Emerging role of artificial intelligence in the care and management of lower extremity amputations and peripheral nerve injuries
Abstract
Lower limb amputation (LLA) secondary to trauma, oncologic, diabetic, and vascular disease represents a significant patient challenge in terms of restoring function to pre-injury levels. This can be secondary to wear and use of a prosthetic limb, as well as limitations in range of motion or chronic pain. This study aimed to review and discuss the available, and potentially soon-to-be-available, roles of artificial intelligence (AI) in extremity amputation care. Specifically, we discuss the current state of AI technology in LLA prevention, management, peripheral nerve injury treatment, and lower limb prosthesis design, as well as highlighting current advancements and the direction of these linked fields.
Keywords
INTRODUCTION
Artificial intelligence (AI) and machine learning (ML) offer the potential to improve treatments and outcomes among lower limb amputation (LLA) patients, with more than 150,000 patients in the United States each year[1]. Of these, over half are secondary to peripheral artery disease (PAD) and diabetes mellitus[2,3]. Chronic limb-threatening ischemia (CLTI), the most severe form of PAD, carries an estimated 1-year major limb amputation rate of 22%[4], and patients with concomitant PAD and diabetes have a four times higher risk of amputation compared to the national average[3,5]. In addition, various forms of oncologic management, severe trauma, and battlefield injuries are affected by LLA. The 5-year mortality rate for patients with index LLA is reported to be as high as 77%[6], especially with comorbid diseases such as diabetes mellitus. When compared to amputation above the ankle, limb free-flap reconstruction has been shown to significantly increase the 5-year survival rate (86.8% vs. 41.4%, P < 0.001)[7].
The conventional socket attachment of a prosthetic limb presents inherent functional limitations, and many of these limitations may remain chronic or present despite numerous treatments. Mechanical imbalance can contribute to difficulty with gait or even increased wear and osteoarthritis on the spine and contralateral lower extremity[8]. Even with an optimal soft-tissue envelope, changes in strength, tactile feedback, and range of motion can be limited. Relative motion between the residual limb and socket may also cause chronic pain, ulceration, and breakdown[9].
Another limiting factor following LLA contributing to decreased prosthetic use, increased rate of surgical revision or proximal amputation can be the various forms of neurogenic pain following amputation. Chronic post-amputation pain, including residual limb pain and/or phantom limb pain (PLP), limits function by interfering with the use of lower limb prosthesis[10-12]. Surgical methods such as targeted muscle reinnervation and regenerative peripheral nerve interfaces have led to improvements in both amputation-related pain symptoms and myoelectric prosthetic control[13-17]. Current autonomous lower limb prostheses can assist in cyclic gait; however, they lack versatility and anticipatory adjustment based on user input[18,19]. In the last decade, research on myoelectric lower limb prostheses has started to emerge[20,21], yet the literature lacks consensus on the methodology for electromyographic control of lower limb prostheses[18].
Novel strategies and technologies such as AI and ML are emerging to overcome the distinct challenges faced by patients with LLA. Herein, we present a scoping review describing how AI and ML can optimize diagnosis, treatment, and postoperative outcomes among patients with LLA. Further, we aimed to describe how AI and ML applications can improve peripheral nerve injury outcomes in this population.
EVALUATING THE ROLE OF AI
AI refers to the ability of computer systems to resemble human cognition in learning, synthesis, and perception of information. ML is a subset of AI in which algorithms can learn from data. ML is driven by mathematical models that are trained to yield optimized predictions based on a training dataset. There are two primary methods by which these models are trained. In supervised training, the algorithm learns from pre-labeled data known as the “ground truth”. In unsupervised training, the input data are not labeled, and the algorithm autonomously derives meaningful organization from the dataset. A subfield of ML known as “deep learning” (DL) employs multiple layers of artificial neural networks. This method allows for an increased level of abstraction and performance via convolutional neural networks (CNN) [Figure 1].
Figure 1. Simplified illustration of an artificial neural network divided into an input layer, a series of interconnected hidden layers that organize and process data, and an output layer. Created in BioRender. Jabbari, K. (2025) https://BioRender.com/9vvp8o3.
While basic science and translational research is well established in lower extremity amputation care, there are limited studies elucidating the direct application of AI in the field of LLA. Until recently, most AI extremity research had focused on hand and upper extremity amputations[22], though an understanding of prior applications can guide efforts to improve LLA outcomes. AI-assisted analysis of medical images is well established in the literature, including the interpretation of radiographs, electrocardiograms, magnetic resonance imaging (MRI) slices, and histopathological images[23-26].
AI in the prevention of LLAs
Although peripheral arterial disease is associated with LLA, rates of PAD diagnosis remain persistently low due to variable, atypical presentation[27]. As such, early diagnosis and staging may help attenuate poor management and amputation rates. Dai et al. recently developed a CNN for the analysis of lower extremity computed tomography angiograms and the classification of PAD[25]. Their CNN utilized 17,050 axial images to develop distinct classification systems for both above-knee and below-knee artery stenoses. Compared to the reference standard of digital subtraction angiography, the CNN model demonstrated an accuracy of greater than 90% across most stenosis classes.
Similar innovations have been made in MRI processing and analysis. Zhang et al. developed a model with accelerated interpretation of dynamic contrast-enhanced MRIs and mapping of calf muscle perfusion[26]. They created a feedforward neural network using pre- and post-exercise MRI scans from subjects with and without PAD. Compared to the reference standard of tracer kinetic analysis, the model produced comparable exercise-stimulated perfusion estimates and notably faster calf muscle perfusion maps. Similarly, another group assessed atherosclerosis of popliteal arteries with a CNN model, which reduced vessel wall segmentation times from an order of hours to only minutes[28,29].
Affordable and convenient PAD screening may offer significant benefits in various clinical settings. Kim
Additionally, timely detection of diabetic foot ulcers is critical in preventing LLA. Several reviews have reported on the application of AI in the diagnosis and treatment of diabetic foot[32,33]. A recent proof-of-concept study by Cassidy et al. demonstrated accurate diabetic foot ulcer detection with an AI system on smartphones[34]. A total of 203 photographs were taken by smartphone, analyzed by the AI system, and compared against expert clinical judgment. The predictions and decisions made by the AI system displayed high sensitivity (0.92) and specificity (0.86).
AI in patient management and clinical decision making
The application of AI in clinical decision making may revolutionize surgical practice through novel patient-centered approaches. Chung et al. used ML to generate an accurate risk prediction model for CLTI[35]. Their multicenter, nested study included clinical trial data from 1,238 patients undergoing infrainguinal vein bypass for the treatment of ischemic rest pain or ischemic tissue loss. Supervised topic model cluster analysis was able to differentiate three distinct clusters of patients within the nested cohort, each designated as a specific stage within CLTI. Cluster analysis revealed 1-year CLTI-free survival rates of 82.3% for stage 1, 61.1% for stage 2, and 53.4% for stage 3. Stratification by stage revealed major limb amputation rates of 4.2% for stage 1, 10.8% for stage 2, and 18.4% for stage 3. Among those without a major amputation, the rate of CLTI recurrence was directly related to increasing stage number. Similarly, Oei et al. developed ML algorithms to predict the risk of LLA in 2,559 patients with diabetic foot ulcers[36]. Their model performed well in the prediction of major [area under the receiver operating characteristic curve (AUROC): 0.820], minor (AUROC: 0.637), and any (AUROC: 0.756) LLA events. They further determined total white cell count, comorbidity score, and red blood cell count as key factors associated with the risk of major amputation. The above studies depict emerging methods for risk stratification and outcome prediction, highlighting the power of AI applications in surgical decision making.
The management of the mangled extremity represents yet another complex decision-making scenario. The decision for amputation or limb salvage will likely be innovated by AI models and replace traditional scoring systems[37]. Perkins et al. developed a Bayesian network (BN) prediction model using a supervised ML approach to estimate the outcome of limb revascularization, a metric often critical to attempting limb salvage versus amputation[38]. The prediction model sourced information from domain knowledge, published data, and US Department of Defense Data. Their model accurately predicted failed revascularization (AUROC: 0.95), with maintained performance on external validation (AUROC: 0.97). The BN prognostic model outperformed the traditional mangled extremity severity score in predicting the need for amputation [AUROC: 0.95 (0.92-0.98) vs. 0.74 (0.67-0.80); P < 0.0001].
Following the decision to perform a procedure, surgeons are often faced with postoperative patient complications. In general, the perioperative period serves as the source of initial exposure for many patients with chronic opioid use[39,40]. Using a ML approach, Gabriel et al. developed predictive models for persistent opioid use following lower extremity joint arthroplasty[41]. They demonstrated that ensemble learning can improve predictive models, as evidenced by the balanced bagging classifier with a F1 score of 0.80 and an AUC of 0.94. This model identified several important features, such as postoperative day 1 opioid use, body mass index, age, preoperative opioid use, prescribed opioids at discharge, and hospital length of stay. The identification of high-risk patients may guide clinical decisions and interventions.
Another postoperative challenge specific to amputation patients is the development of pain or sensation that originates from the absent, amputated limb, known as PLP. Ortiz-Catalan et al. showed that motor execution of the phantom limb via ML, augmented and virtual reality, and gaming may hold potential as a treatment for PLP[42]. Their cohort included fourteen patients with upper limb amputation and chronic intractable PLP. After the 12-session study period, a comparison of pre- and post-treatment PLP demonstrated significant decreases by 47% (P = 0.001) for weighted pain distribution, 32% (P = 0.007) for the numeric rating scale, and 51% (P = 0.0001) for the pain rating index. These findings further exemplify the potential role of AI applications in the evolution of treatment options for LLA patients.
AI in lower extremity nerve injuries
There is a need for innovative peripheral nerve injury strategies among LLA patients, as neurogenic pain secondary to hyperactive terminal neuroma formation is largely responsible for postoperative morbidity. In this effort, AI technologies can be used to understand the pathology of PNI and to better explore therapeutic approaches.
Such an approach has led to the development of new research methods and strategies for nerve regeneration. Romeo-Guitart et al. showed the power of therapeutic performance mapping system (TPMS) technology for the design of drug therapies promoting nerve regeneration and functional recovery after PNI[43]. TPMS develops mathematical models that simulate human physiology in silico, a process that is based on AI and pattern recognition models that source all available biological, medical, and pharmacological knowledge. A total of 5,400 drugs were screened, generating approximately 15 million binary drug combinations. After further screening, the team selected the top 3 binary combinations with more than 75% of potential regenerative capabilities. The neuroprotective effects of these drug combinations were then validated in in vitro and in vivo models. This strategy elucidated the therapeutic actions of combinatorial drug therapy with acamprosate plus ribavirin. Most importantly, the authors demonstrated the discovery of repurposed drug therapies with a network-centric approach, which uses ML tools to validate both efficacy and mechanism of action with preclinical in vivo models.
Additionally, large image datasets can be utilized by AI systems for rapid biomedical research. Daeschler
Beyond its role in drug therapy and image processing, AI has potential applications in the direct repair of PNI using 3D printing and biomaterials. Nerve guidance conduits (NGCs) have been widely explored for the treatment of PNI. Current research on functional NGCs attempts to create microenvironments that promote greater axonal elongation and myelination[45,46]. In this effort, ML modeling can significantly accelerate biomaterial experimentation by identifying optimal biochemical and biophysical properties from large datasets[47]. For instance, Li et al. developed a library of 2,000 peptide-based self-assembling hydrogels to identify optimal motifs for hydrogel self-assembly[48]. In another ML model of biomaterial synthesis, Kosuri et al. discovered chondroitinase ABC complexes that best retained enzymatic activity for neural regeneration applications[49]. Such AI-driven advances in NGC and biomaterial design may be applied to emerging strategies in lower extremity nerve repair and the parallel application of AI technology to nerve regenerative strategies has potential for revolutionary biotechnologies.
AI in lower extremity prosthetic use and design
The ability to stimulate and record signals from the peripheral nervous system (PNS) is an important component of new bioelectronic systems. In neurologically intact individuals, sensory signals from the lower limbs, such as tactile sensation in the foot and proprioception, influence motor output[50]. Traditional prostheses do not restore sensory feedback in amputees, which contributes to asymmetric gait, poor balance, risk of falls, and perception of the prosthesis as an external object (low embodiment)[50-53]. Several strategies have been employed to restore somatosensory feedback to lower extremity amputees[54-57]. Notably, advances in PNS interfacing represent a promising alternative to current neuromodulation modalities[58].
Direct interface with remaining nerves in the residual limb may restore the sensations necessary for human locomotion among patients with LLA[59,60]. Charkhkar et al. mapped elicitation sensations in transtibial amputees with implanted nerve cuff electrodes[61]. Neural stimulation was perceived by patients as originating from the missing limb, with discrete localization to missing toes, foot, and ankle, as well as the residual limb. These findings reflect the paradigm shift in prostheses development, where high-density cuff technology can be applied to neuroprosthesis with natural sensory feedback [Figure 2]. To this end, AI-driven methodology can be applied to the evolution of prosthesis development. Koh et al. used CNN to correlate signals from naturally evoked compound action potentials (CAPs) and neural pathways of interest[62]. Using a rat model, nerve cuff electrodes were implanted on the sciatic nerve and afferent activity was selectively evoked in different fascicles via mechanical stimuli. Based on the predicted firing patterns from the CNN, a recurrent neural network was used to predict joint angles. They showed high accuracy in CAP-based classification, which can track physiological measurements such as joint ankles. These results demonstrate the role of AI in the development of more effective neuroprosthetic systems.
Figure 2. Schematic of implanted nerve cuff electrode technology and potential application of AI to develop patient-specific devices via advanced imaging and computational power. Created in BioRender. Jabbari, K. (2025) https://BioRender.com/t0gdy9v.
Although promising, the above reports lacked prosthesis connection or functional assessment. This was addressed by Petrini et al., who utilized intraneural electrodes to develop a leg neuroprosthesis with real-time tactile and proprioception feedback through nerve stimulation[63]. Functional assessment showed improved mobility, fall prevention, and increased embodiment of the prosthesis. It has become evident that induced sensory feedback integration is an important component of care for LLA patients. As such, there is a need to optimize neural interface design. Zelechowski et al. developed a computational model of sciatic nerve behavior in response to electrical stimulation[64]. Their model reported optimal interfaces for use in humans and their surgical placement. The authors noted, however, that limitations in imaging technique and computational power precluded their ability to develop patient-specific devices. Instead, their study suggests indications for the use and design of these devices. This barrier represents yet another potential application of AI in the natural evolution of lower limb prostheses.
Osseointegration of prosthetic implants has recently emerged as a viable alternative to traditional socket prostheses, which are not always suitable for LLA patients[65]. Yet, to our knowledge, the application of AI technology to osseointegration strategies is not well studied outside the field of implant dentistry[66,67]. Lu
CONCLUSIONS
AI-based strategies complement clinical judgment and support innovations in lower extremity amputation care. In this scoping review, we described the current and emerging roles of AI in LLA prevention, management, peripheral nerve injury treatment, postoperative outcomes, and lower limb prosthesis design. AI as a methodology holds promise in revolutionizing the practice of LLA by way of computational analysis of large datasets. This feature of AI represents both an inherent strength and challenge in the field. Recent research has underscored that AI algorithms could be susceptible to security breaches[68]. Thus, the integration of AI into LLA care also necessitates comprehensive guidelines for secure use and safety. Nonetheless, our review suggests that the integration of AI in LLA is not only rapidly growing but is seemingly inevitable.
DECLARATIONS
Authors’ contributions
Made substantial contributions to the conception and design of the study and performed data analysis and interpretation: Jabbari K, Orfahli LM, Iorio ML
Availability of data and materials
Not applicable.
Financial support and sponsorship
None.
Conflicts of interest
All authors declared that there are no conflicts of interest.
Ethical approval and consent to participate
Not applicable.
Consent for publication
Not applicable.
Copyright
© The Author(s) 2025.
REFERENCES
1. Molina CS, Faulk J. Lower extremity amputation. StatPearls Publishing: 2024. Available from: https://www.ncbi.nlm.nih.gov/books/NBK546594/. [Last accessed on 22 Apr 2025].
2. Ziegler-Graham K, MacKenzie EJ, Ephraim PL, Travison TG, Brookmeyer R. Estimating the prevalence of limb loss in the United States: 2005 to 2050. Arch Phys Med Rehabil. 2008;89:422-9.
3. Goodney PP, Beck AW, Nagle J, Welch HG, Zwolak RM. National trends in lower extremity bypass surgery, endovascular interventions, and major amputations. J Vasc Surg. 2009;50:54-60.
4. Abu Dabrh AM, Steffen MW, Undavalli C, et al. The natural history of untreated severe or critical limb ischemia. J Vasc Surg. 2015;62:1642-51.e3.
5. Humphries MD, Brunson A, Li CS, Melnikow J, Romano PS. Amputation trends for patients with lower extremity ulcers due to diabetes and peripheral artery disease using statewide data. J Vasc Surg. 2016;64:1747-55.e3.
6. Fortington LV, Geertzen JH, van Netten JJ, Postema K, Rommers GM, Dijkstra PU. Short and long term mortality rates after a lower limb amputation. Eur J Vasc Endovasc Surg. 2013;46:124-31.
7. Oh TS, Lee HS, Hong JP. Diabetic foot reconstruction using free flaps increases 5-year-survival rate. J Plast Reconstr Aesthet Surg. 2013;66:243-50.
8. Gailey R, Allen K, Castles J, Kucharik J, Roeder M. Review of secondary physical conditions associated with lower-limb amputation and long-term prosthesis use. J Rehabil Res Dev. 2008;45:15-29.
9. Kuiken TA, Fey NP, Reissman T, Finucane SB, Dumanian GA. Innovative use of thighplasty to improve prosthesis fit and function in a transfemoral amputee. Plast Reconstr Surg Glob Open. 2018;6:e1632.
10. Herr HM, Clites TR, Srinivasan S, et al. Reinventing extremity amputation in the era of functional limb restoration. Ann Surg. 2021;273:269-79.
11. Sinha R, van den Heuvel WJ, Arokiasamy P. Factors affecting quality of life in lower limb amputees. Prosthet Orthot Int. 2011;35:90-6.
12. van der Schans CP, Geertzen JH, Schoppen T, Dijkstra PU. Phantom pain and health-related quality of life in lower limb amputees. J Pain Symptom Manage. 2002;24:429-36.
13. Reid RT, Johnson CC, Gaston RG, Loeffler BJ. Impact of timing of targeted muscle reinnervation on pain and opioid intake following major limb amputation. Hand. 2024;19:200-5.
14. Kuiken TA, Barlow AK, Hargrove L, Dumanian GA. Targeted muscle reinnervation for the upper and lower extremity. Tech Orthop. 2017;32:109-16.
15. Dumanian GA, Potter BK, Mioton LM, et al. Targeted muscle reinnervation treats neuroma and phantom pain in major limb amputees: a randomized clinical trial. Ann Surg. 2019;270:238-46.
16. Mauch JT, Kao DS, Friedly JL, Liu Y. Targeted muscle reinnervation and regenerative peripheral nerve interfaces for pain prophylaxis and treatment: a systematic review. PM R. 2023;15:1457-65.
17. Mohanty AJ, Cederna PS, Kemp SWP, Kung TA. Prophylactic regenerative peripheral nerve interface (RPNI) surgery in pediatric lower limb amputation patients. Ann Surg. 2024.
18. Fleming A, Stafford N, Huang S, Hu X, Ferris DP, Huang HH. Myoelectric control of robotic lower limb prostheses: a review of electromyography interfaces, control paradigms, challenges and future directions. J Neural Eng. 2021;18:041004.
19. Sup F, Bohara A, Goldfarb M. Design and control of a powered transfemoral prosthesis. Int J Rob Res. 2008;27:263-73.
20. Huang H, Kuiken TA, Lipschutz RD. A strategy for identifying locomotion modes using surface electromyography. IEEE Trans Biomed Eng. 2009;56:65-73.
21. Hargrove LJ, Simon AM, Young AJ, et al. Robotic leg control with EMG decoding in an amputee with nerve transfers. N Engl J Med. 2013;369:1237-42.
22. Keller M, Guebeli A, Thieringer F, Honigmann P. Artificial intelligence in patient-specific hand surgery: a scoping review of literature. Int J Comput Assist Radiol Surg. 2023;18:1393-403.
23. Komura D, Ishikawa S. Machine learning methods for histopathological image analysis. Comput Struct Biotechnol J. 2018;16:34-42.
24. Siontis KC, Noseworthy PA, Attia ZI, Friedman PA. Artificial intelligence-enhanced electrocardiography in cardiovascular disease management. Nat Rev Cardiol. 2021;18:465-78.
25. Dai L, Zhou Q, Zhou H, et al. Deep learning-based classification of lower extremity arterial stenosis in computed tomography angiography. Eur J Radiol. 2021;136:109528.
26. Zhang JL, Conlin CC, Li X, et al. Exercise-induced calf muscle hyperemia: rapid mapping of magnetic resonance imaging using deep learning approach. Physiol Rep. 2020;8:e14563.
27. McDermott MM. Lower extremity manifestations of peripheral artery disease: the pathophysiologic and functional implications of leg ischemia. Circ Res. 2015;116:1540-50.
28. Hippe DS, Balu N, Chen L, et al. Confidence weighting for robust automated measurements of popliteal vessel wall magnetic resonance imaging. Circ Genom Precis Med. 2020;13:e002870.
29. Chen L, Canton G, Liu W, et al. Fully automated and robust analysis technique for popliteal artery vessel wall evaluation (FRAPPE) using neural network models from standardized knee MRI. Magn Reson Med. 2020;84:2147-60.
30. Kim S, Hahn JO, Youn BD. Detection and severity assessment of peripheral occlusive artery disease via deep learning analysis of arterial pulse waveforms: proof-of-concept and potential challenges. Front Bioeng Biotechnol. 2020;8:720.
31. Allen J, Liu H, Iqbal S, Zheng D, Stansby G. Deep learning-based photoplethysmography classification for peripheral arterial disease detection: a proof-of-concept study. Physiol Meas. 2021;42:054002.
32. Chemello G, Salvatori B, Morettini M, Tura A. Artificial intelligence methodologies applied to technologies for screening, diagnosis and care of the diabetic foot: a narrative review. Biosensors. 2022;12:985.
33. Howard T, Ahluwalia R, Papanas N. The advent of artificial intelligence in diabetic foot medicine: a new horizon, a new order, or a false dawn? Int J Low Extrem Wounds. 2023;22:635-40.
34. Cassidy B, Hoon Yap M, Pappachan JM, et al. Artificial intelligence for automated detection of diabetic foot ulcers: a real-world proof-of-concept clinical evaluation. Diabetes Res Clin Pract. 2023;205:110951.
35. Chung J, Freeman NLB, Kosorok MR, Marston WA, Conte MS, McGinigle KL. Analysis of a machine learning-based risk stratification scheme for chronic limb-threatening ischemia. JAMA Netw Open. 2022;5:e223424.
36. Oei CW, Chan YM, Zhang X, et al. Risk prediction of diabetic foot amputation using machine learning and explainable artificial intelligence. J Diabetes Sci Technol. 2024:19322968241228606.
37. Tjardes T, Marche B, Imach S. Mangled extremity: limb salvage for reconstruction versus primary amputation. Curr Opin Crit Care. 2023;29:682-8.
38. Perkins ZB, Yet B, Sharrock A, et al. Predicting the outcome of limb revascularization in patients with lower-extremity arterial trauma: development and external validation of a supervised machine-learning algorithm to support surgical decisions. Ann Surg. 2020;272:564-72.
39. Soffin EM, Lee BH, Kumar KK, Wu CL. The prescription opioid crisis: role of the anaesthesiologist in reducing opioid use and misuse. Br J Anaesth. 2019;122:e198-208.
40. Lawal OD, Gold J, Murthy A, et al. Rate and risk factors associated with prolonged opioid use after surgery: a systematic review and meta-analysis. JAMA Netw Open. 2020;3:e207367.
41. Gabriel RA, Harjai B, Prasad RS, et al. Machine learning approach to predicting persistent opioid use following lower extremity joint arthroplasty. Reg Anesth Pain Med. 2022;47:313-9.
42. Ortiz-Catalan M, Guðmundsdóttir RA, Kristoffersen MB, et al. Phantom motor execution facilitated by machine learning and augmented reality as treatment for phantom limb pain: a single group, clinical trial in patients with chronic intractable phantom limb pain. Lancet. 2016;388:2885-94.
43. Romeo-Guitart D, Forés J, Herrando-Grabulosa M, et al. Neuroprotective drug for nerve trauma revealed using artificial intelligence. Sci Rep. 2018;8:1879.
44. Daeschler SC, Bourget MH, Derakhshan D, et al. Rapid, automated nerve histomorphometry through open-source artificial intelligence. Sci Rep. 2022;12:5975.
45. Huang Y, Wu W, Liu H, et al. 3D printing of functional nerve guide conduits. Burns Trauma. 2021;9:tkab011.
46. Xiao B, Feturi F, Su AA, et al. Nerve wrap for local delivery of FK506/tacrolimus accelerates nerve regeneration. Int J Mol Sci. 2024;25:847.
47. Guo JL, Januszyk M, Longaker MT. Machine learning in tissue engineering. Tissue Eng Part A. 2023;29:2-19.
48. Li F, Han J, Cao T, et al. Design of self-assembly dipeptide hydrogels and machine learning via their chemical features. Proc Natl Acad Sci U S A. 2019;116:11259-64.
49. Kosuri S, Borca CH, Mugnier H, et al. Machine-assisted discovery of chondroitinase ABC complexes toward sustained neural regeneration. Adv Healthc Mater. 2022;11:e2102101.
50. Miller WC, Speechley M, Deathe AB. Balance confidence among people with lower-limb amputations. Phys Ther. 2002;82:856-65.
51. Miller WC, Speechley M, Deathe B. The prevalence and risk factors of falling and fear of falling among lower extremity amputees. Arch Phys Med Rehabil. 2001;82:1031-7.
52. Blanke O. Multisensory brain mechanisms of bodily self-consciousness. Nat Rev Neurosci. 2012;13:556-71.
53. Jaegers SM, Arendzen JH, de Jongh HJ. Prosthetic gait of unilateral transfemoral amputees: a kinematic study. Arch Phys Med Rehabil. 1995;76:736-43.
54. Crea S, Cipriani C, Donati M, Carrozza MC, Vitiello N. Providing time-discrete gait information by wearable feedback apparatus for lower-limb amputees: usability and functional validation. IEEE Trans Neural Syst Rehabil Eng. 2015;23:250-7.
55. Fan RE, Culjat MO, King CH, et al. A haptic feedback system for lower-limb prostheses. IEEE Trans Neural Syst Rehabil Eng. 2008;16:270-7.
56. Dietrich C, Nehrdich S, Seifert S, et al. Leg prosthesis with somatosensory feedback reduces phantom limb pain and increases functionality. Front Neurol. 2018;9:270.
57. Crea S, Edin BB, Knaepen K, Meeusen R, Vitiello N. Time-discrete vibrotactile feedback contributes to improved gait symmetry in patients with lower limb amputations: case series. Phys Ther. 2017;97:198-207.
59. Tan DW, Schiefer MA, Keith MW, Anderson JR, Tyler J, Tyler DJ. A neural interface provides long-term stable natural touch perception. Sci Transl Med. 2014;6:257ra138.
60. Davis TS, Wark HA, Hutchinson DT, et al. Restoring motor control and sensory feedback in people with upper extremity amputations using arrays of 96 microelectrodes implanted in the median and ulnar nerves. J Neural Eng. 2016;13:036001.
61. Charkhkar H, Shell CE, Marasco PD, Pinault GJ, Tyler DJ, Triolo RJ. High-density peripheral nerve cuffs restore natural sensation to individuals with lower-limb amputations. J Neural Eng. 2018;15:056002.
62. Koh RGL, Balas M, Nachman AI, Zariffa J. Selective peripheral nerve recordings from nerve cuff electrodes using convolutional neural networks. J Neural Eng. 2020;17:016042.
63. Petrini FM, Valle G, Bumbasirevic M, et al. Enhancing functional abilities and cognitive integration of the lower limb prosthesis. Sci Transl Med. 2019;11:eaav8939.
64. Zelechowski M, Valle G, Raspopovic S. A computational model to design neural interfaces for lower-limb sensory neuroprostheses. J Neuroeng Rehabil. 2020;17:24.
65. Hebert JS, Rehani M, Stiegelmar R. Osseointegration for lower-limb amputation: a systematic review of clinical outcomes. JBJS Rev. 2017;5:e10.
66. Lu L, Zhang J, Guan K, Zhou J, Yuan F, Guan Y. Artificial neural network for cytocompatibility and antibacterial enhancement induced by femtosecond laser micro/nano structures. J Nanobiotechnology. 2022;20:365.
67. Revilla-León M, Gómez-Polo M, Vyas S, et al. Artificial intelligence applications in implant dentistry: a systematic review. J Prosthet Dent. 2023;129:293-300.
Cite This Article

How to Cite
Download Citation
Export Citation File:
Type of Import
Tips on Downloading Citation
Citation Manager File Format
Type of Import
Direct Import: When the Direct Import option is selected (the default state), a dialogue box will give you the option to Save or Open the downloaded citation data. Choosing Open will either launch your citation manager or give you a choice of applications with which to use the metadata. The Save option saves the file locally for later use.
Indirect Import: When the Indirect Import option is selected, the metadata is displayed and may be copied and pasted as needed.
About This Article
Special Issue
Copyright
Data & Comments
Data
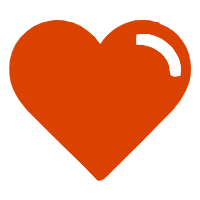
Comments
Comments must be written in English. Spam, offensive content, impersonation, and private information will not be permitted. If any comment is reported and identified as inappropriate content by OAE staff, the comment will be removed without notice. If you have any queries or need any help, please contact us at [email protected].