Revamping medical coding with AI: a systematic review of interdisciplinary applications and perspectives for plastic surgery
Abstract
Aim: This review evaluates the use of artificial intelligence (AI), machine learning (ML), and natural language processing (NLP) technologies for enhancing current procedural terminology (CPT) coding accuracy and efficiency in plastic and reconstructive surgery and related disciplines to define a precedent for future implementation.
Methods: A systematic search of PubMed, Scopus, and Web of Science Core Collection was performed to identify studies that leveraged artificially intelligent technologies in coding related to surgical procedures commonly managed by plastic and reconstructive surgeons.
Results: 11 peer-reviewed articles, which encompassed more than 1,000 CPT codes across numerous surgical subspecialties with overlap in plastic and reconstructive surgery and model systems, were included. The key findings highlight that AI-driven models demonstrate high sensitivity, specificity, area under the receiver operating curve (AUROC), and accuracy. While performance metrics varied considerably depending on the specific AI model employed, these systems were found to be effective assistive technologies for medical coding. Studies underscored the advantages of integration, maximizing billing workflow and reducing administrative workload. However, studies of AI performance for billing and medical coding within plastic surgery settings specifically were sparse. Notably, these investigations emphasized the need to tailor models for targeted suitability.
Conclusions: This review highlights the potential of AI technologies to improve CPT coding by enabling time and resource management and ultimately combatting the mounting presence of surgeon burnout. The sparsity of plastic surgery-specific literature on this emerging topic and untested promise in the specialty calls for intentional plastic surgeon-driven initiatives in the development of such applications.
Keywords
INTRODUCTION
The innovative nature of plastic and reconstructive surgery makes the accurate assignment of current procedural terminology (CPT) codes critical to ensure correct reimbursement, drive quality improvement, and effectively manage surgical treatment[1]. Medical coding in surgical departments has traditionally been a complex process requiring detailed documentation, influenced by consistently changing coding guidelines. CPT code assignment is an ongoing subject of debate, impacted by regulatory oversight and susceptible to human error and inconsistencies[2,3].
Recent works have highlighted the challenges inherent in predicting CPT codes solely through manual efforts, underscoring the need for novel solutions to enhance accuracy and efficiency in this domain[4]. Emerging technologies, particularly artificial intelligence (AI) applications utilizing machine learning (ML) and natural language processing (NLP), have given way to an innovative frontier for improving coding practices[5]. New studies have documented the ability of various AI/ML/NLP methods to not only serve as an administrative assistant, but also generate CPT codes based on patient operative notes in the hospital setting[6].
ML is a subdivision of AI that relies upon algorithms and statistical models to enable computers to learn from and make predictions or decisions based on input data. Instead of being explicitly programmed to perform a task, ML systems are trained on a large amount of data, enabling them to improve their utility through repetitive, automated decision-making processes. Common applications of ML include recommendation systems, image recognition, and predictive analytics. For instance, studies employing a random forest (RF) classification model have demonstrated the ability to achieve 74%-76% accuracy in predicting primary CPT codes by integrating both structured and unstructured data[7]. Furthermore, advanced algorithms have shown the potential to enhance predictive performance by reorganizing possible CPT codes based on identified key features, achieving a remarkable 20%-35% improvement in outcome quality[7].
NLP promotes computers to comprehend, interpret, and produce human language in a way that is useful to the user experience, including tasks such as language translation, sentiment analysis, speech recognition, and text summarization. The application of NLP techniques has gained attention for their capability to streamline and standardize the codification process, reducing the burden of substantiating manual coding while minimizing the likelihood of potential error[8,9]. Notably, analyses of common musculoskeletal CPT codes indicate that traditional NLP approaches can outperform more complex models like BERT, achieving an accuracy rate of 97% and offering crucial interpretability for clinical applications[10].
Time spent on billing and administrative tasks largely detracts from the ability to allocate adequate time toward patient care, whether in the clinical or operative setting, breeding burnout that heightens the risk of medical errors. Mistakes not only lead to improper surgical outcomes, but also under-compensation for surgical services[11,12]. With the utility of AI undergoing rapid improvement and continued integration into the medical setting, the possibility of automating CPT code assignment by reading operative notes or listening to dictations has piqued interest. Reducing administrative coding tasks can reallocate surgeons’ time to direct patient care, enhancing overall patient outcomes.
This review aims to synthesize insights from the literature on the deployment of AI, ML, and NLP technologies for algorithm-dependent CPT code assignment applicable to the field of plastic and reconstructive surgery. By examining metric-based performance, as well as current challenges and advancements, this work leverages perspective on the transforming mode by which plastic and reconstructive surgeons may engage with their practice and their patients, amidst a shifting technological landscape in the broader surgical community.
METHODS
Study design
This review follows the standard protocol for preferred reporting items for systematic reviews and meta-analyses, providing a general overview of the applicable existing literature. This study design was selected with the goal of ultimately identifying gaps and growing opportunities for the utilization of AI, ML, and NLP in the proper assignment of CPT codes for plastic surgery procedures.
Search strategy
A systematic search was conducted across three databases exclusively: PubMed, Scopus, and Web of Science Core Collection. These databases were selected due to their extensive coverage of AI applications in medicine. Database results were sought to collect a comprehensive pool of relevant peer-reviewed articles published prior to September 10th, 2024. Search terminology included “Artificial Intelligence”, “Natural Language Processing”, or “Machine Learning”, combined with “CPT” and “Surgery”.
Inclusion and exclusion criteria
The inclusion criteria for screening peer-reviewed articles from database searches focused on studies examining the application of AI, ML, or NLP technologies in CPT coding for surgical procedures commonly managed by plastic and reconstructive surgeons. The exclusion criteria for full-text screening eliminated literature that did not specifically discuss the use of AI, ML, or NLP technologies in CPT code assignment, opinion pieces or commentaries, and studies written in a non-English language without an English translation available.
Data extraction
Data from the 11 identified studies were extracted using a standardized form that recorded authorship, publication year, procedure or subspecialty area of plastic and reconstructive surgery, AI/ML/NLP methods employed (with technology specified), coding accuracy results (with preservation of measurements in the original study format), sample sizes (operative notes, patients, and/or procedures), involved CPT codes, and key findings (unstructured qualitative). No additional variables were considered.
Quality assessment and data analysis
Two authors (M.L. and T.M.) independently screened peer-reviewed articles for eligibility, where each author was blinded to the inclusion/exclusion decisions of the other until the screening process had concluded. Peer-reviewed literature that then underwent data extraction was evaluated for adequate sample size to substantiate study conclusions, appropriate methodology for chosen study design, and adherence to reporting guidelines specific to study type. The role of AI/ML/NLP technologies was evaluated for accuracy and efficiency in CPT code application, advantages and disadvantages in clinical and administrative workflow, and indirect facets implicated in a plastic and reconstructive surgeon’s ability to provide patient care. Sources were cross-compared to understand innovations and perceptions in terms of chronological trends regarding the implementation of AI, ML, or NLP. Any studies that did not contain all variables outlined for data extraction were manually removed from the final pool of included literature.
Data were grouped such that study identifiers (publication year, procedure/subspecialty, AI/ML/NLP methods, CPT codes), corresponding quantitative characteristics (sample sizes) and results (accuracy measurements) could be synthesized into tables using Microsoft Excel. Figures were generated from qualitative results to visually represent the key findings in a collective manner: Graphically (Microsoft Excel) and diagrammatically (Biorender.com). These methods for representation were selected to best preserve extracted data in the format in which they originated.
RESULTS
Of 123 unique database search results, 11 articles met the eligibility criteria, as shown in Figure 1. The publication year of included articles ranged from 2015 to 2024, with 5/11 (45.5%) having been published within 1 year of database searches [Table 1].
Study characteristics
Author | Year | Study design | Procedure domain(s) | Sample size | AI/ML/NLP tested | CPT codes assessed |
Cheng et al.[13] | 2024 | Retrospective cohort study | Head and neck surgery | 549 total operative notes | ML (bagging DT, RF DT, SVM, LR, NB), NLP (count vectorizer, TF-IDF, Word2Vec) | 6 total CPT codes (30520, 31255, 31267, 31276, 31288, and 61580) |
Isch et al.[14] | 2024 | Observational study | Craniofacial surgery | 20 procedures per model (15 complex procedures, 5 simple procedures) | LLM (Bard, Perplexity.AI, BingAI, ChatGPT 3.5, ChatGPT 4.0) | CPT codes for craniofacial surgery procedures (unspecified) |
O’Malley et al.[15] | 2024 | Comparative performance evaluation | Neurosurgery (endovascular, spinal, cranial procedures) | 30 total procedures | LLM [Bard, Perplexity.AI, BingAI, ChatGPT 3.5, ChatGPT 4.0, Google Search (control)] | Various CPT codes for each procedure, depending on the number of actions performed |
Tavabi et al.[10] | 2024 | Retrospective cohort study | Orthopedic surgery and sports medicine | 44002 operative notes | NLP (TF-IDF, Doc2Vec, Clinical-BERT), ML (SVM with RBF kernel) | 20100-29999 |
Zaidat et al.[16] | 2024 | Retrospective cohort study | Spine surgery | 922 operative notes | NLP [XLNet (generalized autoregressive pretraining method)] | 24 CPT codes (analysis limited to codes with at least 50 appearances in operative notes) |
Kim et al.[17] | 2023 | Retrospective cohort study | Spine surgery | 391 operative notes | Deep learning (bidirectional long short-term memory), ML (RF), NLP | 15 CPT codes (analysis limited to codes with at least 50 appearances in operative notes) |
Shost et al.[18] | 2023 | Retrospective cohort study | Spine surgery | 12239 operative notes | NLP (TensorFlow open-source package for Python) | CPT codes specific to 7 types of cervical spine surgery |
Zaidat et al.[19] | 2023 | Retrospective cohort study | Spine surgery | 50 operative notes | LLM (ChatGPT-4), ML | Various (most frequent codes include CPT 22551, 22552, 20931, 20936) |
Khaleghi et al.[7] | 2021 | Retrospective observational study | General surgery | 28,000 patients | RF classifier, CWR, TFIDF, levenshtein distance | 891 unique CPT codes in the full dataset |
Kim et al.[20] | 2020 | Retrospective cohort study | Spine surgery | 391 operative notes | Bidirectional long short-term memory network with attention (deep learning NLP algorithm) | 36 CPT codes with high performance |
Brat et al.[21] | 2015 | Retrospective cross-sectional study | Abdominal openings/closure | 92,886 patients | Word vector algorithm, GBT model | Quantity unspecified |
Studies were characterized by study design, for which a retrospective approach to investigation was observed in all studies. AI/ML/NLP methods employed exhibited heterogeneity between studies, with most investigator groups opting to cross-compare multiple technologies for accuracy in CPT code generation [Table 1]. A variety of subspecialties were found in the procedures examined, all possessing similarity to plastic and reconstructive surgery due to direct overlap in the domain of surgical practice or similarity in techniques such that analogous performance of AI/ML/NLP methods would be expected [Table 1].
All measures of accuracy and efficiency were analyzed in the context of their unique grouping of computational techniques [Table 2]. The risk of bias evaluated through quality assessment was minimized via data interpretation and presentation in the originating format following data extraction. Simpler models (coinciding with earlier studies), reliant on a less elaborate collection of methods, tended to perform better in terms of achieved accuracy, and specificity was almost always reported to be greater than sensitivity, independent of model choice [Table 2].
Reported accuracy and efficiency of CPT code assignment
AI method(s) employed (ML/NLP) | Specificity | Sensitivity | ROC values | Accuracy |
Bing AI[13,15] | 33.3% (endovascular) | 46.7% (simple), 20% (complex) | N/A | 40% |
ChatGPT 4.0[13,15] | 20% (simple), 40% (complex) | 33.3% (simple), 40% (complex) | N/A | 25% |
ChatGPT 3.5[13,15] | 26.7% (simple), 0% (complex) | 20% (simple), 60% (complex) | N/A | 20% |
RF[13,16] | 74%-92% | 74%-82% | N/A | 74%-84% |
NN[16] | N/A | N/A | N/A | 39%-54% |
SVM[10] | 0.918-1.00 | 0.594-0.897 | 0.422-0.967 | 0.650-0.884 |
LR[10,16] | 0.937-0.955 | 0.594-0.873 | 0.445-0.983 | 0.644-0.965 |
NB[10] | 0.571-0.981 | 0.594-0.806 | 0.513-0.981 | 0.636-0.973 |
GBT[18,21] | 92%-93% | 65%-67% | 0.89-0.90 | N/A |
DT[14] | 0.940-0.971 | 0.594-0.970 | 0.441-0.984 | 0.636-0.973 |
RF + CWR[14] | Improved by 8% | Improved by 8% | N/A | 82%-84% |
Text mining (ICD-9-CM, CPT codes)[10,13,18,19] | 93%-100% | 40%-100% | 0.76-0.99 | 0.98 |
TF-IDF[13,15] | 74%-100% | 74%-100% | N/A | 74%-76% |
BiLSTM[20] | 80% | 80% | 80% | 98% |
Overall trends in public perception concerning the role of generative AI and integrative technologies discussed in literature sources are illustrated in Figure 2.
Figure 2. Key advantages (green) and disadvantages (red) derived from the literature represented as presence (absolute count) in selected sources. Key advantages and disadvantages were defined as points biased toward the benefits or drawbacks of integration of AI, ML, or NLP, respectively. AI: Artificial intelligence; ML: machine learning; NLP: natural language processing.
Surgeon workflow, involving the cyclic relationship between billing and administrative tasks, AI, and patient care, is illustrated in Figure 3.
Figure 3. Model of the relationship between relevant interrelated facets of the surgeon’s workflow (outer), within which core responsibilities (including operative time, collaboration with others, obligations related to hospital management, and maintenance of education and field knowledge) can be found. The goals of workflow components are specified (arrow ends). The image was created using Biorender.com.
DISCUSSION
The epidemic of physician burnout reportedly ranges from 29% to 55% among physicians[22], frequently resulting in poor work-life balance, decreased career satisfaction, increased medical errors, and diminished quality of patient care[23]. Tasks such as procedural coding and other administrative obligations are often cited as reasons for dissatisfaction and stress among surgeons, contributing to burnout[22,24]. A 2020 study of surgeon self-reported activity, recorded in a smartphone app, found that US physicians across six specialties in 16 US states spent 7.7% of their time on administrative tasks, 20.7% of their time on electronic health record (EHR) input, and a total of 44.9% of their time utilizing EHR[25]. Surgeons are at an especially high risk for burnout, with billing associated with a 32% increased risk for burnout, according to the American College of Surgeons[23]. The time consumed by administrative tasks, including significant EHR input, detracts from patient care, and contributes to the misalignment between the expectations of a surgeon’s role and the day-to-day realities of rising administrative requirements[22].
Medical coding is a particularly time-consuming and expensive administrative process, with insurance and billing-related expenses comprising 62% of healthcare administrative costs[12]. However, improper codes have been shown to not only decrease physician pay but also lead to inaccurate or incomplete descriptions of patient encounters, which could lead to insurance denials and financial burdens that fall on the provider and patient[11]. In fact, nearly 12% of a physician’s net service revenue is spent covering the cost of administrative billing tasks[11]. The process of CPT code selection and relationship with relative value units remains both complex and critical[26], as the total monetary units for the 26 most common plastic surgery procedures have shown an average decrease of 1.55% in physician relative value units between 2010-2020[27].
One emergent factor influencing adequate reimbursement is the automation of CPT code assignment. For instance, one study within the insurance industry employed ML by integrating individual claim data of patients to enhance the precision of loss reserve estimates, which is vital to financial reporting by accelerating the timeframe for reimbursement and lowering the chance of billing errors[28]. In another example, CodaMetrix has previously been integrated into Epic systems, combining AI methodologies to reduce the workload of human coding. In fact, the CodaMetrix CMX Automate solution boasts a remarkable 60% reduction in coding costs, a 70% reduction in claims denials, and an acceleration of compensation processing (time to cash)[29].
We identified a severe sparsity of articles evaluating plastic surgery-specific AI-driven CPT code assignment, including an absence of codes commonly used in several subspecialties, such as cosmetics, hand surgery, or gender-affirming surgery. Of the 11 studies included, only four directly reported investigating CPT codes that overlapped with plastic surgery. The corresponding CPT codes included 30520, which may be used in the context of craniofacial surgery, 69990 for operation of a microscope used in microsurgery, 20932 for autografting, and 20930 for morselized allograft material and osteoinductive materials, applicable to craniofacial procedures. It is imperative to note that nearly half (n = 5, 45.5%) of the studies included in our review were published within 1 year of database query, which highlights the emergence of AI applications to billing and coding.
Related specialties, such as orthopedic and general surgery, possess some established, tested, and documented models that may serve as a starting point for plastic and reconstructive surgery to utilize[30]. A Text Mining model performed superiorly in the context of spine surgery, which contains overlap with soft tissue flap-based closure performed in spino-plastic procedures. Hand surgery would likewise serve as an ideal candidate setting for implementing these AI methods with respect to procedural overlap of hardware use. Hand surgeons grapple with basal thumb arthritis and carpal tunnel syndrome as two of the most frequently encountered conditions[31], exhibiting unique overlap between plastic surgery and orthopedics, in which the latter has demonstrated multiple NLP and ML technologies successfully integrated for medical coding[10].
Intriguingly, the combined metrics for CPT code efficacy explored in this review proved consistently high and statistically similar for several technologies. Support vector machine (SVM), logistic regression (LR), Naïve Bayes (NB), and decision tree (DT) models all showed area under the receiver operating curve (AUROC) performance values > 0.98, where 1.00 or 100% represents perfect discriminative capabilities between right and wrong CPT codes. The expansive variety of procedures within gender-affirming surgery, from breast and chest surgery to facial or genital reconstruction, and the corresponding diversity of CPT codes implicated[32] highlight a potential target for application of these AI models, which performed well, independent of the specialty-specific setting. Moreover, ChatGPT 4.0 with Bing accurately identified 75% of appropriate CPT codes for craniofacial procedures, indicating the potential for these tools to expedite code identification and enhance coding accuracy and efficiency[14], establishing a guideline for integration into other areas of the specialty.
While the evident advantages of these technologies may seem unanimously agreeable, a shared understanding of how their autonomous capabilities influence workflow within plastic and reconstructive surgery exclusively is less certain. Cosmetic surgery remains subject to debate concerning the incorporation of AI into medical coding practices, primarily due to the lack of insurance coverage for elective cosmetic and aesthetic procedures. In such contexts, the AI methods described may serve little purpose, as reimbursement may not be sought routinely, or at all, as with cash-pay practices where payment plans do not invoke CPT code usage. Furthermore, plastic and reconstructive surgery procedures that harness both reconstructive and cosmetic components display compatibility concerns, as any technology employed for billing management would need to accurately distinguish between CPT codes for requested reimbursement from those universally covered by the patient without the aid of medical insurance. The added complexity of split insurance coverage found abundantly in plastic surgery necessitates higher-order AI development using current models as a foundation to distinctly meet the needs of plastic and reconstructive surgeons.
Beyond initial implementation costs such as software licensing, hardware expenses, staff training, and data migration, ongoing costs for subscription fees, system upgrades, and technical support services must also be considered. For example, a 2011 study found that the cost of implementing a new EHR system for a five-physician practice in the state of Texas cost an estimated $162,000, with $85,500 in maintenance expenses in the first year[33]. Nonetheless, trending initiatives streamlined by healthcare technology companies, such as Epic Systems and Oracle Health, have incorporated generative AI into electronic medical record (EMR) systems. Just last year, Epic announced their collaboration with Microsoft to integrate large language model tools and AI into its EHR software; they additionally developed an AI tool with ambient voice technology to aid in charting progress notes directly into the EHR after a patient exam, a feature available at 186 organizations as of April 2023[34]. Yet to be reported, another area of future investigation could include evaluating differences in cost between implementing a new EHR system versus the cost of adding and integrating AI technology for administrative purposes into the existing EHR. A 2017 WinterGreen market shares report determined that as much as 88% of CPT code assignments for the purpose of billing and reimbursement could occur automatically without any need for human review[35]. This raises an important, yet unanswered question: How much money could a healthcare system save on administrative costs by implementing such technology and do these savings and trickle effects previously discussed justify the upfront investment?
In terms of data security, the effectiveness of AI models is highly dependent on the quality and representativeness of the training dataset: Poor-quality data can perpetuate existing biases, potentially leading to disparities in care and ill-representative reimbursement for services provided. The 2023 report from the Centers for Medicare and Medicaid Services on the Comprehensive Error Rate showed a 0.6% to 34.9% fee-for-service error rate, which was dependent on medical subspecialty[36]. Therefore, it is imperative to regard these novel integrative technologies as learning tools that must be trained prior to assisting with definitive tasks and responding with efficient solutions. This calls for collaboration and investment from the plastic surgery community to train such specialty-specific models and help ensure there are robust regulations in place[37,38].
These young methods for CPT coding also lend to the idea that such technology remains fallible to breaches in cybersecurity, which can include unauthorized access to protected patient information. Ensuring compliance with data privacy regulations like HIPAA is essential, which requires regulatory efforts by medical institutions and greater failsafe measures by medical coders and developers of healthcare AI[14,15]. Per prior recommendations for AI use in medical coding, regular audits and compliance checks should be established alongside increased AI integration, both during the initial stages of development and piloting, as well as throughout its long-term use, with scheduled checkpoints during routine use of these technologies[15]. Further, implementing encrypted data storage solutions and secure access protocols is recommended to mitigate potential security breaches[15].
This study possesses several important limitations involving sample size, potential biases, and study rigor. Due to the limited available literature that met eligibility criteria in this review, which resulted in a small sample size of AI applications, generalizability is restricted. Furthermore, only a subset of our studies demonstrated direct overlap in CPT codes, thus reducing the rigor of this work. Potential biases, exhibited by the lack of unsuccessful instances of pilot AI model applications, also portray an area of weakness. Nevertheless, this work strengthens a broader understanding of the current state of AI integration in billing and medical coding within comparable settings, highlighting a present gap in the current literature, as well as a feasible framework for understanding how current models could provide practical benefit to the tailored administrative needs of plastic surgeons.
With enhanced efficiency and accuracy established across multiple domains of surgical practice and prior work demonstrating high skill and procedural transferability[39], these same AI models applied in other contexts have the potential to interpret complex operative notes characteristic of this specialty for the purpose of CPT code assignment. Future work should embrace existing AI methods to assess their performance in the plastic surgery setting. As these technologies become more affordable and accessible, even integrated as a default extension of EMR systems nationally, it is imperative that plastic surgeons explore the functional role of AI in billing and administrative management. Plastic and reconstructive surgeons must strive to trial medical coding with AI, documenting the effects of training on large datasets and de-identified clinical operative notes to actively keep pace with technological innovation, mitigating burnout and improving the quality of surgical management.
DECLARATIONS
Acknowledgments
The authors appreciate the authors of both included peer-review studies and gray literature for the provision of their investigation and insight from which this review was cultivated.
Authors’ contributions
Conception and design: Landau MB, Mortell T, Chaffin AE
Administrative support: Landau MB, Schlosser A, Chaffin AE
Collection and assembly of data: Landau MB, Rosbrugh J, Rizzuto K
Data analysis and interpretation: Landau MB, Mortell T, Rosbrugh J, Rizzuto K
Manuscript writing: Landau MB, Rosbrugh J, Rizzuto K, Mortell T, Schlosser A, Chaffin AE
Manuscript editing: Landau MB, Mortell T, Schlosser A
Final approval of manuscript: Landau MB, Rosbrugh J, Rizzuto K, Mortell T, Schlosser A, Chaffin AE
Availability of data and materials
Data needed are available within the manuscript and publicly available online.
Financial support and sponsorship
None.
Conflicts of interest
All authors declared that there are no conflicts of interest.
Ethical approval and consent to participate
Not applicable.
Consent for publication
Not applicable.
Copyright
© The Author(s) 2025.
REFERENCES
1. Zhu C, Attaluri PK, Wirth PJ, Shaffrey EC, Friedrich JB, Rao VK. Current applications of artificial intelligence in billing practices and clinical plastic surgery. Plast Reconstr Surg Glob Open. 2024;12:e5939.
2. Esposito T, Reed R, Adams RC, Fakhry S, Carey D, Crandall ML. Acute care surgery billing, coding and documentation series part 1: basic evaluation and management (E/M), emergency department E/M, prolonged services, adult critical care documentation and coding. Trauma Surg Acute Care Open. 2020;5:e000578.
3. Dotson P. CPT® Codes: what are they, why are they necessary, and how are they developed? Adv Wound Care. 2013;2:583-7.
4. Venkatesh KP, Raza MM, Kvedar JC. Automating the overburdened clinical coding system: challenges and next steps. NPJ Digit Med. 2023;6:16.
5. Abràmoff MD, Roehrenbeck C, Trujillo S, et al. A reimbursement framework for artificial intelligence in healthcare. NPJ Digit Med. 2022;5:72.
6. Varnosfaderani S, Forouzanfar M. The role of AI in hospitals and clinics: transforming healthcare in the 21st century. Bioengineering. 2024;11:337.
7. Khaleghi T, Murat A, Arslanturk S. A tree based approach for multi-class classification of surgical procedures using structured and unstructured data. BMC Med Inform Decis Mak. 2021;21:328.
8. Xu HA, Maccari B, Guillain H, Herzen J, Agri F, Raisaro JL. An end-to-end natural language processing application for prediction of medical case coding complexity: algorithm development and validation. JMIR Med Inform. 2023;11:e38150.
9. Jarvis T, Thornburg D, Rebecca AM, Teven CM. Artificial intelligence in plastic surgery: current applications, future directions, and ethical implications. Plast Reconstr Surg Glob Open. 2020;8:e3200.
10. Tavabi N, Singh M, Pruneski J, Kiapour AM. Systematic evaluation of common natural language processing techniques to codify clinical notes. PLoS One. 2024;19:e0298892.
11. Blanchfield BB, Heffernan JL, Osgood B, Sheehan RR, Meyer GS. Saving billions of dollars - and physicians’ time - by streamlining billing practices. Health Aff. 2010;29:1248-54.
12. Tseng P, Kaplan RS, Richman BD, Shah MA, Schulman KA. Administrative costs associated with physician billing and insurance-related activities at an academic health care system. JAMA. 2018;319:691-7.
13. Cheng CP, Sicard R, Vujovic D, et al. Replicating current procedural terminology code assignment of rhinology operative notes using machine learning. World J Otorhinolaryngol Head Neck Surg. 2024.
14. Isch EL, Sarikonda A, Sambangi A, et al. Evaluating the efficacy of large language models in CPT coding for craniofacial surgery: a comparative analysis. J Craniofac Surg. 2024.
15. O’Malley GR Jr, Sarwar SA, Cassimatis ND, et al. Can publicly available artificial intelligence successfully identify current procedural terminology codes for common procedures in neurosurgery? World Neurosurg. 2024;183:e860-70.
16. Zaidat B, Tang J, Arvind V, et al. Can a novel natural language processing model and artificial intelligence automatically generate billing codes from spine surgical operative notes? Global Spine J. 2024;14:2022-30.
17. Kim JS, Vivas A, Arvind V, et al. Can natural language processing and artificial intelligence automate the generation of billing codes from operative note dictations? Global Spine J. 2023;13:1946-55.
18. Shost MD, Meade SM, Steinmetz MP, Mroz TE, Habboub G. Surgical classification using natural language processing of informed consent forms in spine surgery. Neurosurg Focus. 2023;54:E10.
19. Zaidat B, Lahoti YS, Yu A, Mohamed KS, Cho SK, Kim JS. Artificially intelligent billing in spine surgery: an analysis of a large language model. Global Spine J. 2023:21925682231224753.
20. Kim JS, Arvind V, Schwartz JT, et al. P72. Natural language processing of operative note dictations to automatically generate CPT codes for billing. Spine J. 2020;20:S181-2.
21. Brat G, Beam A, Salim A, Christopher K. 1160: Using machine learning algorithms to identify open abdomen procedures in administrative databases. Crit Care Med. 2015;43:291-2.
22. Khansa I, Janis JE. A growing epidemic: plastic surgeons and burnout - a literature review. Plast Reconstr Surg. 2019;144:298e-305e.
23. Streu R, Hansen J, Abrahamse P, Alderman AK. Professional burnout among US plastic surgeons: results of a national survey. Ann Plast Surg. 2014;72:346-50.
24. Holzer E, Tschan F, Kottwitz MU, Beldi G, Businger AP, Semmer NK. The workday of hospital surgeons: what they do, what makes them satisfied, and the role of core tasks and administrative tasks; a diary study. BMC Surg. 2019;19:112.
25. Toscano F, O’Donnell E, Broderick JE, et al. How physicians spend their work time: an ecological momentary assessment. J Gen Intern Med. 2020;35:3166-72.
26. Donaldson R, Hallman TG, Qureshi U, et al. Quantifying plastic and reconstructive surgery engagement in the evolution of ICD-10 codes. Plast Reconstr Surg Glob Open. 2024;12:e6304.
27. Siotos C, Cheah MA, Damoulakis G, et al. Trends of medicare reimbursement rates for common plastic surgery procedures. Plast Reconstr Surg. 2021;147:1220-5.
28. Baudry M, Robert CY. A machine learning approach for individual claims reserving in insurance. Appl Stoch Models Bus Ind. 2019;35:1127-55.
29.
30. Kiwan O, Al-Kalbani M, Rafie A, Hijazi Y. Artificial intelligence in plastic surgery, where do we stand? JPRAS Open. 2024;42:234-43.
31. Ankarath S. Navigating complications in hand surgery: a crucial discussion. J Hand Surg Eur Vol. 2024;49:139-41.
32. Wright JD, Chen L, Suzuki Y, Matsuo K, Hershman DL. National estimates of gender-affirming surgery in the US. JAMA Netw Open. 2023;6:e2330348.
33. Fleming NS, Culler SD, McCorkle R, Becker ER, Ballard DJ. The financial and nonfinancial costs of implementing electronic health records in primary care practices. Health Aff. 2011;30:481-9.
34. Landi H. Epic touts new AI applications to streamline charting and bring research insights to the point of care. 2024. Available from: https://www.fiercehealthcare.com/ai-and-machine-learning/epic-touts-new-ai-applications-streamline-charting-and-bring-research. [Last accessed on 3 Jan 2025].
35. Curtiss ET, Susan E. Computer assisted coding: market shares, strategies, and forecasts, worldwide 2016 to 2022. WinterGreen Res 2017. Available from: https://scholar.google.com/scholar?hl=en&as_sdt=0%2C5&q=Curtiss+E+T+SE.+Computer+Assisted+Coding%3A+Market+Shares%2C+Strategies%2C+and+Forecasts%2C+Worldwide+2016+to+2022.+WinterGreen+Research.+2017&btnG=. [Last accessed on 3 Jan 2025].
36. Centers for Medicare & Medicaid Services. Comprehensive error rate testing (CERT). 2023. Available from: https://www.cms.gov/data-research/monitoring-programs/improper-payment-measurement-programs/comprehensive-error-rate-testing-cert/. [Last accessed on 3 Jan 2025].
37. Ozmen BB, Schwarz GS. Future of artificial intelligence in plastic surgery: toward the development of specialty-specific large language models. J Plast Reconstr Aesthet Surg. 2024;93:70-1.
38. Spoer DL, Kiene JM, Dekker PK, et al. A systematic review of artificial intelligence applications in plastic surgery: looking to the future. Plast Reconstr Surg Glob Open. 2022;10:e4608.
Cite This Article
How to Cite
Download Citation
Export Citation File:
Type of Import
Tips on Downloading Citation
Citation Manager File Format
Type of Import
Direct Import: When the Direct Import option is selected (the default state), a dialogue box will give you the option to Save or Open the downloaded citation data. Choosing Open will either launch your citation manager or give you a choice of applications with which to use the metadata. The Save option saves the file locally for later use.
Indirect Import: When the Indirect Import option is selected, the metadata is displayed and may be copied and pasted as needed.
About This Article
Special Issue
Copyright
Data & Comments
Data
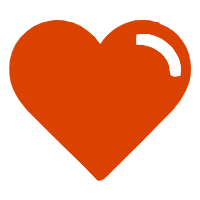
Comments
Comments must be written in English. Spam, offensive content, impersonation, and private information will not be permitted. If any comment is reported and identified as inappropriate content by OAE staff, the comment will be removed without notice. If you have any queries or need any help, please contact us at [email protected].