Predictive models for the run-out distance of clay slopes based on material point method
Abstract
This paper aims to propose run-out distance predictive models for clay slopes using the material point method (MPM), which can simulate the progressive failure process of slopes considering the strain softening effect of soils. A suite of 100 ground motions is selected from the NGA-West2 database and then scaled for conducting the dynamic analysis of slopes. The permanent slope displacements (D) can be classified into two categories, namely the “un-failure” category with D smaller than 0.4 m and the “failure” category with D in the range of 10 m to 15 m. It is found that peak ground velocity (PGV) exhibits the highest correlation with D for the “un-failure” category, whereas all ground-motion intensity measures (e.g., PGV, peak ground acceleration) are less correlated with D for the “failure” category. Therefore, the run-out distance of collapsed clay slopes is more related to the failure model rather than the triggering shaking intensities. Moreover, thousands of slope models with various slope angles, slope heights (H), soil densities, and peak and residual strength parameters are developed based on MPM. The run-out distances for the slopes being collapsed are then collected. Predictive models for different slope angles are proposed, which predict the run-out distance as a function of H, unit weight, residual cohesion, and residual friction angle. The proposed models are applicable for clay slopes with slope angles in the range of 30° to 45° and H in the range of 10 m to 30 m.
Keywords
INTRODUCTION
Landslides are devastating geological disasters that frequently cause significant casualties and property damage. Specifically, major earthquakes commonly induce landslides on a large scale. For example, the 2008 Wenchuan earthquake resulted in more than 15,000 landslides[1,2]. To mitigate and prevent the coseismic landslide disasters, it is necessary to conduct the slope stability analysis under seismic excitations.
When assessing the slope stability under seismic loading, the earthquake-induced permanent sliding displacement is typically taken as the metric to evaluate the seismic performance. The original and modified Newmark rigid-block approaches[3-6] have been commonly used to calculate the sliding displacements. For example, Rathje and Saygili[7] proposed a probabilistic framework to calculate the probability of slope failures based on Newmark’s rigid-block approach. Nayek and Gade[8] developed new data-driven prediction models for the sliding displacement based on artificial neural networks. Existing predictive models[9-12] usually predict the permanent displacement as a function of ground motion intensity measures (IMs) and the critical acceleration of the slope, which is determined by its soil parameters (e.g., slope height (H), slope angle, and soil strength).
Based on relatively complicated stress-deformation analysis, several predictive models have been developed to study the dynamic response of slopes in a more accurate way. Fotopoulou and Pitilakis[13] used FLAC2D to calculate the permanent displacements of slopes under earthquake loading and proposed a new displacement model based on the numerical results. Cho and Rathje[14] analyzed the permanent displacements of 49 slopes subjected to more than 1,000 earthquake motions and proposed prediction models for an earthquake-induced permanent displacement of slopes. However, the above studies mainly focus on assessing the seismic stability of slopes while ignoring the post-failure behavior of slopes. In addition, these studies were generally conducted by finite-element or finite-difference numerical approaches, which cannot tackle large-deformation problems given mesh distortions.
The material point method (MPM) proposed by Sulsky[15] is a relatively advanced numerical approach that is capable of solving large-deformation problems. Compared with the finite-element or finite-difference method, the MPM approach adopts the Lagrange particles with material information and Euler mesh. The Euler mesh is used for effectively solving the momentum equations only. Therefore, the MPM approach can avoid the mesh distortions and has been increasingly used in a variety of applications[16-20], such as the simulation of a massive Hongshiyan landslide triggered by the 2014 magnitude 6.5 Ludian earthquake in China based on the coupled SEM-MPM method[21]. Liu et al. proposed a chart tool to estimate the run-out distance of slopes based on MPM[22].
This paper, therefore, aims to propose MPM-based prediction models for estimating the earthquake-induced run-out distance of clay slopes, which can estimate the run-out distance of slopes based on soil strength and geometry parameters. The remaining part of this paper is structured as follows. First, the MPM approach is introduced in detail, and the failure process of a clay slope under seismic loading, considering the soil strain softening effect, is simulated. Second, the earthquake-induced horizontal displacement of slopes is taken as the representative engineering demand parameter, and the influence of seismic loading characteristics on the deformation of slopes is analyzed. The prediction model of the run-out distance of homogeneous clay slopes is proposed based on numerical simulation results, and conclusive remarks are provided finally.
MATERIAL POINT METHOD
Theory and method
In MPM, all material properties are carried by particles, and no information is stored on the grid nodes. The deformed grids are discarded at the end of each time step, and a new grid system would be utilized to solve the equilibrium equations in the following time step. In other words, the grids are only used to solve the momentum equations in MPM. Therefore, MPM can effectively avoid mesh-distortion problems. The calculation process of the MPM scheme is illustrated in Figure 1.
Figure 1. The calculation process of MPM at each time step: (A) initial configuration; (B) incremental deformation; and (C) resetting background mesh.
The mass conservation equations based on the Lagrange scheme are illustrated as[23]:
where ρ is the density of the particle; v is the particle velocity; σ is the Cauchy stress tensor; b is the unit mass force of the particle; and
where the definitions of the parameters are the same as those in Equations (1) and (2).
Simulation of seismic slope failure
The open-source code in MPM was modified to simulate seismic slope failure[23]. The schematic representation of the numerical slope model based on MPM is shown in Figure 2, in which L1 (i.e., distance from slope toe to the right boundary) and L2 (i.e., distance from slope toe to the left boundary) are 100 m and 50 m, respectively, and the depth of basement (H2) is twice the height of slope (H1) to minimize the wave reflections from the slope boundaries. The cell size is 1 m × 1 m × 1 m, with each cell containing four material points. The space of the material points is 0.5 m. The acceleration of gravity increases linearly from 0 to 9.8 m/s2.
In the current MPM model, the free-field condition is assigned to both sides to absorb the seismic waves. The Lysmer-Kuhlemeyer transmitting boundary condition is added at the bottom of the slope model to simulate the finite rigidity of the underneath bedrock layer. Such formulation of transmitting boundaries can be found in existing studies (e.g., Feng et al.[19]). The reasonableness of the above boundary conditions can be verified by a one-dimensional s-wave propagation test on an elastic column, with the diagram and monitoring points illustrated in Figure 3. The heights of these monitoring points (i.e., A1 to A5) are 0 m,
Figure 4 shows the recorded wave velocities at five monitoring points when the bottom of the elastic column is excited by a sinusoidal wave with the amplitude of
Figure 4. (A) The input sinusoidal wave; and the recorded wave velocities at (B) point A1; (C) point A2; (D) point A3; (E) point A4; and (F) point A5, respectively.
Therefore, the whole process of the earthquake-induced slope failure is simulated based on the modified MPM. The slope model introduced by Feng et al. is considered herein as the demonstrated example, which is shown in Figure 5[19]. Five monitoring points (i.e., D1 to D5) are also considered in the slope model, with the heights of these points as 0 m, 5 m, 10 m, 15 m, and 20 m, respectively.
The Drucker-Prager strain softening constitutive model[22] is used herein to describe the dynamic behavior of soils. The bedrock material is modeled as rigid. The formulations of the soil strain softening model are expressed as follows[19]:
where cp, φp, and Ψp are peak strength parameters, and cr, φr, and Ψr are the residual strength parameters; εp and η are the equivalent plastic strain and strain-softening parameters, respectively. The values of some slope parameters are summarized in Table 1. The transmitting boundary is added at the bottom of the bedrock, and then a sinusoidal wave with peak ground acceleration (PGA) of 0.2 g (shown in Figure 6) is applied to the boundary of the slope model. Figure 7 shows the displacement-time histories recorded at the five monitoring points (i.e., D1 to D5). As expected, the displacements of monitoring points in the upper part of the slope (i.e., D4 and D5) are much larger than those of the other monitoring points.
The values of slope parameters assigned
Young’s modulus E (MPa) | Poisson’s ratio v | Soil density (kN/m3) | Peak cohesion cp (kPa) | Residual cohesion cr (kPa) | Peak friction angle φp (°) | Residual friction angle φp (°) | Softening parameter η | Dilation angle Ψp (°) |
187 | 0.3 | 18 | 10 | 1 | 40 | 30 | 20 | 0 |
The whole slope deformation process is represented by the equivalent plastic strain contours at different time slots, as shown in Figure 8. At first, the slope is in a static condition, and the input wave is excited at
SLOPE DISPLACEMENT ANALYSIS BASED ON MPM
Influence of ground-motion IMs on slope displacement
In this section, the MPM approach introduced above is used to simulate the whole dynamic failure process of a 15-m-high clay slope, which is schematically shown in Figure 2. The geometry and soil strength parameters of the slope are enlisted in Table 2. The relationship between the maximum permanent horizontal displacement (D) and ground-motion IMs is then analyzed based on the calculated results.
Parameters assigned to the slope for conducting the numerical analysis
Height of slope H1 (m) | Depth of basement H2 (m) | Slope angle β (°) | Soil density (kN/m3) | Peak cohesion (kPa) | Peak friction angle (°) | Residual cohesion (kPa) | Residual friction angle (°) |
15 | 30 | 45 | 20 | 40 | 20 | 6 | 10 |
Subsequently, 100 ground motion records are selected from the NGA-West2 database (Ancheta et al.[24]). Figure 9A shows the distribution of the spectral acceleration of the selected motions, and the median PGA value of these ground motions is 0.29 g. Figure 9B shows the rupture distance and moment magnitude of the selected ground motions. The moment magnitudes and rupture distances of the selected ground motions vary from 5.17 to 7.9 and from 0.21 km to 36.34 km, respectively. These original ground motions are further scaled so that a total of 200 acceleration-time histories are obtained for conducting the dynamic analysis. Furthermore, IMs, including PGA, peak ground velocity (PGV), cumulative absolute velocity (CAV), and Arias intensity (Ia) of these motions, are calculated. Figure 10 shows the dynamic process of the slope when subjected to the ground motion record with the record sequence number (RSN) of 143 in the NGA-West2 database. A permanent maximum displacement of 12 m is observed at the end of shaking. Consequently, based on the 200 acceleration-time histories collected, dynamic analyses are conducted for the above slope model, resulting in 200 displacement data points in total.
Figure 9. (A) Spectral distributions of the selected ground motions; (B) the rupture distance and moment magnitude of selected ground motions.
Figure 10. The displacement contours of the 15-m-high clay slope for (A) t = 15 s; (B) t = 20 s; (C) t = 30 s; and (D) t = 55 s, respectively.
Figure 11 illustrates the distribution of D vs. PGV for the 15-m-high clay slope based on the 200 acceleration-time histories collected. Taking the maximum D of 0.4 m as the threshold, we divide the displacement data into two categories, representing the small and large deformations of the slope, respectively. The slope could be considered as “un-failure” when the maximum D is less than 0.4 m. Figure 12A shows the relationship between the maximum D and PGV for the small-deformation group of the slope. It can be observed that the slope displacements generally increase with the increase of PGV. The relationship between the permanent displacement and PGV for the large-deformation group of the slope is illustrated in Figure 12B, from which it can be seen that the maximum permanent displacements are distributed in the range of 10 m to 15 m. Such large deformation indicates that the slope is at the complete "failure" status.
Figure 11. The distribution of the permanent horizontal displacement D vs. PGV for the 15-m-high clay slope based on the 200 acceleration-time histories collected.
Figure 12. The relationships between D and PGV for the 15-m-high clay slope at the (A) un-failure state and (B) failure state, respectively.
The results shown in Figure 12 clearly demonstrate that at the un-failure (i.e., small deformation) state, the permanent displacement of a slope generally increases with increasing PGV, whereas PGV (or the ground-motion intensity) has a relatively minor influence on the permanent displacement once the slope is at the complete failure state. This is anticipated because the permanent displacement of collapsed slopes is mainly determined by the failure modes rather than the triggering shaking intensities.
Figure 13 shows the correlation coefficient results of the maximum D for the slope with four ground motion IMs, namely PGA, PGV, Ia, and CAV. For the “un-failure” category, the calculated correlations are in the range of 0.29 to 0.87, in which PGA and PGV exhibit the lowest and highest correlations, respectively. This observation is generally consistent with existing studies[25]. On the other hand, for the "failure" category, the calculated correlations are in the range of 0.18 to 0.55, in which PGA and Ia exhibit the lowest and highest correlations, respectively. As expected, the correlations for the displacements of the "failure" category are significantly lower than those of the "un-failure" category. Consequently, it is not feasible to derive the IM-based predictive model for estimating D of the "failure" category, while on the other hand, PGV could be considered as the appropriate predictor variable for predicting D for the “un-failure” category.
Figure 13. The correlation coefficients between the permanent horizontal displacement and ground motion IMs for the slope considered.
Based on the results shown in Figure 12A, a two-order polynomial model is utilized to regress the displacement data based on PGV and expressed as:
where D denotes the permanent horizontal displacement of the slope in the unit of m, and PGV is in the unit of cm/s. The standard deviation of the polynomial model is 0.09 m. This model can be used to estimate D for the 15-m-high clay slope, considering the soil strain softening effect. The calculated displacement can be further used to reflect the damage states (i.e., minor, moderate, or severe) of un-failure slopes.
Influence of soil strength parameters on the slope displacement
The influence of the strain softening effect on the D of slopes is first analyzed. The ground motion record with the RSN of 1080 in the NGA-West2 database is considered herein, which is further scaled to 0.05 g,
Figure 14. The maximum permanent horizontal displacements of the clay slope considering both the strain-softening and non-strain-softening constitutive models, respectively.
The influences of the peak and residual soil strength values on the magnitude of D are subsequently investigated. After that, we developed five slope models with different peak and residual soil strength parameters based on the above 15-m-high clay slope to analyze the influence of peak and residual soil strength parameters on D. The peak and residual soil strength parameters assigned for slopes I-V are shown in Table 3. As listed in this table, different peak and identical residual strength parameters are assigned for slopes I-III, whereas identical peak and different residual strength parameters are assigned for slopes of I, IV, and V. The ground motion record with the RSN of 125 in the NGA-West2 database is considered as the input motion herein, and its acceleration-time history is shown in Figure 15.
The peak and residual soil strength parameters of slopes I-V
ID | Peak cohesion cp (kPa) | Peak friction angle φp (°) | Residual cohesion cr (kPa) | Residual friction angle φr (°) |
Slope I | 12.5 | 27.5 | 10 | 20 |
Slope II | 15 | 30 | 10 | 20 |
Slope III | 17.5 | 32.5 | 10 | 20 |
Slope IV | 12.5 | 27.5 | 7.5 | 17.5 |
Slope V | 12.5 | 27.5 | 5 | 15 |
Figure 16A and B shows the maximum displacement-time histories for comparing the results of slopes I-III and slopes I, IV, and V, respectively. The permanent displacements of slope I-V are 2.87 m, 2.51m, 2.12 m, 5.91 m, and 8.04 m, respectively. From Figure 16A, it is clear that the variation of the peak strength parameters has a minor influence on the magnitude of D. On the other hand, as shown in Figure 16B, as the residual strength parameters decrease gradually, the permanent slope displacement increases dramatically. Specifically, when cr and φr decrease from 10 kPa to 5 kPa and from 20° to 15°, respectively, the D values increase from 2.87 m to 8.04 m. It is, therefore, indicated that the post-failure deformation of clay slopes is predominately determined by the residual strength parameters rather than the peak strength parameters.
PREDICTIVE MODELS FOR THE RUN-OUT DISTANCE OF CLAY SLOPES
Besides the parameter D as introduced above, the run-out distance of the collapsed slopes, termed Lf hereafter, is also an important parameter in slope engineering. The information of Lf is important for conducting the risk assessment of landslide hazards, such as the chart method for estimating the run-out distance of slope under excavation proposed by Liu et al.[22]. Note that Lf is defined as the horizontal distance between the furthest slope material point and the slope toe when the slope is completely damaged.
In this section, the relationship between two dimensionless parameters, Lf /H and crtanφr/γH, is developed, which is conceptually similar to Taylor’s slope stability chart[26]. A number of numerical analyses are conducted herein to generate adequate data of Lf. Specifically, thousands of slope models with various slope angles, H, soil densities, and peak and residual strength parameters are developed based on MPM. The values considered for the geometry and strength parameters are summarized in Table 4. Specifically, the clay slopes with heights ranging from 10 m to 30 m and slope angles in the range of 30° to 45° are modeled. The ratios of peak soil strength to residual soil strength values (e.g., cr/cp) are assigned as 0.4 and 0.7, respectively. The strain parameter η is assigned a value of 20. The elastic modulus and Poisson’s ratio are assigned constant values of 100 MPa and 0.35, respectively. The number of the developed slope models is 1,152 (3 × 4 × 3 × 4 × 4 × 2 = 1,152). Note that the combination of different soil strength and geometry parameters yields 150 statically unstable cases, which are eliminated from the slope database. In addition, since seismic shaking intensities have a minor effect on the magnitude of Lf, a sinusoidal wave, rather than real acceleration-time series, is taken as the input seismic loading. The sinusoidal wave having the PGA value of 0.5 g and duration of 20 s, as shown in Figure 6, is utilized herein.
The values of geometry and strength parameters for generic clay slopes
Slope height H (m) | Slope angle β (°) | Soil density γ (kN/m3) | Peak cohesion cp (kPa) | Peak friction angle φp (°) |
10 | 30 | 18 | 10 | 25 |
20 | 35 | 20 | 20 | 30 |
30 | 40 | 22 | 30 | 35 |
- | 45 | - | 40 | 40 |
Dynamic analyses are thus conducted for the 1,002 statically stable slope models subjected to the sinusoidal wave. The input wave is strong enough to make all slope cases collapse. Therefore, approximately 1,000 sets of Lf are obtained, which are subsequently used for developing the predictive model.
Figure 17 shows the relationships between the two dimensionless parameters (Lf/H and crtanφr/γH) for different slope angles. It shows that there is an obvious linear trend between Lf/H and crtanφr/γH for each slope angle. Therefore, it seems feasible to perform the regression process for individual slope angles. The empirical data are then classified based on the four slope angles considered, and multiple empirical models are proposed for predicting Lf/H based on simple linear regression. As shown in Figure 17, the fitting curves match the empirical data reasonably well, with the resultant coefficients of determination (R2) values in the range of 0.80 to 0.85. Consequently, the predictive models for Lf for different slope angles have the functional forms:
Figure 17. The relationships between Lf /H and crtanφr/γH for clay slopes with (A) β = 45°; (B) β = 40°; (C) β = 35°; and (D) β = 30°, respectively.
in which Lf is the run-out distance of the collapsed slopes in the unit of m; cr is the residual cohesion in the unit of kPa; φr is the peak angle of friction; γ is the soil density in the unit of kN/m3; H is the slope height in the unit of m; and B0 and B1 are the regression coefficients, respectively. Table 5 summarizes the coefficients of B0 and B1, together with the standard errors of the proposed models corresponding to different slope angles.
Coefficients of the proposed predictive models of run-out distance
Slope angle (°) | B0 | B1 | Standard error of regression (m) |
45 | 49.55 | 1.22 | 1.34 |
40 | 71.73 | 1.29 | 1.49 |
35 | 99.13 | 1.42 | 1.61 |
30 | 113.78 | 1.40 | 1.45 |
Figure 18 shows the fitting curves of Lf/Hvs.crtanφr /γH for different slope angles. As expected, a larger slope angle corresponds to a larger run-out distance for a given magnitude of crtanφr /γH. In addition, as listed in Table 5, the coefficients of B0 and B1 generally decrease with increasing slope angles. Therefore, for a slope angle different from these four values (in the range of 30° to 45°), a linear interpolation approach can be used to estimate the approximate coefficients and the run-out distance.
DISCUSSION
The process of the homogeneous soil slope progressive failure is simulated by the MPM, and the relationships between the permanent slope displacement and ground motion IMs are investigated. It is found that the displacement data can be classified into two categories, namely the “un-failure” category with D smaller than 0.4 m and the "failure" category with D in the range of 10 m to 15 m. For the “un-failure” category, the slope is at the stable state, and D generally increases with the increase of ground-motion intensities such as PGV. On the other hand, for the "failure" category, the permanent displacements are less correlated with the ground-motion intensities. Based on the above results, predictive models are proposed to estimate the run-out distance of generic clay slopes. The predictor variables employed include H, γ, cr, and φp. In engineering applications, the two residual strength parameters can be determined by either conducting shear tests or using empirical formulas (e.g., Kanji[27]).
The coefficient of friction is determined by the cohesions and friction angles of soil mass in MPM. Therefore, the proposed models consider the effect of the friction coefficient on the run-out distance. The proposed models can predict the run-out distances based on the slope geometry and strength parameters, which is similar to some existing predictive models for the run-out distance of a slope[28-33]. However, existing predictive models were commonly developed based on the statistical methods and available landslide inventory data, incorporating the parameters of H and the sliding mass volume in the functional forms. The run-out distances of clay slopes can be estimated based on the proposed models, which could help to conduct the seismic risk-based design of slopes and take appropriate measures for reducing the seismic risk of landslides.
CONCLUSION
The whole failure process of homogeneous clay slopes was simulated based on MPM in this study. The Drucker-Prager strain softening constitutive model is used to consider the strain softening effect and describe the dynamic behavior of soils under seismic excitations. A suite of 100 ground motions is first selected from the NGA-West2 database. Dynamic analyses are then conducted based on these motions for a 15-m-high clay slope. The calculated permanent displacement data are classified into two groups. Specifically, when a slope is at the un-failure state (i.e., D < 0.4 m), the slope displacement generally increases with increasing ground motion IMs such as PGA, PGV, and Ia. PGV exhibits the highest correlation with D for the “un-failure” group, and then a PGV-based predictive model is developed to regress the displacement data. The predicted D values can be used to reflect the damage states of clay slopes with given PGV ordinates. When a slope is at the failure state (i.e., D is in the range of 10 m-15 m), the D values are less influenced by the magnitude of ground motion IMs. It is thus indicated that when an earth slope is collapsed, its permanent run-out distance is mainly determined by the failure mode rather than the triggering shaking intensity. Note that this conclusion may be inappropriate for some slope types, such as a high-speed and long-distance landslide, in which the run-out distance is closely related to the earthquake intensity.
The influence of soil strength parameters on the slope displacement is also investigated. First, using the MPM model incorporating the strain softening effect of soils brings in a notable increase of D when the slope loses its stability. When subjected to the excitation of PGA as 0.4 g, the calculated D values are 11.7 m and 0.44 m when considering and neglecting the soil strain softening effect, respectively. Therefore, it is necessary to consider the strain softening effect in the modeling of the failure process of slopes. Second, it is found that the permanent deformation of slopes is mainly influenced by the residual strength parameters rather than peak strength parameters.
Thousands of slope models with various slope angles, H, γ, and peak and residual strength parameters are developed based on MPM. Dynamic analyses are conducted for these slope models, and the run-out distances for the slopes being collapsed are collected. The relationships between two dimensionless parameters, Lf /H and crtanφr /γH, are then investigated based on the results collected. Four predictive models for different slope angles are proposed, respectively. The models predict Lf as a function of H, γ, cr, and φr, and the R2 values calculated are larger than 0.8, indicating good predictive efficiency of the proposed models. The proposed models can be used to estimate the run-out distances for clay slopes with slope angles in the range of 30° to 45° and H in the range of 10 m to 30 m.
DECLARATIONS
Authors’ contributions
Made substantial contributions to the conception and design of the study and performed data analysis and interpretation: Zhao Y, Du W
Performed data acquisition and provided administrative, technical, and material support: Wu Q
Availability of data and materials
All data, models, or codes that support the findings of this study are available from the corresponding author upon reasonable request.
Financial support and sponsorship
This work was supported by the National Natural Science Foundation of China (Grant numbers U2240211 and 52078393) and the Fundamental Research Funds for the Central Universities (No. 2042023kfyq03).
Conflicts of interest
All authors declared that there are no conflicts of interest.
Ethical approval and consent to participate
Not applicable.
Consent for publication
Not applicable.
Copyright
© The Author(s) 2023.
REFERENCES
1. Huang D, Wang G, Du C, Jin F, Feng K, Chen Z. An integrated SEM-Newmark model for physics-based regional coseismic landslide assessment. Soil Dyn Earthq Eng 2020;132:106066.
2. Zhao X, Hu K, Burns SF, Hu H. Classification and sudden departure mechanism of high-speed landslides caused by the 2008 Wenchuan earthquake. Environ Earth Sci 2019;78:125.
3. Du W, Huang D, Wang G. Quantification of model uncertainty and variability in Newmark displacement analysis. Soil Dynam Earthq Eng 2018;109:286-98.
4. Du W, Wang G, Huang D. Evaluation of seismic slope displacements based on fully coupled sliding mass analysis and NGA-West2 database. J Geotech Geoenviron Eng 2018;144:06018006.
5. Wang M, Li D, Du W. Probabilistic seismic displacement hazard assessment of earth slopes incorporating spatially random soil parameters. J Geotech Geoenviron Eng 2021;147:04021119.
6. Li D, Wang M, Du W. Influence of spatial variability of soil strength parameters on probabilistic seismic slope displacement hazard analysis. Eng Geol 2020;276:105744.
7. Rathje EM, Saygili G. Probabilistic assessment of earthquake-induced sliding displacements of natural slopes. BNZSee 2009;42:18-27.
8. Nayek PS, Gade M. Artificial neural network-based fully data-driven models for prediction of newmark sliding displacement of slopes. Neural Comput Appl 2022;34:9191-203.
9. Bray JD, Travasarou T. Simplified procedure for estimating earthquake-induced deviatoric slope displacements. J Geotech Geoenviron Eng 2007;133:381-92.
10. Jibson RW. Regression models for estimating coseismic landslide displacement. Eng Geol 2007;91:209-18.
11. Rathje EM, Antonakos G. A unified model for predicting earthquake-induced sliding displacements of rigid and flexible slopes. Eng Geol 2011;122:51-60.
12. Feng W, Lu Z, Yi X, Dong S. A dynamic method to predict the earthquake-triggered sliding displacement of slopes. Math Probl Eng 2021;2021:1-11.
13. Fotopoulou SD, Pitilakis KD. Predictive relationships for seismically induced slope displacements using numerical analysis results. Bull Earthq Eng 2015;13:3207-38.
14. Cho Y, Rathje EM. Generic predictive model of earthquake-induced slope displacements derived from finite-element analysis. J Geotech Geoenviron Eng 2022;148:04022010.
15. Sulsky D, Chen Z, Schreyer HL. A particle method for history-dependent materials. Comp Methods Appl Mech Eng 1994;118:179-96.
16. Nguyen TS, Yang K, Wu Y, Teng F, Chao W, Lee W. Post-failure process and kinematic behavior of two landslides: case study and material point analyses. Comput Geotech 2022;148:104797.
17. Wang B, Vardon P, Hicks M. Investigation of retrogressive and progressive slope failure mechanisms using the material point method. Compu Geotech 2016;78:88-98.
18. Qu C, Wang G, Feng K, Xia Z. Large deformation analysis of slope failure using material point method with cross-correlated random fields. J Zhejiang Univ Sci A 2021;22:856-69.
19. Feng K, Wang G, Huang D, Jin F. Material point method for large-deformation modeling of coseismic landslide and liquefaction-induced dam failure. Soil Dyn Earthq Eng 2021;150:106907.
20. Troncone A, Pugliese L, Conte E. Analysis of an excavation-induced landslide in stiff clay using the material point method. Eng Geol 2022;296:106479.
21. Feng K, Huang D, Wang G, Jin F, Chen Z. Physics-based large-deformation analysis of coseismic landslides: a multiscale 3D SEM-MPM framework with application to the Hongshiyan landslide. Eng Geol 2022;297:106487.
22. Liu L, Zhang P, Zhang S, et al. Efficient evaluation of run-out distance of slope failure under excavation. Eng Geol 2022;306:106751.
23. Zhang X, Lian YP, Liu Y, Zhou X. Material point method. Beijing: Tsinghua University Press; 2013. pp. 15-26.
25. Zhang J, Chen S, Wang T, Wu F. Study on correlation between ground motion parameters and soil slope seismic response. Bull Eng Geol Environ 2022;81:226.
26. Taylor DW. Stability of earth slopes. J Boston Soc Civil Eng 1937;24:197-246. Available from: https://www.bscesjournal.org/journal/volume-24-number-3-july-1937/ [Last accessed on 30 June 2023]
27. Kanji MA. The relationship between drained friction angles and Atterberg limits of natural soils. Géotechnique 1974;24:671-4.
28. Qiu H, Cui P, Hu S, Regmi AD, Wang X, Yang D. Developing empirical relationships to predict loess slide travel distances: a case study on the Loess Plateau in China. Bull Eng Geol Environ 2018;77:1299-309.
29. Guo D, Hamada M, He C, Wang Y, Zou Y. An empirical model for landslide travel distance prediction in Wenchuan earthquake area. Landslides 2014;11:281-91.
30. Ma Z, Liao H, Dang F, Cheng Y. Seismic slope stability and failure process analysis using explicit finite element method. Bull Eng Geol Environ 2021;80:1287-301.
31. Pu X, Wan L, Wang P. Initiation mechanism of mudflow-like loess landslide induced by the combined effect of earthquakes and rainfall. Nat Hazards 2021;105:3079-97.
32. Zhang Z, Zeng R, Meng X, et al. Estimating landslide sliding distance based on an improved Heim sled model. CATENA 2021;204:105401.
Cite This Article
Export citation file: BibTeX | EndNote | RIS
OAE Style
Zhao Y, Wu Q, Du W. Predictive models for the run-out distance of clay slopes based on material point method. Dis Prev Res 2023;2:11. http://dx.doi.org/10.20517/dpr.2023.14
AMA Style
Zhao Y, Wu Q, Du W. Predictive models for the run-out distance of clay slopes based on material point method. Disaster Prevention and Resilience. 2023; 2(2): 11. http://dx.doi.org/10.20517/dpr.2023.14
Chicago/Turabian Style
Yuhan Zhao, Qiang Wu, Wenqi Du. 2023. "Predictive models for the run-out distance of clay slopes based on material point method" Disaster Prevention and Resilience. 2, no.2: 11. http://dx.doi.org/10.20517/dpr.2023.14
ACS Style
Zhao, Y.; Wu Q.; Du W. Predictive models for the run-out distance of clay slopes based on material point method. Dis. Prev. Res. 2023, 2, 11. http://dx.doi.org/10.20517/dpr.2023.14
About This Article
Copyright
Data & Comments
Data
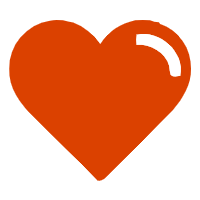
Comments
Comments must be written in English. Spam, offensive content, impersonation, and private information will not be permitted. If any comment is reported and identified as inappropriate content by OAE staff, the comment will be removed without notice. If you have any queries or need any help, please contact us at support@oaepublish.com.