 81- | 32 232 195 202 202 180 131 9 | 2.7 19.6 16.5 17.1 17.1 15.2 11.1 0.8 |
Annual income [Japanese yen (JPY)] | 1.99 million JPY or lower 2-3.99 million JPY 4-5.99 million JPY 6-7.99 million JPY 8-9.99 million JPY 10-11.99 million JPY 12-13.99 million JPY 14 million JPY or higher | 212 293 240 164 126 61 29 58 | 17.9 24.8 20.3 13.9 10.7 5.2 2.5 4.9 |
Educational status | Junior high school or less High school Vocational school/specialized training college College of technology Junior college University Graduate school | 43 346 135 25 91 480 63 | 3.6 29.2 11.4 2.1 7.7 40.6 5.3 |
Model estimation results
In the estimation of measurement models, it was necessary to fix the value of one of the variables at one; Table 5 shows the results when “Living_area” in GREEN and GRAY, “Pre1_1” in PRE_1, and “Pre2_1” in PRE_2 were fixed in accordance. Among the variables in the measurement models, Risk_1 was statistically significant at the 10% level for GREEN but not for GRAY. Other observed variables of GREEN and GRAY and all variables of PRE_1 and PRE_2 were statistically significant at the 1% level. Figure 1 provides a graphical description of the SEM results.
Estimation results: measurement model
Estimate | Std. err. | z value | P value | Std. all | |
GREEN | |||||
Living_area | 1.000 | 0.950 | |||
KL_GN | -1.342 | 0.177 | -7.563 | 0.000 | -0.749 |
Risk_1 | 0.108 | 0.056 | 1.951 | 0.051 | 0.193 |
Risk_2 | -0.386 | 0.111 | -3.487 | 0.000 | -0.359 |
Avoidance | 0.797 | 0.128 | 6.229 | 0.000 | 0.745 |
GRAY | |||||
Living_area | 1.000 | 0.871 | |||
KL_GY | 2.410 | 0.492 | 4.897 | 0.000 | 1.044 |
Risk_1 | 0.053 | 0.058 | 0.904 | 0.366 | 0.086 |
Risk_2 | -0.417 | 0.118 | -3.524 | 0.000 | -0.355 |
Avoidance | 0.649 | 0.127 | 5.109 | 0.000 | 0.556 |
PRE_1 | |||||
Pre1_1 | 1.000 | 0.776 | |||
Pre1_2 | 1.064 | 0.033 | 31.753 | 0.000 | 0.909 |
Pre1_3 | 0.978 | 0.043 | 22.873 | 0.000 | 0.658 |
Pre1_4 | 0.995 | 0.034 | 29.030 | 0.000 | 0.809 |
PRE_2 | |||||
Pre2_1 | 1.000 | 0.684 | |||
Pre2_2 | 1.161 | 0.051 | 22.925 | 0.000 | 0.729 |
Pre2_3 | 1.194 | 0.052 | 23.024 | 0.000 | 0.765 |
Pre2_4 | 1.065 | 0.059 | 18.127 | 0.000 | 0.566 |
Pre2_5 | 1.151 | 0.045 | 25.367 | 0.000 | 0.817 |
Pre2_6 | 1.105 | 0.045 | 24.296 | 0.000 | 0.810 |
Ten control variables were included in the final structural model, in addition to the latent variables [Table 6, Figure 1]. These variables were Men, Married, Education, and Garbage from variableQ and Age_mean, Income_mean, Forest, Life_exp_m, Habitable, and Pop_per from variablee-Stat. All the latent variables (GRAY, PRE_1, and PRE_2) and Men, Married, Garbage, and Age_mean were statistically significant at the 1% level.
Estimation results: structural model
Estimate | Std. err. | z value | P value | Std. all | |
GRAY | -1.001 | 0.031 | -32.094 | 0.000 | -0.918 |
PRE_1 | -0.080 | 0.023 | -3.457 | 0.001 | -0.067 |
PRE_2 | -0.064 | 0.024 | -2.621 | 0.009 | -0.062 |
Men | -0.107 | 0.032 | -3.387 | 0.001 | -0.062 |
Married | 0.130 | 0.033 | 3.938 | 0.000 | 0.074 |
Education | 0.004 | 0.008 | 0.445 | 0.656 | 0.008 |
Garbage | -0.107 | 0.012 | -8.658 | 0.000 | -0.219 |
Age_mean | -0.030 | 0.007 | -4.433 | 0.000 | -0.110 |
Income_mean | -0.000 | 0.000 | -1.174 | 0.241 | -0.029 |
Forest | 0.008 | 0.005 | 1.537 | 0.124 | 0.255 |
Life_exp_m | -0.026 | 0.019 | -1.357 | 0.175 | -0.027 |
Habitable | 0.008 | 0.005 | 1.597 | 0.110 | 0.266 |
Pop_per | 0.000 | 0.000 | 1.090 | 0.276 | 0.028 |
Goodness-of-fit
The GFIs for the final model are listed in Table 7. Although the value of AGFI (0.784) was slightly lower than 0.8, the values of CFI (0.940), GFI (0.850), and IFI (0.940) were greater than 0.8, and the value of RMSEA (0.072) indicated a fair fit. Therefore, the goodness-of-fit results of the model were acceptable.
Results of goodness-of-fit estimation
Parameter estimates | Rule of thumb | |
CFI | 0.940 | > 0.8 |
GFI | 0.850 | > 0.8 |
AGFI | 0.784 | > 0.8 |
IFI | 0.940 | > 0.8 |
RMSEA | 0.072 | fair fit (0.05-0.08) |
Hypotheses testing and sign conditions
The results of hypotheses testing for the six hypotheses tested in this study are presented in Table 8. All hypotheses were accepted at the 1% significance level. The signs of PRE_1, PRE_2, Garbage, and GRAY were negative, suggesting that H2, H1, H3, and H4 were accepted. The sign of KL_GN was negative, supporting H5. The sign of KL_GY was positive; thus, H6 was also supported.
Hypotheses testing and sign conditions
Variable | Hypothesis | Sign condition | Result |
PRE_2 | H1 | Negative | Accepted*** |
PRE_1 | H2 | Negative | Accepted*** |
Garbage | H3 | Negative | Accepted*** |
GRAY | H4 | Negative | Accepted*** |
KL_GN | H5 | Negative | Accepted*** |
KL_GY | H6 | Positive | Accepted*** |
The sign conditions of the latent variables in the structural model were satisfied, indicating the appropriateness of variable selection in the model. The structural model was constructed in an exploratory manner by selecting control variables. Some control variables were noted to be statistically significant at the 1% level. Among them, the sign for Men was negative, implying that men preferred GN less than women did. The sign for Married was positive, indicating that those who were not married preferred GN to those who were married. Finally, the sign of Age_mean was negative, implying that if the average age of the respondents’ city was low, they preferred GN.
DISCUSSION
Hypotheses testing and implications
Promoting GN could serve as a promising new tool for preventing and mitigating natural disasters under cost restrictions, particularly in depopulated regions. Because GN is nature-based infrastructure that relieves rather than completely prevents natural damage, citizens may suffer lesser damage and issues, such as having to walk on waterlogged roads during rains. By contrast, if GY is adopted, natural damage would not happen as long as the hazards are moderate or low. This study hypothesized that citizens who prefer GN are those who do not take the initiative to learn about it and that some would select GN without recognizing the existence of its heterogeneous risks.
H1, H2, H3, and H4 were accepted, suggesting that the preference of GN (GREEN) is explained by soft measures related to natural disaster prevention and risk reduction activities (PRE_2), hard measures related to the same (PRE_1), knowledge about matters related to garbage disposal (Garbage), and the preference for GY (GRAY). It follows that those who do not prepare for natural disasters, who lack relevant knowledge, and who do not prefer conventional types of disaster prevention infrastructure prefer GN. Further, H5 and H6 were accepted, implying that individuals who prefer GN are those who do not take the initiative to learn about it, while those who favor GY do.
The results of the structural models showed that the signs of observed variables for GREEN and GRAY were the same except for KL_GN and KL_GY. These results are reasonable, considering that GY is a conventional type of infrastructure and people are familiar with it, while GN is a new concept and some citizens may be unfamiliar with it. Despite some similarities regardless of the preference for GN or GY, as the signs of commonly observed variables were the same, those who do not like GY and those who do not take the initiative to learn about GN prefer GN. In other words, the results point to the existence of a distinct category of citizens, Type 4, as categorized in Table 1, and their unrecognized potential risks need to be considered in future policymaking.
Existing studies pointed out that people perceive lower risks if an area is not protected by GY such as dikes, and such a tendency is observed especially among those with high confidence in GY[31,55]. Thus, it follows that those who prefer GY may accept GN without much consideration if GY is not provided. Moreover, because those who prefer GN are those who do not take the initiative to learn about it, they may perceive lower risks in the above situation and may not consider the possible risks of GN. The results of this study imply the necessity of future studies and considerations in implementing real-world policies, as discussed further ahead.
Comparisons with existing studies
In this section, the consistency of the current results with existing studies is described. Existing studies suggest that people’s cognition and/or behavior toward natural disasters may not change until they experience a serious disaster. Some studies have shown that the provision of detailed realistic information (e.g., if a large-scale natural disaster such as a flood occurs in a mega city, there is no possibility of being rescued by helicopter soon because thousands of people will face the same situation) has no impact on people’s consciousness[37]. By contrast, other studies suggest that severe disaster experiences may lead to important changes in citizens’ preferences with regard to trust, risk, and time[35]. For example, some existing studies pointed out that those who had experienced floods take more preparedness actions[31,36], and other studies suggested that those who experience major natural disasters engage in risk-averse behavior[16].
In the case of Japan, most citizens have not experienced severe natural disasters. For example, the number of fatalities, including disaster-related deaths, and missing people due to the 2011 Great East Japan Earthquake was approximately 20,000, while the total population of Japan is 120 million. It is reasonable to assume that the former (i.e., cognition and/or behavior toward natural disasters has not changed yet) was the case in this study; the participants of the study are regarded to reflect the Japanese population because they were randomly selected. The signs of commonly observed variables were the same regardless of their preference for GN and GY, which may attest to the former perspective.
The consistency of the signs of sociodemographic control variables with existing studies was also examined. In this study, the variables Men, Married, Garbage, and Age_mean were statistically significant at the 1% level. In existing studies, the signs of the variables may be sensitive to the context explored. For example, considering activity categories based on gender, infrastructure for leisure, outdoor, and socializing activities was evaluated more positively by women than men, and the same tendency was observed with respect to age in the use of urban green spaces[56]. Some nature-related activities were more important for older adults, but they tended to use fewer urban green spaces for physical activities[56]. However, the results from Hynes et al. showed the opposite trend[57]. Hynes et al. compared gray and green (oyster reef) infrastructures to protect the coast and observed that those over 45 years old were expected to visit sites with more GN, while gender was not a statistically significant variable[57].
The results of this study suggest that men preferred GN less (Men); those who were not married preferred GN (Married); those who had less knowledge on matters related to garbage disposal preferred GN (Garbage); and if the average age of the respondents’ city was low, they preferred GN (Age). Among these four variables, the signs of Men, Married, and Age could be positive or negative, depending on the context, as indicated above, but the sign of Garbage was negative. It was expected that those who had more knowledge about garbage disposal would be concerned about degraded natural environments, including GN. Indeed, the sign for Garbage was negative, indicating that the participants in this study responded as expected.
Policy implications
The frequency and severity of natural disasters have worsened substantially in recent decades worldwide; however, conventional disaster prevention tools, such as GY, are less applicable because of cost constraints, especially in depopulated regions. The idea of utilizing GN appears attractive to both citizens and policymakers because of its abundance and low initial/management costs in depopulated areas. However, this study found that citizens may not recognize the difference between GY and GN and demonstrated that those who prefer GN are citizens who do not take the initiative to learn about it. Citizens’ views and activities are modified through life experiences over time; for example, Baccini and Leemann show that the experience of a flood has a sizeable effect on pro-climate policies throughout voting[58]. However, in the short run and in the context of implementable policies, Type 4 citizens should be treated carefully because their knowledge level is low but their risk level is high. White pointed out a time lag between the acquisition of knowledge and its application and subsequent outcomes[59].
When developing the eco-DRR functions of GN in a region, involvement of local citizens in the processes is highly recommended because it would facilitate the accumulation of citizens’ relevant knowledge and modify their perceptions toward GN. Eco-DRR activities involve the conservation, management, and restoration of the ecosystems of a region[60], which citizens may be asked to proactively join to enhance multiple ecosystem functions through their daily life activities. This may result in the accumulation of relevant and accurate perceptions about GN.
Study limitations and suggestions for future research
This study implicitly assumed that the region that received benefits from GN was the one that developed GN; however, this may not be the case in real-life contexts. For example, activities undertaken in upstream regions close to a river may benefit downstream areas. In such cases, the heterogeneous risk distribution is much more complicated and skewed. Furthermore, the beneficial effects received in a downstream region can be regarded to be technical externality, an effect that directly reaches other regions (agencies) without payment[61]. The internalization of external effects would optimize the GN level in the upstream region, which would conserve the ecosystem and lower disaster risks throughout watershed areas. Future research could investigate more complicated risk distributions and the internalization of external effects in more realistic contexts.
In this study, the possibility that GN and GY could be used complementarily was ignored for simplicity. The number of studies examining the hybrid use of GN and GY has been increasing[62-65]. In real-life situations, GY is often used, especially in urbanized areas, and GN has also been utilized to relieve natural hazards in recent years. The hybrid use of GN and GY makes it possible to reduce the heterogeneous risk distribution in a region and increase cost-effectiveness in more flexible ways. Nevertheless, warning citizens to be cautious about their own disaster risks is essential. The findings of this study suggest that we should emphasize such warnings, especially for Type 4 citizens. Future investigations may consider the hybrid use of GN and GY to further explore typical issues such as cost-benefit and citizens’ risk perceptions.
Other prospects and directions for further research include the following. Altruistic behavior is related to pro-environmental activities[66,67]. For example, Nketiah et al. investigated people’s willingness to pay for energy from local anaerobic digestion schemes using SEM[68]; knowledge and altruistic value were noted as important factors in the study. Future considerations may include the possibility that altruistic behavior affects the selection of GN and GY.
This study considered GN in general and different types of disaster risks were considered when respondents replied to the questionnaire, which made it difficult to apply a moderated mediation model. Future studies may improve the investigation by specifying the types of disasters.
Further, this study proposed measurement models similar to GREEN and GRAY. Its results showed that the signs of all common variables except for knowledge (KL_GN and KL_GY) were the same. Subsequent research may further investigate the reasons for the same signs.
Finally, SEM in this study was conducted in an exploratory manner because the relevant knowledge and amount of existing studies considering GN and citizens’ risk perception toward it remain limited. It is hoped that future explorations will examine more sophisticated hypotheses based on existing literature and contribute to advancing current knowledge.
DECLARATIONS
Acknowledgments
The author is grateful to the editor and five reviewers for the many constructive comments shared as part of the review process.
Author contributions
The author contributed solely to the article.
Availability of data and materials
The datasets generated and/or analyzed during the current study are available from the corresponding author upon reasonable request.
Financial support and sponsorship
This work was supported by JSPS KAKENHI Grant Number JP 21K12357.
Conflicts of interest
The author declared that there are no conflicts of interest.
Ethical approval and consent to participate
Not applicable.
Consent for publication
Not applicable.
Copyright
© The Author 2024.
REFERENCES
1. Klomp J. Financial fragility and natural disasters: an empirical analysis. J Financ Stab 2014;13:180-92.
2. Noth F, Schüwer U. Natural disasters and bank stability: evidence from the U.S. financial system. J Environ Econ Manag 2023;119:102792.
3. Akter M, Cumming D, Ji S. Natural disasters and market manipulation. J Bank Finance 2023;153:106883.
4. Davis KF, Downs S, Gephart JA. Towards food supply chain resilience to environmental shocks. Nat Food 2021;2:54-65.
5. Hua R, Liu Q, Tse Y, Yu Q. The impact of natural disaster risk on the return of agricultural futures. J Asian Econ 2023;87:101632.
6. Vineyard D, Ingwersen WW, Hawkins TR, Xue X, Demeke B, Shuster W. Comparing green and grey infrastructure using life cycle cost and environmental impact: a rain garden case study in Cincinnati, OH. J Am Water Resour Assoc 2015;51:1342-60.
7. Dong X, Guo H, Zeng S. Enhancing future resilience in urban drainage system: green versus grey infrastructure. Water Res 2017;124:280-9.
8. Lima MF, Ward Thompson C, Aspinall P. Friendly communities and outdoor spaces in contexts of urban population decline. Land 2020;9:439.
9. Nakamura F, Ishiyama N, Yamanaka S, et al. Adaptation to climate change and conservation of biodiversity using green infrastructure. River Res Apps 2020;36:921-33.
10. Wu Z, Lei S, Yan Q, Bian Z, Lu Q. Landscape ecological network construction controlling surface coal mining effect on landscape ecology: a case study of a mining city in semi-arid steppe. Ecol Indic 2021;133:108403.
11. Collentine D, Futter M. Realising the potential of natural water retention measures in catchment flood management: trade-offs and matching interests. J Flood Risk Manag 2018;11:76-84.
12. Taylor JR, Hanumappa M, Miller L, Shane B, Richardson ML. Facilitating multifunctional green infrastructure planning in Washington, DC through a tableau interface. Sustainability 2021;13:8390.
13. Korkou M, Tarigan AK, Hanslin HM. The multifunctionality concept in urban green infrastructure planning: a systematic literature review. Urban For Urban Green 2023;85:127975.
14. Dhakal KP, Chevalier LR. Managing urban stormwater for urban sustainability: barriers and policy solutions for green infrastructure application. J Environ Manag 2017;203:171-81.
15. Falco C, Corbi R. Natural disasters and preferences for the environment: evidence from the impressionable years. Econ Lett 2023;222:110946.
16. Ingwersen N, Frankenberg E, Thomas D. Evolution of risk aversion over five years after a major natural disaster. J Dev Econ 2023;163:103095.
17. Venkataramanan V, Lopez D, McCuskey DJ, et al. Knowledge, attitudes, intentions, and behavior related to green infrastructure for flood management: a systematic literature review. Sci Total Environ 2020;720:137606.
18. Chen Y, Guo K, Ji Q, Zhang D. “Not all climate risks are alike”: heterogeneous responses of financial firms to natural disasters in China. Finance Res Lett 2023;52:103538.
19. Otsuka C, Fukutomi H, Niwa Y. Effect of cost-benefit perceptions on evacuation preparedness for urban flood disasters. Int J Disaster Risk Reduct 2022;81:103254.
20. Cai J, Hu S, Que T, Li H, Xing H, Li H. Influences of social environment and psychological cognition on individuals’ behavioral intentions to reduce disaster risk in geological hazard-prone areas: an application of social cognitive theory. Int J Disaster Risk Reduct 2023;86:103546.
21. Bandura A. Social foundations of thought and action: a social cognitive theory. Englewood Cliffs, NJ: Prentice-Hall; 1986.
22. Bandura A, Freeman WH, Lightsey R. Self-efficacy: the exercise of control. New York: Freeman; 1997.
24. Shi J, Xu K, Duan K. Investigating the intention to participate in environmental governance during urban-rural integrated development process in the Yangtze River Delta Region. Environ Sci Policy 2022;128:132-41.
25. Ajzen I. From intentions to actions: a theory of planned behavior. In: Kuhl J, Beckmann J, editors. Action-control: from cognition to behavior. Heidelberg: Springer; 1985. pp. 11-39.
26. Ajzen I. Attitudes, traits, and actions: dispositional prediction of behavior in personality and social psychology. Adv Exper Soc Psychol 1987;20:1-63.
28. Dargin J, Berk A, Mostafavi A. Assessment of household-level food-energy-water nexus vulnerability during disasters. Sustain Cities Soc 2020;62:102366.
29. He J, Yang W, He Q, et al. Chinese pregnant women’s knowledge, attitude, and practice of self-protection against coronavirus disease 2019 during the post-pandemic period: a structural equation modeling-based survey. Int J Disaster Risk Reduct 2023;87:103559.
30. Du S, Scussolini P, Ward PJ, et al. Hard or soft flood adaptation? Advantages of a hybrid strategy for Shanghai. Glob Environ Chang 2020;61:102037.
31. Wachinger G, Renn O, Begg C, Kuhlicke C. The risk perception paradox-implications for governance and communication of natural hazards. Risk Anal 2013;33:1049-65.
32. Adelman M, Ivaschenko O, Packard TG, Suri V. Hardship and vulnerability in the Pacific island countries (English). Washington, DC: World Bank Group; 2015. Available from: http://documents.worldbank.org/curated/en/649891468098658407/Hardship-and-vulnerability-inthe-Pacific-island-countries [Last accessed on 14 Jun 2024].
33. Xu D, Liu Y, Deng X, et al. Earthquake disaster risk perception process model for rural households: a pilot study from Southwestern China. Int J Environ Res Public Health 2019;16:4512.
34. AlQahtany AM, Abubakar IR. Public perception and attitudes to disaster risks in a coastal metropolis of Saudi Arabia. Int J Disaster Risk Reduct 2020;44:101422.
35. Cassar A, Healy A, von Kessler C. Trust, risk, and time preferences after a natural disaster: experimental evidence from Thailand. World Dev 2017;94:90-105.
36. Harries T, Penning-rowsell E. Victim pressure, institutional inertia and climate change adaptation: the case of flood risk. Glob Environ Chang 2011;21:188-97.
37. EMCEI. The 6th Euro-Mediterranean Conference for Environmental Integration, 15-18 May 2024. Marrakesh, Morocco. Available from: https://2024.emcei.net/index.php?p=program [Last accessed on 14 Jun 2024].
38. Rufat S, Tate E, Burton CG, Maroof AS. Social vulnerability to floods: review of case studies and implications for measurement. Int J Disaster Risk Reduct 2015;14:470-86.
39. Wang J, Tsai N. Factors affecting elementary and junior high school teachers’ behavioral intentions to school disaster preparedness based on the theory of planned behavior. Int J Disaster Risk Reduct 2022;69:102757.
40. Spialek ML, Houston JB. The influence of citizen disaster communication on perceptions of neighborhood belonging and community resilience. J Appl Commun Res 2019;47:1-23.
41. Zhang F, Bao X, Deng X, Wang W, Song J, Xu D. Does trust help to improve residents’ perceptions of the efficacy of disaster preparedness? Evidence from Wenchuan and Lushan earthquakes in Sichuan province, China. Int J Environ Res Public Health 2022;19:4515.
42. Wang R, Eckelman MJ, Zimmerman JB. Consequential environmental and economic life cycle assessment of green and gray stormwater infrastructures for combined sewer systems. Environ Sci Technol 2013;47:11189-98.
43. Wu J, Yang X, Deng X, Xu D. Does disaster knowledge affect residents’ choice of disaster avoidance behavior in different time periods? Evidence from China's earthquake-hit areas. Int J Disaster Risk Reduct 2022;67:102690.
44. e-Stat. Portal site for the official statistics of Japan. Available from: https://www.e-stat.go.jp/ [Last accessed on 14 Jun 2024].
46. D'souza C, Taghian M, Lamb P, Peretiatkos R. Green products and corporate strategy: an empirical investigation. Soc Bus Rev 2006;1:144-57.
47. Lim TS, Dzulkifli DZ, Osman Z, Mohidin R, Abdul Jamal AA. Determinants of perception toward life insurance and its impact on intention to purchase. LBIBF 2020;18:16-26.
48. Lim TS, Mail R, Karim MRA, Ulum ZKAB, Miflia M, Jaidia J. An investigation of financial investment intention using covariance-based structural equation modelling. Glob Bus Financ Rev 2020;25:37-50.
49. Oktari RS, Latuamury B, Idroes R, Sofyan H, Munadi K. Validating knowledge creation factors for community resilience to disaster using structural equation modelling. Int J Disaster Risk Reduct 2022;81:103290.
50. R Core Team. The R project for statistical computing. Vienna, Austria: R foundation for statistical computing; 2019. Available from: https://www.R-project.org/ [Last accessed on 14 Jun 2024].
52. Gefen D, Straub D, Boudreau M. Structural equation modeling and regression: guidelines for research practice. Commun Assoc Inf Syst 2000;4.
53. Steiger JH. Understanding the limitations of global fit assessment in structural equation modeling. Pers Individ Dif 2007;42:893-8.
54. Rosseel Y, Jorgensen TD, De Wilde L, et al. Package ‘lavaan.’ Available from: https://cran.r-project.org/web/packages/lavaan/lavaan.pdf [Last accessed on 14 Jun 2024].
55. Botzen WJW, Aerts JCJH, van den Bergh JCJM. Dependence of flood risk perceptions on socioeconomic and objective risk factors. Water Resour Res 2009;45:2009WR007743.
56. Sang ÅO, Knez I, Gunnarsson B, Hedblom M. The effects of naturalness, gender, and age on how urban green space is perceived and used. Urban For Urban Green 2016;18:268-76.
57. Hynes S, Burger R, Tudella J, Norton D, Chen W. Estimating the costs and benefits of protecting a coastal amenity from climate change-related hazards: nature based solutions via oyster reef restoration versus grey infrastructure. Ecol Econ 2022;194:107349.
58. Baccini L, Leemann L. Do natural disasters help the environment? How voters respond and what that means. Political Sci Res Methods 2021;9:468-84.
59. White GF, Kates RW, Burton I. Knowing better and losing even more: the use of knowledge in hazards management. Global Environ Change B Environ Hazards 2001;3:81-92.
60. Estrella M, Saalismaa N. Ecosystem-based disaster risk reduction (Eco-DRR): an overview. In: Renaud F, Sudmeier-Rieux K, Estrella M, editors. The role of ecosystem management in disaster risk reduction. Tokyo: UNU Press; 2013. pp. 26-54.
61. William R, Endres AB, Stillwell AS. Integrating green infrastructure into stormwater policy: reliability, watershed management, and environmental psychology as holistic tools for success. UCLA J Environ Law Policy 2020;38:37-60.
62. Alves A, Vojinovic Z, Kapelan Z, Sanchez A, Gersonius B. Exploring trade-offs among the multiple benefits of green-blue-grey infrastructure for urban flood mitigation. Sci Total Environ 2020;703:134980.
63. Mulligan J, Bukachi V, Clause JC, Jewell R, Kirimi F, Odbert C. Hybrid infrastructures, hybrid governance: new evidence from Nairobi (Kenya) on green-blue-grey infrastructure in informal settlements. Anthropocene 2020;29:100227.
64. Chen W, Wang W, Huang G, Wang Z, Lai C, Yang Z. The capacity of grey infrastructure in urban flood management: a comprehensive analysis of grey infrastructure and the green-grey approach. Int J Disaster Risk Reduct 2021;54:102045.
65. Kim J, Kang J. Development of hazard capacity factor design model for net-zero: evaluation of the flood adaptation effects considering green - gray infrastructure interaction. Sustain Cities Soc 2023;96:104625.
66. de Groot JIM, Steg L. Value orientations to explain beliefs related to environmental significant behavior: how to measure egoistic, altruistic, and biospheric value orientations. Environ Behav 2008;40:330-54.
67. Ucar GK, Kaynak Malatyalı M, Özdemir Planalı G, Kanik B. Personality and pro-environmental engagements: the role of the dark triad, the light triad, and value orientations. Pers Individ Dif 2023;203:112036.
Cite This Article
Export citation file: BibTeX | EndNote | RIS
OAE Style
Kawata Y. Are there possible issues that we overlook when selecting green infrastructure?. Dis Prev Res 2024;3:6. http://dx.doi.org/10.20517/dpr.2023.36
AMA Style
Kawata Y. Are there possible issues that we overlook when selecting green infrastructure?. Disaster Prevention and Resilience. 2024; 3(2): 6. http://dx.doi.org/10.20517/dpr.2023.36
Chicago/Turabian Style
Yukichika Kawata. 2024. "Are there possible issues that we overlook when selecting green infrastructure?" Disaster Prevention and Resilience. 3, no.2: 6. http://dx.doi.org/10.20517/dpr.2023.36
ACS Style
Kawata, Y. Are there possible issues that we overlook when selecting green infrastructure?. Dis. Prev. Res. 2024, 3, 6. http://dx.doi.org/10.20517/dpr.2023.36
About This Article
Copyright
Data & Comments
Data
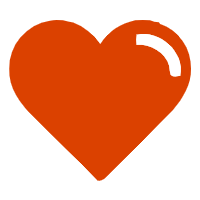
Comments
Comments must be written in English. Spam, offensive content, impersonation, and private information will not be permitted. If any comment is reported and identified as inappropriate content by OAE staff, the comment will be removed without notice. If you have any queries or need any help, please contact us at support@oaepublish.com.